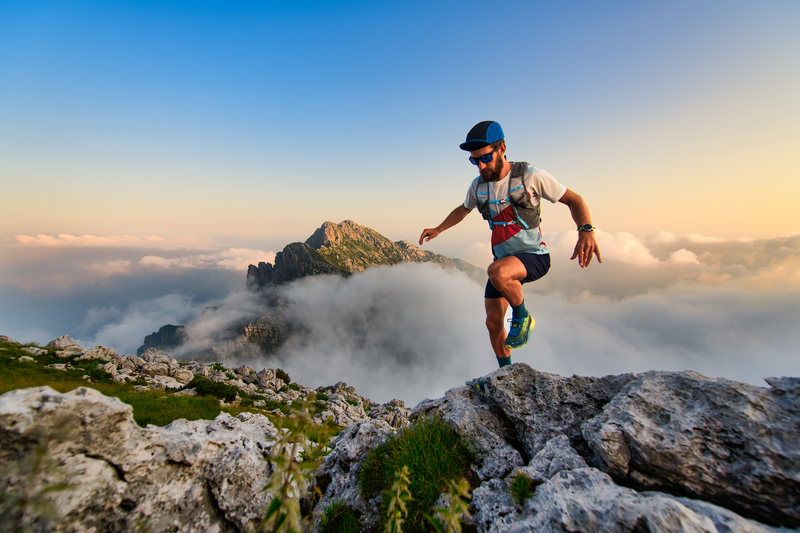
94% of researchers rate our articles as excellent or good
Learn more about the work of our research integrity team to safeguard the quality of each article we publish.
Find out more
CONCEPTUAL ANALYSIS article
Front. Epidemiol.
Sec. Occupational and Environmental Epidemiology
Volume 5 - 2025 | doi: 10.3389/fepid.2025.1557023
This article is part of the Research Topic Mineral Particles and Fibers and Human Health Risks: State-of-the-Art in Characterization, Analysis, Tissue Analytics, Exposure Thresholds for Risk, Epidemiology, and Risk Assessment for Science-Based Regulation and Disease Prevention and Implications for Occupational Health and Safety View all 3 articles
The final, formatted version of the article will be published soon.
You have multiple emails registered with Frontiers:
Please enter your email address:
If you already have an account, please login
You don't have a Frontiers account ? You can register here
This paper summarizes several presentations in the Thresholds in Epidemiology and Risk Assessment session at the Monticello III conference. These presentations described evidence regarding thresholds for particles, including asbestos and silica, and cancer (e.g., mesothelioma) and non-cancer (e.g., silicosis) endpoints. In the case of exposure to various types of particles and malignancy, it is clear that even though a linear non-threshold model has often been assumed, experimental and theoretical support for thresholds exist (e.g., through particle clearance, repair mechanisms, and various other aspects of the carcinogenic process). For mesothelioma and exposure to elongate mineral particles (EMPs), there remains controversy concerning the epidemiological demonstration of thresholds. However, using data from the Québec mining cohort studies, it was shown that a "practical" threshold exists for chrysotile exposure and mesothelioma. It was also noted that, in such evaluations, measurement error in diagnosis and exposure assessment needs to be incorporated into risk analyses. Researchers were also encouraged to use biobanks that collect specimens and data on mesothelioma to more precisely define cases of mesothelioma and possible variants for cases of all ages, and trends that may help define background rates and distinguish those mesotheliomas related to EMP exposures from those that are not, as well as other factors that support or define thresholds. New statistical approaches have been developed for identifying and quantifying exposure thresholds, an example of which is described for respirable crystalline silica (RCS) exposure and silicosis risk. Finally, the application of Artificial Intelligence (AI) to considering the multiple factors influencing risk and thresholds may prove useful.
Keywords: Thresholds, Asbestos, Elongate Mineral Particles, silica, Mesothelioma, Silicosis
Received: 08 Jan 2025; Accepted: 28 Feb 2025.
Copyright: © 2025 Goodman, Rhomberg, Cohen, Mundt, Case, Burstyn, Becich and Gibbs. This is an open-access article distributed under the terms of the Creative Commons Attribution License (CC BY). The use, distribution or reproduction in other forums is permitted, provided the original author(s) or licensor are credited and that the original publication in this journal is cited, in accordance with accepted academic practice. No use, distribution or reproduction is permitted which does not comply with these terms.
* Correspondence:
Julie Goodman, Gradient, Boston, United States
Disclaimer: All claims expressed in this article are solely those of the authors and do not necessarily represent those of their affiliated organizations, or those of the publisher, the editors and the reviewers. Any product that may be evaluated in this article or claim that may be made by its manufacturer is not guaranteed or endorsed by the publisher.
Research integrity at Frontiers
Learn more about the work of our research integrity team to safeguard the quality of each article we publish.