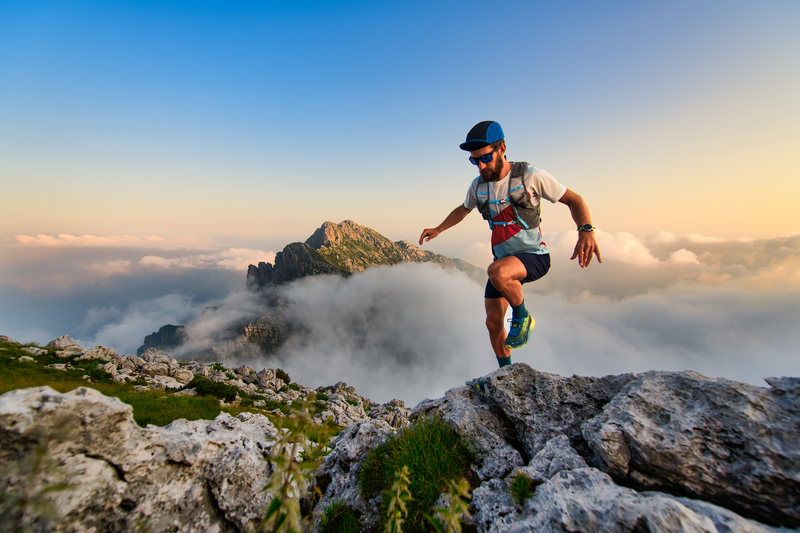
95% of researchers rate our articles as excellent or good
Learn more about the work of our research integrity team to safeguard the quality of each article we publish.
Find out more
ORIGINAL RESEARCH article
Front. Environ. Sci.
Sec. Water and Wastewater Management
Volume 13 - 2025 | doi: 10.3389/fenvs.2025.1578528
This article is part of the Research Topic Tackling the Global Water Crisis: Risks, Challenges, and Sustainable Solutions View all articles
The final, formatted version of the article will be published soon.
You have multiple emails registered with Frontiers:
Please enter your email address:
If you already have an account, please login
You don't have a Frontiers account ? You can register here
Water resources were the lifelines of the agricultural development in Xinjiang. Currently, the problem of water shortage for agriculture in this region was becoming increasingly severe. Against this backdrop, predicting the changing trends of water supply and agricultural water use in Xinjiang and analyzing the supply and use relationship between them were of great practical significance for ensuring the sustainable development of regional agriculture. To enhance prediction accuracy, this study initially evaluated and compared various methodologies, including neural networks and fractional-order models. By integrating the Fractional Grey GM(1,1) Model and the Neural Network model using a linear weighting approach, we developed a novel GM(1,1)-NN ensemble model. The validation results indicated that this proposed model demonstrated superior accuracy in forecasting water supply and agricultural water use compared to other models such as the Fractional Grey GM(1,1) model, Fractional Grey GM(1,2) model, Neural Network model, and Holt Exponential Smoothing model.Subsequently, we undertook a comprehensive analysis of the spatio-temporal trends in water supply and agricultural water use in Xinjiang over the past two decades. We then utilized the newly developed GM(1,1)-NN model to forecast and analyze local water supply and agricultural water use for the period from 2024 to 2028. Based on these findings, we formulated policy recommendations from multiple perspectives,including economic and technological considerations.The results indicated that: (1) Due to the development and utilization of water resources facilitated by China's counterpart assistance to Xinjiang, the water supply and agricultural water use in Xinjiang increased significantly by 12.7% and 15.0%, respectively, from 2011 to 2012.(2)From 2000 to 2023, the sources of water supply in various prefectures,cities of Xinjiang
Keywords: Water use, Water Supply, Model Comparison, Forecasting models, Arid area
Received: 18 Feb 2025; Accepted: 26 Mar 2025.
Copyright: © 2025 Yao, Zhang, Luo and Lin. This is an open-access article distributed under the terms of the Creative Commons Attribution License (CC BY). The use, distribution or reproduction in other forums is permitted, provided the original author(s) or licensor are credited and that the original publication in this journal is cited, in accordance with accepted academic practice. No use, distribution or reproduction is permitted which does not comply with these terms.
* Correspondence:
Chi Zhang, Shihezi University, Shihezi, China
Geping Luo, Xinjiang Institute of Ecology and Geography, Chinese Academy of Sciences (CAS), Ürümqi, 830011, Xinjiang Uyghur Region, China
Disclaimer: All claims expressed in this article are solely those of the authors and do not necessarily represent those of their affiliated organizations, or those of the publisher, the editors and the reviewers. Any product that may be evaluated in this article or claim that may be made by its manufacturer is not guaranteed or endorsed by the publisher.
Research integrity at Frontiers
Learn more about the work of our research integrity team to safeguard the quality of each article we publish.