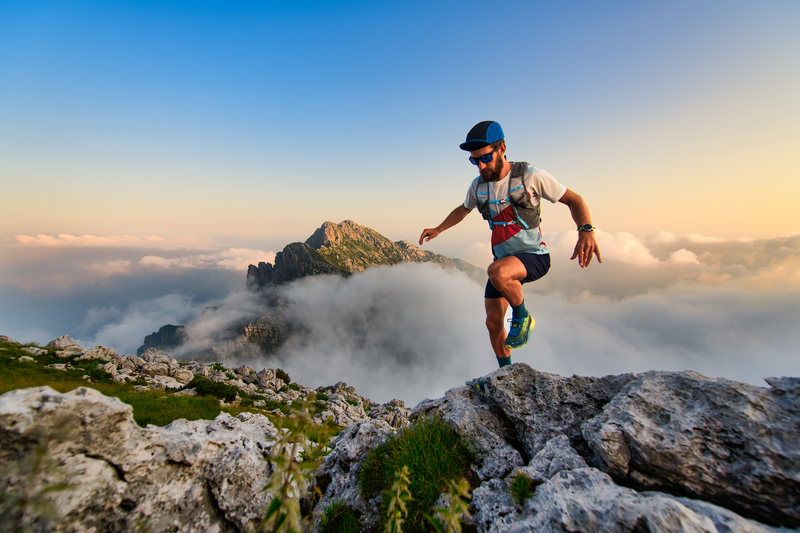
95% of researchers rate our articles as excellent or good
Learn more about the work of our research integrity team to safeguard the quality of each article we publish.
Find out more
BRIEF RESEARCH REPORT article
Front. Fish Sci. , 28 October 2024
Sec. Fish Experimental Biology
Volume 2 - 2024 | https://doi.org/10.3389/frish.2024.1482878
This article is part of the Research Topic Patterns, causes and consequences of intraspecific variation in environmental tolerance in fishes View all 4 articles
Despite the importance of juvenile growth to lifetime fitness, high variability exists within many fish species where some individuals grow markedly faster than others. Here, we explored whether social context mediated growth performance in n = 35 Galaxias maculatus by manipulating the social environment after identifying different growth phenotypes. We found that initially fast-growing fish slowed their growth rate when pooled with similarly fast-growing individuals, whereas none of the initially slow-growing fish markedly improved their growth when pooled with other slow-growers. We examined for any metabolic underpinnings to the findings by measuring standard, routine, and maximum metabolic rates (SMR, RMR, and MMR) at two time points separated by ~4 months. SMR and RMR positively correlated with growth rates when the fish were pooled into growth phenotypes, but not when they were returned to their original (mixed) social groups. We highlight research directions to further elucidate how social context and metabolism interact to govern growth phenotype.
Growth rate is a critical life history trait across all animals. In fishes, rapid growth can fast-track juveniles through size ranges that are most vulnerable to predation, and it can determine the outcome of conflicts in which larger individuals of some species cannibalize slower-growing conspecifics (1–3). Thus, a fish is more likely to succeed and grow to maturity if it grows quickly, and yet individuals of the same species can grow at dramatically different rates even when habitat conditions and food availability are equal (3).
In some species like salmonids, inter-individual differences in growth are reported to be mediated by behavior and the social environment, in which aggressive and dominant fish consistently grow faster and bigger than subordinate individuals (4, 5). This has been attributed to dominant individuals being more competitive for limited food resources, while also chronically elevating the stress levels and energy expenditure of subordinate fish (4–8).
A stress-induced elevation in blood cortisol is thought to play some role in the poorer growth performance of subordinate fish (8, 9), potentially causing dysfunction of the digestive system (5, 6, 10). Evidence for this stems from a study by Gilmour et al. (9), which reported that blocking glucocorticoid receptors in rainbow trout (Oncorhynchus mykiss) significantly improved the specific growth rate (SGR; % increase/day) of subordinate fish.
It follows that inter-individual differences in growth rate could be altered by modifying the social environment. It could be expected that pitting dominant individuals against each other would attenuate their growth, while placing subordinate phenotypes together would allow at least some of them to assume dominant, fast-growing phenotypes. While the reciprocal relationship between size and dominance is well-studied, we are unaware of any study that has manipulated the social environment to investigate the plasticity of this relationship within individual fish.
Metabolic rates have also been implicated in mediating inter-individual differences in growth rates of fish. While heightened routine metabolic rate (RMR) has been linked with a socially mediated stress response and reduced growth in subordinate fish (11, 12), links between metabolism and growth have also been revealed in fish housed individually without competition for food (13). There appears to be a metabolic underpinning to growth, but it may be masked by prevailing social contexts.
The present study provides a first step to exploring the growth consequences of manipulated social structures and the potential roles of metabolism in governing these relationships. Using wild-caught Galaxias maculatus as our model, we first screened 30 individuals across five tanks for their growth rate across 3 months and then pooled the five fastest growers in one tank and the five slowest growers in another (Supplementary Figure S1). The growth impacts of the new social environment were assessed for all fish across 3 months before the fast- and slow-growers rejoined their original conspecifics for a final 3 months. Metabolic traits of all fish were taken during the period when fast- and slow-growers were pooled and again at the conclusion of the study.
We hypothesized that (1) initially fast-growing fish would slow in growth once pooled with other apparently competitive fast-growers, (2) at least some of the initially slow-growing fish would assume a dominant competitive role and exhibit enhanced growth rates when pooled with other slow-growers, and (3) initially intermediate-growing fish would have an overall increase in growth rate once the fastest-growing (and apparently most competitive) fish were removed. While the metabolic correlates of growth were a secondary objective of the study, we hypothesized that (4) standard metabolic rate (SMR) and RMR would correlate positively with the growth rate achieved in the 3-month period preceding the metabolic measurements.
All research was conducted in compliance with the guidelines set by the Deakin University Animal Ethics Committee (permits B27-2018 and B31-2022) as well as the Australian Code for the Care and Use of Animals for Scientific Purposes as determined by the Australian Federal Government.
In late May 2022, N = 86 juvenile common galaxias (Galaxias maculatus; length 71 ± 7.9 mm; mean ± SD) were collected using box traps from the Cumberland River, Lorne, Victoria, Australia and placed in an aerated transport tank (water temperature 9.1°C, salinity <0.2 ppt). G. maculatus is a temperate estuarine fish prolific throughout the Southern Hemisphere. As a species, they are gregarious and show pronounced variability in life history traits, including growth and maturation (14–17). They are not known to have high levels of intra-specific conflict or aggression, and preferentially form shoals (18–20).
The fish were brought by car to Deakin University's Queenscliff Marine Science Centre. Remaining in the transport tank, the fish were gradually warmed over the following day to reach the target temperature of 18°C at a salinity of 10 ppt, before they were transferred to the long-term holding system. The system consisted of a rack supporting 15 opaque plastic, rectangular, 25 L tanks. The rack of tanks recirculated independently, draining into a 200 L sump where water passed through a particle filter, a biological filter, and a protein skimmer before being pumped back up to the tanks via an inline UV sterilizer. This thorough filtration and sterilization ensured that the recirculating water was cleaned during each cycle and allowed each tank to be treated as an independent replicate. The sump was supplied with a light inflow of both fresh water and sea water (total flowthrough ~2,000 L/day), balanced to maintain an estuarine salinity of ~10 ppt. The lights in the room were set on a timer to come on at 06:30 and turn off by 18:30, with a one-hour ramp-up or -down period to simulate sunrise and sunset, respectively. The fish were randomly distributed across five of the tanks in the rack system in groups of 17–18 and initially fed every second day for a week with ~0.08 g/individual of a commercial pellet feed (Otohime C2, BMAQUA, Frederickton, Australia).
After 1 week of acclimation to lab conditions, n = 30 fish (mass: 1.427 ± 0.545 g) were randomly selected from each of the five holding tanks and individually lightly anesthetized in a 0.3 ml/L AQUI-S solution in water (AQUI-S, Lower Hutt, New Zealand). First, wet mass to the nearest 0.01 g and total length to the nearest 1 mm were measured, then fish were laterally injected subcutaneously on either the left, right, or both sides with a visible implant elastomer tag in yellow or red (Northwest Marine Technology, Anacortes, USA). Tag position and color were recorded alongside the size metrics for each individual, and the fish were distributed evenly across five new tanks such that no tag color and position combination was repeated in a tank. One randomly chosen un-tagged and un-measured fish was added to each tank as a procedural control to assess post-tagging survival. No post-tagging mortalities were observed, resulting in a total experimental sample size of N = 35 or n = 7 fish per tank. All remaining fish were held separately and used in a separate experiment.
In Stage 1 and the two subsequent stages, the fish in each tank were fed to satiation with the abovementioned feed every second day. An average of 4.6% of tank biomass was delivered in feed every second day to each tank over the course of the study, with the amount of feed increasing to compensate for increases in fish mass at each stage. This ensured that meals continued to provide satiation as the fish grew. With the exception of feeding and cleaning, fish were left undisturbed during this initial growth period.
Stage 2 of the experiment began on September 7th, 2022, once fish had 3 months (98 days) to grow, at which point all 35 fish were lightly anesthetized to measure their wet mass and total length. This was the first size measurement for the five un-tagged fish, so growth rate for those individuals could only be calculated from this point forward. For the remaining 30 fish, specific growth rate (SGR) was calculated as:
where SGR is in units of % body mass gained per day, mass1 and mass2 are the body masses of an individual at the start and end of the growth period, respectively, and ln is the natural logarithm.
The SGR of each individual was used to rank the population from fastest- to slowest-growing. After recovering in their initial tanks for 48 h, n = 5 fish identified as the fastest growers among the population were removed and placed together in a new tank with identical water conditions and the same feed availability as previously. The n = 5 slowest growing fish, excluding one outlier with visibly poor health, were also removed and placed together in a different new tank with the same conditions. Because the two newly established tanks combined fish from across four of the five initial tanks, some elastomer tag color and position combinations were repeated within the new groupings. To maintain individual-level identification capabilities, all 10 moved fish were photographed and differences in their tag shapes and positions along the body were noted. This left n = 20 intermediate-growing fish and n = 5 untagged controls across the five original tanks, which had new population sizes ranging between n = 4 to n = 7 (Supplementary Figure S1).
On November 22–24, 2022, following 76–78 days in their new social groupings, measurements of individual metabolic performance were taken for all 35 individuals (see Section 2.5 below). This stage also included group behavioral monitoring using GoPro cameras (see Section 2.6 below). The feeding regime was interrupted once per tank to ensure fish were fasted for >24 h prior to metabolic measurements, but otherwise continued on schedule.
After 3 months of the fast-, intermediate- and slow-growers being separated, on December 15, 2022, each individual's length and mass were recorded once again and the fast- and slow-growing fish were returned to their original tanks detailed in Stage 1. Once all fish were returned to their initial groupings, we took a second measurement of metabolic performance for each individual and continued to video-monitor the behavior of the five tanks.
The experiment concluded at the end of the metabolic measurements on March 18, 2023, and all fish were euthanized in a lethal AQUI-S solution (0.6 ml/L).
We used an intermittent-flow respirometry system to measure whole animal oxygen uptake rate (ṀO2) for each individual fish (n = 35) as a proxy for aerobic metabolic rate [see Clark et al. (21) for best practices].
The respirometry system consisted of four aerated holding trays (900 × 500 × 200 mm) filled with 65 L of water recirculating from a shared 105 L sump held at the same temperature and salinity as the holding tanks in the rack system. Each holding tray contained four submerged respirometry chambers of adjustable volume (0.7–1.35 L depending on number of watertight displacers used), consisting of plastic containers with clip-down lids and equipped with a recirculation loop to maintain thorough mixing in the chamber as well as constant water flow over an oxygen sensor (Firesting, PyroScience, Aachen, Germany) positioned within the loop. The sensor recorded oxygen concentration (mg/L) every 2 s to a laptop running Oxygen Logger software (PyroScience, Aachen, Germany). Each group of four respirometers was intermittently supplied with water from their respective holding tray via an Eheim flush pump connected to a timer on a 10 min:10 min on:off cycle (Smart_shifter, National Instruments, Austin, USA). This allowed for continuous measurements of ṀO2 across all 16 respirometers every 20 min between flush cycles. All respirometry chambers were thoroughly tested for leaks, and background respiration was recorded for at least 30 min before and after each trial.
Fish were fasted for at least 24 h before being netted from their holding tank and introduced to a respirometry chamber. For each fish, the tag ID and tank number were recorded before the fish was vigorously chased in a large tub. The chase was designed to elicit maximum metabolic rate (MMR) and consisted of swirling the water in one direction before chasing the fish against the current by hand. All fish were chased for 2 min, then air exposed while they were weighed (~30 s). Each fish was then placed in an empty respirometry chamber and immediately sealed from flushing using a manual valve. Smaller fish (<2 g) were placed in chambers with more displacers (lower chamber volume), while the largest fish (>5 g) were placed in empty chambers. Chambers were left sealed for MMR measurements for ~20 min and never more than 30 min.
Once MMR was measured for all fish in a trial, the manual valves were reopened and the chambers continued on an automated 10 min:10 min flush:seal cycle while fish recovered from the chase. The trays were covered with black plastic sheets to minimize visual disturbance of the recovering fish, and the system ran undisturbed for at least 16 h to obtain a measure of standard metabolic rate (SMR) for each individual. The lights in the room were set on a timer identical to those in the holding tank room, including the simulated sunrise and sunset. All fish were removed from the respirometry chambers the following morning and returned to their holding tanks in the rack system.
While fish were disturbed as little as possible during Stage 1, behavioral monitoring commenced in Stage 2, using GoPro Hero 11 cameras (GoPro, Inc., San Mateo, California, USA) to record video of each tank at feeding and non-feeding times to quantify feeding attempts and aggressive behaviors (chasing, biting) that could help explain discrepancies in access to food or differences in growth. A camera was placed in a tank at least 2 h prior to recording, allowing the fish to become calm and accustomed to its presence. The cameras were then remotely powered on via Bluetooth, or very briefly removed from the water to be switched on.
The videos were recorded either just before feeding each tank, or at approximately feeding time on a non-feeding day. Video was recorded for the full length of the camera battery, ~90 min. Recordings also continued into Stage 3 to look for differences in behavior after fish were returned to their initial social groups, used to capture the behavioral baseline in mixed-performance groups. Three cameras were rotated among all seven tanks, resulting in 22 recordings taken between November 16 and December 21, 2022.
Statistical analyses were conducted using R version 4.3.2 (22).
Specific growth rates were calculated for each fish over each 3-month stage (June to September 2022, September to December 2022, and December 2022 to March 2023). One individual SGR measurement in the September to December stage was excluded from the analysis as an outlier with the only z-score >|3.29| in the distribution. A z-score is the number of standard deviations away from the mean a value falls, and only one in 1,000 observations exceeds |3.29| in a normal distribution. ANOVA tests were conducted to look for differences in SGR across stages, tank groups, and between fast-, slow- and intermediate-growers (Supplementary Figure S2).
Using the lme4 package in R (23), the relationship between initial mass and SGR across each stage was modeled logarithmically, with fish ID included as a random effect to account for repeated measurements of individuals across the stages:
Residuals were generated for each SGR value from this model, and these residuals were then used in place of SGR values in all further analyses to account for the natural decline in SGR with increasing mass (see Section 3).
To analyze metabolic data, raw data from Firesting .txt files were first reformatted for LabChart (ADInstruments, Sydney, Australia) using Excel. In LabChart, raw slopes of oxygen (mg/L) per unit time were selected from each channel over a 4-min interval in the middle of each sealed period to ensure linearity, then ṀO2 was calculated from each slope after accounting for respirometer chamber volume and background respiration (calculated dynamically using a linear relationship between the background measurement taken before and after each trial).
For each fish, SMR was calculated as the mean of the lowest 10% of ṀO2 values, and MMR was taken as the highest 3-min ṀO2 recorded in the 20-min interval immediately following the chase protocol. Routine metabolic rate (RMR) was calculated as the mean of all slopes, after excluding the first 2 h of measurements post-chase. SMR, RMR, and MMR values were mass-standardized across the experiment to the overall mean mass of 6.06 g by first producing power regression analyses between body mass and the respective metabolic rate. The mass-standardized values were then calculated for each of SMR, RMR and MMR by taking each individual's residual and adding this to the regression-predicted value for a 6.06 g fish.
A Bonferroni outlier t-test of the distribution of studentized residuals identified one influential outlier among the mass-standardized SMR data collected in Stage 2 (November), which was then excluded from further analyses but, for transparency, included in plots of raw data as a gray point. We then tested for linear correlation with Pearson's coefficient between SGR and each of mass-standardized SMR, RMR, and MMR at both the Stage 2 (November) and Stage 3 (March) timepoints.
To analyze the behavioral videos for the n = 2 isolation tanks, two independent observers counted the number of burst swimming events and the number of feeding events (n = 8 recordings, across four dates with paired recordings for the two tanks). The same procedure was conducted for the remaining n = 5 tanks by one observer. All observations started at the beginning of the recording and covered 1 min out of every 5 min (e.g. 0:00–1:00, 5:00–6:00, etc.). Because the camera field did not capture the entire tank, the number of fish observed in each clip was also noted. Counts were generally consistent between the two observers in the n = 8 instances when the videos were analyzed by two people. Where they differed, a consensus value was reached after re-watching the clip. Where a consensus could not be reached but values were reasonably close, a simple mean was taken. The total number of burst swimming events for a recording was then divided by the number of minutes watched to calculate bursts per minute. A t-test was conducted to assess the difference in bursts per minute between the isolation tanks, although we caution that this assessment is limited to observations of only one tank per growth phenotype category, and does not account for random tank effects.
We found G. maculatus had highly pronounced variability in their growth performance. While individual mass initially ranged from 0.78 to 2.73 g, by the end of the experiment it spanned from 1.70 to 10.08 g. Specific growth rate (SGR) during Stage 1 ranged from 0.50 to 1.73% (excluding the visually unhealthy fish with an SGR of −0.45%). The five slowest growers were characterized by an SGR (0.78 ± 0.19%; mean ± SD) that was less than half that of the five fastest growers (1.59 ± 0.12%; Supplementary Table S1). There was a natural decline in SGR as fish grew (Figure 1A), which is common within most fish species (24) and resulted in significantly higher SGR in Stage 1 relative to Stages 2 and 3 (Supplementary Figure S2). After accounting for this background decline in SGR by calculating residuals from a logarithmic model (see Section 2.7; Figure 1B), we tested our initial hypotheses aimed at determining the growth consequences of altered social environments.
Figure 1. Specific growth rate (SGR) (A) and model residuals of SGR (B) as a function of body mass for Galaxias maculatus across the three stages of the study (body mass taken at the beginning of each growth interval stage). Note that the logarithmic model in (A) is for visual purposes, while the values in (B) are from the formal model incorporating Fish ID as a random effect (Equation 2). Growth phenotype categorization is indicated by color (fast-, slow- and intermediate-growers), and Stages 1–3 are represented by different shapes. Unfilled shapes indicate untagged control fish.
Following the separation of growth phenotypes, we found all five of the fish that grew fastest initially displayed negative SGR residuals during Stage 2 (Figure 1B). This lends weight to our first hypothesis that initially fast-growing fish would slow in growth when they were made to compete against each other in the same tank. The behavioral footage gave no indication that this slower growth period was characterized by aggressive interactions between individuals, as we observed very few antagonistic encounters and similar levels of feeding among the fast-growers relative to the slow-growing fish (Figure 2; Supplementary Table S2). While consistent with previous descriptions of the species' behavior, this near-complete lack of competitive interactions observed in the video footage was surprising, as we had expected the fastest growers to also be the most antagonistic.
Figure 2. Burst swimming (chasing, fleeing, or otherwise startling) events observed per min in each video analysis of Galaxias maculatus during Stage 2 (Nov–Dec 2022) and Stage 3 (Dec 2022–Mar 2023) of the study. The tanks containing only fast- or slow-growing fish during Stage 2 are shown at the left of the figure in green and red, respectively (T1 and T2), while the other five tanks contained only intermediate-growers during this stage. T1 and T2 differed significantly in their bursts per minute, indicated by * symbol (t = −3.389, df = 3.0063, p = 0.04). Stage 3 saw fast- and slow-growers rejoin intermediate-growers in their original tanks (represented by tri-color symbols). One tank (T3) did not contain any fast- or slow-growers from the outset, and therefore there were no changes to its social structure over time.
With the current dataset, we are unable to identify whether the success of the initially fast-growers was due to them allocating less energy to aggressive activity or whether they did not need to expend energy in aggression because they were already growing successfully. It is possible their growth rates can be attributed to some combination of the two, where they showed more dominant behaviors early in life, but calmed after reaching a threshold size. This idea finds support in a study by Farr (25), which concluded that dominance and fast growth were most important earlier rather than later in an individual's life history for determining fitness among mature metallic livebearer fish (Girardinus metallicus).
Our second hypothesis predicted that initially slow-growing fish would exhibit substantial growth benefits from being isolated from fast-growing competitors. In contrast, when the five initially slowest-growing fish were placed together in a tank for 3 months for Stage 2, they continued to grow poorer than predicted for their size (all five fish had negative residuals; Figure 1B). Surprisingly, despite our assumption that these slow-growers would be timid and relatively inactive, the video footage revealed that they exhibited the most bursting activity of all growth phenotypes, associated with competitive interactions (Figure 2; Supplementary Table S2). A t-test showed that the slow-growers exhibited significantly more burst behaviors per minute relative to the fast growers (t = −3.389, df = 3.0063, p = 0.043).
These findings for the slow-growers suggest they were following a different life history strategy relative to their faster-growing conspecifics, as no individual took advantage of being pitted against other slow-growers to dramatically improve their growth performance. That is, growth did not appear to be a priority for these slow-growing phenotypes, despite our prediction that they would exhibit “compensatory” growth, a phenomenon whereby fish experience accelerated growth following a period of food restriction [reviewed in Ali et al. (26)]. Our observations are generally consistent with the findings of Segers and Taborsky (27), who reported that compensatory growth was not possible for fish in highly competitive or aggressive social environments. The effect of social groupings on growth is likely species-specific, depending on their habits in the wild and the magnitude of their stress responses. For example, while Huang et al. (28) found that crucian carp (Carassius carassius) grew faster in isolation than in groups, Sun et al. (29) saw the opposite in grass carp (Ctenopharyngodon idella), despite both species showing social tendencies in the wild.
Many of the fish with initially intermediate growth assumed the fastest-growing phenotypes throughout Stage 2 (Figure 1B). This supports our third hypothesis that initially intermediate-growers would improve their growth performance when the fastest-growing individuals were removed, demonstrating a socially-mediated example of compensatory growth. The behavioral footage for the initially intermediate-growers generally showed fewer aggressive encounters relative to the tank of isolated slow-growers, but numerically more than the tank of fast-growers, although the low sample size prevents a statistical assessment of this difference (Figure 2). Due to the nature of the experiment, group size became an unavoidable confounding factor on the growth performance of the intermediate-growing fish. Following the removal of fast- and slow-growers during Stage 2, tank populations ranged from 4 to 7 fish. The growth performance was not statistically different between the intermediate growth tanks here (Supplementary Figure S2), but future experiments should consider also isolating a fixed sample of fish from the middle of the growth performance spectrum to control for group size.
When all fish were returned to their original tanks for Stage 3, there was a general increase in growth rate, although SGR of all tanks was still below the levels seen in Stage 1 (Figure 1; Supplementary Table S1; Supplementary Figure S2). Whereas the residual SGR for initially slow-growers was −0.28 ± 0.13% (mean residual ± SD) in Stage 2, it improved to 0.01 ± 0.09% throughout Stage 3. Initially fast-growers improved from an SGR residual of −0.21 ± 0.08% during Stage 2, to a mean positive residual of 0.04 ± 0.06% throughout Stage 3, and initially intermediate-growers improved their SGR residuals from −0.06 ± 0.17% in Stage 2 to 0.11 ± 0.16% in Stage 3 (Figure 1; Supplementary Table S1). Many initially intermediate-growers continued to outperform the initially fast-growers in Stage 3, as they did in Stage 2, presumably as a result of our social interventions to modify the dominance structure in the tanks.
We found evidence that SMR and RMR (but not MMR) played some role in determining SGR residuals (Figure 3), but these trends were only significant for the first respirometry period (Stage 2; Figures 3B, C). These results support previous work which found positive correlations between SGR and each of SMR (13, 30) and RMR (31–34), although contradicting results exist (35–38).
Figure 3. Residuals of specific growth rate (SGR) as a function of maximum metabolic rate (MMR) (A, D), routine metabolic rate (RMR) (B, E), and standard metabolic rate (SMR) (C, F) for both November 2022 (A–C; Stage 2) and March 2023 (D–F; Stage 3) respirometry periods. SGR residuals were calculated over the 3-month interval that includes each metabolic measurement, and fast/intermediate/slow categorization was based on initial SGR determinations between June and September 2022 (Supplementary Table S1). Two points in November were identified as outliers and therefore excluded from the data analysis but have been retained as gray points in the plots (note broken axes shown in red in B and C). Unfilled shapes indicate untagged control fish. Dashed line is a linear model, R and p-values are from a Pearson's correlation test.
The relatively low strength of the relationships found here and across other studies in fish suggests that metabolism is only one of several contributors to inter-individual variability in growth performance, with complex interactions from the social environment. Indeed, as our results attest, the growth rates of individuals within social groups are likely to be governed by inherent life history traits (i.e., genetic factors), physiological factors (e.g., metabolism), and the magnitude of competitive behavioral interactions, several of which may change as fish achieve threshold sizes or life stages.
The challenge of quantifying the role of the social environment on individual metabolic traits is that respirometry is typically performed on individuals, or else metabolic measurements can be partitioned between individuals in group respirometry protocols based on various assumptions. Neither of these methods provides a true indication of the impact of social interactions on the metabolic rates of individuals (39). Millidine et al. (40) proposed a way of overcoming this limitation by estimating individual RMR from opercular beat frequency (OBF). This remains an interesting avenue to pursue but would rely on continuous or frequent measurements of OBF as well as building a robust relationship between metabolism and OBF at an individual level.
To our knowledge, this study is the first to take repeated measurements of both inter- and intra-individual variability in growth performance under changing social conditions, building on previous research that has identified phenotypic plasticity in inter-individual growth rates in response to sexual competition (41), social isolation (29, 42), environmental condition (43), and feeding regimes (26, 27).
This experiment was conducted to elucidate areas of particular interest for understanding the roles of the social environment and the metabolic phenotype in determining growth performance. With this baseline, we have been left with a range of questions that we believe will help direct the field toward larger-scale experiments aimed at resolving the drivers of differential growth performance within fish populations:
1. What is the diversity of growth phenotypes within fish populations, and is there evidence for genotypes that do not prioritize growth?
2. Are all slow-growers “subordinate” or can this be a successful life history strategy?
3. How does manipulating the social environment mediate growth phenotypes, and what circumstances allow “losers” to become “winners”?
4. Can early-life intra-individual screening of metabolic parameters yield accurate predictions of subsequent growth?
5. How do the relationships between social status and metabolism interact to govern growth as an individual progresses through its life history?
This study unearthed a range of unexpected findings that we believe are exciting and warrant further investigation on a larger scale. We hope our study sparks interest in this topic and leads to a better understanding of the drivers of inter-individual growth performance in wild fish populations, perhaps with lessons for selecting fast-growing phenotypes in aquaculture and fish stocking programs.
The datasets presented in this study can be found in online repositories. The names of the repository/repositories and accession number(s) can be found at: https://github.com/Bhoots99/Galaxiids.
The animal study was approved by Deakin University Animal Ethics Committee. The study was conducted in accordance with the local legislation and institutional requirements.
EH: Conceptualization, Data curation, Formal analysis, Investigation, Methodology, Project administration, Visualization, Writing – original draft, Writing – review & editing. JD: Formal analysis, Methodology, Writing – review & editing, Data curation, Investigation. LK: Formal analysis, Investigation, Methodology, Writing – review & editing. MS: Formal analysis, Investigation, Methodology, Writing – review & editing. TC: Formal analysis, Methodology, Writing – review & editing, Conceptualization, Funding acquisition, Resources, Supervision, Visualization, Writing – original draft.
The author(s) declare financial support was received for the research, authorship, and/or publication of this article. This work was supported by an Australian Research Council Future Fellowship to TC (FT180100154) funded by the Australian Government. EH, LK, and MS were funded by Deakin University Postgraduate Research Scholarships. LK was also supported by the Cawthron Institute. JD was funded by a Deakin University Faculty of Science, Engineering and Built Environment Associate Dean Research 2022 Summer Project Prize.
We thank Sam Wines and Lisa Grubb for their technical support.
The authors declare that the research was conducted in the absence of any commercial or financial relationships that could be construed as a potential conflict of interest.
All claims expressed in this article are solely those of the authors and do not necessarily represent those of their affiliated organizations, or those of the publisher, the editors and the reviewers. Any product that may be evaluated in this article, or claim that may be made by its manufacturer, is not guaranteed or endorsed by the publisher.
The Supplementary Material for this article can be found online at: https://www.frontiersin.org/articles/10.3389/frish.2024.1482878/full#supplementary-material
1. Duk K, Pajdak J, Terech E, Szarek J. Intracohort cannibalism and methods for its mitigation in cultured freshwater fish. Rev Fish Biol Fish. (2017) 27:193–208. doi: 10.1007/s11160-017-9465-2
2. Stige LC, Rogers LA, Neuheimer AB, Hunsicker ME, Yaragina NA, Ottersen G, et al. Density- and size-dependent mortality in fish early life stages. Fish Fish. (2019) 20:962–76. doi: 10.1111/faf.12391
3. Goodrich HR, Clark TD. Why do some fish grow faster than others? Fish Fish. (2023) 24:796–811. doi: 10.1111/faf.12770
4. Abbott JC, Dill LM. The relative growth of dominant and subordinate juvenile steelhead trout (Salmo gairdneri) fed equal rations. Behaviour. (1989) 108:104–13. doi: 10.1163/156853989X00079
5. DiBattista JD, Levesque HM, Moon TW, Gilmour KM. Growth depression in socially subordinate rainbow trout Oncorhynchus mykiss: more than a fasting effect. Physiol Biochem Zool. (2006) 79:675–87. doi: 10.1086/504612
6. Peters G. The effect of stress on the stomach of the European eel. Anguilla anguilla L. J Fish Biol. (1982) 21:497–512. doi: 10.1111/j.1095-8649.1982.tb02855.x
7. Metcalfe NB, Wright PJ, Thorpe JE. Relationships between Social Status, Otolith Size at first feeding and subsequent growth in Atlantic Salmon (Salmo salar). J Anim Ecol. (1992) 61:585–9. doi: 10.2307/5613
8. Øvrli Ø, Harris CA, Winberg S. Short-term effects of fights for social dominance and the establishment of dominant-subordinate relationships on brain monoamines and cortisol in rainbow trout. Brain Behav Evol. (1999) 54:263–75. doi: 10.1159/000006627
9. Gilmour KM, DiBattista JD, Thomas JB. Physiological causes and consequences of social status in salmonid fish. Integr Comp Biol. (2005) 45:263–73. doi: 10.1093/icb/45.2.263
10. Madison BN, Tavakoli S, Kramer S, Bernier NJ. Chronic cortisol and the regulation of food intake and the endocrine growth axis in rainbow trout. J Endocrinol. (2015) 226:103–19. doi: 10.1530/JOE-15-0186
11. Sloman KA, Motherwell G, O'Connor KI, Taylor AC. The effect of social stress on the Standard Metabolic Rate (SMR) of brown trout, Salmo trutta. Fish Physiol Biochem. (2000) 23:49–53. doi: 10.1023/A:1007855100185
12. Grobler JMB, Wood CM. The physiology of rainbow trout in social hierarchies: two ways of looking at the same data. J Comp Physiol B. (2013) 183:787–99. doi: 10.1007/s00360-013-0752-5
13. Norin T, Malte H, Clark TD. Differential plasticity of metabolic rate phenotypes in a tropical fish facing environmental change. Funct Ecol. (2016) 30:369–78. doi: 10.1111/1365-2435.12503
14. McDowall RM. Galaxias maculatus (Jenyns), the New Zealand whitebait. Morehead City, NC; Fisheries Research Division, Marine Department (1968).
15. Barbee NC, Hale R, Morrongiello J, Hicks A, Semmens D, Downes BJ, et al. Large-scale variation in life history traits of the widespread diadromous fish, Galaxias maculatus, reflects geographic differences in local environmental conditions. Mar Freshw Res. (2011) 62:790–800. doi: 10.1071/MF10284
16. Rojo JH, Boy CC. Life-history traits in the southernmost landlocked population of the fish Galaxias maculatus. Polar Biol. (2022) 45:1093–103. doi: 10.1007/s00300-022-03064-x
17. Skeeles MR, Scheuffele H, Clark TD. Supplemental oxygen does not improve growth but can enhance reproductive capacity of fish. Proc R Soc B Biol Sci. (2023) 290:20231779. doi: 10.1098/rspb.2023.1779
18. Higgins KL. Social Behaviour, Aggression and the Indirect Effects of Competition and Predation in the Fish Species Galaxias maculatus and Gambusia holbrooki. Hobart, TAS: University of Tasmania (2021).
19. Rowe DK, Smith JP, Baker C. Agonistic interactions between Gambusia affinis and Galaxias maculatus: implications for whitebait fisheries in New Zealand rivers. J Appl Ichthyol. (2007) 23:668–74. doi: 10.1111/j.1439-0426.2007.00912.x
20. Bonnett ML, Mc Intosh AR. The influence of juvenile brown trout (Salmo trutta) on habitat use of inanga (Galaxias maculatus) in a stream simulator. J R Soc N Z. (2004) 34:357–67. doi: 10.1080/03014223.2004.9517772
21. Clark TD, Sandblom E, Jutfelt F. Aerobic scope measurements of fishes in an era of climate change: respirometry, relevance and recommendations. J Exp Biol. (2013) 216:2771–82. doi: 10.1242/jeb.084251
22. R Core Team. R: A Language and Environment for Statistical Computing. Vienna: R Foundation for Statistical Computing (2023). Available at: https://www.R-project.org/
23. Bates D, Mächler M, Bolker B, Walker S. Fitting linear mixed-effects models using lme4. J Stat Softw. (2015) 67:1–48. doi: 10.18637/jss.v067.i01
24. Hopkins KD. Reporting fish growth: a review of the basics. J World Aquac Soc. (1992) 23:173–9. doi: 10.1111/j.1749-7345.1992.tb00766.x
25. Farr JA. The effects of juvenile social interaction on growth rate, size and age at maturity, and adult social behavior in Girardinus metallicus Poey (Pisces: Poeciliidae). Z Tierpsychol. (1980) 52:247–68. doi: 10.1111/j.1439-0310.1980.tb00715.x
26. Ali M, Nicieza A, Wootton RJ. Compensatory growth in fishes: a response to growth depression. Fish and Fisheries. (2003) 4:147–90. doi: 10.1046/j.1467-2979.2003.00120.x
27. Segers FHID, Taborsky B. Competition level determines compensatory growth abilities. Behav Ecol. (2012) 23:665–71. doi: 10.1093/beheco/ars013
28. Huang Y, Fu S, Cooke SJ, Xia J. Is repeatability of metabolic rate influenced by social separation? A test with a teleost fish. Biol Lett. (2020) 16. doi: 10.1098/rsbl.2019.0825
29. Sun C-Y, Fu S-J, Zeng L-Q. Social isolation does not alter the relationship between flexibility in metabolic rate and growth in grass carp (Ctenopharyngodon idella) under changing food availability. J Comp Physiol B. (2022) 193:95–108. doi: 10.1007/s00360-022-01467-0
30. Boldsen MM, Norin T, Malte H. Temporal repeatability of metabolic rate and the effect of organ mass and enzyme activity on metabolism in European eel (Anguilla anguilla). Comp Biochem Physiol. (2013) 165:22–9. doi: 10.1016/j.cbpa.2013.01.027
31. Yamamoto T, Ueda H, Higashi S. Correlation among dominance status, metabolic rate and otolith size in masu salmon. J Fish Biol. (1998) 52:281–90. doi: 10.1111/j.1095-8649.1998.tb00799.x
32. McCarthy ID. Temporal repeatability of relative standard metabolic rate in juvenile Atlantic salmon and its relation to life history variation. J Fish Biol. (2000) 57:224–38. doi: 10.1111/j.1095-8649.2000.tb00788.x
33. Fu S-J, Cao Z-D, Peng J-L. The stability of standard metabolic rate and its relationship to growth performance variation in southern catfish. Silurus meridionalis Chen. J Fish Aquat Sci. (2007) 2:162–7. doi: 10.3923/jfas.2007.162.167
34. Hoogenboom MO, Armstrong JD, Groothuis TGG, Metcalfe NB. The growth benefits of aggressive behavior vary with individual metabolism and resource predictability. Behav Ecol. (2013) 24:253–61. doi: 10.1093/beheco/ars161
35. Cutts CJ, Metcalfe NB, Taylor AC. Aggression and growth depression in juvenile Atlantic salmon: the consequences of individual variation in standard metabolic rate. J Fish Biol. (1998) 52:1026–37. doi: 10.1111/j.1095-8649.1998.tb00601.x
36. Moyano M, Illing B, Christiansen L, Peck MA. Linking rates of metabolism and growth in marine fish larvae. Mar Biol. (2018) 165. doi: 10.1007/s00227-017-3252-4
37. Cornwell TO, McCarthy ID, Biro PA. Integration of physiology, behaviour and life history traits: personality and pace of life in a marine gastropod. Anim Behav. (2020) 163:155–62. doi: 10.1016/j.anbehav.2020.03.009
38. Chrétien E, Cooke SJ, Boisclair D. Does shelter influence the metabolic traits of a teleost fish? J Fish Biol. (2021) 98:1242–52. doi: 10.1111/jfb.14653
39. Nadler LE, Killen SS, McClure EC, Munday PL, McCormick MI. Shoaling reduces metabolic rate in a gregarious coral reef fish species. J Exp Biol. (2016) 219:2802–5. doi: 10.1242/jeb.139493
40. Millidine KJ, Metcalfe NB, Armstrong JD. Presence of a conspecific causes divergent changes in resting metabolism, depending on its relative size. Proc R Soc B Biol Sci. (2009) 276:3989–93. doi: 10.1098/rspb.2009.1219
41. Buston PM, Cant MA. A new perspective on size hierarchies in nature: patterns, causes, and consequences. Oecologia. (2006) 149:362–72. doi: 10.1007/s00442-006-0442-z
42. Campton DE, Gall GAE. Effect of individual and group rearing on age and size at maturity of male mosquitofish, Gambusia affinis. J Fish Biol. (1988) 33:203–12. doi: 10.1111/j.1095-8649.1988.tb05463.x
Keywords: metabolic rate, specific growth rate, growth phenotype, intra-specific variation, Galaxias maculatus
Citation: Hoots EC, Doling JBN, Kuchenmüller LL, Skeeles MR and Clark TD (2024) Social and metabolic mediation of growth performance in a temperate estuarine fish. Front. Fish Sci. 2:1482878. doi: 10.3389/frish.2024.1482878
Received: 18 August 2024; Accepted: 10 October 2024;
Published: 28 October 2024.
Edited by:
David J. McKenzie, Centre National de la Recherche Scientifique, FranceReviewed by:
Øyvind Øverli, Norwegian University of Life Sciences, NorwayCopyright © 2024 Hoots, Doling, Kuchenmüller, Skeeles and Clark. This is an open-access article distributed under the terms of the Creative Commons Attribution License (CC BY). The use, distribution or reproduction in other forums is permitted, provided the original author(s) and the copyright owner(s) are credited and that the original publication in this journal is cited, in accordance with accepted academic practice. No use, distribution or reproduction is permitted which does not comply with these terms.
*Correspondence: Elizabeth C. Hoots, YmV0aC5ob290c0ByZXNlYXJjaC5kZWFraW4uZWR1LmF1
Disclaimer: All claims expressed in this article are solely those of the authors and do not necessarily represent those of their affiliated organizations, or those of the publisher, the editors and the reviewers. Any product that may be evaluated in this article or claim that may be made by its manufacturer is not guaranteed or endorsed by the publisher.
Research integrity at Frontiers
Learn more about the work of our research integrity team to safeguard the quality of each article we publish.