- 1Shanghai Key Laboratory of Brain Functional Genomics, Key Laboratory of Brain Functional Genomics (Ministry of Education), Affiliated Mental Health Center (ECNU), School of Psychology and Cognitive Science, East China Normal University, Shanghai, China
- 2Department of Neurosurgery, China Medical University, The First People’s Hospital of Kunshan, Suzhou, Jiangsu, China
- 3Gait and Motion Analysis Laboratory, Department of Rehabilitation Sciences, The Hong Kong Polytechnic University, Hong Kong, Hong Kong SAR, China
- 4Department of Neurosurgery, Shanghai Tenth People’s Hospital, Tongji University, Shanghai, China
- 5Phylo-Cognition Laboratory, Division of Natural and Applied Sciences, Data Science Research Center, Duke Kunshan University, Duke Institute for Brain Sciences, Kunshan, Jiangsu, China
- 6Shanghai Key Laboratory of Magnetic Resonance, East China Normal University, Shanghai, China
- 7Shanghai Changning Mental Health Center, Shanghai, China
Metacognitive abilities, the capacity to think about one’s own thinking processes, offer a range of advantages that may drive their evolution in non-primate animals (NPAs). These advantages include enhancing adaptive decision-making in uncertain situations, efficient resource management, error detection and correction, and facilitating complex social interactions and problem-solving. In this comprehensive study, we have chosen two key paradigms — namely, uncertainty monitoring and information-seeking tasks — to study metacognitive phenomena in NPAs. The first paradigm involves an extensive meta-analysis of existing research, shedding light on how NPAs monitor and respond to uncertainty. We then transition to the second paradigm, which focuses on information-seeking behaviors, employing a different analytical approach. Our study aims to provide a holistic understanding of these cognitive processes in NPAs, contributing valuable insights into their cognitive complexity and ecological contexts. Through a coverage of 30 articles spanning 13 different NPA species, we bridge gaps in our understanding of metacognition beyond primates and explore potential divergent evolutionary paths, challenging assumptions about cognitive capability in NPAs.
1 Introduction
Metacognitive abilities offer several advantages that may drive their evolution in diverse species. Firstly, they enhance adaptive decision-making by enabling individuals to assess the certainty of their knowledge or perceptions, allowing for more informed choices, especially in uncertain situations. Secondly, metacognition aids in efficient resource management by helping animals prioritize mental efforts based on task importance or likelihood of success, conserving cognitive resources for tasks with higher rewards. Additionally, metacognition allows for error detection and correction, vital for survival in dynamic environments. Lastly, metacognition is advantageous in situations requiring tool use and complex problem-solving, as it enables animals to reflect on their thought processes and devise effective strategies for novel challenges. These benefits suggest that the evolution of metacognitive abilities in non-human species is closely tied to their cognitive complexity and ecological contexts.
In the absence of linguistic markers, however, it has not been possible to ask animals to report their self-monitoring by means of language. To test animals’ metacognitive ability, a number of paradigms that do not require language have been developed, such as uncertainty responses, betting, confidence ratings, temporal wagering, information-seeking tasks (Call and Carpenter, 2001; Shields et al., 2005; Middlebrooks and Sommer, 2011; Lak et al., 2014; Miyamoto et al., 2017; Cai et al., 2022). Most of these have been used in primates (e.g., macaque monkeys) and have produced evidence suggestive of metacognitive abilities in primates(e.g., Kornell et al., 2007; Hampton, 2001; Miyamoto et al., 2017; Cai et al., 2022). In comparison, paradigms that are applicable for non-primate animals (hereafter called NPAs) are more limited. Without assuming lower cognitive capabilities in NPAs, we acknowledge the variability across species, including the impact of sensory capacities and morphological adaptations on NPA’s task performance. Accounting for species-specific variations and the need for a nuanced evaluation of cognitive abilities, two important tasks have been developed in the NPA literature: the uncertainty responses (“opt-out”) task and the information-seeking task.
The uncertainty monitoring task is the first paradigm used to study metacognition in non-human animals. This was designed to assess the level of confidence or doubt an organism has in the accuracy of its cognitive judgments or decisions. It is fundamentally linked to metacognition, which encompasses the ability to think about one’s own thinking processes. In the context of metacognition, uncertainty monitoring represents an organism’s awareness of its own cognitive states, allowing it to adapt its behavior based on the perceived reliability of its mental processes. The first report of such monitoring behaviors in a NPA species described a bottlenosed dophin (Tursiops truncatus) being able to “opt-out” when the decision threshold was at its perceptual limit in an auditory psychophysical task (Smith et al., 1995). The dolphin’s opt-out behavior indicated its uncertainty in the decision process. The opt-out rate is defined as the within-subjects difference in the percentage of trials declined between difficult trials and easy trials. Similar experiments were conducted to test uncertainty monitoring abilities in rats (Rattus norvegicus), pigeons (Columba livia), large-billed crows (Corvus macrorhynchos), and bees (Apis mellifera).
However, the opt-out tasks usually demand substantial training for the animals. Compared with the uncertainty monitoring paradigm, the information-seeking paradigm offers a simpler alternative. The latter paradigm, which was originally developed for non-human primates (e.g., Call and Carpenter, 2001), is designed to probe the active processes of seeking and evaluating information in the animals. Because the foraging part embedded in this simpler alternative uses searching behaviors that are natural in the animals’ natural behavioral repertoire, there is usually no need to train the subjects to elicit information-seeking behavior (i.e., looking into the tubes, Watanabe and Clayton, 2016). To provide a balanced coverage, we included an analysis of performance data on this task reported in the extant papers and an extended discussion of the results.
In this article, we embark on a comprehensive exploration of metacognition in non-primate animals with a focus on two key paradigms, namely, uncertainty monitoring and information-seeking tasks. The first paradigm, which focuses on uncertainty monitoring, is examined through an extensive meta-analysis that rigorously analyzes existing research. We then transition to our second paradigm, which centers on information-seeking behaviors. In this paradigm, we employ a different analytical approach to uncover insights into how non-primate animals gather and utilize information. By structuring our study in this manner, we aim to provide a coherent and comprehensive understanding of these cognitive phenomena and their potential implications for diverse species.
2 Paradigm 1: uncertainty monitoring
In this paradigm, experimenters granted animals an “opt-out” option to let them decline to perform any given trials, resulting in two key parameters indicative of task success: the chosen-forced advantage and the opt-out rate (Smith et al., 2003). These two parameters are a metric first reported in Teller (1989) and Inman and Shettleworth’s (1999) studies. The prediction is that an animal would perform better in trials where they choose to respond rather than opting out (when an “opt-out” option is available) compared to trials of the same type where there is no option to decline (when an “opt-out” option is not available). During the former condition, the animal would more likely accept trials that it considers itself capable of responding correctly. Under a metacognitive account assumption, the “opt-out” rate should thus be higher in trials with higher difficulty. In the following analysis, the opt-out rate is calculated as the within-subjects difference in the percentage of trials declined between the highest difficulty level and the lowest difficulty level.
Additionally, there are several variants to the opt-out task, as categorized by two factors, namely task order and task domain. The factor “task order” refers to whether a cognitive task is prospective or retrospective in nature. Prospective tasks involve actions or decisions made before an event (i.e., the actual test) occurs, whereas retrospective tasks involve actions or decisions made after the test has taken place. Moreover, regarding the factor “domain”, the test is typically administered to the animals by either assessing their memory performance or by testing their perceptual performance. Memory domain tasks involve assessing an individual’s ability to remember past events or information (e.g., recognition memory), whereas perception domain tasks pertain to assessing an individual’s ability to process and interpret sensory information (e.g., discrimination of stimuli intensity). These two factors will be examined by a modulator analysis in the meta-analysis.
To date, there have been no systematic reviews or meta-analyses exploring NPA’s performance on this metacognitive task, nor the potential moderators contributing to the inconsistencies across different studies. In this study, we collected data from opt-out studies that used the chosen-forced advantage and the opt-out rate to quantify animals’ performance from 11 published articles. We also computed a composite score, which is the average of an individual animal’s chosen-forced advantage and the opt-out rate to offer us a 2-in-1 measure for evaluating its performance.
2.1 Meta-analysis
A meta-analysis approach is the ideal method for understanding NPA animals’ group performance when the findings are discrepant across studies. The strength of a meta-analysis is that it allowed us to run statistical tests on the results. By taking heterogeneity across studies (variability in effect sizes) into account, meta-analyses provide a general and combined estimate of NPA’s performance in the opt-out task. We also included a moderator analysis to examine the variability attributed to some key factors (e.g., species) across studies.
Our aim was to (1) estimate the overall effect size for the chosen-forced advantage, opt-out rate, and composite score across different NPA species; and (2) understand how the factors “species”, “task order” (prospective vs. retrospective), and “task domain” (memory vs. perception) would influence these animals’ performances.
2.1.1 Selection of studies
We followed the conventional procedure for the systematic reviews and meta-analyses (PRISMA) approach for the data collection. We first conducted a literature search for papers that contained empirical studies on NPA performance on the opt-out task prior to October 2020 through PubMed, PsycNET, Web of Science, and ProQuest Dissertations and Theses Global. Search terms included (‘metacognition’) and (‘animals’ or ‘non-primate’). We also manually searched for papers that cited the most relevant reviews (Smith et al., 2003; Hampton, 2009; Smith, 2009) in the field. Articles were included in the meta-analysis if they met the following criteria: (a) written in English; (b) published in journals or dissertations; (c) using a standard opt-out paradigm and reported both chosen-forced advantage and opt-out rate. In cases wherein the chosen-forced advantage and the opt-out rate were not available in the articles, we have reached out to relevant authors and obtained the raw data. In cases wherein we were not able to obtain the original data, we used estimated values based on figures published in the papers. Some studies used a variant of the paradigm, or conducted multiple experimental sessions aiming to understand how advanced these animals’ meta-ability is (e.g., test on an immediate transfer to new stimuli). We will discuss these variants in the discussion section, and these studies/sessions were not included into the standard meta-analysis; and (d) only NPA species were included as subjects. We excluded two unpublished studies (one on pigeons and one on rats, data unavailable), and a study conducted on a dolphin because the study was conducted on one single subject, giving us insufficient sample size to run the analysis. In the end, we included 11 papers covering pigeons, rats, large-billed crows, and bees in the meta-analysis Figure 1 and Table 1.
2.2 Coding of studies
The following information was extracted from each of the included studies: author(s) and publication year; type of publication (journal or dissertation); species; test domain (perception or memory); test order (prospective or retrospective); sample size; chosen-forced advantage; opt-out rate; data status (collected or estimated).
2.3 Calculating effect size
To allow cross-species and cross-experiment comparisons, we evaluated all of the extant data using the metric composed of chosen-forced advantage and opt-out rate. For each individual animal, the chosen-forced advantage is calculated by obtaining the average “percentage correct on chosen trials” minus “percentage correct on forced trials” on all difficulty levels. We expected the chosen-forced advantage to be statistically higher than zero if NPAs were able to use the opt-out option when they feel uncertain. The opt-out rate is calculated as “percentage of tests declined at the highest difficulty level” minus “percentage of tests declined at the lowest difficulty level”. Note that the magnitude of the opt-out rate is tied to the distribution between difficult and easy conditions. While the degree of difficulty varies across studies, it generally falls within a range of levels (e.g., 1 to 4). These levels were systematically calibrated to align with the cognitive capacities of the animals being studied. Therefore, in a within-subjects context, the across-study differences in this regard do not impose biases in the effect size estimation. The composite score is the average of an individual animal’s chosen-forced advantage and the opt-out rate. We extracted the average rate for these three scores, as well as the standard error of the means among the individual animals within-study for the meta-analysis.
2.3 Data analysis
The Comprehensive Meta-Analysis software version 2 (Borenstein, 2005) was used to synthesize the data and perform the statistical analysis. Due to the heterogeneity in sampling methods, assessment instruments, and sample size across studies, a random-effects model was used to estimate the effect size for the three scores. Cochran’s Q and the I2 statistic was used to assess the degree of heterogeneity across included studies. Subgroup analyses were used to examine the sources of heterogeneity and the key moderators that contributed to the heterogeneity. Publication bias was evaluated with the funnel plots and Egger’s test.
Moreover, we obtained the species’ absolute brain weight and number of neurons in the brain from the published source (Herculano-Houzel, 2011) and ran correlation analyses correlating the species’ chosen-forced advantage, opt-out rate, and composite score with their brain weight and number of neurons in the cortex.
3 Results
We report the main results in Figure 2, in which effect sizes for each individual study, their relative weights, as well as the overall aggregated effect size are estimated. From the eleven studies that we obtained the three scores, the pooled effect size for chosen-forced advantage, opt-out rate, and composite score are 0.060 (95% CI: 0.028 to 0.092, I2 = 91.823%), and 0.297 (95% CI: 0.154 to 0.440, I2 = 93.957%), and 0.173 (95% CI: 0.112 to 0.233, I2 = 94.458%) respectively, indicating a small but significant effect size. Take the composite score as an example (Figure 2, bottom panel). It shows all studies (except pigeons reported Inman and Shettleworth, 1999), as well as the overall estimation, produced a significantly positive effect size. This result therefore led us to conclude that the species in question are capable of uncertainty monitoring. We also visualized the distribution of all the data in Figure 3, showing that most animals have positive scores in both measurements.
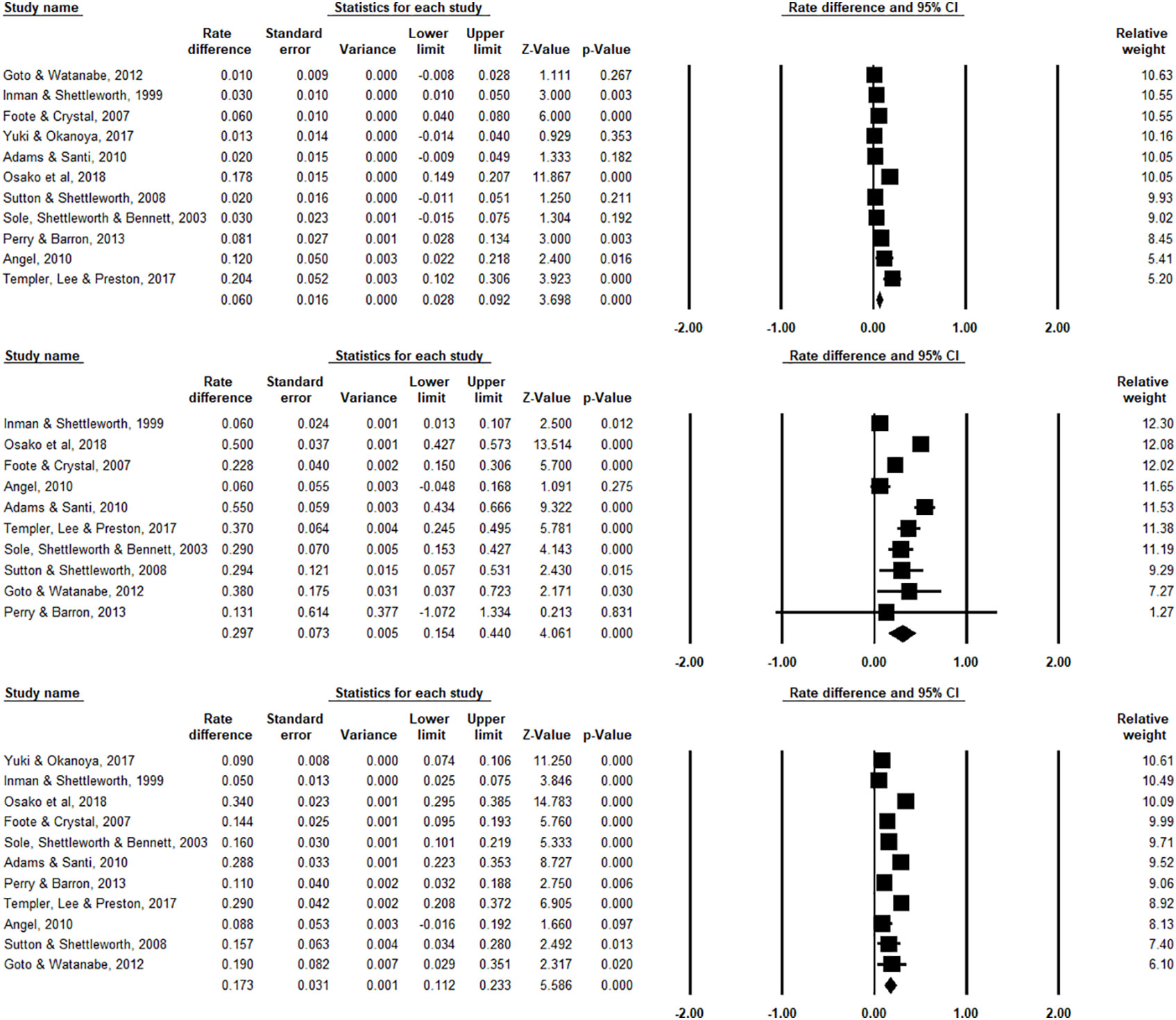
Figure 2 Forest plots for chosen-forced advantage (top panel), opt-out rate (middle panel), and composite score (bottom panel). An effect size is estimated for each study, and the relative weight represents (rightest column) how much this specific study contributed to the overall analysis. Horizontal lines show 95% confidence intervals. Importantly, this analysis provided us with the overall pooled effect size with point estimate and confidence interval across studies (denoted by the diamond symbol, located in the last row for each Forest plot). Note that for Yuki and Okanoya (2017), we found the mean opt-out rate for the 4 rats in the experiment and we were not able to acquire the standard error of the means among the individual animals, we thus excluded this study from the forest plot for the opt-out rate (middle panel).
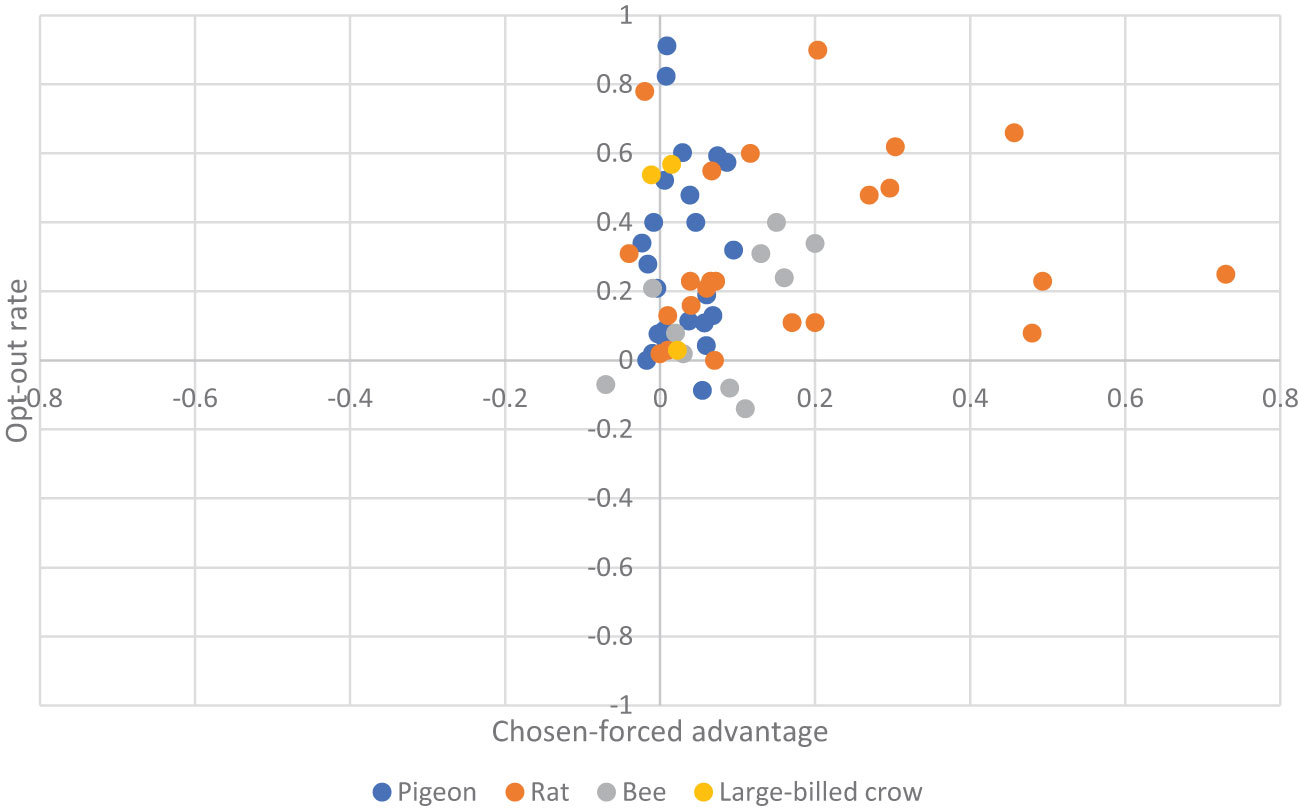
Figure 3 Scatter plot for chosen-forced advantage and opt-out rate. Each dot represents an individual animal. The colors correspond to the four NPA species.
Substantial heterogeneity was also identified, with Q(10) =122.291 (p < .001) for chosen-forced advantage; Q(9) =148.942 (p < .001) for opt-out rate, and Q(10) = 180.455 (p < .001) for composite score. A limitation of Cochran’s Q test is that it might be underpowered when few studies have been included or when event rates are low, thus we tested the I2, which provides an estimate of the percentage of variability in results across studies that is due to real differences and not due to chance. We found I2 =90.559% for chosen-forced advantage, I2 =96.934% for opt-out rate, and I2 =94.859 for composite score. According to Higgins et al. (2003), I2 values of 25%, 50%, and 75% indicate low, moderate and high heterogeneity, respectively. Our data contain very high heterogeneity.
3.1 Moderator analysis
To delve further into the factors that lead to the high heterogeneity, we conducted subgroup analysis for the three scores. We found that the three key factors (Order, Species, and Domain) did not systemically play a significant role in modulating the heterogeneity. The factor “Domain” (memory/perception tasks, Q=6.163, p=0.013, Table 2 top) and “Order” (prospective/retrospective in opting-out; Q=4.655, p=0.031, Table 2 middle) showed some effects on heterogeneity, but the factor “Species” did not impose any statistical significance (Table 2). Taking the composite score as an example (bottom Table), the p-values for “Heterogeneity” are 0.109, 0.944, and 0.411 for the three factors respectively; none of them was statistically significant. This implies that the three factors did not affect (modulate) the heterogeneity of our meta-analysis significantly. Note that the p-values for the factor “Species” for heterogeneity are also not significant for chosen-forced advantage (top table) and opt-out rate (middle table) either. This led to the conclusion that the effects of species differences on heterogeneity were not statistically significant.
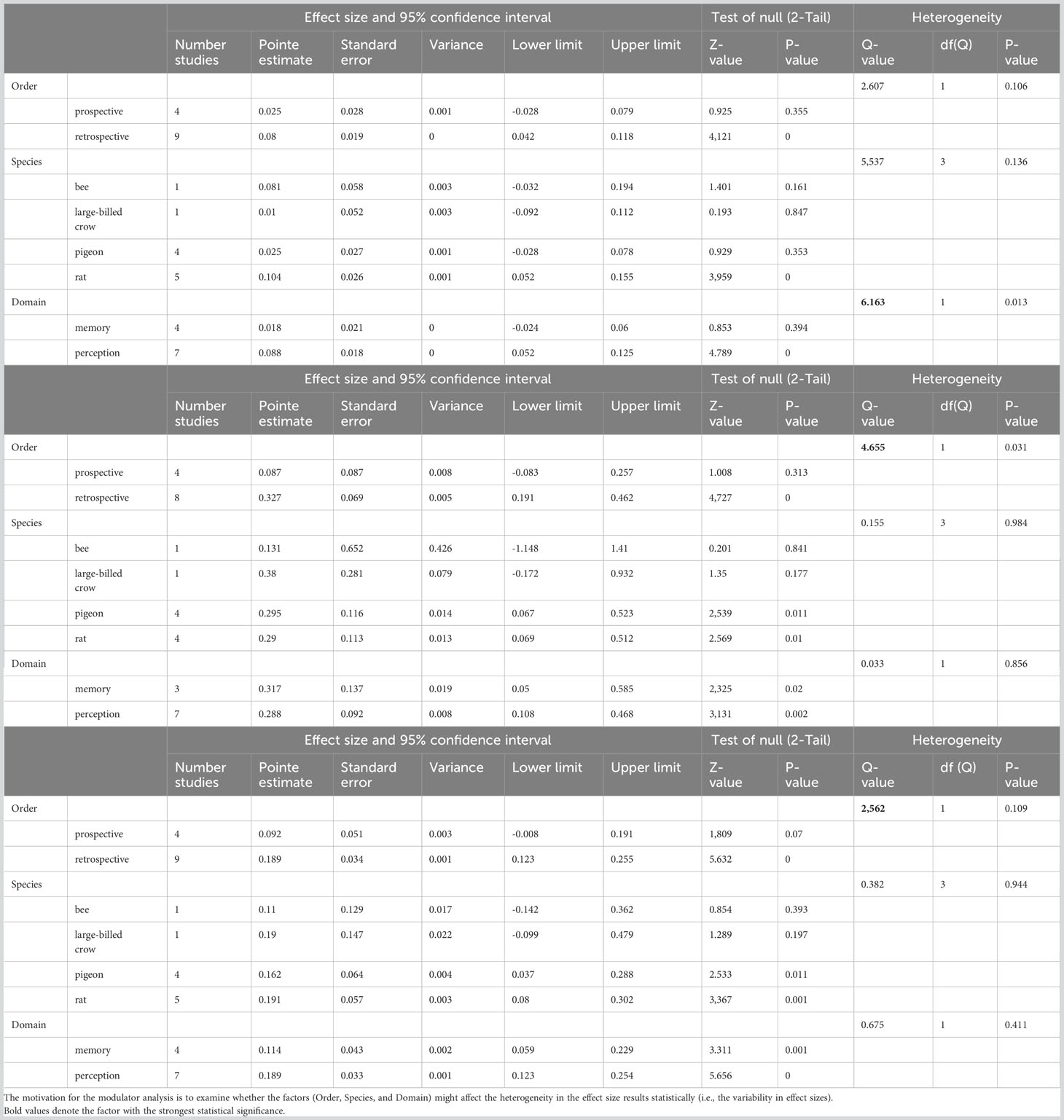
Table 2 Moderator analysis results for chosen-forced advantage (top), opt-out rate (middle), and composite score (bottom).
3.2 Publication bias
To examine if publication bias existed in the study, we used funnel plots for chosen-forced advantage, opt-out rate, and the composite score; a symmetric plot would indicate a lack of publication bias (Sterne et al., 2005). Egger’s tests were also performed to examine whether the assumption of a symmetrical distribution of effect sizes is viable; a p value greater than.05 would suggest a lack of sufficient evidence for publication bias (Egger et al., 1997). Egger’s tests revealed that there was no publication bias for chosen-forced advantage (t=1.246, p =0.244; Figure 4 top panel), opt-out rate (t=1.034, p =0.331; Figure 4 middle panel) and the composite score (t=1.859, p =0.096; Figure 4 bottom panel).
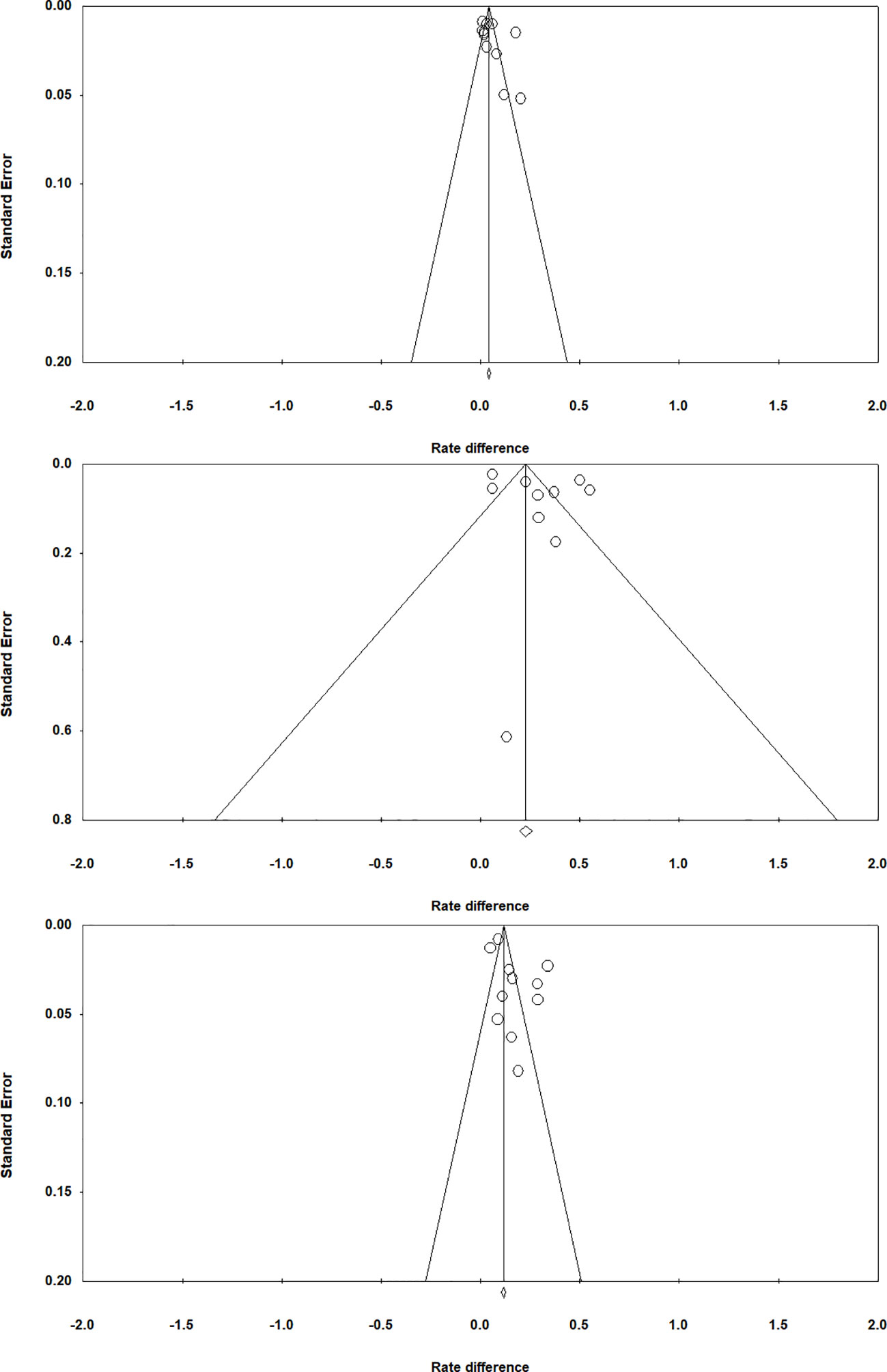
Figure 4 Funnel plots for chosen-forced advantage (top panel), opt-out rate (middle panel), and composite score (bottom panel).
3.3 Relationship with published brain data
These results may be at odds with other researchers who claim that the prefrontal cortex or a well-developed neocortex is a biological prerequisite to such high-level cognition. Some scholars also hold the belief that high-level metacognition involves consciousness (Dehaene et al., 2017), an ability closely linked to human and primates’ prefrontal cortex. Considering that these NPAs are not thought to possess a well-developed PFC, our results seem to be contrary to these claims. However, recent research shows that despite the lack of layered neocortex, large areas of the avian forebrain are homologous to mammalian cortex (Jarvis et al., 2005; Pfenning et al., 2014; Güntürkün and Bugnyar, 2016) and play similar roles in higher cognitive functions (Kirsch et al., 2008). Similarly, complex brain functions can emerge from simple neural circuits such as honeybees’ mushroom body (Caron and Abbott, 2017) and miniature brains can complete a range of cognitive operations (Chittka and Niven, 2009; Chittka, 2017).
In light of these possibilities, we tested if meta-ability is correlated with the animal’s absolute brain weight and number of neurons in the brain (Herculano-Houzel, 2011), as these factors are known to be a predictor for some high-level cognitive abilities (Deaner et al., 2007; MacLean et al., 2014). We ran a series of correlation analyses correlating the species’ chosen-forced advantage, opt-out rate, and composite score with their brain weight and number of neurons in the cortex, respectively. We found that there is no significant correlation between species’ meta-scores, absolute brain weight, and neurons in the brain (all Cor < 0.103, all ps > 0.051). We then ran correlation tests between species’ meta-scores and their brain-to-body ratio, which also yielded nonsignificant results (all Cor < -0.183, all ps > 0.114). This suggests that brain volume per se is not sufficient in accounting for the species’ meta-ability. Rather, the existence of meta-ability could be due to other factors, such as interneuronal distance and axonal conduction velocity (Dicke and Roth, 2016), fundamental neural circuits that are similar across species (Chittka and Niven, 2009) or higher neuron packing densities in the forebrain (Olkowicz et al., 2016).
4 Paradigm 2: information seeking
Another widely used paradigm in NPA metacognition research is the information-seeking paradigm. A typical information-seeking paradigm presents animals with a situation in which they are not given enough information to complete a trial. Their behavior is nonetheless monitored to determine whether they subsequently seek information from the environment to help them complete the task. The first reports of such a paradigm were on great apes and children, where apes and children saw the experimenter hiding food in one of several tubes, either in plain sight or not. They were then asked to choose one of the tubes but were allowed to look into the tubes before choosing. Under a metacognitive assumption, animals should collect more information when they feel uncertain, and therefore should tend to look into the tubes when they do not know where the food was placed (sight was occluded during baiting) (Call and Carpenter, 2001). The study demonstrates such ability in great apes. Tests on other primates, such as rhesus monkeys (Macaca mulatta), also showed that they will look into opaque tubes or under opaque containers to check the location of a hidden reward; and they look less often when other cues are available indicating the location of the reward, such as visible baiting, transparent tubes or containers, or logical inference (Beran and Smith, 2011; Rosati and Santos, 2016). The paradigm was also applied in rats (Rattus norvegicus), scrub jays (Aphelocoma californica), pigeons (Columba livia), dogs (Canis familiaris), dingoes (Canis dingo), ravens (Corvus corax), an arctic fox (Vulpes lagopus), and horses (Equus caballus) to test if they demonstrate such spontaneous information-seeking tendencies (Royka et al., 2020).
In order to formally evaluate the published data in this task, we compiled raw data from 8 NPA species including 123 individuals with a total of 302 experimental trials (see Table 3). Each subject will give out a binary outcome (pass or fail). As a group, the proportion of individuals in each species that succeeded at least once at the task varied: a fox (0% passing rate), horses (0% passing rate), dogs (37.5% passing rate), dingoes (33.3% passing rate), pigeons (63.6% passing rate), western scrub-jays (84.6% passing rate), ravens (100% passing rate), and rats (81.5% passing rate). We hypothesized that within-group 0% or more of the animals passing the test is considered a significant group effect. Having this as the expected distribution, a Pearson’s chi-square test with Yates’ continuity correction was performed to determine whether these animal groups as a group passed the test at an above chance level. This difference was statistically significant, χ2 = 531.56, df = 1, p < 0.001 [alternatively, one-sample t-test: t (7) = 3.690, p = 0.008], thus rejecting the null hypotheses that these animals merely had a chance level passing rate.
Among these animals, scrub jays are the only species which demonstrate a similar level of mastery as primates. In a classic foraging task, some experimental trials required scrub jays to observe the hiding location to know the location of the food, whilst in other trials the location of the food could be easily found without watching (Watanabe and Clayton, 2016). The jays allocated more time to the peepholes when the reward location was obscure than when it was apparent. Uncertainty about the food location was induced in three ways: by occluding sight during the baiting process, by adding a delay between the baiting and food retrieval, or by moving the location of the bait. The jays looked into the tubes more often during the conditions that were consistent with high uncertainty (Watanabe and Clayton, 2016). Moreover, bees were found to allocate different amounts of time looking at a reward location depending on how much information they had, suggesting that the degree of investment in time correlates with the value of the information the animals obtained (Lehrer, 1993; Wei et al., 2002).
By contrast, dogs and horses failed to demonstrate information-seeking behavior in such foraging tests, even after having received large/sufficient amounts of training. In a foraging task conducted on dogs (McMahon et al., 2010), dogs were trained to make a visual discrimination and then researchers gradually altered the cues to test if the dogs would seek information by moving into a new spatial position. None of the dogs succeeded in doing so. In another study, horses learned to use a cue to identify where a food reward was located but failed to learn to move or look behind a sheet that would have revealed a previously learned discriminative cue (Zupet, 2010). As these NPA species do not show the spontaneous information-seeking behavior that primates do, a simpler version of the paradigm was developed and applied to dogs (Bräuer et al., 2004), pigeons (Roberts et al., 2009; Zentall and Stagner, 2010; Castro and Wasserman, 2013; Iwasaki et al., 2013; 2018), and rats (Kirk et al., 2014). These studies revealed that if NPAs are given a forced choice between stimuli that either do or do not yield information about the location of a reward, they can make an informative choice.
Interestingly, we found that pigeons and rats are generally more successful in uncertainty monitoring but less so in the information seeking paradigms. These two paradigms offer different contexts for the application of metacognitive skills, with the opt-out paradigm centered on decision-making whereas the information-seeking paradigm on the active process of seeking and evaluating information. In this case, pigeons and rats passed the opt-out paradigm but not the information-seeking paradigm; it suggests that they demonstrated metacognitive abilities related to decision-making and the recognition of uncertainty but struggled with actively searching for and evaluating information to address specific questions or problems.
5 Discussion
5.1 Interpretations for the meta-analysis results
Our findings from the meta-analysis that four NPA species passed the uncertainty monitoring test at the group level carry theoretical implications for the field. In the modulator analysis, we found that the factor “Species” for heterogeneity is not significant for the chosen-forced advantage, the opt-out rate, nor for the composite score. This leads to the conclusion that the effects of species differences on heterogeneity are not statistically significant in this study. However, to avoid overgeneralization, we maintain that our conclusion should only be applicable to the few species included here.
In the meta-analysis, we found that test order played an important role in leading to heterogeneity across studies. This result is in line with previous findings that animals are better in performance for retrospective tests than for prospective tests (Morgan et al., 2014; Siedlecka et al., 2016). Interestingly, we did not find the factor species to be a determinant factor leading to the heterogeneity of animals’ performance, and this is in alignment with the emerging evidence that metacognitive-like abilities detected from animals from various distant families. One study on pharaoh’s ants found that individual ants spontaneously upregulate or downregulate pheromones depending on the reliability of their own memories (Czaczkes and Heinze, 2015). This demonstrates certain metamemory-like ability in ants, echoing other insect studies wherein honeybees were found to be able to monitor their uncertainty (Perry and Barron, 2013). It is possible that the evolution of metacognition is not a representation of a linear sequence of cortex-dependent evolution, but rather as representatives of different clades that diverged at different points during evolution.
We acknowledge the potential influence of low-level mechanisms on uncertainty response. While our study suggests that the four NPAs exhibited metacognitive-like abilities, we cannot entirely rule out alternative explanations involving lower-level mechanisms and/or associative learning or other mechanisms that do not require invoking introspection or access to private internal states (Hampton, 2009). Therefore we should be cautious in the interpretation of the significance of our findings in advancing our understanding of cognitive processes in NPAs.
Moreover, we note that the selection of these four species in our uncertainty monitoring meta-analysis was primarily driven by data availability. While we acknowledge this limitation, our choice aimed to represent diverse taxonomic groups (mammals, birds, insects) and comparable experimental paradigms. This allowed for preliminary insights into metacognitive-like abilities across different species. We recognize the need for further research beyond these four species to expand our understanding of metacognition in non-primate animals.
5.2 Critiques for the paradigms and transfer tasks as a solution
There are several caveats with the analyses and our interpretation. Firstly, this all-in-one meta-analysis could not take into account the variations in individual studies, such as factors related to rewards, training intensity, and each task’s temporal variations. Secondly, the mechanisms of how the two scores operationally complement each other remains unclear and therefore we remain tentative in how much information might be missed out by considering these two scores separately. The meta-like performance on these two paradigms remains debatable as the measures do not unambiguously reflect second-order (i.e., metacognitive) computations. To address this issue, a number of transfer tasks related to both paradigms have been developed in the field. In these transfer tasks, animals were tested on whether they could transfer the concept of opting out or information seeking to a novel task. The impetus for developing such tasks is that if animals are able to pass a transfer task following a primary task, this would indicate that their uncertainty monitoring ability and information-seeking behavior represent a task-independent cognitive state (Washburn et al., 2006). For example, researchers have tested if uncertainty monitoring ability is transferable to novel stimuli in pigeons (Sole et al., 2003; Nakamura et al., 2011), bantams (Sole et al., 2003; Nakamura et al., 2011), and bees (Perry and Barron, 2013). Five out of six pigeons and two out of three bantams in Nakamura’s study (Nakamura et al., 2011) generalized their uncertainty responses to novel stimuli at least once. Four of the ten bees could transfer the concept of opting out to a novel task (Perry and Barron, 2013). Other researchers have also tested if pigeons’ information-seeking behavior (Castro and Wasserman, 2013; Iwasaki et al., 2013) is transferable to new tasks. One out of four pigeons in Castro and Wasserman’s (2013) study successfully transferred the information-seeking behavior into two transfer tasks. One out of 2 pigeons in Iwasaki’s study (2013) transferred the information-seeking behavior from a hint-seeking serial learning task to a hint-seeking visual search task.
In these tasks, subjects demonstrated a generalized mechanism whereby a first-order (cognitive) representation is internally assessed through a second-order (metacognitive) process that directly evaluates its quality, although it remains unknown if such a mechanism contains introspection. Such performance has prompted theorists to land a “middle ground” between the low level and the high level to discuss animals’ meta-behavior. Metcalfe and Son (2012) layer three levels of metacognition. The middle level is referred to as “noetic metacognition”, which allows an organism to be aware of, and to cognitively operate on, objects and events, as well as relations among objects and events, in the absence of those objects and events. Smith et al. (2012) advocates that animals’ uncertainty responses are not appropriately considered associative responses in the traditional sense. They can be independent from stimuli, reinforcement, and so forth. Similarly, Beran (2012) proposes that a metacognitive process can represent a first-order state, without acknowledging that this state has a certain representational function. It is possible that animals, like humans, are capable of making uncertainty judgments based on internal cues without awareness of meaningful self-reflection. Uncertainty monitoring capacity could emerge phylogenetically as a gradual feeling of the indeterminacy response from its primary responses (Beran et al., 2009). The middle ground emphasizes the functional feature of metacognition rather than true self-reflection and has allowed us to appreciate NPA’s metacognition performance in a more realistic way. It opens up possibilities to interpret the existing NPA metacognition literature in relation to their evolutionary significance.
5.3 Insights from human and non-human primates’ metacognition studies
There has been a great amount of discussion regarding the theoretical implications of these approaches. Different levels of metacognition have been proposed by scholars to describe animals’ meta behaviors, such as object versus meta level (Shields et al., 2005), first-order versus second-order (Crystal and Foote, 2009), public mechanism versus private mechanism (Hampton, 2009), anoetic metacognition, noetic metacognition, and autonoetic metacognition (Metcalfe and Son, 2012), and low-level versus high level (Smith et al., 2012). Although NPAs can pass these two paradigms and certain transfer tasks, there are alternative explanations that can explain the results without implicating metacognition per se (Carruthers, 2008; Jozefowiez et al., 2009; Le Pelley, 2012). One way to help support the metacognitive argument |is to follow the emerging trends in human and non-human primate metacognition research to measure an individual’s metacognitive sensitivity in a more quantitative way. A widely used approach to measure human and non-human primates’ metacognition is to calculate the comparison between the type 1 sensitivity (d’) and the type 2 sensitivity (meta-d’). This approach can quantify meta-ability under the signal detection theory framework (Maniscalco and Lau, 2012) or by a recently developed hierarchical Bayesian estimation method (Fleming, 2017). To attain these analyses, confidence ratings are needed. This subjective confidence measurement is deemed practically very difficult in animals but there are now viable ways to attain these, such as temporal wagering tasks (Kepecs et al., 2008; Lak et al., 2014; Cai et al., 2022), in which animals express choice confidence by the amount of time they are willing to wait for reward. This has been demonstrated to be a successful paradigm in mice (Lak et al., 2014), rats (Stolyarova et al., 2019), and monkeys (Cai et al., 2022). It has become a hot topic to examine whether metacognition in the primate shows domain specificity, with supporting evidence for both domain-specific and domain-general metacognitive representations (Morales et al., 2018; Ye et al., 2018; Kwok et al., 2019; Zheng et al., 2021; Cai et al., 2022). This aspect will provide an important line for further investigation in the NPA species. Finally, in order to reduce publication bias, standardize research protocols, and ultimately expand the data volume in this niche field, researchers in the field could benefit from taking the ManyPrimates project model as well as other similar initiatives as an approach to establish an infrastructure for large-scale collaboration in non-primate animals cognition research (Altschul et al. 2019; ManyPrimates et al., 2019; Milham et al., 2022).
6 Conclusion
We present a cross-species comparative meta-analysis on NPA’s meta-ability measured by the opt-out paradigm as well as a performance analysis measured by the information-seeking paradigm. By aggregating the existing evidence in support of some degree of meta-ability in these animals, we show that NPA species pass the uncertainty monitoring test and the information seeking test. The literature on animal metacognition has grown tremendously over the last two decades or so, and we hope this study will help consolidate the research in the field and stimulate research towards a less anthropocentric direction in the study of metacognition.
Data availability statement
The original contributions presented in the study are included in the article/supplementary material. Further inquiries can be directed to the corresponding author.
Author contributions
ZQ and SCK conceived the study. ZQ performed the literature review, data collection and data analysis. ZQ and SCK wrote the manuscript. LS, CS, and JY helped with data analyses and interpretation. All authors contributed to the article and approved the submitted version.
Funding
The author(s) declare financial support was received for the research, authorship, and/or publication of this article. The research results of this publication are sponsored by the Kunshan Municipal Government research funding Project Number: 23KKSGR017.
Acknowledgments
We thank Lei Wang for his advice on the chi-square test.
Conflict of interest
The authors declare that the research was conducted in the absence of any commercial or financial relationships that could be construed as a potential conflict of interest.
Publisher’s note
All claims expressed in this article are solely those of the authors and do not necessarily represent those of their affiliated organizations, or those of the publisher, the editors and the reviewers. Any product that may be evaluated in this article, or claim that may be made by its manufacturer, is not guaranteed or endorsed by the publisher.
References
Altschul D. M., Beran M. J., Bohn M., Caspar K. R., Fichtel C., Försterling M., et al. (2019). Collaborative open science as a way to reproducibility and new insights in primate cognition research. Japan. psychol. Rev. 62 (3), 205–220. doi: 10.24602/sjpr.62.3_205
Beran M. J., Smith J. D. (2011). Information seeking by rhesus monkeys (Macaca mulatta) and capuchin monkeys (Cebus apella). Cognition 120 (1), 90–105. doi: 10.1016/j.cognition.2011.02.016
Beran M. J., Smith J. D., Coutinho M. V., Couchman J. J., Boomer J. (2009). The psychological organization of “uncertainty“ responses and “middle” responses: A dissociation in capuchin monkeys (Cebus apella). J. Exp. Psychology: Anim. Behav. Processes 35 (3), 371. doi: 10.1037/a0014626
Borenstein M. (2005). Software for publication bias. Publication bias in meta-analysis: Prevention, assessment and adjustments Eds. Rothstein H. R., Sutton A. J., Borenstein M., 193–220. doi: 10.1002/0470870168
Bräuer J., Call J., Tomasello M. (2004). Visual perspective taking in dogs (Canis familiaris) in the presence of barriers. Appl. Anim. Behav. Sci. 88 (3-4), 299–317. doi: 10.1016/j.applanim.2004.03.004
Cai Y., Jin Z., Zhai C., Wang H., Wang J., Tang Y., et al. (2022). Time-sensitive prefrontal involvement in associating confidence with task performance illustrates metacognitive introspection in monkeys. Commun. Biol. 5, 799. doi: 10.1038/s42003-022-03762-6
Call J., Carpenter M. (2001). Do apes and children know what they have seen? Anim. Cogn. 3 (4), 207–220. doi: 10.1007/s100710100078
Caron S., Abbott L. F. (2017). Neuroscience: intelligence in the honeybee mushroom body. Curr. Biol. 27 (6), R220–R223. doi: 10.1016/j.cub.2017.02.011
Carruthers P. (2008). Meta-cognition in animals: a skeptical look. Mind Lang. 23 (1), 58–89. doi: 10.1111/j.1468-0017.2007.00329.x
Castro L., Wasserman E. A. (2013). Information-seeking behavior: Exploring metacognitive control in pigeons. Anim. Cogn. 16 (2), 241–254. doi: 10.1007/s10071-012-0569-8
Chittka L., Niven J. (2009). Are bigger brains better? Curr. Biol. 19 (21), R995–R1008. doi: 10.1016/j.cub.2009.08.023
Crystal J. D., Foote A. L. (2009). Metacognition in animals. Comp. Cogn. Behav. Rev. 4, 1. doi: 10.3819/ccbr.2009.40001
Czaczkes T. J., Heinze J. (2015). Ants adjust their pheromone deposition to a changing environment and their probability of making errors. Proc. R. Soc. B: Biol. Sci. 282 (1810), 20150679. doi: 10.1098/rspb.2015.0679
Deaner R. O., Isler K., Burkart J., Van Schaik C. (2007). Overall brain size, and not encephalization quotient, best predicts cognitive ability across non-human primates. Brain Behav. Evol. 70 (2), 115–124. doi: 10.1159/000102973
Dehaene S., Lau H., Kouider S. (2017). What is consciousness, and could machines have it? Science 358 (6362), 486–492. doi: 10.1126/science.aan8871
Dicke U., Roth G. (2016). Neuronal factors determining high intelligence. Philos. Trans. R. Soc. B: Biol. Sci. 371 (1685), 20150180. doi: 10.1098/rstb.2015.0180
Eaton T., Billette P., Vonk J. (2020). Are there metacognitivists in fox holes? A preliminary test of information seeking in an arctic fox (Vulpes lagopus). Behav. Sci. 10, 81. doi: 10.3390/bs10050081
Egger M., Smith G. D., Schneider M., Minder C. (1997). Bias in meta-analysis detected by a simple, graphical test. Bmj 315 (7109), 629–634. doi: 10.1136/bmj.315.7109.629
Fleming S. M. (2017). HMeta-d: hierarchical bayesian estimation of metacognitive efficiency from confidence ratings. Neurosci. Consciousness 2017 (1), nix007. doi: 10.1093/nc/nix007
Güntürkün O., Bugnyar T. (2016). Cognition without cortex. Trends Cogn. Sci. 20 (4), 291–303. doi: 10.1016/j.tics.2016.02.001
Hampton R. R. (2001). Rhesus monkeys know when they remember. Proc. Natl. Acad. Sci. 98 (9), 5359–5362. doi: 10.1073/pnas.071600998
Hampton R. R. (2009). Multiple demonstrations of metacognition in nonhumans: Converging evidence or multiple mechanisms? Comp. Cogn. Behav. Rev. 4, 17. doi: 10.3819/ccbr.2009.40002
Herculano-Houzel S. (2011). Brains matter, bodies maybe not: the case for examining neuron numbers irrespective of body size. Ann. New York Acad. Sci. 1225 (1), 191–199. doi: 10.1111/j.1749-6632.2011.05976.x
Higgins J. P., Thompson S. G., Deeks J. J., Altman D. G. (2003). Measuring inconsistency in meta-analyses. Bmj 327 (7414), 557–560. doi: 10.1136/bmj.327.7414.557
Inman A., Shettleworth S. J. (1999). Detecting metamemory in nonverbal subjects: A test with pigeons. J. Exp. Psychol.: Anim. Behav. Process. 25 (3), 389.
Iwasaki S., Watanabe S., Fujita K. (2013). Do pigeons (Columba livia) seek information when they have insufficient knowledge? Anim. Cogn. 16 (2), 211–221. doi: 10.1007/s10071-012-0566-y
Iwasaki S., Watanabe S., Fujita K. (2018). Pigeons (Columba livia) know when they will need hints: prospective metacognition for reference memory? Anim. Cogn. 21 (2), 207–217. doi: 10.1007/s10071-017-1153-z
Jarvis E. D., Güntürkün O., Bruce L., Csillag A., Karten H., Kuenzel W., et al. (2005). Avian brains and a new understanding of vertebrate brain evolution. Nat. Rev. Neurosci. 6 (2), 151. doi: 10.1038/nrn1606
Jozefowiez J., Staddon J., Cerutti D. (2009). Metacognition in animals: how do we know that they know? Comp. Cogn. Behav. Rev., 4. doi: 10.3819/ccbr.2009.40003
Kepecs A., Uchida N., Zariwala H. A., Mainen Z. F. (2008). Neural correlates, computation and behavioural impact of decision confidence. Nature 455 (7210), 227. doi: 10.1038/nature07200
Kirk C. R., McMillan N., Roberts W. A. (2014). Rats respond for information: Metacognition in a rodent? J. Exp. Psychology: Anim. Learn. Cogn. 40 (2), 249. doi: 10.1037/xan0000018
Kirsch J. A., Güntürkün O., Rose J. (2008). Insight without cortex: lessons from the avian brain. Conscious. Cogn. 17 (2), 475–483. doi: 10.1016/j.concog.2008.03.018
Kornell N., Son L. K., Terrace H. S. (2007). Transfer of metacognitive skills and hint seeking in monkeys. Psychol. Sci. 18 (1), 64–71. doi: 10.1111/j.1467-9280.2007.01850.x
Kwok S. C., Cai Y., Buckley M. J. (2019). Mnemonic introspection in macaques is dependent on superior dorsolateral prefrontal cortex but not orbitofrontal cortex. J. Neurosci. 39 (30), 5922–5934. doi: 10.1523/JNEUROSCI.0330-19.2019
Lak A., Costa G. M., Romberg E., Koulakov A. A., Mainen Z. F., Kepecs A. (2014). Orbitofrontal cortex is required for optimal waiting based on decision confidence. Neuron 84 (1), 190–201. doi: 10.1016/j.neuron.2014.08.039
Lambert M. L., Osvath M. (2020). Investigating information seeking in ravens (Corvus corax). Anim. Cogn. 23 (4), 671–680. doi: 10.1007/s10071-020-01372-5
Lehrer M. (1993). Why do bees turn back and look? J. Comp. Physiol. A 172 (5), 549–563. doi: 10.1007/BF00213678
Le Pelley M. (2012). Metacognitive monkeys or associative animals? simple reinforcement learning explains uncertainty in nonhuman animals. J. Exp. Psychology: Learning Memory Cogn. 38 (3), 686. doi: 10.1037/a0026478
MacLean E. L., Hare B., Nunn C. L., Addessi E., Amici F., Anderson R. C., et al. (2014). The evolution of self-control. Proc. Natl. Acad. Sci. 111 (20), E2140–E2148. doi: 10.1073/pnas.1323533111
Maniscalco B., Lau H. (2012). A signal detection theoretic approach for estimating metacognitive sensitivity from confidence ratings. Consciousness Cogn. 21 (1), 422–430. doi: 10.1016/j.concog.2011.09.021
McMahon S., Macpherson K., Roberts W. A. (2010). Dogs choose a human informant: Metacognition in canines. Behav. Processes 85 (3), 293–298. doi: 10.1016/j.beproc.2010.07.014
Metcalfe J., Son L. K. (2012). Anoetic, noetic, and autonoetic metacognition (Oxford, UK: The foundations of metacognition. Oxford University Press), 289–301.
Middlebrooks P. G., Sommer M. A. (2011). Metacognition in monkeys during an oculomotor task. J. Exp. Psychol.: Learn. Memory Cogn. 37 (2), 325. doi: 10.1037/a0021611
Milham M., Petkov C., Belin P., Hamed S. B., Evrard H., Fair D., et al. (2022). Toward next-generation primate neuroscience: a collaboration-based strategic plan for integrative neuroimaging. Neuron 110 (1), pp.16–pp.20. doi: 10.1016/j.neuron.2021.10.015
Miyamoto K., Osada T., Setsuie R., Takeda M., Tamura K., Adachi Y., et al. (2017). Causal neural network of metamemory for retrospection in primates. Science 355 (6321), 188–193. doi: 10.1126/science.aal0162
Morales J., Lau H., Fleming S. M. (2018). Domain-general and domain-specific patterns of activity supporting metacognition in human prefrontal cortex. J. Neurosci. 38 (14), 3534–3546. doi: 10.1523/JNEUROSCI.2360-17.2018
Morgan G., Kornell N., Kornblum T., Terrace H. S. (2014). Retrospective and prospective metacognitive judgments in rhesus macaques (Macaca mulatta). Anim. Cogn. 17 (2), 249–257. doi: 10.1007/s10071-013-0657-4
Nakamura N., Watanabe S., Betsuyaku T., Fujita K. (2011). Do birds (pigeons and bantams) know how confident they are of their perceptual decisions? Anim. Cogn. 14 (1), 83–93. doi: 10.1007/s10071-010-0345-6
Olkowicz S., Kocourek M., Lučan R. K., Porteš M., Fitch W. T., Herculano-Houzel S., et al. (2016). Birds have primate-like numbers of neurons in the forebrain. Proc. Natl. Acad. Sci. 113 (26), 7255–7260. doi: 10.1073/pnas.1517131113
Perry C. J., Barron A. B. (2013). Honey bees selectively avoid difficult choices. Proc. Natl. Acad. Sci. 110 (47), 19155–19159. doi: 10.1073/pnas.1314571110
Pfenning A. R., Hara E., Whitney O., Rivas M. V., Wang R., Roulhac P. L., et al. (2014). Convergent transcriptional specializations in the brains of humans and song-learning birds. Science 346 (6215), 1256846. doi: 10.1126/science.1256846
Roberts W. A., Feeney M. C., McMillan N., MacPherson K., Musolino E., Petter M. (2009). Do pigeons (Columba livia) study for a test? J. Exp. Psychology: Anim. Behav. Processes 35 (2), 129. doi: 10.1037/a0013722
Rosati A. G., Santos L. R. (2016). Spontaneous metacognition in rhesus monkeys. psychol. Sci. 27 (9), 1181–1191. doi: 10.1177/09567976166537
Royka A. L., Johnston A. M., Santos L. R. (2020). Metacognition in canids: A comparison of dogs (Canis familiaris) and dingoes (Canis dingo). J. Comp. Psychol. 134 (3), 303. doi: 10.1037/com0000246
Shields W. E., Smith J. D., Guttmannova K., Washburn D. A. (2005). Confidence judgments by humans and rhesus monkeys. J. Gen. Psychol. 132 (2), 165.
Siedlecka M., Paulewicz B., Wierzchoń M. (2016). But I was so sure! Metacognitive judgments are less accurate given prospectively than retrospectively. Front. Psychol. 7, 218. doi: 10.3389/fpsyg.2016.00218
Smith J. D. (2009). The study of animal metacognition. Trends Cogn. Sci. 13 (9), 389–396. doi: 10.1016/j.tics.2009.06.009
Smith J. D., Couchman J. J., Beran M. J. (2012). The highs and lows of theoretical interpretation in animal-metacognition research. Philos. Trans. R. Soc. London B: Biol. Sci. 367 (1594), 1297–1309. doi: 10.1098/rstb.2011.0366
Smith J. D., Schull J., Strote J., McGee K., Egnor R., Erb L. (1995). The uncertain response in the bottlenosed dolphin (Tursiops truncatus). J. Exp. Psychol.: Gen. 124 (4), 391. doi: 10.1037/0096-3445.124.4.391
Smith J. D., Shields W. E., Washburn D. A. (2003). The comparative psychology of uncertainty monitoring and metacognition. Behav. Brain Sci. 26 (3), 317. doi: 10.1017/S0140525X03000086
Sole L. M., Shettleworth S. J., Bennett P. J. (2003). Uncertainty in pigeons. Psycho. Bull. Rev. 10 (3), 738–745. doi: 10.3758/BF03196540
Sterne J. A., Becker B. J., Egger M. (2005). The funnel plot. In Publication Bias in Meta-Analysis: Prevention, Assessment and Adjustments. Eds. Rothstein H. R., Sutton A. J., Borenstein M. (UK: Wiley), 75–98.
Stolyarova A., Rakhshan M., Hart E. E., O’Dell T. J., Peters M. A., Lau H., et al. (2019). Contributions of anterior cingulate cortex and basolateral amygdala to decision confidence and learning under uncertainty. Nat Commun. 10, 4704. doi: 10.1038/s41467-019-12725-1
Teller S. A. (1989). Metamemory in the pigeon: prediction of performance on a delayed matching to sample task (Reed College).
Washburn D. A., Smith J. D., Shields W. E. (2006). Rhesus monkeys (macaca mulatta) immediately generalize the uncertain response. J. Exp. Psychol.: Anim. Behav. Process. 32 (2), 185. doi: 10.1037/0097-7403.32.2.185
Watanabe A., Clayton N. S. (2016). Hint-seeking behaviour of western scrub-jays in a metacognition task. Anim. Cogn. 19 (1), 53–64. doi: 10.1007/s10071-015-0912-y
Wei C., Rafalko S., Dyer F. (2002). Deciding to learn: modulation of learning flights in honeybees, apis mellifera. J. Comp. Physiol. A 188 (9), 725–737. doi: 10.1007/s00359-002-0346-2
Ye Q., Zou F., Lau H., Hu Y., Kwok S. C. (2018). Causal evidence for mnemonic metacognition in human precuneus. J. Neurosci. 38 (28), 6379–6387. doi: 10.1523/JNEUROSCI.0660-18.2018
Yuki S., Okanoya K. (2017). Rats show adaptive choice in a metacognitive task with high uncertainty. J. Exp. Psychol.: Anim. Learn. Cogn. 43 (1), 109. doi: 10.1037/xan0000130
Zentall T. R., Stagner J. P. (2010). Pigeons prefer conditional stimuli over their absence: A comment on roberts et al.(2009). J. Exp. Psychology: Anim. Behav. Processes 36 (4), 506. doi: 10.1037/a0020202
Zheng Y., Wang D., Ye Q., Zou F., Li Y., Kwok S. C. (2021). Diffusion property and functional connectivity of superior longitudinal fasciculus underpin human metacognition. Neuropsychologia 156, 107847. doi: 10.1016/j.neuropsychologia.2021.107847
Keywords: metacognition, non-primate animals, meta-analysis, opt-out, information seeking, evolution
Citation: Qu Z, Shi L, So BCL, Yin J and Kwok SC (2023) Uncertainty monitoring and information seeking in non-primate animals: Meta-analysis and systematic review. Front. Ethol. 2:1246370. doi: 10.3389/fetho.2023.1246370
Received: 24 June 2023; Accepted: 29 September 2023;
Published: 24 October 2023.
Edited by:
Enrique Font, University of Valencia, SpainReviewed by:
Janusz Strychalski, University of Warmia and Mazury in Olsztyn, PolandMariana V. C. Coutinho, United Arab Emirates University, United Arab Emirates
Jennifer Vonk, Oakland University, United States
Copyright © 2023 Qu, Shi, So, Yin and Kwok. This is an open-access article distributed under the terms of the Creative Commons Attribution License (CC BY). The use, distribution or reproduction in other forums is permitted, provided the original author(s) and the copyright owner(s) are credited and that the original publication in this journal is cited, in accordance with accepted academic practice. No use, distribution or reproduction is permitted which does not comply with these terms.
*Correspondence: Sze Chai Kwok, c3plLWNoYWkua3dva0BzdC1odWdocy5veG9uLm9yZw==