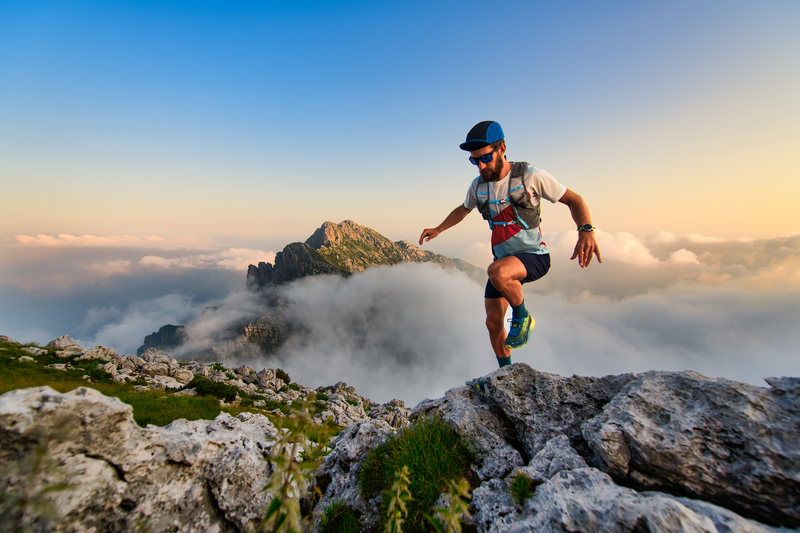
95% of researchers rate our articles as excellent or good
Learn more about the work of our research integrity team to safeguard the quality of each article we publish.
Find out more
ORIGINAL RESEARCH article
Front. Epidemiol. , 21 March 2023
Sec. Epidemiology of Chronic Diseases and Prevention
Volume 3 - 2023 | https://doi.org/10.3389/fepid.2023.1054108
This article is part of the Research Topic Insights in Epidemiology of Chronic Diseases and Prevention: 2022 View all 5 articles
Introduction: In sub-Saharan African settings, the increasing non-communicable disease mortality is linked to migration, which disproportionately exposes sub-populations to risk factors for co-occurring HIV and NCDs.
Methods: We examined the prevalence, patterns, and factors associated with two or more concurrent diagnoses of chronic diseases (i.e., multimorbidity) among temporary within-country migrants. Employing a cross-sectional design, our study sample comprised 2144 residents and non-residents 18–40 years interviewed and with measured biomarkers in 2018 in Wave 1 of the Migrant Health Follow-up Study (MHFUS), drawn from the Agincourt Health and Demographic Surveillance System (AHDSS) in rural north-eastern South Africa. We used modified Poisson regression models to estimate the association between migration status and prevalent chronic multimorbidity conditional on age, sex, education, and healthcare utilisation.
Results: Overall, 301 participants (14%; 95% CI 12.6–15.6), median age 31 years had chronic multimorbidity. Multimorbidity was more prevalent among non-migrants (14.6%; 95% CI 12.8–16.4) compared to migrants (12.8%; 95% CI 10.3–15.7). Non-migrants also had the greatest burden of dual-overlapping chronic morbidities, such as HIV-obesity 5.7%. Multimorbidity was 2.6 times as prevalent (PR 2.65. 95% CI 2.07–3.39) among women compared to men. Among migrants, men, and individuals with secondary or tertiary education manifested lower prevalence of two or more conditions.
Discussion: In a rural community with colliding epidemics, we found low but significant multimorbidity driven by a trio of conditions: HIV, hypertension, and obesity. Understanding the multimorbidity burden associated with early adulthood exposures, including potential protective factors (i.e., migration coupled with education), is a critical first step towards improving secondary and tertiary prevention for chronic disease among highly mobile marginalised sub-populations.
In the past two post-apartheid decades, South Africa has continued to experience rising levels of within-country rural-to-urban migration, which coincides with an emerging double burden of communicable and non-communicable diseases (1). This epidemiologic transition within the epicentre of the HIV pandemic complicates population health needs as the prevalence of multimorbidity (co-occurring chronic conditions) increases and migration patterns diversify (2–4). About 25% of South African households have a member who is currently living away from home (5)—a legacy of apartheid-era policies designed to simultaneously coerce African men to move into cities to support the mining and industrial complex while prohibiting them from living in these areas permanently or bringing their rural-based families with them (6). Today, high migration flows, increasingly involving women, to rapidly urbanising locations are a constant feature associated with the search for employment opportunities and an improved quality of life (7).
Despite the urban promise of economic and education opportunities, frequent relocations disproportionately expose migrants to stressors, behaviours, occupations, and environments that put them at risk of acute and chronic health conditions (8, 9). One contributor is the “urban penalty”: migrants from rural areas often settle in crowded informal settlements near major cities where they work, and the air pollution, and sanitation challenges confer risks of respiratory illness and infectious diseases (10). These jobs are often unstable, stressful, and dangerous, placing migrants at risk of injuries and adverse mental health outcomes (11). Outside of work, living away from home uproots migrants from their social and sexual networks, which increases stress, reduces social support, and may lead to high-risk sexual encounters (12) within areas with a high prevalence of HIV. Daily life is also affected by acculturation and urbanisation: nutritional changes such as the consumption of unhealthy “fast” foods and adopting sedentary habits (11) elevate the risk of cardio-vascular outcomes. Before migrants encounter these risks, they must be healthy enough to undertake the move (13), but after migration, they often utilise healthcare services less frequently (14, 15) than their non-migrant counterparts. As a result, they may miss opportunities for disease screening, diagnoses, and treatment at a time when they need these services the most.
Examining the scale of multiple disease burden in light of health transition has been an emerging priority in South Africa with several studies linking long-term survival on antiretroviral therapy to greater risk of developing other concurrent complications (16–20). Lalkhen and Mash (21) estimated the prevalence of multimorbidity among in-patients with NCDs in South Africa. However, this study was weakened by exclusively recruiting a hospital-based population (i.e., health-seekers, likely with advanced disease), potentially underestimating the totality of patients with morbidities managed with less frequent visitations to healthcare facilities, or undiagnosed multimorbidity in communities. Only recent studies taking a population-based approach (22), have identified mechanisms behind comorbid NCDs and HIV, with prevalent NCDs being significantly associated with female gender, high cholesterol, older age, and higher wealth status (23). One study found that being an internal migrant or immigrant, was associated with lower hypertension and diabetes risk independently based on self-reported diagnoses of these morbidities (24), reflecting the varying definition and measurement considerations of migration status needed to understand the current evidence landscape.
The Agincourt Health and Demographic Surveillance System (AHDSS) via the Migrant Health Follow-Up Study (MHFUS), an open general population-based census and cohort set up to explore internal migration and prevalent communicable and NCDs in the Bushbuckridge District, South Africa, captures residential location data of individuals from study communities, availing a rare opportunity to cross-sectionally examine the association between internal migration (including destination factors) and multimorbidity. As research unfolds, there is greater need to broaden our understanding of the burden of multimorbidity among populations hypothesized to have an increased disease risk, such as temporary labour migrants. As opportunities for employment continue to require migration to urban settings, those who move may have poor access to preventive interventions and appropriate care for chronic conditions in the new locations. Given the high HIV risk among migrants (25–27) and associated treatment engagement complications, analysis focused on multimorbidity is a crucial first step to identify modifiable infectious and non-communicable disease risk factors, filling public health gaps.
In this study we examine prevalence, patterns, and factors associated with the multimorbidity burden within a highly mobile cohort using population-based cross-sectional data from a rural-origin community in north-eastern South Africa undergoing health transition. Building on past evidence, we hypothesized that multimorbidity is associated with a complex interplay of individual, social, and environmental factors, and is expected to vary based on age, gender, migration status, and access to healthcare.
We used data from Wave 1 of the Migrant Health Follow-Up Study (MHFUS), a five-year cohort study of resident and non-resident adults aged between 18 and 40 years from the ongoing Agincourt Health and Demographic Surveillance System (AHDSS) collected in 2018 (15). The AHDSS study site, which is in the subdistrict of Bushbuckridge, Mpumalanga Province, South Africa, is characterised by high unemployment rates, such that rural livelihoods depend on labour migration. For instance, between 2013 and 2017, just over 60% of men aged 30–44 years had migrated (15) and many remit wages back to their origin households contributing to household income (28). The high prevalence of temporary migration in younger adult ages motivated the choice of age range on which the cohort was based. Analysis of prior data from the AHDSS has shown circular migration to worsen the burden of disease and mortality, demonstrated by excess AIDS- and TB-related mortality among returning migrants compared to permanent residents of the Agincourt study area between 1994 and 2006, increasing the crude death rate by 78·7% [95% CI, 77·4–80·1] for men and 44·4% [95% CI, 43·2–46·1] for women (29). However, longer duration migration among temporary migrants has been found to be associated with reduced risk of non-communicable disease mortality over time compared to non-migrants, HR = 0.60 (0.48–0.74) between 2008 and 2011 (11).
The MHFUS questionnaire collected data on residence history, socio-demographic factors and self-reported healthcare utilisation. In addition, several biomarkers were measured at baseline, Wave 1, on a sub-sample including dried-blood spot (DBS) specimens via a finger prick to determine HIV antibodies, and glucose. Blood glucose levels were measured through a haemoglobin A1C test. Blood pressure (BP) was measured with the Omron (HBP-1100-E), a clinically validated automatic BP monitor. Height and weight were measured, and body mass index (BMI) calculated.
A simple random sample of 3,800 resident and non-resident adults aged between 18 and 40 years was drawn from the AHDSS, but 702 potential participants were not interviewed in Wave 1 because of ineligibility, refusals, or because they or their households were untraceable (15). Wave 1 data (2018) from the MHFUS consisted of 3,098 participants, with biomarker measures available from 2,144 people. This multimorbidity study is based upon the sub-sample of 2,144 participants who consented and had specimen collection, anthropometric measurements, and self-reported health history.
Participants were either resident (located within the surveillance site in Agincourt/Bushbuckridge) or non-resident members (i.e., migrants living outside of the study site). For recruitment of non-resident members, current contact information was availed through a member of the origin household and fieldworkers reached them in their destinations for face-to-face interviews upon scheduled visits. Although most migrants do not sever relations with the origin household (thus considered temporary migrants), MHFUS retains in its sample those individuals who are no longer considered AHDSS household members.
The AHDSS has had a 30-year presence in the study site and established efforts to continuously involve participants through the Public Engagement Office. For instance, the political and traditional hierarchy of the constituent villages are consulted annually prior to each census launch through Agincourt’s Community Advisory Board (CAB). Importantly, focus for this study (i.e., inclusion of questions on chronic conditions and collection biological samples and data) builds on pre-existing empirical HIV and NCD mortality data in the migrant population of this rural community (8, 19, 30), serving as direct input to the design of this study. Thus, building on the long-running connection and ongoing links to the community enabled the follow-up of migrants.
Ethical approval was obtained from University of the Witwatersrand Human Research Ethics Committee (clearance certificate number M170277) and the Mpumalanga Province Human Research Ethics Committee, South Africa. Written informed consent was obtained from all participants and consent material was translated into the local Xitsonga language, with verbal consent received from those interviewed via telephone.
The primary outcome of interest is multimorbidity, defined as two or more co-existing chronic (i.e., long-term) conditions, of the nine conditions that were investigated: HIV, tuberculosis (TB), hypertension, obesity, diabetes, asthma, depression, chronic obstructive pulmonary disease (COPD), and stroke.
We collected biomarker measures for four (HIV, hypertension, obesity, and diabetes) of the nine conditions. For the other five conditions, diagnoses were self-reported but not validated for all the 2,144 participants via either face-to-face or telephone interviews. Thus, no verification of disease status was performed through confirmatory biomarker testing or medical records at data collection for these five conditions. As per study design, BP readings were taken three times sequentially after five minutes of rest with two-minute intervals between measurements. Hypertension was defined as mean systolic BP ≥140 mm Hg or mean diastolic BP ≥90 mm Hg using the mean of the second and third BP readings, or self-reported current treatment for hypertension. Obesity was defined as BMI ≥30 kg/m2. Diabetes was determined by having hemoglobin A1C levels of ≥6.5% or self-reported diagnosis. In summary, 2,144 participants had both biomarker/anthropometric and self-reported data to assign disease (i.e., to merit inclusion in our multimorbidity definition), thus comprising the analytic sample for this sub-study.
In Wave 1, interview respondents were required to provide written informed consent for both completing the interview and presenting for DBS collection to enable HIV testing. DBS collection was only possible for respondents available for in-person interviews. Many respondents in more remote locations were interviewed telephonically and thus have no biomarker information; others refused DBS-HIV testing. Supplementary Table S1 presents characteristics of those included and excluded from the study. Our analytic sample of 2,144 excluded 954 participants from the original total of 3,098 due to mode of interview (phone) or refusal for anthropometric or HIV measurement. These individuals were disproportionately migrants, and particularly migrants less geographically accessible to our fieldwork teams. In turn these excluded individuals were somewhat more likely to be male, have tertiary education, and were less likely to be in the youngest (18–24) age group (see Supplementary S1). Given that migrants were more represented within the excluded population highlights challenges of collecting data for highly mobile populations, who constituted 43.1% of the wider initial 3,098 sample. For participants with complete biomarker information, positive HIV diagnosis was determined by a participant testing positive using an HIV ELISA test.
The primary exposure of the study was migration status of men and women aged 18–40 years. We defined migrant status based on current residence status, defined as a respondent's current place of residence—or the place where a respondent typically spent four or more nights a week. The questionnaire validated the answer to this open-ended question through a sequence of branched questions about the province and location of current residence. Participants living in one of the 31 constituent villages of the Agincourt study site in Mpumalanga province were categorised as Agincourt residents/non-migrants, while respondents residing outside of the study area were assigned as migrants.
The covariates examined include sex, age, and education status. For primary healthcare system utilised, categories were western biomedical services, faith/traditional/western combined (i.e., medical pluralism) and no healthcare utilisation history in the last 12 months.
First, we summarised participants' key socio-demographic and health characteristics by sex using descriptive statistics, frequencies, percentages and chi-squares. Second, we measured the distribution of single morbidities for participants with one condition and then repeated this by migration status, assessing the relationship between individual health conditions using correlation analysis. We further identified common dual-disease combinations and measured the frequency of disease patterns for both the migrant and non-migrant groups. Lastly, we fitted modified Poisson regression models to independently estimate the prevalence of multimorbidity for key socio-demographic and health factors. In separate models, we included interaction terms between sex, education, and migration status with multimorbidity to consider relationships suggested in prior studies (31, 32).
Of the 2,144 participants in the present multimorbidity analysis, 35% were aged between 18 and 24 years, median age 31 years (IQR = 9.4), 30% were living outside of the AHDSS study area (migrants) at the time of the Wave 1 interview, and 47% had exclusively used formal health care services in the prior year (Table 1). Using our definition of multimorbidity, 14% of respondents had at least two or more conditions. Comparing prevalence across migration profiles, 14.6% of non-migrants (95%CI, 12.8–16.4) compared to 12.9% of migrants (95% CI, 10.3–15.7) had multimorbidity. The prevalence of chronic conditions varied, with 24% (n = 513) being HIV positive, 19.9% (n = 428) having obesity, 16.6% (n = 356) having hypertension and 3.9% (n = 83) having diabetes. There were significant differences in the prevalence of conditions by migration status, 25.3% of non-migrants compared to 20.7% migrants had tested HIV positive, 20.5% non-migrants vs. 18.7% migrants had obesity and more migrants (21.9%) compared to non-migrants (14.4%) had hypertension. We detected non-negligible differences by migration status in the distributions for gender, age, education status, primary healthcare system, number of health conditions and the prevalence of “Other” (low prevalence) conditions.
Table 1. Description of the sociodemographic and health characteristics of adults in the multimorbidity sample from the migrant health follow-up study (MHFUS) (N = 2,144).
A total of 1,055 adults had at least one of nine conditions (Figure 1). HIV accounted for the largest share of single disease morbidities 36% (95% CI, 33.8–38.9) followed by obesity 30.3% (95% CI, 27.9–32.8) and hypertension 25.2% (95% CI, 22.9–27.6).
Figure 1. Distribution of existing single diseases among those with at least one morbidity in the MHFUS (n = 1,055).
Table 2 presents the correlation matrix of pairwise comorbidities for those with at least one condition i.e., 1,055 of 2,144 participants. To highlight significant results, the largest correlation between health conditions was between hypertension and obesity (tetrachoric rho = 0.31). We also observed large correlations between diabetes and obesity (tetrachoric rho = 0.24) as well as HIV and other lesser represented chronic conditions (tetrachoric rho = 0.26). This reflects the clinical importance of both obesity and HIV, as other additional health conditions (hypertension and diabetes) were associated with being obese or HIV positive.
Table 2. Correlation matrices for pairwise comorbidities in the MHFUS for those with two or more conditions (N = 301).
We found that hypertension and obesity comorbidity accounted for 3.9% (95% CI, 3.1–4.8) of all those with two or more diseases (n = 84 out of 2144) (Table 3). Non-migrants compared to migrants had a greater burden of dual overlapping chronic and cardio-metabolic conditions, such as HIV-obesity (4%) p < 0.001 and diabetes-obesity pairings (1%) p < 0.001. In other infrequent combinations, obesity co-occurred with several other respiratory and metabolic disorders such as TB and diabetes.
Several sociodemographic factors (age, sex, education, and healthcare utilisation) were associated with multimorbidity in multivariate regressions (Table 4). Multimorbidity was higher in women compared to men, those aged 25–29 years, 30–34 years and ≥35 years compared to 18–24 years, individuals using formal healthcare exclusively in the past 12 months compared to those with no such healthcare use history. In contrast, multimorbidity was lower among individuals with secondary education (PR = 0.57, 95% CI, 0.39–0.84) compared to primary.
Table 4. Multimorbidity prevalence ratios for socio-demographic and health factors in a rural population, South Africa (N = 2,144).
Table 4 highlights further results based on interaction effects. Prevalent multimorbidity was low among both migrant and non-migrant men compared to non-migrant women, with a prevalence ratio of (PR = 0.36, 95% CI, 0.24–0.54) and (PR = 0.39, 95% CI, 0.29–0.52), respectively, however migrant-non-migrant differences among men were negligible. While greater educational attainment is associated with lower prevalence of multimorbidity, as above, further differentiation by migrant status is modest. Migrant-non-migrant differences among those with secondary education are marginal (PR = 0.62, 95% CI, 0.39–0.99 vs. PR = 0.57 (95% CI, 0.37–0.88). Migrant-non-migrant differences among those with tertiary education are more evident (PR = 0.32, 95% CI, 0.15–0.65 vs. PR = 0.98, CI, 0.60–1.61).
This study set out to characterise multimorbidity patterns and identify associated risk factors, including migration status, in a rural community in north-eastern South Africa. In this study sample of persons aged 18–40, we found that 14% of the participants had prevalent chronic multimorbidity (≥2 conditions). Migrants exhibited a slightly lower prevalence of multimorbidity than non-migrants. HIV (24%) was the most prevalent morbidity; for instance, 25.3% of non-migrants and 20.7% of migrants had tested HIV positive. Overall, obesity and hypertension had greater co-existence in the prevalence of pairwise morbidities of the four measured conditions and five other minor conditions. The prevalence ratio for multimorbidity 2.65 higher among women compared to men and 5.61 higher among those aged over 35 years compared to 18–24 years.
HIV, hypertension and obesity were somewhat equally represented among the nine conditions considered in this study. This disease profile mirrors a turning point through early phase health transition marked by waning dominance of infectious diseases before experiencing an era of chronic non-communicable diseases (NCDs) (33). This may be in large part due the adoption of unhealthy, sedentary habits that include reduced exercise, poor dietary choices, alcohol consumption and smoking (34) Rural communities represent an important epidemiological paradox; they serve as a net receiver of return-migrants who potentially have higher levels of exposure to HIV or NCD risk factors (8, 35, 36) than the permanent residents of the rural “home”. Health facilities in rural areas face an increasing demand for appropriate care for chronic conditions in the context of underdeveloped secondary care models (20).
The attenuating effect of higher education status on multimorbidity for migrants provides important insight into identifying protective characteristics against adverse consequences in the context of ongoing temporary migration in South Africa. Mobility increases the burden of morbidity by disconnecting patients from clinic-based HIV care (i.e., leading to poor drug adherence) and erratic secondary healthcare utilisation, which delays disease prevention, diagnoses and treatment normally supported through routine NCD screening and continued engagement to care (37, 38). Recent evidence from the Agincourt study area showed that migrants compared to non-migrants, and men compared to women, each had ∼70% lower odds of health service use in the last 12-months among individuals living with a chronic condition (15). However to interpret of our finding, there are two scenarios to consider; that educational and health selectivity upon migration (39, 40) or observed health and wellbeing benefits occurring post-migration (41) is mediated through improved socio-economic status (42, 43), which cannot be demonstrated using a cross-sectional design. Taking previous data into consideration, we posit that while migrants are at risk of gaps in healthcare engagement continuity, being more educated (i.e., higher socio-economic status and health literacy) helps overcoming barriers to accessing healthcare (i.e., both when at destination and origin) confers the preventive benefit to multimorbidity. Despite concerns about potential misclassification, we provide important single time-point data to support the health advantage enjoyed by more educated migrants from rural areas residing in urban areas, thus helping to close the health gap across by migration status.
Our study further demonstrates lower prevalent multimorbidity among migrant men however, migrant women registered no such significantly lower rates which may be due to gendered destination dynamics systematically promoting men and not women's health. Beyond the stressful migration process, women are expected to continue social roles such as being the homemaker and taking care of family and may lack opportunities for social integration compared to men (44). We echo others' (45) attribution of elevated BP measures, a driver of syndemic NCDs—diabetes and obesity, among migrant women compared to men. Thus, indicative of two scenarios; that women either accrue fewer health benefits from urban living than men do, or the benefits women accrue do not manifest as lower BP as is the case for men (45).
The interpretation of our results should be in view of several limitations. We examined migration status (residing inside or outside the rural-origin Agincourt HDSS area) at a point in time using data from the first study wave. Thus, we do not account for prior years' migration history in the analysis. Moreover, the cross-sectional approach limits our ability to examine the timing of migration, diagnosis of HIV and co-existing conditions. More recently evidence exists that the migrant health advantage recedes over time, even for within-country migrants (44) warranting the need for more research accounting for other migration dimensions such as duration and recency. A strength of our study is being the first in South Africa to analyse population specific survey data recording residential patterns, sampling migrants from a rural origin area and capturing destination circumstances, such as healthcare utilisation behaviours while away from home. Community health screening data such as contained in the MHFUS provide unique non-clinic-based estimates of undiagnosed multimorbidities thus using a more versatile design than previous studies (46).
Understanding the heightened multimorbidity burden that occurs with migration is an essential first step towards improving preventive interventions for marginalised populations and underserved communities including spatially optimised access to appropriate care for mobile individuals. Results from this study address clinical and public health gaps, implying the need for expanded scope of community-based health screening services beyond HIV to include NCDs and the risk factors preceding morbidity (22). In keeping with this, classification of the four main morbidities examined in this research study, HIV, hypertension, and obesity were ascertained through biomarker data collection, which can be a model for the expansion of testing services into communities, which is a new focus in South Africa (47). Building on learnings from HIV care services (with a comprehensive network of intermediaries in South Africa), healthcare delivery can also be adapted to monitor comorbidities and NCDs in young women in particular (48). Additionally, interventions involving early initiation of longer-term life-course care can be explored, building on debut presentation of women for sexual reproductive health services (49, 50). Awareness interventions for younger populations can be uniquely customised to counteract personal perceptions (51–53). For instance, these may help to improve the effectiveness of weight loss campaigns and help in tackling obesity.
In a rural South African community with colliding epidemics of communicable and non-communicable diseases, we assessed multimorbidity prevalence. Among adults aged 18–40, we found high prevalent trio conditions HIV, obesity, and hypertension. Migrants were observed to have multimorbidity pairings that were higher for HIV-Hypertension and lower for HIV-Obesity. Unadjusted migrant-non-migrant differentials in overall multimorbidity prevalence were modest, but selected interactions with demographic characteristics were observed in multivariate statistical analysis. These multivariate statistical analyses also revealed, notably, that women, those with limited education, and those older than 24 years manifested a higher prevalence of two or more conditions. These data highlight longstanding gaps in achieving health equity for these vulnerable groups. Understanding the potential role of education in disrupting the multimorbidity patterns associated with early-life exposures such as migration is a critical first step towards improving chronic disease preventive interventions for marginalised populations and underserved communities including spatial optimisation of access to secondary care for those mobile.
Data from the Migrant Health Follow-Up Study are available from the corresponding author on valid request. Agincourt Health and Demographic Surveillance Systems data are available through SAPRIN URL http://saprin.mrc.ac.za/ and http://saprindata.samrc.ac.za/.
The studies involving human participants were reviewed and approved by Ethical approval for data collection, linkage, and analysis was obtained from University of the Witwatersrand Human Research Ethics Committee (clearance certificate number M170277) and the Mpumalanga Province Human Research Ethics Committee, South Africa. The patients/participants provided their written informed consent to participate in this study.
FXG-O and AD conceived the work. FXG-O, AD and CWK designed the analysis. AD conducted the analysis and developed initial drafts under the supervision of FXG-O. CG and CWK contributed to early conceptual and analysis support from design through interpretation phases. MW, STM and CG rigorously reviewed the manuscript pre- and post-initial journal submission. All authors revised and approved the final draft. The corresponding author attests that all listed authors meet the guidelines for authorship and that no others meeting the criteria have been omitted. All authors contributed to the article and approved the submitted version.
The MHFUS study received financial support from US NIH Grant 1R01HD083374, “Migration Urbanization and Health in a Transition Setting” (PI: MJW). It is nested within the Agincourt Health and Demographic Surveillance System site/The MRC/Wits Rural Public Health and Health Transitions Research Unit (Agincourt) funded from The Wellcome Trust, UK (grants 058893/Z/99/A; 069683/Z/02/Z; 085477/Z/08/Z; 085477/B/08/Z) (https://wellcome.ac.uk/), and the Medical Research Council, South Africa, (http://www.mrc.ac.za/) and the South African Department of Science and Innovation through the South African Population Research Infrastructure Network hosted by the South African Medical Research Council (https://www.dst.gov.za/). MJW, RRY, ML and STM's affiliation Brown University Population Studies Training Center receives funding from an NIH P2CHD041020 grant (https://www.nih.gov/).
We would like to thank Stephen B. Asiimwe for the early data analysis advisory and technical support on which we built to produce this work.
The authors declare that the research was conducted in the absence of any commercial or financial relationships that could be construed as a potential conflict of interest.
All claims expressed in this article are solely those of the authors and do not necessarily represent those of their affiliated organizations, or those of the publisher, the editors and the reviewers. Any product that may be evaluated in this article, or claim that may be made by its manufacturer, is not guaranteed or endorsed by the publisher.
The Supplementary Material for this article can be found online at: https://www.frontiersin.org/articles/10.3389/fepid.2023.1054108/full#supplementary-material.
AHDSS, Agincourt Health and Demographic Surveillance System; AIDS, Acquired Immune Deficiency Syndrome; BP, Blood Pressure; BMI, Body Mass Index; CAB, Community Advisory Board; COPDvChronic Obstructive Pulmonary Disease; CI, Confidence Interval; DBS, Dried Blood Spot; HIV, Human Immune Virus; HR, Hazard Ratio; NCD, Non-Communicable Disease; MHFUS, Migrant Health Follow-Up Study; PR, Prevalence Ratio; SES, Socio-Economic Status; TB, Tuberculosis.
1. Ajayi IO, Adebamowo C, Adami H-O, Dalal S, Diamond MB, Bajunirwe F, et al. Urban–rural and geographic differences in overweight and obesity in four sub-Saharan African adult populations: a multi-country cross-sectional study. BMC Public Health. (2016) 16(1):1126. doi: 10.1186/s12889-016-3789-z
2. Collinson MA. Striving against adversity: the dynamics of migration, health and poverty in rural South Africa. Glob Health Action. (2010) 3(1):5080. doi: 10.3402/gha.v3i0.5080
3. Reed HE. Moving across boundaries: migration in South Africa, 1950–2000. Demography. (2013) 50(1):71–95. doi: 10.1007/s13524-012-0140-x
4. Dobra A, Bärnighausen T, Vandormael A, Tanser F. Space-time migration patterns and risk of HIV acquisition in rural South Africa. AIDS. (2017) 31(1):137–45. doi: 10.1097/QAD.0000000000001292
5. Posel D, Casale D. Moving during times of crisis: migration, living arrangements and COVID-19 in South Africa. Sci Afr. (2021) 13:e00926. doi: 10.1016/j.sciaf.2021.e00926
6. Lurie M. Migration and AIDS in Southern Africa: a review. S Afr J Sci. (2000) 96(6):343–7. doi: 10.1080/21642850.2013.866898
7. Muhwava W, Hosegood V, Nyirenda M, Herbst K, Newell M-L. Levels and determinants of migration in rural KwaZulu-natal, South Africa. Afr Popul Stud. (2013) 24(3):243–302. doi: 10.11564/24-3-302
8. Clark SJ, Collinson MA, Kahn K, Drullinger K, Tollman SM. Returning home to die: circular labour migration and mortality in South Africa. Scandanavian J Public Health. (2007) 35:35–44. doi: 10.1080/14034950701355619
9. Bernays S, Lanyon C, Dlamini V, Ngwenya N, Seeley J. Being young and on the move in South Africa: how “waithood” exacerbates HIV risks and disrupts the success of current HIV prevention interventions. Vulnerable Child Youth Stud. (2020) 15(4):368–78. doi: 10.1080/17450128.2020.1739359
10. Vearey J, Palmary I, Thomas L, Nunez L, Drimie S. Urban health in Johannesburg: the importance of place in understanding intra-urban inequalities in a context of migration and HIV. Health Place. (2010) 16(4):694–702. doi: 10.1016/j.healthplace.2010.02.007
11. Collinson M, White M, Bocquier P, McGarvey S. Migration and the epidemiological transition: insights from the Agincourt sub-district of northeast South Africa. Glob Health Action. (2014) 7(1):23514. doi: 10.3402/gha.v7.23514
12. Olawore O, Tobian AAR, Kagaayi J, Bazaale JM, Nantume B, Kigozi G, et al. Migration and risk of HIV acquisition in Rakai, Uganda: a population-based cohort study. Lancet HIV. (2018) 5(4):e181–9. doi: 10.1016/S2352-3018(18)30009-2
13. Anglewicz P, VanLandingham M, Manda-Taylor L, Kohler H-P. Health selection, migration, and HIV infection in Malawi. Demography. (2018) 55(3):979–1007. doi: 10.1007/s13524-018-0668-5
14. Tanser F, Bärnighausen T, Vandormael A, Dobra A. HIV Treatment cascade in migrants and mobile populations. Curr Opin HIV&AIDS. (2015) 10(6):430–8. doi: 10.1097/COH.0000000000000192
15. Ginsburg C, Collinson MA, Gómez-Olivé FX, Gross M, Harawa S, Lurie MN, et al. Internal migration and health in South Africa: determinants of healthcare utilisation in a young adult cohort. BMC Public Health. (2021) 1:1–5. doi: 10.1186/s12889-021-10590-6
16. Bärnighausen T, Welz T, Hosegood V, Bätzing-Feigenbaum J, Tanser F, Herbst K, et al. Hiding in the shadows of the HIV epidemic: obesity and hypertension in a rural population with very high HIV prevalence in South Africa. J Hum Hypertens:(2008) 22(3):236–9. doi: 10.1038/sj.jhh.1002308
17. Levitt NS, Steyn K, Dave J, Bradshaw D. Chronic noncommunicable diseases and HIV-AIDS on a collision course: relevance for health care delivery, particularly in low-resource settings—insights from South Africa. Am J Clin Nutr. (2011) 94(6):1690S–6S. doi: 10.3945/ajcn.111.019075
18. Oni T, Youngblood E, Boulle A, McGrath N, Wilkinson RJ, Levitt NS. Patterns of HIV, TB, and non-communicable disease multi-morbidity in peri-urban South Africa- a cross sectional study. BMC Infect Dis. (2015) 15(1):20. doi: 10.1186/s12879-015-0750-1
19. Clark SJ, Gómez-Olivé FX, Houle B, Thorogood M, Klipstein-Grobusch K, Angotti N, et al. Cardiometabolic disease risk and HIV status in rural South Africa: establishing a baseline. BMC Public Health. (2015) 15(1):1–9. doi: 10.1186/s12889-015-1467-1
20. Osetinsky B, Hontelez JAC, Lurie MN, McGarvey ST, Bloomfield GS, Pastakia SD, et al. Epidemiological and health systems implications of evolving HIV and hypertension in South Africa and Kenya. Health Aff. (2019) 38(7):1173–81. doi: 10.1377/hlthaff.2018.05287
21. Lalkhen H, Mash R. Multimorbidity in non-communicable diseases in South African primary healthcare. S Afr Med J. (2015) 105(2):134–8. doi: 10.7196/SAMJ.8696
22. van Heerden A, Barnabas RV, Norris SA, Micklesfield LK, van Rooyen H, Celum C. High prevalence of HIV and non-communicable disease (NCD) risk factors in rural KwaZulu-Natal, South Africa. J Int AIDS Soc. (2017) 20(2):e25012. doi: 10.1002/jia2.25012
23. Chang AY, Gómez-Olivé FX, Payne C, Rohr JK, Manne-Goehler J, Wade AN, et al. Chronic multimorbidity among older adults in rural South Africa. BMJ Glob Health. (2019) 4(4):e001386. doi: 10.1136/bmjgh-2018-001386
24. Motlhale M, Ncayiyana JR. Migration status and prevalence of diabetes and hypertension in Gauteng province, South Africa: effect modification by demographic and socioeconomic characteristics—a cross-sectional population-based study. BMJ Open. (2019) 9(9):e027427. doi: 10.1136/bmjopen-2018-027427
25. Dzomba A, Tomita A, Vandormael A, Govender K, Tanser F. Effect of ART scale-up and female migration intensity on risk of HIV acquisition: results from a population-based cohort in KwaZulu-Natal, South Africa. BMC Public Health. (2019) 19(1):1–8. doi: 10.1186/s12889-019-6494-x
26. Dzomba A, Tomita A, Govender K, Tanser F. Effects of migration on risky sexual behavior and HIV acquisition in South Africa. AIDS Behav. (2019) 23(6):1396–430. doi: 10.1007/s10461-018-2367-z
27. McGrath N, Bärnighausen T, Iwuji C, Diallo MH, Thiébaut R, Pillay D, et al. The impact of population dynamics on the population HIV care cascade: results from the ANRS 12249 treatment as prevention trial in rural KwaZulu-Natal (South Africa). J Int AIDS Soc. (2018) 21:e25128. doi: 10.1002/jia2.25128
28. Collinson MA, Biyase M. Migration and remittances in South Africa. Migration and remittances in South Africa. In: Oqubay A, Tregenna F, Valodia I, editors. The Oxford handbook of the South African economy. Oxford: Oxford University Press (2021) p. 777–9.
29. Bocquier P, Collinson MA, Clark SJ, Gerritsen AAM, Kahn K, Tollman SM. Ubiquitous burden: the contribution of migration to AIDS and Tuberculosis mortality in rural South Africa. Etude Popul Afr. (2014) 28(1):691–701. doi: 10.11564/28-0-525
30. Ginsburg C, Collinson MA, Gomez-Olive FX, Gross M, Harawa S, Lurie M, et al. Internal migration and health in South Africa: determinants of healthcare utilisation in a young adult cohort. BMC Public Health. (2021) 21:554. doi: 10.1186/s12889-021-10590-6
31. Lewis EG, Gray WK, Walker R, Urasa S, Witham M, Dotchin C. The prevalence of multimorbidity and its associations in community-dwelling older adults in rural Tanzania; a cross-sectional study. doi: 10.21203/rs.3.rs-1473383/v1
32. Sharma P, Maurya P. Gender differences in the prevalence and pattern of disease combination of chronic multimorbidity among Indian elderly. Ageing Int. (2022) 47(2):265–83. doi: 10.1007/s12126-021-09419-9
33. Omran AR. The epidemiologic transition theory revisited thirty years later. World Health Stat Q. (1998) 53(2–4):99–119. 10030991354
34. Monti M, Ruggieri MP, Vincentelli GM, Capuano F, Pugliese FR. Cardiovascular risk factors in sub-Saharan Africa: a review. Italian J Med. (2015) 9(4):305–13. doi: 10.4081/itjm.2015.533
35. Welaga P, Hosegood V, Weiner R, Hill C, Herbst K, Newell ML. Coming home to die? The association between migration and mortality in rural South Africa. BMC Public Health. (2009) 9(1):1–8. doi: 10.1186/1471-2458-9-193
36. Addressing the global challenges of multimorbidity-lessons from South Africa. Available from: https://acmedsci.ac.uk/file-download/6857885 (cited October 11, 2019).
37. Mbuagbaw L, Medley N, Darzi AJ, Richardson M, Garga KH, Ongolo-Zogo P. Health system and community level interventions for improving antenatal care coverage and health outcomes. Cochrane Database Syst Rev. (2015) 2015(12):1–156. doi: 10.1002/14651858.CD004246.pub4
38. Colebunders R, Kenyon C. Behaviour, not mobility, is a risk factor for HIV. Lancet HIV. (2015) 2(6):e223–4. doi: 10.1016/S2352-3018(15)00057-0
39. Clouse K, Vermund SH, Maskew M, Lurie MN, MacLeod W, Malete G, et al. Mobility and clinic switching among postpartum women considered lost to HIV care in South Africa. J Acquir Immune Defic Syndr. (2017) 74(4):383–9. doi: 10.1097/QAI.0000000000001284
40. Ginsburg C, Béguy D, Augusto O, Odhiambo F, Soura A, White MJ, et al. Human capital on the move: education as a determinant of internal migration in selected INDEPTH surveillance populations in Africa. Demogr Res. (2016) 34(1):845–84. doi: 10.4054/DemRes.2016.34.30
41. Ichou M, Wallace M. The healthy immigrant effect. Demogr Res. (2019) 40:61–94. doi: 10.4054/DemRes.2019.40.4
42. Ginsburg C, Collinson MA, Gómez-Olivé FX, Harawa S, Pheiffer CF, White MJ. The impact of COVID-19 on a cohort of origin residents and internal migrants from South Africa’s rural northeast. SSM-Popul Health. (2022) 17:101049. doi: 10.1016/j.ssmph.2022.101049
43. Duboz P, Boëtsch G, Guisse A, Macia E. Assessing health impacts of an environmental pan-African development project: a migration perspective. SSM - Popul Health. (2020) 11:100633. doi: 10.1016/j.ssmph.2020.100633
44. Zhang L, Liu S, Zhang G, Wu S. Internal migration and the health of the returned population: a nationally representative study of China. BMC Public Health. (2015) 15(1):1–9. doi: 10.1186/1471-2458-15-1
45. Pheiffer CF. Internal migration, urban living, and non-communicable disease risk in South Africa. Soc Sci Med. (2021) 1(274):113785. doi: 10.1016/j.socscimed.2021.113785
46. Roomaney RA, van Wyk B, Turawa EB, Pillay-van Wyk V. Multimorbidity in South Africa: a systematic review of prevalence studies. BMJ Open. (2021) 11(10):e048676. doi: 10.1136/bmjopen-2021-048676
47. Puoane TR, Tsolekile LP, Egbujie BA, Lewy M, Sanders D. Advancing the agenda on noncommunicable diseases: prevention and management at community level. South Afr Health Rev. (2017) 1:171–9. doi: 10.10520/EJC-c84906221
48. Lankoande B, Sié A, Shapiro D. Selective adult migration and urban-rural mortality differentials in Burkina Faso. Population (Paris). (2017) 72(2):197–218. doi: 10.3917/popu.1702.0201
49. Nauman E, VanLandingham M, Anglewicz P, Patthavanit U, Punpuing S. Rural-to-urban migration and changes in health among young adults in Thailand. Demography. (2015) 52(1):233–57. doi: 10.1007/s13524-014-0365-y
50. Kintu A, Sando D, Okello S, Mutungi G, Guwatudde D, Menzies NA, et al. Integrating care for non-communicable diseases into routine HIV services: key considerations for policy design in sub-Saharan Africa. J Int AIDS Soc. (2020) 23(S1):12–7. doi: 10.1002/jia2.25508
51. Brumana L, Arroyo A, Schwalbe NR, Lehtimaki S, Hipgrave DB. Maternal and child health services and an integrated, life-cycle approach to the prevention of non-communicable diseases. BMJ Global Health. (2017) 2(3):e000295. doi: 10.1136/bmjgh-2017-000295
52. Spires M, Delobelle P, Sanders D, Puoane T, Hoelzel P, Swart R. Diet-related non-communicable diseases in South Africa: determinants and policy responses. S Afr Health Rev. (2016) 2016(1):35–42. https://hdl.handle.net/10520/EJC189320
Keywords: human migration, epidemics, infectious diseases, non-communicable diseases, LMIC
Citation: Dzomba A, Ginsburg C, Kabudula CW, Yorlets RR, Ndagurwa P, Harawa S, Lurie MN, McGarvey ST, Tollman S, Collinson MA, White MJ and Gomez-Olive FX (2023) Epidemiology of chronic multimorbidity and temporary migration in a rural South African community in health transition: A cross-sectional population-based analysis. Front. Epidemiol. 3:1054108. doi: 10.3389/fepid.2023.1054108
Received: 26 September 2022; Accepted: 3 March 2023;
Published: 21 March 2023.
Edited by:
Eva S Schernhammer, Medical University of Vienna, AustriaReviewed by:
Leslie Johnson, Emory University, United States© 2023 Dzomba, Ginsburg, Kabudula, Yorlets, Ndagurwa, Harawa, Lurie, McGarvey, Tollman, Collinson, White and Gomez-Olive. This is an open-access article distributed under the terms of the Creative Commons Attribution License (CC BY). The use, distribution or reproduction in other forums is permitted, provided the original author(s) and the copyright owner(s) are credited and that the original publication in this journal is cited, in accordance with accepted academic practice. No use, distribution or reproduction is permitted which does not comply with these terms.
*Correspondence: Armstrong Dzomba ZHpvbWJhYXJtc3Ryb25nNjI1QGdtYWlsLmNvbQ==
†These authors share last authorship
Specialty Section: This article was submitted to Epidemiology of Chronic Diseases and Prevention, a section of the journal Frontiers in Epidemiology
Disclaimer: All claims expressed in this article are solely those of the authors and do not necessarily represent those of their affiliated organizations, or those of the publisher, the editors and the reviewers. Any product that may be evaluated in this article or claim that may be made by its manufacturer is not guaranteed or endorsed by the publisher.
Research integrity at Frontiers
Learn more about the work of our research integrity team to safeguard the quality of each article we publish.