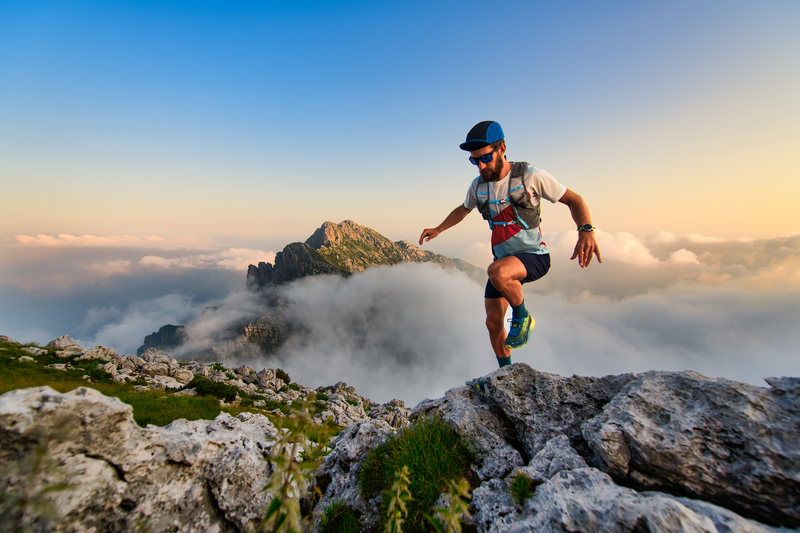
95% of researchers rate our articles as excellent or good
Learn more about the work of our research integrity team to safeguard the quality of each article we publish.
Find out more
ORIGINAL RESEARCH article
Front. Environ. Sci.
Sec. Environmental Informatics and Remote Sensing
Volume 13 - 2025 | doi: 10.3389/fenvs.2025.1580797
This article is part of the Research Topic New Artificial Intelligence Methods for Remote Sensing Monitoring of Coastal Cities and Environment View all articles
The final, formatted version of the article will be published soon.
You have multiple emails registered with Frontiers:
Please enter your email address:
If you already have an account, please login
You don't have a Frontiers account ? You can register here
With the advent of remote sensing (RS) big data, deep learning (DL) has shown remarkable potential in uncovering intricate features and detecting environmental changes from vast RS imagery, particularly for intelligent environmental monitoring, such as coastal land cover classification and urbanization analysis. Accordingly, the remote sensing imagery sample datasets have become crucial in ensuring robust training models with satisfactory performance across various AI(Artificial Intelligence)-enabled remote sensing applications. However, the demand for abundant and diverse RS imagery samples has surged, leading to data scarcity as a critical challenge for large-scale AI-enabled RS applications. Moreover, the inconsistencies in labeling semantic categories among sample datasets, coupled with the limits of the commonly used single-label representation of datasets, have posed a huge barrier to fully leveraging the training samples across diverse remote sensing sample datasets. Additionally, existing RS datasets are dispersed across platforms with supplier-specific data structures, making it difficult to gather suitable samples for training. To address these challenges, we propose an intelligent RS sample retrieval approach that integrates label semantics and visual features for AI-enabled RS monitoring. It measures similarity distances between samples and label category clusters to dynamically build tailored datasets, overcoming single-label limits and label disparities. Moreover, it also establishes a dynamic RS label category system that is capable of dynamically expanding distinct categories from new sample datasets through label semantic similarity mapping to resolve label inconsistencies across sample datasets. Supported by a virtual distributed storage system, it enables in-memory sample discovery and integration across clouds, leveraging multi-source RS datasets with limited interoperability. Finally, the comparative performance experiments have also confirmed the effectiveness and efficiency of this approach.
Keywords: remote sensing sample datasets, remote sensing sample retrieval, remote sensing sample database, AI-enabled remote sensing application, remote sensing imagery, deep learning, label category system, Remote sensing big data
Received: 21 Feb 2025; Accepted: 27 Mar 2025.
Copyright: © 2025 Ren, Ma and Zhou. This is an open-access article distributed under the terms of the Creative Commons Attribution License (CC BY). The use, distribution or reproduction in other forums is permitted, provided the original author(s) or licensor are credited and that the original publication in this journal is cited, in accordance with accepted academic practice. No use, distribution or reproduction is permitted which does not comply with these terms.
* Correspondence:
Yan Ma, Aerospace Information Research Institute, Chinese Academy of Sciences (CAS), Beijing, China
Disclaimer: All claims expressed in this article are solely those of the authors and do not necessarily represent those of their affiliated organizations, or those of the publisher, the editors and the reviewers. Any product that may be evaluated in this article or claim that may be made by its manufacturer is not guaranteed or endorsed by the publisher.
Research integrity at Frontiers
Learn more about the work of our research integrity team to safeguard the quality of each article we publish.