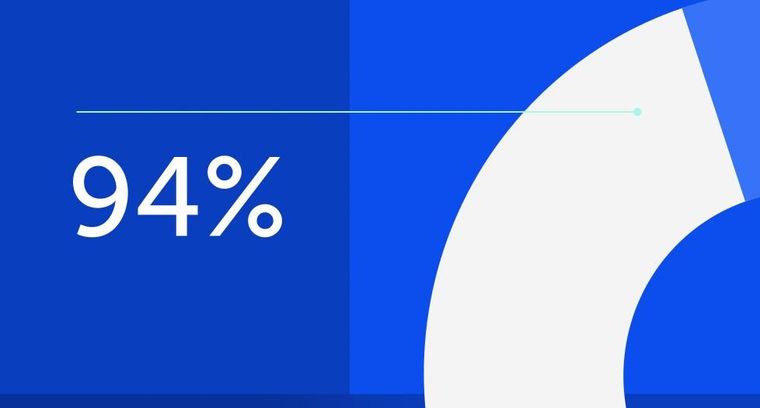
94% of researchers rate our articles as excellent or good
Learn more about the work of our research integrity team to safeguard the quality of each article we publish.
Find out more
ORIGINAL RESEARCH article
Front. Environ. Sci.
Sec. Interdisciplinary Climate Studies
Volume 13 - 2025 | doi: 10.3389/fenvs.2025.1562087
This article is part of the Research TopicInnovations in Climate Resilience, Volume IIView all articles
The final, formatted version of the article will be published soon.
Select one of your emails
You have multiple emails registered with Frontiers:
Notify me on publication
Please enter your email address:
If you already have an account, please login
You don't have a Frontiers account ? You can register here
Oil and gas reservoirs represent suitable containers to sequester carbon dioxide (CO2) in a supercritical state because they are accessible, reservoir properties are known, and they previously contained stored buoyant fluids. However, planners must quantify the relative magnitude of the CO2 storage resource in these reservoirs to formulate a comprehensive strategy for CO2 mitigation. Even reconnaissance-type estimates of CO2 storage resources of known oil and gas reservoirs may require complicated calculations involving (1) estimates of recoverable oil and gas, (2) reservoir properties (depth, temperature, pressure, etc.), and (3) the physical qualities of the retained fluids. We demonstrate the application of machine learning (ML) algorithms to bypass these computations to yield more rapid estimates of CO2 storage resources in reservoirs capable of hosting CO2 in a supercritical state. ML algorithms are computationally efficient because they do not impose the strong assumptions on the data-generating process that standard statistical or engineering procedures require. Further, ML algorithms can capture highly complex, particularly nonlinear, relationships among predictor variables. We demonstrate the application of four different ML algorithms using data from onshore and offshore oil and gas reservoirs in Europe, and show they perform well when predictions are compared to engineering estimates. The proposed methods and models provide an effective and novel way to more rapidly and directly determine the subsurface CO2 storage capacity of oil and gas reservoirs around the world, information that operators, researchers, and policy makers alike require to meet energy transition and decarbonization goals.
Keywords: machine learning, carbon dioxide storage resources, carbon dioxide sequestration, Oil and gas reservoirs, Supercritical carbon dioxide
Received: 16 Jan 2025; Accepted: 11 Apr 2025.
Copyright: © 2025 Attanasi, Freeman and Coburn. This is an open-access article distributed under the terms of the Creative Commons Attribution License (CC BY). The use, distribution or reproduction in other forums is permitted, provided the original author(s) or licensor are credited and that the original publication in this journal is cited, in accordance with accepted academic practice. No use, distribution or reproduction is permitted which does not comply with these terms.
* Correspondence: Timothy Coburn, Colorado State University, Fort Collins, United States
Disclaimer: All claims expressed in this article are solely those of the authors and do not necessarily represent those of their affiliated organizations, or those of the publisher, the editors and the reviewers. Any product that may be evaluated in this article or claim that may be made by its manufacturer is not guaranteed or endorsed by the publisher.
Supplementary Material
Research integrity at Frontiers
Learn more about the work of our research integrity team to safeguard the quality of each article we publish.