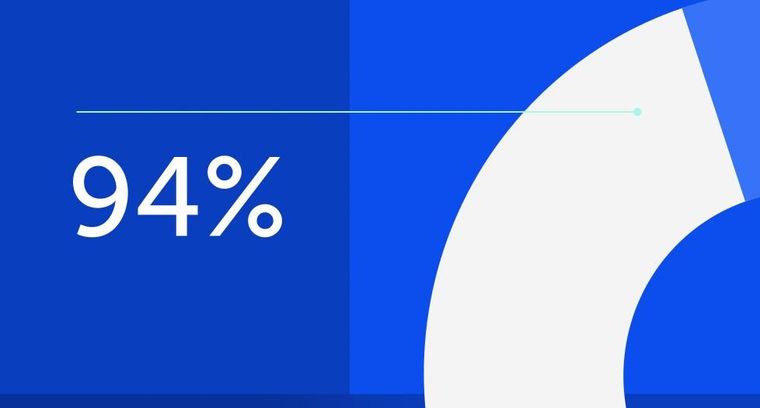
94% of researchers rate our articles as excellent or good
Learn more about the work of our research integrity team to safeguard the quality of each article we publish.
Find out more
ORIGINAL RESEARCH article
Front. Environ. Sci.
Sec. Big Data, AI, and the Environment
Volume 13 - 2025 | doi: 10.3389/fenvs.2025.1569621
This article is part of the Research TopicAdvanced Techniques For Maintenance And Reliability Management In The Oil & Gas IndustryView all articles
The final, formatted version of the article will be published soon.
Select one of your emails
You have multiple emails registered with Frontiers:
Notify me on publication
Please enter your email address:
If you already have an account, please login
You don't have a Frontiers account ? You can register here
Gas pipeline leaks contribute to one-third of methane emissions annually, posing environmental damage and safety risks. However, accurate and timely detection of the leak presents several challenges, including remote and inaccessible environments, low accuracy and efficiency, and high hardware and labor costs. To address these challenges, we propose a gas pipeline leakage detection architecture based on multiple multimodal deep feature selections and the optimized Improved Deep Forest Classifier (IDFC). First, the multimodal data, thermal images and gas sensor data, are pre-processed. Then a deep feature pool is constructed using the selected Convolutional Neural Network (CNN) models, including AlexNet, ResNet-50, MobileNet, VggNet, and EfficientNet, as well as their inner layers. Aided by the heatmaps created using Gradient-weighted Class Activation Mapping (Grad-CAM), a visualization-based primary feature selection is applied to determine the best features to form an initial CNN pool.The output of the flattened features from this CNN pool is then fed into the IDFC for classification.Hyperparameters of the base learners are then selected for an explainable and enhanced diversity tree-structured deep forest classifier, using the selected multimodal features as inputs. Finally, the Accuracy-Size Comprehensive Indicator (ASCI) is introduced for the secondary feature selection and the optimized deep forest classifier construction, which balances the model accuracy and size and reduces hardware resource requirements. Using the simulated testing dataset created for this research, our architecture demonstrated superior accuracy (98.9%) and deployability with its lower model size (115MB). This lightweight AI architecture is successfully deployed on a soft robotic system for real-time gas leak detection. Our proposed architecture is also extensible to other environmental hazard detection situations, such as liquid leaks in the pipelines.
Keywords: Gas Pipeline Leakage Detection, Multimodal modeling, deep feature selection, Explainable artificial intelligence, Tree-based Deep Learning, Accuracy Size Comprehensive Indicator, soft robotics
Received: 01 Feb 2025; Accepted: 09 Apr 2025.
Copyright: © 2025 Zhang and Zhang. This is an open-access article distributed under the terms of the Creative Commons Attribution License (CC BY). The use, distribution or reproduction in other forums is permitted, provided the original author(s) or licensor are credited and that the original publication in this journal is cited, in accordance with accepted academic practice. No use, distribution or reproduction is permitted which does not comply with these terms.
* Correspondence: Eddie Zhang, The Harker School, San Jose, United States
Disclaimer: All claims expressed in this article are solely those of the authors and do not necessarily represent those of their affiliated organizations, or those of the publisher, the editors and the reviewers. Any product that may be evaluated in this article or claim that may be made by its manufacturer is not guaranteed or endorsed by the publisher.
Supplementary Material
Research integrity at Frontiers
Learn more about the work of our research integrity team to safeguard the quality of each article we publish.