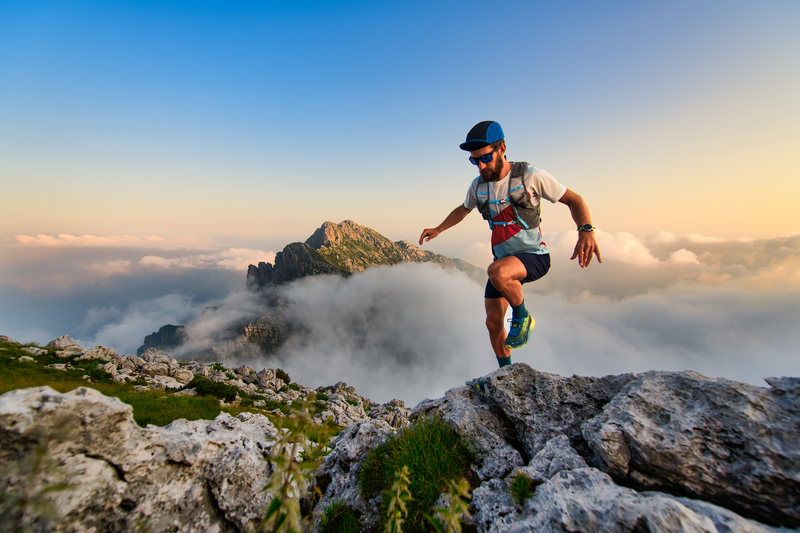
94% of researchers rate our articles as excellent or good
Learn more about the work of our research integrity team to safeguard the quality of each article we publish.
Find out more
EDITORIAL article
Front. Environ. Sci.
Sec. Environmental Informatics and Remote Sensing
Volume 13 - 2025 | doi: 10.3389/fenvs.2025.1584485
This article is part of the Research Topic Advancements in Land Cover Classification and Machine Learning Techniques for Urban Areas Using Remote Sensing Big Data View all 5 articles
The final, formatted version of the article will be published soon.
You have multiple emails registered with Frontiers:
Please enter your email address:
If you already have an account, please login
You don't have a Frontiers account ? You can register here
This innovative approach improves building feature extraction by enhancing the model's ability to detect structures at various scales and levels. The method demonstrates a high level of precision (95.17%) and an Intersection over Union (IoU) of 90.18%, proving its effectiveness for urban planning and land resource management 2024) explores the integration of machine learning and geospatial analysis for predicting gully erosion susceptibility in the Erer watershed of Ethiopia. Using models such as XGBoost, Random Forest, Support Vector Machine (SVM), and Artificial Neural Networks (ANN), the study identifies key geo-environmental factors contributing to gully erosion. The results reveal that XGBoost is the most robust model, offering a comprehensive approach for erosion susceptibility mapping. This work underscores the value of machine learning in addressing critical land degradation issues and supports more sustainable land management practices.Together, these studies represent significant advancements in the integration of machine learning with remote sensing data, contributing to improved land cover classification, environmental monitoring, and natural resource management. The methodologies discussed here are not only enhancing our ability to process vast amounts of remote sensing data but also enabling more accurate and actionable insights for urban planning and sustainable development. As remote sensing and machine learning technologies continue to evolve, we can anticipate even greater progress in the ability to monitor and manage land cover changes, offering powerful tools for conservation and urban management in the future.
Keywords: land cover classification, machine learning, Remote sensing big data, satellite image processing, feature extraction, change detection, Classification algorithms, deep learning
Received: 27 Feb 2025; Accepted: 28 Feb 2025.
Copyright: © 2025 Al Najjar, Kalantar and ABDUL HALIN. This is an open-access article distributed under the terms of the Creative Commons Attribution License (CC BY). The use, distribution or reproduction in other forums is permitted, provided the original author(s) or licensor are credited and that the original publication in this journal is cited, in accordance with accepted academic practice. No use, distribution or reproduction is permitted which does not comply with these terms.
* Correspondence:
Husam Al Najjar, University of Technology Sydney, Sydney, Australia
Bahareh Kalantar, RIKEN Center for Advanced Intelligence Project (AIP), Chuo-ku, 351-0198, Tokyo, Japan
ALFIAN ABDUL HALIN, Faculty of Computer Science and Information Technology, Putra Malaysia University, Serdang, 43400, Selangor, Malaysia
Disclaimer: All claims expressed in this article are solely those of the authors and do not necessarily represent those of their affiliated organizations, or those of the publisher, the editors and the reviewers. Any product that may be evaluated in this article or claim that may be made by its manufacturer is not guaranteed or endorsed by the publisher.
Research integrity at Frontiers
Learn more about the work of our research integrity team to safeguard the quality of each article we publish.