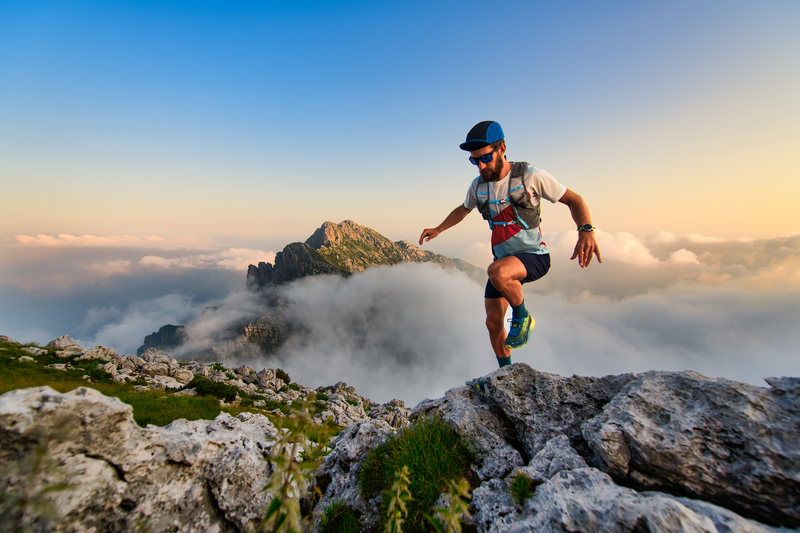
95% of researchers rate our articles as excellent or good
Learn more about the work of our research integrity team to safeguard the quality of each article we publish.
Find out more
ORIGINAL RESEARCH article
Front. Fish Sci. , 15 January 2025
Sec. Fish Experimental Biology
Volume 2 - 2024 | https://doi.org/10.3389/frish.2024.1508746
This article is part of the Research Topic Patterns, causes and consequences of intraspecific variation in environmental tolerance in fishes View all 4 articles
Introduction: The capacity of species and populations to respond to rapid environmental change will be pivotal for their resilience in the coming decades. To explore thermal plasticity, physiological trade-offs, and associations with environmental characteristics, we assessed the heat hardening response and acclimation capacity of juvenile Chinook salmon (Oncorhynchus tshawytscha) from seven hatchery populations spanning diverse ecoregions along the West Coast of the United States.
Methods: Progeny from each population were acclimated to one of three temperatures (11, 16, or 20°C) and subjected to two acute thermal maximum (CTMAX) trials 24 h apart. The heat hardening response (ΔCTM) was calculated as the difference between an individual's second and first CTMAX trials. Acclimation capacity was determined as the maximum difference in CTMAX between fish acclimated to 11°C and 20°C during their second trial, capturing the full acclimatory scope. Bayesian models were employed to test the thermal trade-off hypothesis, which posits that individuals or populations with higher thermal tolerance have reduced capacity for acclimation. Trade-offs were analyzed at both individual and population scales. Associations between ΔCTM, acclimation capacity, and source population environmental characteristics were also examined using data from a landscape-scale, regional, river temperature model.
Results: Fish acclimated to 16°C or 20°C exhibited heat hardening (positive ΔCTM), while those acclimated to 11°C exhibited “heat weakening” (negative ΔCTM), a rare phenomenon in the literature. At the individual level, fish adhered to the thermal trade-off hypothesis, with higher thermal tolerance associated with reduced plasticity. However, no such trade-off was observed at the population level. Acclimation capacity was positively correlated with latitude and the temperature of the natural rearing environment. Additionally, ΔCTM performance improved when fish were acclimated to temperatures closer to those of their natural habitats.
Discussion: Individual fish exhibited a trade-off between thermal tolerance and plasticity, while populations did not. Thermal plasticity was associated with environmental conditions, with populations from warmer habitats demonstrating greater acclimation and heat hardening capacities. This study highlights the importance of understanding interpopulation variation to identify at-risk populations, such as those from colder habitats or with limited thermal plasticity, like the Trinity population. These findings emphasize the need to account for interpopulation differences when predicting species responses to climate change and developing conservation strategies.
Rapid environmental change is confronting populations and species with novel abiotic conditions such as changes in thermal regimes and overall habitat warming. Of particular concern are increases in the severity and frequency of heat waves (2). To contend with increasing temperature, organisms, particularly ectotherms (e.g., fish) must have physiologies capable of acclimating to the increased temperatures or the ability to move to more physiologically viable habitats (3, 4). Given the rapid pace of environmental change, adaptation should not be considered a likely path for species or populations contending with climate change (5, 6). This is particularly true for species of conservation concern, as low abundances limit the genetic diversity that facilitates adaptive change (3). Additionally, movement to track suitable temperatures may not be a viable strategy for vagile organisms that are otherwise geographically bounded (e.g., fish living in freshwater lakes, rivers or estuaries), that have specific geographical locations critical to their life history (e.g., migratory species that converge at an essential stopover location or have constricted breeding grounds), or are constrained by other factors that inhibit movement (e.g., migration barriers, predation risk, food availability). Therefore, resiliency to future environmental warming may hinge on an organism's phenotypic plasticity as well as its capacity to physiologically acclimate to tolerate thermal challenges (7–9).
Mechanisms of thermal acclimation and adaptation have both been shown to underlie organismal thermal tolerance (79). Correspondingly, local differences in the thermal landscape have resulted in interpopulation variation in thermal physiology through patterns such as counter-gradient variation (11–13) and local adaptation (14–17). However, in the case of rapid environmental change and increasing frequency of stochastic thermal events [e.g., long-term droughts (18), episodic heat waves (2)], an organisms' physiological plasticity will likely better predict a population's persistence through thermal challenges compared to its adaptive capacity (19). Therefore, understanding the intraspecific variation in thermal acclimation informs our predictions of populations and species persistence through rapid environmental change.
Rapid environmental change can result in increased frequency and magnitude of exposure to supra-optimal temperatures. Therefore, understanding how fish respond to acute thermal challenges is important in determining climate change impacts. ‘Heat Hardening‘ describes the phenomena by which an organism exposed to an initial thermal shock will improve their thermal tolerance in response to a second thermal shock, typically occurring over a short period of time (i.e., hours to days) (20). A heat hardening response is not cost-free [e.g., synthesis of heat shock proteins, (10, 21)] and therefore the ability for organisms to mount a heat-hardening response has been theorized to reflect the evolutionary and acclimatization history of organisms. For instance, intertidal organisms, which are exposed to a tidally fluctuating thermal environment, exhibit improved thermal tolerance when acclimated to a variable thermal regime instead of a static one (22–24). If hardening serves an adaptive purpose, then understanding a population's capacity for heat hardening is important to assessing its resilience to episodic warm extremes that will increase with future environmental warming.
One hypothesis regarding the plasticity of acute thermal tolerance posits that a trade-off exists between the upper thermal tolerance of an organism and its capacity to acclimate, such that populations or individuals with relatively high baseline thermal tolerance will exhibit reduced capacity to acclimate to future change (25, 26). The trade-off hypothesis (TOH) has found support in a broad array of ectothermic organisms including fish (27–29); however, there are recent critiques which find some evidence of the TOH to be a statistical artifact (30). Regardless, understanding the interpopulation variation in acclimation capacity and response to thermal challenges is of particular concern to threatened species that may lack the adaptive potential to respond to environmental change as well as the geographic flexibility to locate to more favorable habitats, such as Pacific salmonids (Oncorhynchus sp.).
Pacific salmonids are a clade of economically-important, anadromous cold-water fishes at risk to both climate change and rapid anthropogenic landscape change. These fishes are constrained spatially, reliant on thermally suitable migratory pathways to complete their life cycle, and temporally, as migration timing is under at least partial genetic control and occurs at specific times of the year (31). Furthermore, impassable dams in many watersheds block passage to high-elevation habitat, such that many populations cannot access suitable habitat. Habitat loss associated with dam construction was the original driver for hatchery supplementation of impacted populations, where embryos and juveniles are reared in artificial hatchery environments prior to being released into the river as smolts (i.e., large juveniles physiologically capable of entering the ocean). Hatchery practices can genetically, behaviorally, and phenotypically modify populations within a single generation (32), and hatchery-reared salmon often have lower rates of survival than wild fish (33). For Chinook salmon (O. tshawytscha) populations from California, Oregon and Washington (USA), hatchery-sourced fish may represent upwards of 90% of the ocean rearing adult population (80), but both hatchery- and wild-spawned individuals must contend with anthropogenically modified river flows and altered thermal regimes. Experimental studies from other salmonids have demonstrated that ancestral thermal history alongside juvenile rearing environments can have transgenerational impacts on juvenile thermal performance (34, 35), demonstrating the importance of assessing ancestral thermal regimes as well as modern rearing temperatures to understand thermal physiological responses.
Increasing temperatures of freshwater habitats pose a considerable risk to salmonids as multiple life stages utilize freshwater ecosystems and are therefore exposed to warming water temperatures (36). Phenology and thermal exposures can vary by population, such that responses to climate change will vary locally (1, 37). Compounding these local thermal experiences is the genetic isolation of salmonid populations due to the natal homing of adult spawning migrations from the ocean to the freshwater reaches of their birth. Past work on salmonids has demonstrated interpopulation variation in physiological traits, hypothesized to reflect local adaptation among interspecific populations (17, 38–40, 66). Zillig et al. (17) found that fish from populations with historically warmer temperatures elicited higher CTMAX specifically when acclimated to warm water conditions (20°C). This supports the idea that the acute tolerances of Chinook salmon populations may be locally adapted to their native habitats. Understanding differences between salmonid populations will be integral to effectively managing and conserving a portfolio of diverse populations (41, 42) and may enable identification of climate resilient or at-risk populations.
We quantified the magnitude of the heat-hardening response among several juvenile Chinook salmon populations to determine if a population's capacity to acclimate is consistent with local adaptation or if there is a tradeoff between absolute tolerance and ability to improve thermal tolerance (i.e., TOH). In doing so, we explored associations between a population's heat hardening response and acclimation capacity with metrics of the population's current and historical thermal environment. If populations are locally adapted then populations from warmer habitats and acclimated to warmer temperatures should elicit a greater heat hardening response than those populations from cooler habitats, or when they are acclimated to cooler temperatures. If a tradeoff exists between thermal tolerance and acclimation capacity, then we would expect populations from warmer habitats or warm-acclimated fish to have greater absolute thermal tolerance but a reduced heat-hardening response in comparison to those from cooler habitats or cold-acclimated fish. Our work helps to predict population-level responses to the increasing frequency and magnitude of episodic warm events expected from climate change, which in turn helps prioritize conservation and management actions for populations that will have difficulty tracking suitable thermal habitat spatially or temporally.
In this experiment we tested seven hatchery-sourced populations of juvenile Chinook salmon from the states of Washington, Oregon and California in the United States of America (Figure 1). Of the seven populations, five exhibit the Fall-run migratory phenotype [Priest Rapids (WA), Trask (OR), Elk River (OR), Trinity (CA), Feather Fall-Run (CA)]. Adult Fall-run Chinook salmon return to freshwater during the Fall months and spawn shortly after arrival to their spawning grounds (43). Embryos and alevin incubate through the winter months and the young predominantly outmigrate during their first spring. Additionally, we tested two populations of early migrating Chinook salmon from California, the threatened Central Valley Spring-run from the Feather River hatchery and the critically endangered Sacramento River Winter-run from the Livingston Stone hatchery. Both populations exhibit early migration with adults entering freshwater during either the spring or winter months, respectively (43, 44). Both populations historically migrated to high elevation headwaters. Spring-run accessed snow-fed rivers in the Sierra Nevada and Southern Cascades while the Winter-run, endemic to northern California, migrated to cold spring-fed rivers in the upper Sacramento River watershed. Components of this research as well as greater detail on these studied populations has been published in (17, 66).
Figure 1. Locations of the seven Chinook salmon hatchery populations in our study shown against the currently accessible Chinook salmon range (gray) and historically occupied but now inaccessible range (dark gray). The current (orange) and historic (light blue) natal grounds for each hatchery population analyzed in this study are shown alongside major migration rivers. Evolutionary Significant Units in this study are emphasized. Populations include the Priest Rapids population from Washington, the Trask and Elk River populations from Oregon and the Trinity, Winter-run, Feather River Spring- and Feather River Fall-runs from California. Figure adapted from Zillig et al. (17).
Chinook salmon smolts (N = 470, mass 22.7 ± 4.4 g; fork length 12.4 ± 0.8 cm; μ ± S.D., n = 46–61 per population) from seven hatchery populations (Figure 1) were acclimated to one of three temperatures (11, 16, or 20°C), for a total of 21 treatment groups (n = 8–22 per treatment group) following methods in Zillig et al. (17). Temperatures were chosen to be ecologically relevant to the rearing and outmigration conditions that a naturally-spawned juvenile Chinook salmon may encounter (37). Experiments were conducted between 2017 and 2019 with two populations assessed in 2017 and 2018 and three in 2019. All experiments were conducted in accordance with the Institutional Animal Care Committee of UC Davis Protocol # 19928.
The studied populations (Figure 1) came from seven hatcheries in six defined Chinook salmon evolutionary significant units [ESU, (45), Trask and Elk River populations share an ESU]; each ESU is a conservation unit managed as a distinct taxonomic unit (46). Hatcheries strip spawned returning adults and incubated the fertilized embryos in systems using natural surface water from each populations resident watershed. We received progeny as either eyed embryos or young fry. Eyed eggs from the Priest Rapids population were received via overnight mail, surface sterilized with iodophor upon arrival, and incubated at 9°C until they hatched and began to feed. Fish from all other populations were acquired from their respective hatcheries when of transportable size (~1–2 g) and trucked to the Center for Aquatic Biology and Aquaculture (CABA) at the University of California, Davis campus in a 765 L tank. Dissolved oxygen was maintained through controlled bubbling of compressed air and water temperatures (7–11°C) were reduced with bagged ice as needed during transit. At CABA, rearing and treatment tanks were supplied with temperature-controlled, flow-through fresh water from a dedicated well and continuously aerated with air stones. Collected and hatched fry from all populations were reared at 11–13°C until distributed into their acclimation treatment tanks (2–89 days depending on population and size at acquisition). Each treatment group (population × acclimation temperature) was housed in two, replicate, 400 L, with 55–105 fish per tank. Fish were exposed to natural photoperiods (38°55′N) and automated belt feeders delivered an excess ration (4% of body mass per day) of 2–4 mm Sinking Salmon Feed (Skretting, USA) during daylight hours. Rations were updated biweekly to account for fish growth and tank density. Acclimation temperatures were achieved by adjusting tank temperature by ~1.5°C per day. Once tanks achieved their specific acclimation temperature, fish were acclimated for at least 60 days prior to any CTMAX trials. Mean tank temperatures (± SEM) were 11.1 ± 0.02°C (n = 14), 16.0 ± 0.03°C (n = 14) and 19.8 ± 0.03°C (n = 14). Tank temperatures were maintained for the duration of the experiments (4–9 months) and fish were tested once they reached the target size (~20 g). As fish growth is temperature dependent [see (17) for growth rates], fish acclimated to 20 and 16°C were acclimated to fewer days (average acclimation: 118 and 119 days, respectively) than fish acclimated to 11°C (150 days) prior to their exposure.
Critical thermal maxima (CTMAX) trials were conducted according to established methods (47) to determine a fish's maximum acute thermal tolerance. Fish were tested individually, each in their own beaker. Six or seven 4L Pyrex beakers were contained within a fiberglass bath (1 × 2 × 0.2 m) and individually aerated with an air stone to ensure normoxic conditions throughout the trial. The volume of water in each beaker (~2.5 L) was calibrated to ensure even heating across all CTMAX beakers (0.33°C min−1). Two pumps (PM700, Danner, USA) were used to circulate water: one pump moved water across three heaters (Process Technology S4229/P11), and the other recirculated heated water through the CTMAX bath via a submerged manifold. Bath temperature for each trial began at the acclimation temperatures (11, 16, or 20°C) of the tested fish. Prior to CTMAX trials, fish were transferred from treatment tanks to separate tanks for fasting. Fish reared at 20 and 16°C were fasted for 24 h and 11°C fish were fasted for 48 h to account for their slower metabolic rate and ensure they were in a fasted state. After fasting, individual fish (n = 8 to 22 per treatment, 22.72 ± 4.40 g mean ± S.D.) were gently netted and placed into individual chambers within the CTMAX bath. After a 30-min acclimation period (48), the CTMAX trial began.
The test chamber temperature was recorded every 5 min using a thermocouple (Omega HH81A) routinely calibrated to a standardized mercury thermometer. Fish were continually observed for signs of distress and loss of equilibrium (LOE). When a fish lost equilibrium the CTMAX trial was concluded and the temperature of the CTMAX beaker was recorded (15, 49). The fish were then immediately transferred to a recovery bath at their acclimation temperature where they were individually housed without food. The following day (μ ± S.D.: 24 h 12 m ± 1 h 1 m, range: 22 h 2 m−26 h 55 m) fish were retested using the same protocol and placed within the same test chamber to measure their heat-hardened CTMAX. Once completed, fish were again returned to their recovery chambers for ~24 h. After the final 24-h recovery period, fish were humanely euthanized in a buffered solution of MS-222 (0.5 g L−1 buffered with 0.42 g L−1 of NaHCO3 and 6.0 g L−1 of NaCl) and then weighed (wet mass ± 0.01 g) and measured (fork length ± 0.1 cm).
A total of 470 fish were tested for heat hardening, but fish whose trials were impacted by experimenter error (n = 11), exhibited unusual behavior (n = 8, discussed further in results) or did not recover from either the first (n = 33) or second (n = 33) CTMAX trial were not included in the analysis, resulting in 385 fish that recovered within 24 h from both the first and second CTMAX trial. We quantified the heat hardening response (ΔCTM) as the difference between an individual fish's second CTMAX trial and its first, with positive ΔCTM values indicating an increase in CTMAX from the first trial to the second. Gunderson (30) highlights the possibility of spurious thermal trade-off results (30) due to regression to the mean (RTM). If the correlation of two datasets is not perfect, then there will be RTM. In the case of repeated CTMAX trials RTM would be expected to yield results where fish with higher initial CTMAX will exhibit a relatively worse performance on their 2nd CTMAX trial then fish with initially poor CTMAX values. Taken collectively, this result would mimic a thermal trade-off, where the plastic response (i.e., ΔCTM) of fish or populations with high CTMAX values is less than that of fish or populations with low CTMAX values. We adjusted a fish's second CTMAX value using the RTM correction to adjust CTMAX data. Put briefly, this statistical transformation approach assesses the change in variance in CTMAX values between the first and second trials to calculate the strength of RTM effect [please refer to Kelly and Price (78) for the mathematical theory and Gunderson (30) for its application to CTMAX data from multiple ectothermic species]. We investigated thermal trade-offs at two scales. The first was at the organismal scale where each fish's ΔCTM value was compared against its first to test for a tradeoff among treatment groups. We then investigated whether populations with a higher initial CTMAX exhibited a reduced ΔCTM by using the model estimated mean values for each treatment (population × acclimation temperature).
We tested for relationships between physiological performance and seven environmental parameters including population latitude and six stream temperature metrics (Table 1). Population latitude, a common metric for assessing biogeographic patterns in physiology (12, 15), was defined by the location of each population's hatchery using Google Earth. Stream temperature metrics were estimated from the predictions of a spatial stream network (SSN) model (37, 50). The SSN model is a tailored regression model that incorporates the complex spatial autocorrelation of streams due to the direction of flow and nested connectivity. The SSN model accurately predicted stream temperatures across the western U.S. throughout the year (R2 = 0.928 for the out-of-sample testing dataset), and mean monthly stream temperatures were predicted for 465,775 river km in the western U.S. (37). We extracted mean monthly stream temperature predictions from the SSN (average of, 2002–2011) for each river kilometer within a population's current natural (i.e., not the hatchery) and historical natal distribution (Figure 2). Juvenile natal rearing habitat was defined for each population from observed spatial distributions of natural spawning (37). To estimate each population's historical pre-dam thermal regime, we defined potential rearing habitat upstream of dams until habitats were bounded by river slope, flow, natural barriers, or intermittency (37, 50–52). From this dataset we calculated the annual maximum mean monthly temperature (AMAX), the maximum mean monthly temperature during the rearing period (RMAX), and the thermal range between the annual maximum and minimum mean monthly temperatures (ARANGE). These were quantified from current (-Cur) and historical (-His) ranges (e.g., Historical annual maximum mean monthly temperature: AMAX-His). For greater detail see description in (17).
Table 1. Environmental predictors used in models detecting associations between physiological traits and environmental parameters.
Figure 2. Modeled distributions of monthly mean temperatures sampled from the spatial stream network (SSN) model (37, 50) for seven populations in both current (A–C) and historical (D–F) habitats. Using the phenology reported in FitzGerald et al. (37), we isolated the maximum monthly mean temperature during the rearing period (A: RMAX-Cur, D: RMAX-His); The maximum monthly mean temperature during the entire year (B: AMAX-Cur, E: AMAX-Hist); and the range between the maximum and minimum monthly average temperatures (C: ARANGE – Cur, F: ARANGE – His).
CTMAX and heat hardening response were modeled with linear mixed effect models implemented using the Bayesian statistical package “brms” (53, 54) in R (version 4.2.2). We used the packages “ggplot2” (55) to visualize model output and “emmeans” (56) to calculate marginal means of the models. Visual inspection of Q-Q plots indicated several outliers which could not be explained as erroneous data. We therefore modeled the observed data using a Student's t-distribution as opposed to a Gaussian distribution as the former produces more robust estimates in the presence of outliers. Priors were uniformed and centered on zero. Models were built using a forward selection of potential predictor variables guided by the biology of the fish and experimental design. We used leave-one-out cross-validation (LOO) to determine the best-fitting models, selecting the model with the lowest LOO score. We assembled three suites of models: “Treatment models,” “Trade-off models,” and “Environmental models,” discussed in detail below.
“Treatment models” assessed differences in CTMAX performance (N = 770 CTMAX measures, 385 individual fish) among treatment groups and trial number (first or second CTMAX) and were used to determine treatment-specific mean CTMAX estimates. Treatment Mean models included fixed effects of hatchery, acclimation temperature, acclimation duration, and trial number. Additional potential predictors included fish mass and condition factor, and we tested the random effects of specific CTMAX beaker as well as fish ID to account for repeated sampling (Supplementary Table 1). Treatment contrasts were considered significant if the 89% credible interval of the posterior distributions did not overlap 0.
“Trade-off models” investigated the presence or absence of a tradeoff between CTMAX and ΔCTM and were developed for two scales; among individual fish (N = 385; Supplementary Table 2) and across populations (N = 21; Supplementary Table 3). Individual fish tradeoff models assessed the relationship between a fish's initial CTMAX and its ΔCTM. Additional predictors included population, acclimation temperature, acclimation duration, condition factor, mass, as well as a random effect for CTMAX beaker. Population tradeoff models used the treatment-specific mean estimates for both the first and second CTMAX trial produced by the final Treatment Mean Model. The calculated ΔCTM for each population was also adjusted for RTM before running models. Fixed predictors included a population's mean CTMAX during its first trial, and the acclimation temperature. We additionally tested a random intercept and slope for each population. Due to the small sample size of these models we employed uninformed, but regularizing priors using a Student's t-distribution (K = 3) and a half-Cauchy distribution for the variance (57, 58). A trade-off was determined to be significant if the 89% credible interval of the estimated slope between initial CTMAX and ΔCTM did not include 0 (59).
Environmental models investigated the relationship between six habitat temperature metrics and the latitude of each population's hatchery against the ΔCTM of individual fish (n = 385). These models also explored the relationship between the environmental predictors and the acclimation capacity of a population (defined below). Models regressed the ΔCTM (RTM adjusted) against each environmental predictor with an interacting effect of acclimation temperature and random intercepts for the hatchery and CTM chamber. Some habitat thermal metrics were correlated, so environmental predictors only co-occurred in models when the Pearson's correlation was <0.7 to reduce collinearity. We also tested additional fixed predictors for mass and condition factor. Models were constructed based upon a prior understanding of predictors using a forward process (see Supplementary Tables 4, 5 for regression coefficients and LOO scores). An environmental predictor was determined to be significant if the 89% credible interval of the estimated association did not include 0. Due to the presence of ΔCTM outliers these models assumed a Student's t-distribution for the response variable as well as for the regularizing priors (k = 3).
We quantified the acclimation capacity for each population (n = 7) using the posterior distributions of the best-fitting treatment mean model. For each population, we then calculated the difference between the treatment mean model-estimated CTMAX of 11°C fish on their first thermal trial with the treatment mean model-estimated CTMAX of 20°C on their second thermal trial. We therefore captured the broadest span between trial-naïve cold-acclimated fish and warm-acclimated fish that have experienced a recent thermal stress. We calculated the variance of the acclimation capacity for each population. We used linear models to regress acclimation capacity against each environmental predictor individually as well as in addition to latitude. The final model was selected based upon LOO scores (Supplementary Table 6). No additional fixed predictors were included or interactions of environmental predictors due to the limited number of populations. Acclimation capacity estimates were weighted by the inverse of their variance which was then scaled and centered to 1.0.
During CTMAX experiments, acute thermal tolerances ranged from 20.8 to 31.0°C (μ = 28.6 ± 1.2°C; Table 2). We found that the CTMAX values were sensitive to the population of origin, the acclimation temperature, and whether the fish had undergone a prior CTMAX experiment. CTMAX estimates generally increased with acclimation temperature, with fish acclimated to 20°C exhibiting the highest thermal tolerances (μ = 29.5 ± 1.0°C), particularly during their second CTMAX challenge (1st CTMAX: 29.3 ± 0.9 and 2nd CTMAX: 29.6 ± 1.0°C). The overall heat hardening response (ΔCTM) was nearly zero (−0.07 ± 1.23°C, μ ± S.D.), however this response was also found to vary by population and acclimation temperature, with fish acclimated to cold temperatures (11°C) exhibiting primarily negative ΔCTM values (−0.8 ± 1.4°C) and those acclimated to warmer temperatures (16 or 20°C) exhibiting primarily positive values (16°C: 0.2 ± 0.9°C, 20°C: 0.6 ± 0.8°C, μ ± S.D.). More detail on the observed data can be found in Table 2.
Bayesian models were used to determine the mean acute thermal tolerances for each treatment group, and we used LOO to determine the best model (Supplementary Table 1). There were two models of fish CTMAX for which LOO values were not significantly different. The difference between the predicted acute thermal tolerances of these two models was 0.001 ± 0.029°C. Therefore, we conducted our analysis with the lowest LOO model which included a three-way interaction of categorical predictors for acclimation temperature, hatchery, and whether it was a fish's first or second trial. There was an additional fixed effect for fish mass and a random effect for fish ID. The discarded model was identical with an additional random effect for CTMAX chamber. The highest model-estimated CTMAX values were exhibited by the Trask River population reared at 20°C during the second CTMAX trial {30.7°C [89% credible interval (C.I.) 30.5–30.9°C]}. The lowest values were exhibited by the Priest Rapids and Winter-run populations acclimated to 11°C during their second CTMAX trial [27.1°C (89% C.I. 26.8–27.4°C) and 27.3°C (89% C.I. 27.0–27.6°C), respectively]. Acclimation duration was not a predictor in the lowest-scoring model, nor did it yield any significant associations with CTMAX in models that contained it.
The capacity for individual juvenile Chinook salmon to physiologically improve their CTMAX performance upon their second trial varied among populations and acclimation conditions with capacity increasing up to 3.2°C (Feather Spring-run individual acclimated to 20°C) and other fish losing capacity by up to −7.9°C (Winter-run individual acclimated to 11°C). There were two models of individual ΔCTM for which LOO values were not significantly different. Comparing the predicted values between these models revealed a mean difference of 0.016 ± 0.12°C. Therefore, we conducted our analysis with the lowest LOO-scoring model which included a three-way interaction of a fish's initial CTMAX, acclimation temperature, and hatchery, an additional fixed effect for fish mass, and a random effect for CTMAX chamber. Acclimation duration was not a predictor in the lowest-scoring ΔCTM model, nor did it yield any significant associations with ΔCTM in models that contained it.
Across all populations, when fish were acclimated to 11°C their 2nd CTMAX was lower than their first (ΔCTM = −1.1° to −0.2°C). In contrast, the heat hardening response was positive with higher 2nd CTMAX measurements among 12 of 14 treatment groups that were acclimated to 16 or 20° (Figure 3A) with the Trinity population being the only exception. Populations also differed in the amount of change in ΔCTM associated with changes in acclimation. For instance, the Priest Rapids hatchery exhibited a 2.5°C change in the heat hardening response between fish acclimated to 11 vs. 20°C. Meanwhile, the Trinity population exhibited a small but positive (0.5°C) change in ΔCTM from fish acclimated to 11–20°C (Figure 3B).
Figure 3. Heat Hardening Response (ΔCTM) of seven populations of juvenile Chinook salmon acclimated to one of three temperatures. Boxplots show the range of the observed data, while black points indicate the model-fitted mean estimated and 89% credible intervals of the ΔCTM of a hypothetical fish eliciting the mean CTMAX (28.7°C). The dashed horizontal line indicates a ΔCTM of 0. +|–|0 indicate whether the ΔCTM is significantly positive, negative, or neither, while capital letters (A–C) indicate significant differences between populations sharing an acclimation temperature (A), and lowercase letters (a,b,c) indicate differences within a population (B). In plot (B), the boxplots are printed in gray for visual clarity against the black horizontal line
Populations differed in the amount of acclimation capacity they possessed (defined as the difference between treatment mean model-estimated CTMAX of 11°C fish on their first thermal trial and the treatment mean model-estimated CTMAX of 20°C on their second thermal trial). The Priest Rapids and Trask hatchery populations exhibited the greatest amount of acclimation capacity [2.6°C (89% C.I. 2.3–2.9°C) and 2.4°C (89% C.I. 2.1–2.7°C), respectively], while the Feather Fall-run and Trinity populations exhibited the least [1.2 (89% C.I. 0.9–1.5°C) and 0.9°C (89% C.I. 0.5–1.3°C), respectively].
We found evidence supporting the TOH between CTMAX and ΔCTM among individual fish (Figure 4A). Only the Trinity hatchery, across all acclimation temperatures, exhibited non-significant associations (where the 89% credible interval overlapped 0) between a fish's initial CTMAX and its ΔCTM, and therefore, no evidence of a tradeoff. For all other populations we found that a fish's initial CTMAX value was significantly negatively associated with ΔCTM. We did not, however, find evidence of the TOH between modeled estimated CTMAX and ΔCTM among treatment groups (i.e., population × acclimation temperature), indicating that populations exhibiting high thermal tolerance did not exhibit a reduced heat hardening response (Figure 4B).
Figure 4. Trade-off between CTMAX and heat hardening response (ΔCTM) for seven populations reared at three acclimation temperatures (11, 16, and 20°C) quantified at the (A) individual level and (B) at the population level. (A) The observed data for individual fish are represented as points, the model estimated trade-off between a given population's initial CTMAX and its ΔCTM are presented as lines (colored), and the overall model estimated trade-off when controlling for individual populations is shown as a thick black line and shaded credible intervals (89% light, 50% dark). Facets capture respective acclimation groups. The y-axis was truncated to improve visualization thereby excluding seven data points [μ = −5.72°C (−7.88 to −4.83°C)]. (B) The relationship between the model-estimated average 1st CTMAX against that population's mean heat hardening response. Each line represents an acclimation group with shaded credible intervals (89% light, 50% dark). Point colors indicate each population.
In addition to our trade-off models, we investigated whether ΔCTM and acclimation capacity were associated with environmental characteristics and latitude of each population. The best fitting (lowest LOO-score) environmental model of ΔCTM included an interaction between the historical maximum mean monthly temperature experienced during the rearing period (RMAX-His) and acclimation temperature, and a fish's condition factor as well as random effects of population and CTMAX beaker (see Supplementary Tables 4, 5 for all environmental predictor models). Latitude as a predictor was only found in low LOO-scoring models alongside RMAX-His, in these models, latitude alone did not yield a significant association with ΔCTM. The relationship between RMAX-His and ΔCTM varied by acclimation temperature (Figure 5). Among fish acclimated to 11°C there was a significant negative association between RMAX-His and ΔCTM (Figure 5). The relationship among fish acclimated to 16°C was slightly positive but was not significantly different from 0. When fish were acclimated to 20°C, the relationship was positive and significant. However, the 16 and 20°C acclimation groups were not significantly different from each other (Figure 5).
Figure 5. Relationship between historical estimates of RMAX-His to fish heat hardening response (ΔCTM). Points represent observed data while lines represent the model fit with the 50 and 89% credible intervals (dark and light shading, respectively). Horizontal dashed line indicates ΔCTM = 0. –/+/0 indicate whether the association between ΔCTM and RMAX-His was significantly negative, positive or not significantly different from 0, while lowercase letters (f,g) indicate whether the associations were significantly different among acclimation temperatures. The y-axis was truncated to improve visualization thereby excluding seven data points [μ = −5.72°C (−7.88 to −4.83°C)] from being plotted. See Supplementary Figures 1–4 for association plots between ΔCTM and the other environmental predictors.
The lowest LOO-scoring model for acclimation capacity and environmental traits included population latitude and the RRANGE-His. There was a significant positive association between acclimation capacity and the RMAX-His, with populations from historically warmer habitats exhibiting a larger acclimation capacity (Figure 6A). Separately, latitude was also a significant predictor of acclimation capacity, with populations from higher latitudes exhibiting greater acclimation capacity (Figure 6B; r = 0.53). All other associations between acclimation capacity and current or historical environmental predictors were not significantly distinguishable from zero (Figure 6C).
Figure 6. Relationships between acclimation capacity and population latitude (A), historical maximum monthly mean temperature during rearing period (B, RMAX-His) for seven populations of juvenile Chinook salmon as well as modeled associations (C) from the lowest LOO-scoring model. In (A, B), the dark line indicates the model estimated association between the given environmental predictor and a populations acclimation capacity, whereas the dark and light shading represent the 50 and 89% credible intervals (C.I.) around this estimate trend, error bars represent the standard deviation of the estimated acclimation capacity of each population. In (C), the model estimate association (dot = mean, box = 50% C.I., line = 89% C.I.) is provided.
Experimental mortality was slightly less during the first CTMAX trial (n = 33 of 461, 7.2%) than during the second trial (n = 33 of 418, 7.9%). Of the 33 fish that did not recover from their second CTMAX trial, 27 had initial CTMAX values that were outside of the 89% credible interval of their respective treatment's modeled mean performance (13 below, 14 above). All of the eight fish that were discarded for unusual behavior involved fish losing equilibrium during the acclimation period prior to their second CTMAX trial or early in the trial when water temperatures were within 3°C of their initial temperature.
In October 2018, one tank of 20°C acclimated Winter-run Chinook salmon experienced an outbreak of columnaris and subsequent mortality (n = 7). The mortality in this tank is theorized to be a result of temperature stress after being housed at 20°C for 202 days. Collection of CTMAX data preceded disease onset by 41 days and data are presumed to be unaffected. However, the loss of animals limited our ability to continue to gather CTMAX data and so reduced the treatment's sample size (Winter-run acclimated at 20°C, n = 8).
In light of rapid environmental change, particularly the warming of aquatic environments (60), understanding the capacity of organisms to physiologically acclimate to and tolerate a changing thermal landscape is essential to understanding species and population responses (61–63). Populations capable of thermal physiological plasticity are anticipated to cope with future climate change and stochastic thermal extremes better than populations that are unable to improve thermal tolerance through physiological acclimation (25). In the present study, we investigated the acclimation capacity of seven populations of Chinook salmon from the West Coast of North America. We assessed the acute thermal response of fish acclimated to a range of ecologically relevant temperatures (11, 16, and 20°C) before and after exposure to a prior acute thermal challenge. In doing so we were able to assess the ability of individuals to physiologically respond to a thermal challenge, the capability for a population to acclimate to thermal conditions, and whether individuals and populations exhibited a physiological trade-off between upper thermal tolerance and their capacity to acclimate. Finally, we assessed whether differences in population performance could be associated with aspects of a population's local, natural environment to support hypotheses of local adaptation (17).
The vast majority of previous research on heat hardening and our estimates of heat hardening among Chinook salmon acclimated to 16 or 20°C have demonstrated an improvement in acute thermal tolerance (Table 3). We found that while Chinook salmon populations differed in their ΔCTM performance, there was a consistent effect of acclimation temperatures across populations, with fish acclimated to 16 or 20°C exhibiting a positive ΔCTM. However, our discovery of significant, “heat weakening” (i.e., negative ΔCTM) exhibited by all populations when acclimated to 11°C is unique. To our knowledge, the only other examples of “heat weakening” occurred when Red Shiner (Notropis lutrensis) and Fathead Minnow (Pimephales promelas) were acclimated to temperatures ~17°C below their initial CTMAX values, but this “heat weakening” was not significant (20). Our 11°C acclimated Chinook salmon were also acclimated to ~17°C below their eventual CTMAX (average 28.0°C), but “heat weakening” in our study was significant. It may be that exposing fish to near-lethal thermal extremes when their thermal history is so ill-matched incurs additional physiological damage leading to the “heat weakening” effect we encountered.
The Trade-off Hypothesis (TOH) (25, 26) posits that organisms with a high heat tolerance will exhibit a reduced plasticity, arising from the idea that organisms exhibiting a higher CTMAX are likely closer to a hypothetical physiological maximum and can therefore exhibit a reduced plastic capacity (e.g., ΔCTM or acclimation capacity). We used repeated CTMAX trials to assess the TOH among juvenile Chinook salmon both within and between populations. Among individual fish, we found a consistent tradeoff wherein a fish with a higher initial CTMAX exhibited a lower ΔCTM. These results may indicate the presence of a “concrete ceiling” of acute thermal performance within a population (64), with individuals losing plasticity as their tolerance approaches a fixed limit. A fixed thermal ceiling may indicate a limited adaptive capacity within a population to evolve greater thermal tolerance.
Interestingly, we did not find evidence for the TOH when populations are compared against one another. After controlling for acclimation temperature, there was no evidence for a trade-off between a population's CTMAX and its average ΔCTM. One interpretation of this mixed support for TOH between individuals and populations is that while populations may be approaching their respective thermal ceilings, the species as a whole retains adaptive capacity, potentially enabling Chinook salmon to adapt their acute thermal tolerance during future colonization and radiation.
Salmonid physiology has been linked to population-specific environmental characteristics (17, 38, 65). In this experiment, we tested seven populations of juvenile Chinook salmon that differed in their migratory phenology (five fall-run populations, one spring-run population and one winter-run population) and thermal physiology (17, 66). In the case of acute thermal maxima, past research by Zillig et al. (17) on a subset of these populations indicated that modeled historical measures of stream temperatures were better predictors of population performance than current estimates. Likewise, work by Eliason et al. (38) found that the metabolic performance of a population of adult Sockeye salmon (O. nerka) were better suited to historical river temperatures rather than current ones. We expanded upon these results, documenting how the heat hardening response and acclimation capacity in CTMAX exhibited by different populations was associated with several environmental characteristics, particularly the historical maximum monthly mean temperature experienced during the rearing period (RMAX-His). We found that when fish were acclimated to 11°C, populations from habitats with cooler RMAX-His performed better than those populations from warmer habitats. Among fish acclimated to 20°C, the opposite pattern was observed, where fish from cold habitats elicited worse ΔCTM than fish from historically warmer habitats. These results are consistent with local adaptation of ΔCTM, wherein fish that may experience warmer habitats exhibit an improved capacity to withstand those conditions. Furthermore, we find a positive association between RMAX-His and acclimation capacity indicating that fish which may have historically encountered higher temperatures are more capable of acclimating to those regimes. Taken together, these results point toward populations from warmer habitats exhibiting greater capacity to both acclimate and withstand thermal extremes, traits that may become increasingly important in a warming world, whereas populations from unusually cold habitats may be expected to exhibit reduced thermal tolerance and acclimation capacity.
Counterintuitively, we also found a significant, positive association between population latitude and acclimation capacity, with populations from higher latitudes exhibiting greater acclimation capacity than those from lower latitudes. This may be explained by the hydrologic idiosyncrasies of our selected populations which include several Northern Californian populations resident to snowmelt-fed rivers and downstream of large, deep storage reservoirs, rain-fed coastal populations (Trask and Elk River) and the Priest Rapids population downstream of run-of-the-river hydropower dams (67). This result highlights the value of local population-specific knowledge and the risk of relying solely upon broad-scale geographical patterns (e.g., latitude) when predicting population response (37).
For instance, the Priest Rapids population was identified as being susceptible to future warming conditions due to its relatively reduced growth when acclimated to 20°C and long migration route (17). In the present study, the Priest Rapids population exhibits both the greatest acclimation capacity (2.1°C) and also a significant loss in ΔCTM among fish acclimated to cold temperatures (11°C). This duality highlights the importance of assessing multiple thermal performance metrics, and additionally, the risks in predicting species performance without a thorough understanding of thermal physiology. Therefore, it is important to note that the Priest Rapids population come from the warmest habitat per the SSN model (RMAX-His: 20.4 ± 0.5°C) and when acclimated to 20°C elicits an above average CTMAX and the 2nd highest ΔCTM. Therefore, the species apparent plasticity when acclimated to warm temperatures may be more relevant to their performance in the wild than their poor performance when acclimated to cold temperatures. Furthermore, these results support ideas of local adaptation in regards to acute thermal tolerance where populations perform optimally under conditions similar to their local environment.
On the other hand, the Trinity hatchery population exhibited both the smallest response to acclimation temperature, the smallest acclimation capacity, and no significant heat hardening effects at any acclimation temperature. The lack of a heat hardening response as well as the overall limited response with acclimation temperature support the idea that the Trinity population possesses very little overall acclimation capacity, a trait that makes it vulnerable to future environmental warming. The modeled environmental traits of the Trinity population identify it as one of the coldest-rearing populations in our study, and therefore this population may lack the acclimatory response to warm temperatures exhibited by other populations. Still, a larger sample size may elucidate individuals with positive heat hardening responses, and further research linking thermal physiology to population genetics would provide insight into these individual and population differences.
Other work on Chinook salmon (New Zealand population) demonstrated that fish acclimated to 13°C were able to improve their CTMAX by 0.6°C after exposure to a salinity stressor or air exposure relative to an unstressed control group (68). This improvement in CTMAX is greater than the differences observed in our study, particularly among fish acclimated to 11°C which elicited a mean ΔCTM of −0.6 ± 1.4°C. It is interesting that the non-thermal stressors (a salinity challenge and air exposure) tested in Rodgers and Gomez Isaza (68), led to greater acute thermal tolerance than the thermal trials conducted presently. Variation in the size of CTMAX increase among stressors [air, salinity or acute thermal exposure (present study)] may be due to the activation of a general protective stress response (69, 70) which may be somewhat mitigated in the present study due to physiological damage associated with a prior near-lethal acute thermal stress which may not be triggered by air exposure or salinity challenges. Given the effect of acclimation temperature as well as interpopulation variation, further study of differences in multi-stressor responses among salmonid populations would be informative for understanding population-specific responses to environmental change.
An important caveat to this work is the timing of CTMAX trials. We exposed fish to a second CTMAX ca. 24 h after their first. While this time frame has some ecological relevance (thermal extremes are typically consistent in their timing day to day), it may be that the heat-hardening response of juvenile Chinook salmon occurs more quickly. In other teleosts, the heat hardening effect was found to peak a few hours after the initial exposure (20, 71). Presumably, acclimation temperature may also impact the timeframe of a heat hardening response as fish acclimated to warm temperatures may be capable of physiological adjustment quicker than those acclimated to cold temperatures. Testing the speed at which a fish mounts a heat hardening response was beyond the scope of this study but would be an interesting avenue for future investigation. Additionally, fish used in this study had no prior experience with fluctuating temperatures whereas wild fish may be able to respond to transient periods of thermal stress and physiologically prepare for future incidents (69, 72). At the time of testing, the fish used in this study were of the smolt size class. Smolting in salmonids is a time of rapid physiological change as they prepare for migration to the ocean. In other salmonids, CTMAX declined gradually as the fish aged (73), however the impact of smoltification on their heat hardening response is unstudied.
Finally, the fish used in this experiment were all of hatchery origin with free-swimming parents who may also have been of hatchery origin. Hatchery embryos are incubated in constant, cold temperatures with high oxygen saturation levels to maximize survival, and juveniles are reared in warmer temperatures with high food intake to maximize growth. These artificial, unnatural conditions can result in juveniles entering the river system with lower fitness, and the effect of hatchery domestication on salmonids has been demonstrated to modify populations and lower fitness over just a handful of generations (32, 74, 77). Hatchery selection may have altered thermal physiology as well, as supported by a study on zebra-fish (Danio rerio), where captive, laboratory-reared fish had reduced thermal plasticity when compared to wild rearing counterparts (27). Indeed, a parent's thermal history can significantly affect the physiological responses of their offspring (34, 35). However, the influence of hatchery domestication on thermal physiology of Pacific salmon is poorly understood and requires comparisons of natural- and hatchery-reared fish, which may be infeasible for populations that are primarily hatchery-origin. Regardless, while hatcheries may have unresolved phenotypic impacts on the thermal physiology of young salmon, our study finds that ancestral thermal history (i.e., historical, natural rearing temperatures) alongside current thermal exposure (i.e., acclimation temperatures) were associated with heat hardening responses and acclimation capacity, indicating that both ancestral and current thermal exposures shape thermal physiology.
In summary, we found that individual fish exhibited a thermal trade-off between their acute thermal tolerance and their heat hardening response, however we did not find this phenomenon at the population scale. We did find that the acclimation capacity of populations was positively correlated with its historical thermal rearing environment. Likewise, we found that environmental temperature predictors are associated with fish heat hardening response. Specifically, our results demonstrated improved performance when populations are acclimated to temperatures close to temperatures they would experience in the wild. Finally, these results indicated that different populations of Chinook salmon will not respond to repeated thermal stresses in the same manner. Consistent with past research, we find that the critically endangered Sacramento River Winter-run salmon is more susceptible to acute thermal stressors. We would also point out that the Trinity population exhibited the lowest thermal plasticity of any of the populations we explored, which may be a harbinger for poor performance in a rapidly changing landscape. Both of those populations have likely already experienced extreme changes when impassable dams built in those watersheds drastically altered seasonal temperature regimes by creating overall warmer, less variable regimes (67). Therefore, close attention should be paid to interpopulation differences when predicting species response to climate change and management actions.
The raw data supporting the conclusions of this article will be made available by the authors, without undue reservation.
The animal study was approved by University of California, Davis Institutional Animal Care and Use Committee. The study was conducted in accordance with the local legislation and institutional requirements.
KZ: Conceptualization, Data curation, Formal analysis, Funding acquisition, Investigation, Methodology, Project administration, Supervision, Validation, Visualization, Writing – original draft, Writing – review & editing. HB: Data curation, Formal analysis, Investigation, Writing – original draft, Writing – review & editing. AF: Conceptualization, Data curation, Formal analysis, Investigation, Resources, Visualization, Writing – original draft, Writing – review & editing. NF: Conceptualization, Funding acquisition, Investigation, Project administration, Resources, Supervision, Writing – original draft, Writing – review & editing.
The author(s) declare financial support was received for the research, authorship, and/or publication of this article. This work was supported by The Environmental Protection Agency (NF, W912P7-15-P-0015); U.S. Fish and Wildlife Service and University of California (NF, F17AC00491), and the University of California, Agricultural Experiment Station (NF, 2098-H).
We thank Dennis Cocherell, Sarah Baird, Trinh Nguyen, and Melissa Crews for research assistance. We thank the salmon hatcheries and associated managers for providing fish used in this study. We thank Martha W. Zillig for help in editing the manuscript drafts. Finally, we thank reviewers from the National Oceanic and Atmospheric Administration (NOAA) and Frontiers in Fish Science. The scientific results and conclusions, as well as any views or opinions expressed herein, are those of the author(s) and do not necessarily reflect those of NOAA or the Department of Commerce.
The authors declare that the research was conducted in the absence of any commercial or financial relationships that could be construed as a potential conflict of interest.
The author(s) declare that no Gen AI was used in the creation of this manuscript.
All claims expressed in this article are solely those of the authors and do not necessarily represent those of their affiliated organizations, or those of the publisher, the editors and the reviewers. Any product that may be evaluated in this article, or claim that may be made by its manufacturer, is not guaranteed or endorsed by the publisher.
The Supplementary Material for this article can be found online at: https://www.frontiersin.org/articles/10.3389/frish.2024.1508746/full#supplementary-material
Supplementary Figure 1 | Statistical association between the heat hardening response of Chinook salmon reared at three acclimation temperatures (11, 16, or 20°C) and the Annual Temperature Range for Current (A: ARANGE-Cur) and historical (B: ARANGE-His) habitats. Points represented observed data while lines represent the model fit with the 50 and 89% credible intervals (dark and light shading, respectively). Horizontal dashed line indicates ΔCTM = 0. –/+/0 indicate whether the association between ΔCTM and RMAX-His was significantly negative, positive or not significantly different from 0, while lowercase letters (a,b) indicate whether the associations were significantly different among acclimation temperatures, The y-axis was truncated to improve visualization thereby excluding seven data points (μ = −5.72°C [−7.88°C to −4.83°C]) from being plotted.
Supplementary Figure 2 | Statistical association between the heat hardening response of Chinook salmon reared at three acclimation temperatures (11, 16, or 20°C) and the Maximum monthly average temperature during the rearing period for current (A: RMAX-Cur) and historical (B: RMAX-His) habitats. Points represented observed data while lines represent the model fit with the 50 and 89% credible intervals (dark and light shading, respectively). Horizontal dashed line indicates ΔCTM = 0. –/+/0 indicate whether the association between ΔCTM and RMAX-His was significantly negative, positive or not significantly different from 0, while lowercase letters (y, z, or f, g) indicate whether the associations were significantly different among acclimation temperatures, The y-axis was truncated to improve visualization thereby excluding seven data points (μ = −5.72°C [−7.88°C to −4.83°C]) from being plotted.
Supplementary Figure 3 | Statistical association between the heat hardening response of Chinook salmon reared at three acclimation temperatures (11, 16, or 20°C) and the annual maximum monthly average temperature current (A: AMAX-Cur) and historical (B: AMAX-His) habitats. Points represented observed data while lines represent the model fit with the 50 and 89% credible intervals (dark and light shading, respectively). Horizontal dashed line indicates ΔCTM = 0. –/+/0 indicate whether the association between ΔCTM and RMAX-His was significantly negative, positive or not significantly different from 0, while lowercase letters (a, b, or j, k) indicate whether the associations were significantly different among acclimation temperatures, The y-axis was truncated to improve visualization thereby excluding seven data points (μ = −5.72°C [−7.88°C to −4.83°C]) from being plotted.
Supplementary Figure 4 | Statistical association between the heat hardening response of Chinook salmon reared at three acclimation temperatures (11, 16, or 20°C) and the population's latitude based upon hatchery location. Points represented observed data while lines represent the model fit with the 50% and 89% credible intervals (dark and light shading, respectively). Horizontal dashed line indicates ΔCTM = 0. –/+/0 indicate whether the association between ΔCTM and RMAX-His was significantly negative, positive or not significantly different from 0, while lowercase letters (y,z) indicate whether the associations were significantly different among acclimation temperatures, The y-axis was truncated to improve visualization thereby excluding seven data points (μ = −5.72°C [−7.88°C to −4.83°C]) from being plotted.
Supplementary Table 1 | Model summaries for nine models constructed to estimate the mean CTMAX value for each of seven juvenile Chinook Salmon populations. Model estimated regression values are provided as a mean and 95% credible interval of the estimate. Models were evaluated based upon Leave-one-out criteria (LOO Scores).
Supplementary Table 2 | Model summaries for nine models constructed to estimate the trade-off relationship between an individual fish's initial CTMAX and its second CTMAX. Model estimated regression values are provided as a mean and 95% credible interval of the estimate. Models were evaluated based upon Leave-one-out criteria (LOO Scores).
Supplementary Table 3 | Model summaries for four models constructed to estimate the trade-off relationship between populations mean initial CTMAX and its second CTMAX. Model estimated regression values are provided as a mean and 95% credible interval of the estimate. Models were evaluated based upon Leave-one-out criteria (LOO Scores).
Supplementary Table 4 | Model summaries of models constructed to estimate the trade-off between a given environmental predictor and a fish's heat hardening response (ΔCTM). Model estimated regression values are provided as a mean and 95% credible interval of the estimate. Models were evaluated based upon Leave-one-out criteria (LOO Scores). Each individual environmental predictor is provided its own sub-table for model results (4.A to 4.G).
Supplementary Table 5 | Model summaries for 25 models constructed to estimate association between a fish's heat hardening response (ΔCTM) and several environmental predictors. Model estimated regression values are provided as a mean and 95% credible interval of the estimate. Models were evaluated based upon Leave-one-out criteria (LOO Scores).
Supplementary Table 6 | Model summaries for thirteen models constructed to estimate the association between a population's acclimation capacity and environmental traits. Model estimated regression values are provided as a mean and 95% credible interval of the estimate. Models were evaluated based upon Leave-one-out criteria (LOO Scores).
1. FitzGerald A. M. (in revision). Incorporating local information to predict thermal stress for diverse species. Can. J. Fish. Aquat. Sci.
2. Stillman JH. Heat waves, the new normal: summertime temperature extremes will impact animals, ecosystems, and human communities. Physiology. (2019) 34:86–100. doi: 10.1152/physiol.00040.2018
3. Williams SE, Shoo LP, Isaac JL, Hoffmann AA, Langham G. Towards an integrated framework for assessing the vulnerability of species to climate change. PLoS Biol. (2008) 6:e325. doi: 10.1371/journal.pbio.0060325
4. Hoffmann AA, Sgrò CM. Climate change and evolutionary adaptation. Nature. (2011) 470:479–85. doi: 10.1038/nature09670
5. Hofmann GE, Todgham AE. Living in the now: physiological mechanisms to tolerate a rapidly changing environment. Annu Rev Physiol. (2010) 72:127–45. doi: 10.1146/annurev-physiol-021909-135900
6. Nadeau CP, Urban MC, Bridle JR. Climates past, present, and yet-to-come shape climate change vulnerabilities. Trends Ecol Evol. (2017) 32:786–800. doi: 10.1016/j.tree.2017.07.012
7. Somero GN. The physiology of climate change: how potentials for acclimatization and genetic adaptation will determine ‘winners' and ‘losers.' J. Exp. Biol. (2010) 213:912–20. doi: 10.1242/jeb.037473
8. Huey RB, Kearney MR, Krockenberger A, Holtum JA, Jess M, Williams SE. Predicting organismal vulnerability to climate warming: roles of behaviour, physiology and adaptation. Phil Trans R Soc B. (2012) 367:1665–79. doi: 10.1098/rstb.2012.0005
9. Seebacher F, White CR, Franklin CE. Physiological plasticity increases resilience of ectothermic animals to climate change. Nat Clim Change. (2015) 5:61–6. doi: 10.1038/nclimate2457
10. Somero GN. Thermal physiology and vertical zonation of intertidal animals: optima, limits, and costs of living. Integr Comp Biol. (2002) 42:780–9. doi: 10.1093/icb/42.4.780
11. Levins R. Thermal acclimation and heat resistance in Drosophila species. Am Nat. (1969) 103:483–99. doi: 10.1086/282616
12. Conover DO, Present TMC. Countergradient variation in growth rate: compensation for length of the growing season among Atlantic silversides from different latitudes. Oecologia. (1990) 83:316–24. doi: 10.1007/BF00317554
13. Arendt JD, Wilson DS. Countergradient selection for rapid growth in pumpkinseed sunfish: disentangling ecological and evolutionary effects. Ecology. (1999) 80:2793–8. doi: 10.1890/0012-9658(1999)080[2793:CSFRGI]2.0.CO;2
14. Ridgway G. Interpopulation variation in blue mussels, Mytilus edulis L., over short distances. Sarsia. (2001) 86:157–61. doi: 10.1080/00364827.2001.10420471
15. Fangue NA, Hofmeister M, Schulte PM. Intraspecific variation in thermal tolerance and heat shock protein gene expression in common killifish, Fundulus heteroclitus. J Exp Biol. (2006) 209:2859–72. doi: 10.1242/jeb.02260
16. Nyboer EA, Chrétien E, Chapman LJ. Divergence in aerobic scope and thermal tolerance is related to local thermal regime in two populations of introduced Nile perch (Lates niloticus). J Fish Biol. (2020) 97:231–45. doi: 10.1111/jfb.14355
17. Zillig KW, FitzGerald AM, Lusardi RA, Cocherell DE, FangueNA. Intraspecific variation among Chinook Salmon populations indicates physiological adaptation to local environmental conditions. Conserv Physiol. (2023) 11:coad044. doi: 10.1093/conphys/coad044
18. Diffenbaugh NS, Swain DL, Touma D. Anthropogenic warming has increased drought risk in California. Proc Nat Acad Sci USA. (2015) 112:3931–6. doi: 10.1073/pnas.1422385112
19. Burggren W. Developmental phenotypic plasticity helps bridge stochastic weather events associated with climate change. J Exp Biol. (2018) 221:jeb161984. doi: 10.1242/jeb.161984
20. Maness JD, Hutchison VH. Acute adjustment of thermal tolerance in vertebrate ectotherms following exposure to critical thermal maxima. J Therm Biol. (1980) 5:225–33. doi: 10.1016/0306-4565(80)90026-1
21. Krebs RA, Feder ME. Hsp70 and larval thermotolerance in Drosophila melanogaster: how much is enough and when is more too much? J Insect Physiol. (1998) 44:1091–101. doi: 10.1016/S0022-1910(98)00059-6
22. Bjelde B, Todgham A. Thermal physiology of the fingered limpet Lottia digitalis under emersion and immersion. J Exp Biol. (2013) 216:jeb.084178. doi: 10.1242/jeb.084178
23. Giomi F, Mandaglio C, Ganmanee M, Han G-D, Dong Y-W, Williams GA, et al. The importance of thermal history: costs and benefits of heat exposure in a tropical, rocky shore oyster. J Exp Biol. (2016) 219:jeb.128892. doi: 10.1242/jeb.128892
24. Drake MJ, Miller NA, Todgham AE. The role of stochastic thermal environments in modulating the thermal physiology of an intertidal limpet, Lottia digitalis. J Exp Biol. (2017) 220:3072–83. doi: 10.1242/jeb.159020
25. Stillman JH. Acclimation capacity underlies susceptibility to climate change. Sci New Ser. (2003) 301:65. doi: 10.1126/science.1083073
26. Van Heerwaarden B, Kellermann V. Does plasticity trade off with basal heat tolerance? Trends Ecol Evol. (2020) 35:874–85. doi: 10.1016/j.tree.2020.05.006
27. Morgan R, Finnøen MH, Jutfelt F. CTmax is repeatable and doesn't reduce growth in zebrafish. Sci Rep. (2018) 8:7099. doi: 10.1038/s41598-018-25593-4
28. Sasaki MC, Dam HG. Integrating patterns of thermal tolerance and phenotypic plasticity with population genetics to improve understanding of vulnerability to warming in a widespread copepod. Glob Chang Biol. (2019) 25:4147–64. doi: 10.1111/gcb.14811
29. Mottola G, López ME, Vasemägi A, Nikinmaa M, Anttila K. Are you ready for the heat? Phenotypic plasticity versus adaptation of heat tolerance in three-spined stickleback. Ecosphere. (2022) 13:e4015. doi: 10.1002/ecs2.4015
30. Gunderson AR. Trade-offs between baseline thermal tolerance and thermal tolerance plasticity are much less common than it appears. Glob Chang Biol. (2023) 29:3519–24. doi: 10.1111/gcb.16710
31. Waples RS, Lindley ST. Genomics and conservation units: the genetic basis of adult migration timing in pacific salmonids. Evol Appl. (2018) 11:9. doi: 10.1111/eva.12687
32. Harrison HL, Berseth V. A commentary on the role of hatcheries and stocking programs in salmon conservation and adapting ourselves to less-than-wild futures. Fish Fish. (2024) 25:750–60. doi: 10.1111/faf.12836
33. Quinn TP. The Behavior and Ecology of Pacific Salmon and Trout. 2nd ed. Seattle, WA: University of Washington Press (2018).
34. Penney CM, Burness G, Tabh JK, Wilson CC. Limited transgenerational effects of environmental temperatures on thermal performance of a cold-adapted salmonid. Conserv Physiol. (2021) 9:coab021 doi: 10.1093/conphys/coab021
35. Penney CM, Tabh JK, Wilson CC, Burness G. Within-generation and transgenerational plasticity of a temperate salmonid in response to thermal acclimation and acute temperature stress. Physiol Biochem Zool. (2022) 95:484–99. doi: 10.1086/721478
36. Crozier LG, McClure MM, Beechie T, Bograd SJ, Boughton DA, Carr M, et al. Climate vulnerability assessment for Pacific salmon and steelhead in the California Current Large Marine Ecosystem. PLoS ONE. (2019) 14:e0217711. doi: 10.1371/journal.pone.0217711
37. FitzGerald AM, John SN, Apgar TM, Mantua NJ, Martin BT. Quantifying thermal exposure for migratory riverine species: Phenology of Chinook salmon populations predicts thermal stress. Glob Change Biol. (2021) 27:536–49. doi: 10.1111/gcb.15450
38. Eliason EJ, Clark TD, Hague MJ, Hanson LM, Gallagher ZS, Jeffries KM, et al. Differences in thermal tolerance among sockeye salmon populations. Science. (2011) 332:109–12. doi: 10.1126/science.1199158
39. Chen Z, Anttila K, Wu J, Whitney CK, Hinch SG, Farrell AP. Optimum and maximum temperatures of sockeye salmon (Oncorhynchus nerka) populations hatched at different temperatures. Can J Zool. (2013) 91:265–74. doi: 10.1139/cjz-2012-0300
40. Stitt BC, Burness G, Burgomaster KA, Currie S, McDermid JL, Wilson CC. Intraspecific variation in thermal tolerance and acclimation capacity in brook trout (Salvelinus fontinalis): Physiological implications for climate change. Physiol Biochem Zool. (2014) 87:15–29. doi: 10.1086/675259
41. Gayeski NJ, Stanford JA, Montgomery DR, Lichatowich J, Peterman RM, Williams RN. The failure of wild salmon management: need for a place-based conceptual foundation. Fisheries. (2018) 43:303–9. doi: 10.1002/fsh.10062
42. Zillig KW, Lusardi RA, Moyle PB, Fangue NA. One size does not fit all: variation in thermal eco-physiology among Pacific salmonids. Rev Fish Biol Fisheries. (2021) 31:95–114. doi: 10.1007/s11160-020-09632-w
43. Moyle PB. Inland Fishes of California. Berkeley, CA; Los Angeles, CA: University of California Press (2002).
44. Yoshiyama RM, Gerstung ER, Fisher FW, Moyle PB. Historical and present distribution of Chinook salmon in the Central Valley drainage of California. In: Fish Bulletin 179. Sacramento, CA: California Department of Fish and Game (2001). p. 71–176.
45. Waples RS. Evolutionary Significant Units and the conservation of biological diversity under the Endangered Species Act. Am Fish Soc Symp. (1995) 17:8–27.
46. Waples RS. Distinct population segments. In: Michael S, Dale DG, Frank WD, editors. The Endangered Species Act at Thirty, Volume 2: Conserving Biodiversity in Human Dominated Landscapes. Washington, DC: Island Press (2006). p. 127–49.
47. Becker CD, Genoway RG. Evaluation of the critical thermal maximum for determining thermal tolerance of freshwater fish. Environ Biol Fishes. (1979) 4:245–56. doi: 10.1007/BF00005481
48. Lee S, Hung SSO, Fangue NA, Haller L, Verhille CE, Zhao J, et al. Effects of feed restriction on the upper temperature tolerance and heat shock response in juvenile green and white sturgeon. Comp Biochem Phys A. (2016) 198:87–95. doi: 10.1016/j.cbpa.2016.04.016
49. Beitinger TL, Bennett WA, McCauley RW. Temperature tolerances of North American freshwater fishes exposed to dynamic changes in temperature. Environ Biol Fishes. (2000) 58:237–75. doi: 10.1023/A:1007676325825
50. Isaak DJ, Wenger SJ, Peterson EE, Ver Hoef JM, Nagel DE, Luce CH, et al. The NorWeST summer stream temperature model and scenarios for the Western U.S.: a crowd-sourced database and new geospatial tools foster a user community and predict broad climate warming of rivers and streams. Water Resour Res. (2017) 53:9181–205. doi: 10.1002/2017WR020969
51. Bjornn TC, Reiser DW. Habitat requirements of salmonids in streams. Am Fish Soc. (1991) 19:83–138.
52. Agrawal A, Schick RS, Bjorkstedt EP, Szerlong RG, Goslin MN, Spence BC, et al. Predicting the potential for historical coho, Chinook and steelhead habitat in Northern California. Santa Cruz, CA: National Oceanic and Atmospheric Administration (2005).
53. Bürkner P-C. brms: an R package for Bayesian multilevel models using Stan. J Stat Soft. (2017) 80:1–27. doi: 10.18637/jss.v080.i01
54. Bürkner P-C. Advanced Bayesian multilevel modeling with the R package brms. R J. (2018) 10:395. doi: 10.32614/RJ-2018-017
55. Wickham H. ggplot2: Elegant Graphics for Data Analysis, 2nd ed. New York, NY: Springer International Publishing (2016).
56. Lenth RV. emmeans: Estimated Marginal Means, aka Least-Squares Means (2020). doi: 10.32614/CRAN.package.emmeans
57. Gelman A. Prior distributions for variance parameters in hierarchical models. (comment on article by Browne and Draper). Bayesian Anal. (2006) 1:BA117A. doi: 10.1214/06-BA117A
58. McNeish D. On using Bayesian methods to address small sample problems. Struct Eq Model. (2016) 23:750–73. doi: 10.1080/10705511.2016.1186549
59. McElreath R. Statistical Rethinking: a Bayesian Course With Examples in R and Stan, 2nd ed. Boca Raton, FL; London, NY: CRC Press, Taylor and Francis Group (2020).
60. Woodward G, Perkins DM, Brown LE. Climate change and freshwater ecosystems: impacts across multiple levels of organization. Phil Trans R Soc B. (2010) 365:2093–106. doi: 10.1098/rstb.2010.0055
61. Chown S, Hoffmann A, Kristensen T, Angilletta MJ, Stenseth NC, Pertoldi C. Adapting to climate change: a perspective from evolutionary physiology. Clim Res. (2010) 43:3–15. doi: 10.3354/cr00879
62. Till A, Rypel AL, Bray A, Fey SB. Fish die-offs are concurrent with thermal extremes in north temperate lakes. Nat Clim Chang. (2019) 9:637–41. doi: 10.1038/s41558-019-0520-y
63. Wagner T, Schliep EM, North JS, Kundel H, Custer CA, Ruzich JK, et al. Predicting climate change impacts on poikilotherms using physiologically guided species abundance models. Proc Natl Acad Sci USA. (2022) 16:2214199120. doi: 10.1073/pnas.2214199120
64. Sandblom E, Clark TD, Gräns A, Ekström A, Brijs J, Sundström LF, et al. Physiological constraints to climate warming in fish follow principles of plastic floors and concrete ceilings. Nat Commun. (2016) 7:11447. doi: 10.1038/ncomms11447
65. Micheletti SJ, Matala AR, Matala AP, Narum SR. Landscape features along migratory routes influence adaptive genomic variation in anadromous steelhead (Oncorhynchus mykiss). Mol Ecol. (2018) 27:128–45. doi: 10.1111/mec.14407
66. Zillig KW, Lusardi RA, Cocherell DE, Fangue NA. Interpopulation variation in thermal physiology among seasonal runs of Chinook salmon. Can J Fish Aquat Sci. (2023) 00:1–13. doi: 10.1139/cjfas-2022-0133
67. Willis AD, Peek RA. Classifying California's stream thermal regimes for cold-water conservation. PLoS ONE. (2021) 16:e0256286. doi: 10.1371/journal.pone.0256286
68. Rodgers EM, Gomez Isaza DF. Stress history affects heat tolerance in an aquatic ectotherm (Chinook salmon, Oncorhynchus tshawytscha). J Therm Biol. (2022) 106:103252. doi: 10.1016/j.jtherbio.2022.103252
69. Todgham AE, Schulte PM, Iwama GK. Cross-Tolerance in the Tidepool Sculpin: The Role of Heat Shock Proteins. Physiol Biochem Zool. (2005) 78:133–44. doi: 10.1086/425205
70. Evans TG, Kültz D. The cellular stress response in fish exposed to salinity fluctuations. J Exp Zool Pt A. (2020) 333:421–35. doi: 10.1002/jez.2350
71. Bilyk KT, Evans CW, DeVries AL. Heat hardening in Antarctic notothenioid fishes. Polar Biol. (2012) 35:1447–51. doi: 10.1007/s00300-012-1189-0
72. Todgham AE, Iwama GK, Schulte PM. Effects of the natural tidal cycle and artificial temperature cycling on hsp levels in the tidepool sculpin Oligocottus maculosus. Physiol Biochem Zool. (2006) 79:1033–45. doi: 10.1086/507664
73. Hines CW, Fang Y, Chan VKS, Stiller KT, Brauner CJ, Richards JG. The effect of salinity and photoperiod on thermal tolerance of Atlantic and coho salmon reared from smolt to adult in recirculating aquaculture systems. Comp Biochem Physiol Part A. (2019) 230:1–6. doi: 10.1016/j.cbpa.2018.12.008
74. Araki H, Ardren WR, Olsen E, Cooper B, Blouin MS. Reproductive success of captive-bred steelhead trout in the wild: evaluation of three hatchery programs in the hood river. Conserv Biol. (2007) 21:181–90. doi: 10.1111/j.1523-1739.2006.00564.x
75. Bard B, Kieffer JD. The effects of repeat acute thermal stress on the critical thermal maximum (CTmax) and physiology of juvenile shortnose sturgeon (Acipenser brevirostrum). Can J Zool. (2019) 6:567–72. doi: 10.1139/cjz-2018-0157
76. Grinder RM, Bassar RD, Auer SK. Upper thermal limits are repeatable in trinidadian guppies. J Therm Biol. (2020) 90:102597. doi: 10.1016/j.jtherbio.2020.102597
77. Araki H, Cooper B, Blouin MS. Genetic effects of captive breeding cause a rapid, cumulative fitness decline in the wild. Science. (2007) 318:100–3. doi: 10.1126/science.1145621
78. Kelly C, Price TD. Correcting for regression to the mean in behavior and ecology. Am Nat. (2005) 166:700–7. doi: 10.1086/497402
79. Hochachka PW, Somero GN. Biochemical Adaptation: Mechanisms and Process in Physiological Evolution. New York, NY: Oxford Univeristy Press (2002).
Keywords: thermal physiology, CTMAX, acclimation, plasticity, fish, temperature, heat hardening
Citation: Zillig KW, Bell HN, FitzGerald AM and Fangue NA (2025) Patterns of interpopulation variation and physiological trade-offs of the acute thermal tolerance of juvenile Chinook salmon (Oncorhynchus tshawytscha). Front. Fish Sci. 2:1508746. doi: 10.3389/frish.2024.1508746
Received: 09 October 2024; Accepted: 17 December 2024;
Published: 15 January 2025.
Edited by:
David J. McKenzie, Centre National de la Recherche Scientifique, FranceReviewed by:
Michael Skeeles, Deakin University, AustraliaCopyright © 2025 Zillig, Bell, FitzGerald and Fangue. This is an open-access article distributed under the terms of the Creative Commons Attribution License (CC BY). The use, distribution or reproduction in other forums is permitted, provided the original author(s) and the copyright owner(s) are credited and that the original publication in this journal is cited, in accordance with accepted academic practice. No use, distribution or reproduction is permitted which does not comply with these terms.
*Correspondence: Nann A. Fangue, bmFmYW5ndWVAdWNkYXZpcy5lZHU=
Disclaimer: All claims expressed in this article are solely those of the authors and do not necessarily represent those of their affiliated organizations, or those of the publisher, the editors and the reviewers. Any product that may be evaluated in this article or claim that may be made by its manufacturer is not guaranteed or endorsed by the publisher.
Research integrity at Frontiers
Learn more about the work of our research integrity team to safeguard the quality of each article we publish.