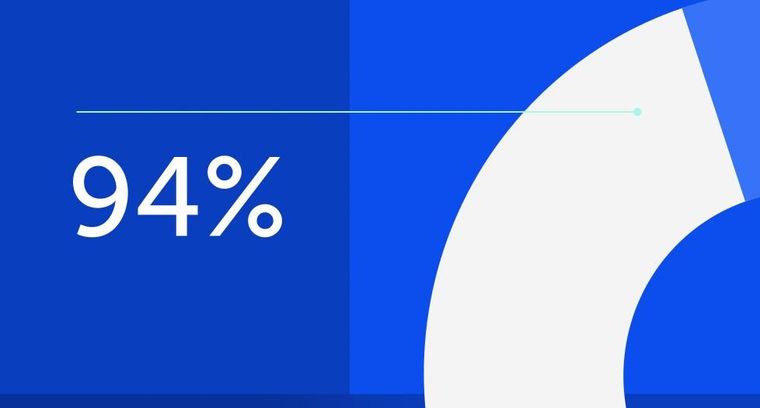
94% of researchers rate our articles as excellent or good
Learn more about the work of our research integrity team to safeguard the quality of each article we publish.
Find out more
ORIGINAL RESEARCH article
Front. Environ. Sci., 09 April 2025
Sec. Toxicology, Pollution and the Environment
Volume 13 - 2025 | https://doi.org/10.3389/fenvs.2025.1558308
This article is part of the Research TopicAdvances in Soil Pollution Research: Risk Assessment and Ecosystems ManagementView all 8 articles
In recent years, the harmful effects of heavy metal pollution in soil and rice on public health have garnered widespread attention. However, most studies focus only on the evaluation of heavy metal pollution in either soil or rice, often overlooking the fact of the combined effects of heavy metal pollution. We conducted an integrated assessment of the heavy metal pollution levels in both soil and rice. This study examined eight common heavy metals (Cd, Ni, As, Cu, Hg, Pb, Cr, and Zn) within the soil-rice system of Wanzhou District, Chongqing City. We employed Positive Matrix Factorization and Monte Carlo simulation to identify the sources of soil pollution and assess associated health risks. The findings revealed an average Impact Index of Comprehensive Quality (IICQ) value of 3.60 for the soil-rice system, indicating a pollution level exceeding “slight pollution”. The primary contributors to soil heavy metal contamination were identified as metal smelting and processing, pesticide and fertilizer use, manure application, geological background and rock weathering, agricultural activities, and coal combustion. Among the assessed metals, As, Cr, Cd, and Ni posed the greatest health risks and should be prioritized for monitoring and control. Given the heightened health risks associated with prolonged consumption of rice contaminated by heavy metals, addressing pollution in the agricultural soil-rice system is an urgent necessity.
Soil, as a critical component of ecosystems, is highly vulnerable to degradation from human activities (Han et al., 2020). Rapid urbanization and industrialization have significantly increased the release of heavy metals into the environment, making soil heavy metal accumulation a pressing global issue (Du et al., 2019; Fei et al., 2023). A 2014 survey by China’s Ministry of Environmental Protection and Ministry of Land and Resources reported exceedance rates of 16.1% for soil and 19.4% for arable land, with 82.4% of pollution attributed to metals and metalloids. Among these, the exceedance rates for cadmium (Cd), nickel (Ni), arsenic (As), copper (Cu), mercury (Hg), lead (Pb), chromium (Cr), and zinc (Zn) were 7.00%, 4.80%, 2.70%, 2.10%, 1.60%, 1.50%, 1.10%, and 0.900%, respectively (Yang Q. et al., 2018). Heavy metal pollution reduces crop yields and quality, posing significant threats to public health and food security, particularly in China, where 7.00% of the world’s arable land sustains 22.0% of the global population. Severe heavy metal contamination in China’s agricultural soil would critically endanger national food security (Qin et al., 2021). Traditional methods for assessing soil quality, such as the single-factor pollution index, Nemerow index, geo-accumulation index, and potential ecological risk index (Chen R. et al., 2022; Zhao G. et al., 2022; Shen et al., 2023), often fail to account for the combined pollution of heavy metals in soil and crops. Recently, the Impact Index of Comprehensive Quality (IICQ) has been introduced as a more effective tool for evaluating combined soil-crop system pollution (Wang et al., 2016; Gan et al., 2018). Additionally, advanced techniques, including Positive Matrix Factorization (PMF) models, and Pearson correlation analysis, have been increasingly used to identify pollution sources in with diverse contamination origins, enabling the development of targeted soil pollution control strategies areas (Hu X. et al., 2021; Han et al., 2022; Yang Z. et al., 2022; Xu et al., 2024).
As the world’s largest consumer and producer of rice, over 65.0% of Chinese households consider rice their primary dietary staple (Zhang et al., 2021). Researches have shown that long-term consumption of rice contaminated with heavy metals can significantly harm public health (Ren et al., 2021; Xu X. et al., 2022; Wang C.-C. et al., 2023). However, due to variability in heavy metal concentrations and individual differences, identifying the most hazardous heavy metal elements remains challenging. Monte Carlo simulation, a probabilistic risk analysis method, is widely employed in heavy metal health risk assessments to pinpoint the most threatening elements and facilitate targeted mitigation measures (Liu et al., 2022; Liu et al., 2023 Z.; Liang et al., 2023). Previously, more studies focused on heavy metal pollution in farmland soils or rice in Chongqing Municipality, as well as on soil - vegetable heavy metal pollution in the region (Wang et al., 2022b; Shi et al., 2024; Zhang et al., 2024). However, fewer studies have paid attention to heavy metal pollution in the soil - rice system. This study focuses on the heavy metal pollution in the soil-rice system of Wanzhou District, Chongqing City. The IICQ index, PMF model, and Monte Carlo simulation are integrated to assess the local combined heavy metal pollution levels and associated health risks to the local population, with the following objectives: (1) to analyze the extent of heavy metal pollution in the soil-rice system; (2) to apply the PMF model to identify and quantify sources of heavy metal pollution; and (3) to evaluate health risks associated with rice consumption for both adults and children. The findings aim to enhance understanding of local heavy metal pollution sources, support pollution reduction strategies, ensure food safety, and protect public health.
The study area, Wanzhou District in Chongqing City (30°23′50″–31°0′18″N, 107°52′22″–108°53′52″E), lies in northeastern Chongqing and forms part of the Three Gorges Reservoir region. Covering approximately 3,456.41 km2, it comprises 14 sub-districts, 27 towns, and 11 townships, with a population of about 1.55 million. The region experiences a subtropical monsoon climate with distinct seasons, an average annual temperature of 15°C–24°C, and average annual precipitation of 1,000–1,200 mm, with the flood season occurring from May to September. As of 2022, agricultural land in the area totaled 83,908 ha, of which paddy fields represented 30,622 ha (36.5%), while dryland accounted for 53,286 ha (63.5%). The primary soil types include purplish soil, paddy soil, and yellow soil. The research focuses on the paddy fields of Wanzhou District. Based on initial soil monitoring data, five representative towns—Lihe, Longju, Longsha, Yanshan, and Luotian—were selected for sampling. A total of 25 rice samples and their corresponding rhizosphere soil samples were collected. Figure 1 Illustrates the sampling site distribution. Rhizosphere soil samples were collected in accordance with the “Soil Environmental Monitoring Technical Specifications (HJ/T166-2004)” (The Technical Specification for soil Environmental monitoring (HJ/T 166, 2004), employing the plum-blossom grid method to uniformly sample soil at depths of 5–20 cm over a 1-m2 area. Each sample, weighing 1 kg, was retained for analysis. Simultaneously, rice samples from the same sites were collected. Following thorough mixing, the quartering method was used to subsample, retaining 500 g per sample, with detailed records maintained.
Figure 1. Distribution of sampling sites for soil and rice samples in Wanzhou District, Chongqing City.
In the laboratory, the collected soil samples were air-dried, ground, and sieved through a 100-mesh sieve. A mixed acid solution (HCl-HNO3-HF-HClO4) was added, and the samples were digested at 150°C for 48 h (Cheng et al., 2022). Rice samples were rinsed with purified water, dried, threshed, and dehulled and then ground to 40-mesh size, and digested using microwave-assisted methods to determine heavy metal concentrations. Soil pH was measured using the potentiometric method specified in NY/T 1377-2007 (Determination of pH in Soil (Ministry of Agriculture of the People's Republic of China, 2007). The Hg content in soil was measured using the catalytic thermal decomposition-cold atomic absorption spectrophotometry method (HJ 923-2017) (Soil and sediment Determination of total mercury Catalytic pyrolysis-cold atomic absorption spectrophotometry (HJ 923, 2017). Heavy metals such as Pb, Cd, Cr, As, Cu, Zn, and Ni in soil were determined using the aqua regia extraction-inductively coupled plasma mass spectrometry (ICP-MS) method (HJ 803, 2016) (Soil and sediment-Determination of aqua regia extracts of 12 metal elements-Inductively coupled plasma mass spectrometry (HJ 803, 2016). Mercury in rice was analyzed through the direct mercury measurement method (Method II of GB 5009.17-2021) (Determination of total mercury and organic mercury in foods (Ministry of Agriculture of the People's Republic of China, 2021), while Pb, Cd, Cr, As, Cu, Zn, and Ni in rice were quantified using the ICP-MS method (Method I of GB 5009.268-2016) (Determination of multiple elements in food (National Health and Family Planning Commission of the People's Republic of China and the China Food and Drug Administration, 2016).
For quality assurance (QA) and quality control (QC), personnel conducting sampling and testing underwent uniform training, all equipment was calibrated and verified, and all standard materials used were within their valid periods. Quality control measures, including laboratory blanks, parallel samples, and recovery tests, were implemented during testing. All quality control results fell within acceptable ranges.
Based on the background values, screening values of the heavy metals present in soil, and the permissible limits of heavy metal content in agricultural products, the Impact Index of Comprehensive Quality (IICQ) was proposed in 2016. This index combines soil environmental quality with the quality of agricultural products, reflecting the mutual influence of heavy metals in the soil and agricultural products on the quality of the farmland soil environment, and promotes the quantitative and comprehensive assessment of heavy metals in the soil-crop systems. This index considers the interaction between soil and crop contamination and is calculated using the Soil Impact Index (IICQs) and the Crop Impact Index (IICQap). The equations for these indices are as follows (Wang et al., 2016; Gan et al., 2019; Liu J. et al., 2023).
(1) Relative impact equivalent (RIE) in the soil:
where Pssi is the single pollution index of element i; N is the number of elements; Csoil and Csi are the content and screening value of element i, respectively; n is the oxidation number of element i (Pb, 2; Hg, 2; As, 5; Cr, 3; Cd, 2; Cu, 2; Ni, 2; Zn, 2).
(2) The degree of deviation between detected and background value (DDDB) in soil:
where Psbi is the ratio of the heavy metal measured value to its background value in soil for element i, and Cbi is the background value of element i.
(3) The degree of deviation of the soil environmental quality standard from the background value (DDSB):
where the symbols correspond to those in the aforementioned equations.
(4) The quality index of agricultural products (QIAP):
where Papi is the ratio of the heavy metal measured value to its threshold limit value of crops for element i, Capi is the measured content of element i in crops, and Cisi is the threshold limit value of element i in crops.
(5) Impact index of Comprehensive Quality (IICQ):
where IICQs and IICQap represent the impact indices for soil and agricultural products, respectively. Variables X, Y, and Z correspond to the concentrations exceeding soil threshold values, soil background values, and permissible agricultural product standards, respectively. The parameter k, typically set to 5, serves as a background correction factor. Based on IICQs, IICQap, and IICQ values, heavy metal pollution risk is classified into five grades: clean (IICQ≤1); slight pollution (1<IICQ≤2); light pollution (2<IICQ≤3); moderate pollution (3<IICQ≤5), and heavy pollution (IICQ>5).
The PMF model applies a statistical approach to process the factor profile and source contribution matrix. The standard deviation of receptor concentrations is incorporated during data input to estimate uncertainty, enhancing the reliability of the results. The calculations for these processes are as follows (Deng et al., 2020; Zhao H. et al., 2022; Jia et al., 2023; Ding et al., 2024):
where
The PMF model aimed to minimize the Q-value iteratively to achieve optimal source contribution and concentration matrices.
where
where
The health risk assessment method proposed by the United States Environmental Protection Agency (US EPA) was employed to evaluate the health risks posed by heavy metals. Recognizing that carcinogenic and non-carcinogenic effects differ in risk characterization, these were separately quantified as carcinogenic and non-carcinogenic risks. The hazard quotient (HQ) and cancer risk index (CR) were utilized to assess the potential non-carcinogenic and carcinogenic risks from heavy metals in crops, respectively. Since multiple heavy metals may exhibit synergistic effects, the total hazard quotient (THQ) and total carcinogenic risk (TCR) were calculated as the sum of the HQs and CRs for individual metals. The relevant equations are provided in references (Hu et al., 2020; Lü et al., 2022):
where Capi is the heavy metal content in crops (
An HQ or THQ value below 1.0 was considered safe, while values exceeding 1.0 indicated potential adverse health effects. For carcinogenic risks, a CR or TCR above 1.0 × 10−4 was deemed unacceptable, values below 1.0 × 10−6 indicated no significant hazard, and those between 1.0 × 10−6 and 1.0 × 10−4 were considered acceptable. To address uncertainties stemming from single-point deterministic parameters, which could lead to over- or underestimation of risks, a Monte Carlo simulation with 10,000 iterations was conducted. The probability distributions and values of the input parameters are detailed in Supplementary Table S1.
The spatial distribution maps were created using ArcGIS 10.2, while the data statistical description, correlation analysis, and principal component analysis were completed using Excel 2016 and IBM SPSS 27. Figures were generated using RStudio and Origin 2024. Source apportionment of heavy metals and uncertainty analyses were performed using EPA PMF 5.0 software, and Monte Carlo simulations were conducted with Crystal Ball software.
Statistical descriptions of heavy metal content in soil and rice samples from the study area are summarized in Table 1. Soil pH ranged from 4.70 to 7.60, with samples distributed across four pH categories: pH ≤ 5.5 (28%), 5.5 < pH ≤ 6.5 (56%), 6.5 < pH ≤ 7.5 (12%), and pH > 7.5 (4%). The average concentrations of Pb, Hg, As, Cr, Cd, Cu, Ni, and Zn in soil were 20 ± 3.61, 0.05 ± 0.06, 6.18 ± 1.66, 60 ± 9.58, 0.27 ± 0.09, 16 ± 6.48, 26 ± 4.97, and 90 ± 35 mg/kg, respectively. Except for Pb and Cr, all eight heavy metals exceeded the background values for Chongqing City. The exceedance rates were as follows: Zn (60%) > As (44%) > Cd (36%) > Hg (12%) > Ni (8%) > Cu (4%). Based on the Soil Environmental Quality Risk Control Standard for Agricultural Land (Soil environmental quality Risk control standard for soil contamination of agricultural land (Ministry of Ecology and Environment of the People's Republic of China and State Administration for Market Regulation, 2018), 12 of Cd samples exceeded the risk screening values, suggesting notable Cd enrichment in the study area. This enrichment may be attributed to both high natural background levels of Cd and the widespread use of pesticides and fertilizers in agricultural activities (Xiao et al., 2022; Alam et al., 2023). The average concentrations of Pb, Hg, As, Cr, Cd, Cu, Ni, and Zn in rice were 0.06 ± 0.02, 0.01 ± 0.01, 0.22 ± 0.16, 1.56 ± 0.70, 0.07 ± 0.08, 2.06 ± 0.78, 0.96 ± 0.41, and 16 ± 5.85 mg/kg, respectively. According to national standards, Pb, Cu, and Zn were within acceptable limits, whereas Cd, As, Cr, Ni, and Hg showed exceedance rates of 92%, 80%, 12%, 12%, and 8%, respectively (Tolerance limit of zinc in foods (Ministry of Health of the People's Republic of China, 1991; Tolerance limit of copper in foods (Ministry of Health of the People's Republic of China, 1994). The coefficients of variation, indicative of human activity impacts, were particularly high for soil Hg (104%) and rice Hg (108%), As (74%), and Cd (116%), highlighting significant spatial heterogeneity in heavy metal distribution. This heterogeneity may stem from elevated background levels and extensive pesticide and fertilizer use in the region (Kan et al., 2021; Delplace et al., 2022; Faisal et al., 2022; Yang J. et al., 2022). Spatial distribution maps of heavy metals in soil and rice samples are shown in Supplementary Figures S1, S2. Notably, Hg exhibited consistent spatial patterns in soil and rice, with hotspots concentrated in the northwest of Wanzhou District, Chongqing City, suggesting a correlation between Hg levels in soil and rice.
Table 1. Statistical distribution description of the heavy metals in soil and rice (n soil = n rice = 25, units in mg·kg−1).
The IICQ index evaluates the mutual impact of heavy metals in soil and crops on farmland environmental quality, quantifying heavy metal pollution within the soil-crop system. In the soil-rice system, the average IICQ value is 3.60, with all samples exceeding slight pollution levels. Specifically, slight, light, moderate, and heavy pollution account for 8.00%, 44.00%, 28.00%, and 20.00% of samples, respectively. The average IICQs value for soil heavy metals is 1.14, indicating the absence of heavy pollution among the samples. Clean and slightly polluted soils represent 56.00% and 28.00% of the samples, respectively, suggesting that most soils are not severely contaminated by heavy metals. Regional studies reveal varying pollution patterns. For instance, slight pollution predominates in the Shizuishan Industrial Area of Ningxia (Wang Y. et al., 2023), while the industrial towns of the Yangtze River Delta show higher proportions of moderate and heavy pollution (Yu et al., 2024). These findings underscore that heavy metal pollution in soil is significantly influenced by anthropogenic emissions and persists over long durations (Järup, 2003; Hu Y. et al., 2021). For rice, the average IICQap value is 2.46, with no instances of heavy pollution. Light and moderate pollution dominate, accounting for 68.00% and 20.00%, respectively, reflecting a notable degree of contamination. However, a study in Zhuhai indicates that although soil exhibits some pollution, the majority of rice remains free from heavy metal contamination (Liu et al., 2020). Regional differences may stem from the high natural background levels of Cd, Cr, Ni, and Zn in Southwest China (Xia et al., 2022; Jiang et al., 2023; Liu J. et al., 2023). Additionally, specific sampling points reveal significant exceedances of Hg levels, and rice shows a strong tendency to accumulate metals such as Cd, Cr, Hg, Ni, Cu, Zn, and As (Li et al., 2014; Hao et al., 2022; Zulkafflee et al., 2022; Tian et al., 2023; Wang J. et al., 2023; Yang et al., 2023). Rice contributes approximately 68.33% to the overall IICQ of the soil-rice system, making it the primary source of heavy metal exposure. However, soil still plays a substantial role, accounting for 31.67% (Supplementary Table S2). Spatial analysis reveals that IICQ and IICQs values are higher in the northwest and southwest of the study area (Figure 2), while IICQap values for rice are elevated across the region, with the southeast showing the most significant levels. It is worth noting that there is a weak spatial correlation between IICQs and IICQap, which may be related to factors such as the limited sample size, as well as the growth habits and metal enrichment characteristics of rice, leading to different spatial distributions of IICQs and IICQap. Adjusting crop varieties could mitigate heavy metal accumulation and reduce the health risks associated with consuming contaminated rice (Ali et al., 2020; Xiang et al., 2022; Wang C.-C. et al., 2023). These findings provide a valuable basis for identifying heavy metal sources in soil and assessing associated health risks.
Figure 2. Spatial Distribution of the IICQ Index in the soil-rice System of Wanzhou District, Chongqing City.
Through principal component analysis (PCA), factor selection was optimized to achieve a cumulative variance exceeding 86.19%. The analysis considered 4–8 factors, balancing the need to avoid an overly complex or overly simplistic model. Ultimately, 4, 5, 6, and 7 factors were tested. Using the PMF model to analyze soil heavy metal sources, the best fit occurred with five factors, as indicated by a strong determination coefficient (R2 > 0.7) between observed and predicted values for eight heavy metals (Figure 3a; Supplementary Figure S3). This confirms the PMF model’s high accuracy and reliability for source identification.
Figure 3. Identification of heavy metal sources in soil; (a) Source contributions for the five factors; (b) Variance contributions for the five factors.
Factor 1 was predominantly influenced by Cr (67.38%) and Ni (30.18%). The study shows that the average levels of Cr and Ni in the soil are lower than the background values in Chongqing. The correlation analysis reveals a high correlation between Cr and Ni (Supplementary Table S3). This is consistent with the fact that, during soil formation, the concentrations of Cr and Ni do not change significantly and are closely related to the soil parent material (Li F. et al., 2022; Li et al., 2023 J.; Wang et al., 2025). Therefore, Factor 1 is considered a natural source associated with the soil parent material.
Factor 2 was characterized by significant contributions from Cu (30.00%) and Zn (38.96%). The accumulation of these metals is largely attributed to livestock manure, particularly pig manure (Li N. et al., 2022). In the study area, manure application is a common agricultural practice, potentially explaining the elevated levels of copper and zinc in the soil. Additionally, widespread use of Cu- and Zn-containing pesticides and fertilizers to enhance rice yields further exacerbates the risk of soil contamination (Guan et al., 2018). Therefore, Factor 2 is associated with the use of pesticides, fertilizers, and livestock manure.
Factor 3 was dominated by Cd, with a contribution rate of 47.42%. Approximately 36% of soil samples exceeded Chongqing’s background values for Cd, which itself is higher than the national soil background values. This enrichment is attributed to condensation processes during weathering and soil formation in carbonate rock regions of southwestern China, leading to significant Cd accumulation in the soil (Chen Z. et al., 2022; Xia et al., 2022; Zhang Q. et al., 2022). Consequently, Factor 3 is considered a natural source tied to rock weathering.
Factor 4 exhibited a complex composition of heavy metals, including Pb (37.52%), Cd (38.66%), As (58.09%), Cr (30.18%), Cu (36.35%), Zn (35.99%), and Ni (35.44%). Research indicates that soil contamination by Cu, Zn, Cd, As, and Cr is closely linked to the application of livestock manure, organic fertilizers, pesticides, and chemical fertilizers in agriculture (Guo et al., 2022; Jiao et al., 2022; Wang S. et al., 2022; Yang J. et al., 2022; Ma et al., 2023; Xu et al., 2023). In 2019, Chongqing consumed 9.11 × 105 tons of chemical fertilizers, and although usage has declined, excessive fertilization remains an issue (Liang et al., 2024). Additionally, livestock manure continues to be widely used in some regions (Chen et al., 2019). From 2003 to 2012, pesticide consumption in the Three Gorges Reservoir area increased annually, peaking at 7.20 kg/hm2 by 2011 (Zhang et al., 2016). Pb contamination is largely attributed to traffic emissions, including fuel combustion, engine wear, braking, and tire wear (Long et al., 2021; Peng et al., 2022). The use of agricultural machinery further heightens the risk of Pb pollution. The region’s complex topography—characterized by hills, mountains, sloping land, and low average wind speeds—promotes atmospheric stability, reducing pollutant dispersion and intensifying Pb accumulation in the soil. Soil Ni primarily originates from fossil fuel combustion and vehicle exhaust (El-Naggar et al., 2021). Thus, Factor 4 is linked to agricultural activities, including the use of chemical fertilizers, pesticides, and transportation-related emissions.
Factor 5 accounted for 63.05% of the total contribution to Hg pollution. The coefficient of variation for Hg in soil samples was 103.92%, highlighting an uneven distribution that points to human activities as the primary source of Hg contamination (Baltas et al., 2020). Previous studies indicate that coal combustion contributes approximately 40% of the external Hg input to agricultural soils and more than 70% of atmospheric Hg deposition (Peng et al., 2019). Elemental mercury (Hg0), gaseous oxidized mercury (Hg2+), and particulate-bound mercury (HgP) released during coal combustion accumulate in nearby or distant soils via dry and wet deposition processes (Lv, 2019). Despite a decline in total coal consumption in Chongqing in recent years, coal remains the dominant energy source, accounting for 57.68% of total energy use (Yang S. et al., 2018). Hg emitted during coal combustion ultimately settles in soils through atmospheric deposition, linking Factor 5 to coal combustion.
The primary sources of heavy metals in the research area’s soils include natural sources from soil parent material (16.5%), pesticides and fertilizers (12.86%), natural sources from rock weathering (23.19%), agricultural activities (34.03%), and coal combustion (13.41%) (Figure 3b; Supplementary Figure S4). The PMF model effectively quantified the contribution of these sources while enhancing the precision of identifying heavy metal origins in the rice-soil system, despite inherent uncertainties (Anaman et al., 2022).
Correlation analysis of the soil-rice system (Figure 4; Supplementary Table S3) revealed several key patterns. Soil pH exhibited a significant positive correlation with Cu and Zn concentrations in the soil, while Pb showed a significant positive correlation with Cd, As, Cr, Ni, and Hg. As in the soil correlated positively with Cr and Ni, and Cu showed a strong association with Zn. Cr and Ni were also significantly correlated, reflecting the homogeneity of pollution sources, such as the associations of Cr with Ni, and Cu with Zn, Pb, Cd, and As (Liao et al., 2019; Xu L. et al., 2022; Yang X. et al., 2022; Zhang H. et al., 2022; Li Q. et al., 2023). In rice, soil pH correlated positively with Cu absorption and negatively with Hg uptake, confirming the influence of pH on the bioavailability of these metals, as reported in prior studies (Liu et al., 2015; Wang et al., 2022c). Additionally, As in rice correlated significantly with Pb and As in the soil, consistent with previous findings (Mu et al., 2019). This indicates that soil pH indirectly affects the bioavailability of heavy metals, thus influencing rice uptake of these elements. The mechanism by which Pb in the soil affects As in rice is not clear. It may be related to soil pH, redox conditions, organic matter content, and the competitive or synergistic interactions among various metals. Nevertheless, further experimental research is needed to verify this. Under flooded conditions, As(V) in the soil is more likely to be reduced to As(III), which has higher bioavailability and is more readily absorbed by rice roots.
Figure 4. Correlation matrix of eight heavy metals of soil and crop in the soil-rice systems in Wanzhou District, Chongqing City. S represents soil, and C represents rice.
For non-carcinogenic risks, the average HQ values ranked as follows: As > Zn > Cd > Pb > Hg > Cr > Cu > Ni for both children and adults (Figures 5a–h). The average HQ values for As were 1.021 in adults and 0.761 in children, with 37.49% and 22.61% of rice samples exceeding the safety threshold of 1.0, respectively (Figure 5c). HQ values for other heavy metals generally fell below 1.0, except for occasional exceedances for Cd and Cr. The average total hazard quotient (THQ) was 1.222 (90% CI: 0.407–2.772) for adults and 0.902 (90% CI: 0.304–2.079) for children, highlighting potential health risks (Supplementary Table S4). The exceedance rates were 49.82% for adults and 30.12% for children (Figure 5i). Non-carcinogenic risks in adults were 1.35 times higher than in children, exceeding the guideline threshold of 1.0. This highlights the potential health risks associated with rice consumption, particularly for adults. A study in Fujian’s Minxi region reported THQ values of 4.150 for adults and 2.434 for children, both indicating significant non-carcinogenic risks (Lü et al., 2022). Conversely, research in Iran and Thailand found no such risks (Djahed et al., 2018; Kukusamude et al., 2021), possibly due to variations in sample size and regional heavy metal pollution. As was the primary contributor to non-carcinogenic risk, accounting for 83.55% and 84.37% of THQ for adults and children, respectively. These findings align with previous studies emphasizing the significant hazard posed by As (Baruah et al., 2021; Lü et al., 2021).
Figure 5. Probability distribution of the health risk index of non-carcinogenic heavy metals in crops. Hazard quotient (HQ) for (a) Pb, (b) Hg, (c) As, (d) Cr, (e) Cd, (f) Cu, (g) Ni, (h) Zn, and index for (i) total hazard quotient (THQ). (The red and blue dashed lines indicate the mean values, whereas black indicates the guideline value for non-carcinogenic risk [1.0]).
For carcinogenic risks, the average CR values followed the sequence Ni > Cr > Cd > As > Pb for both adults and children (Figures 6a–f). Except for Pb, the CR values of these heavy metals exceeded the unacceptable threshold of 1.0 × 10−4. Specifically, As contributed 57.61% for adults and 42.47% for children; Cr, 93.84% for adults and 88.59% for children; Cd, 58.17% for adults and 45.83% for children; and Ni, 94.49% for adults and 89.46% for children. Pb values, however, remained within acceptable limits (Figure 6a). The average TCR was 1.09 × 10−3 (90% CI: 5.18 × 10−4 to 1.86 × 10−3) for adults and 8.06 × 10−4 (90% CI: 3.81 × 10−4 to 1.40 × 10−3) for children, with nearly all TCR values surpassing the 1.0 × 10−4 threshold (Supplementary Table S4). Similar patterns were observed in studies from India and China (Baruah et al., 2021; Zhao et al., 2024), underscoring the significant carcinogenic risks posed by heavy metals. As, Cr, Cd, and Ni emerged as the most hazardous elements, consistent with findings from a health risk assessment of rice in Hunan, China (Zeng et al., 2015). Adults faced higher overall risks, likely due to greater rice consumption and prolonged exposure (Li et al., 2018; Tang et al., 2019; Singh et al., 2023). Nonetheless, children may face heightened vulnerability because of lower body weight and reduced tolerance to toxic metals (Cai et al., 2019; Wan et al., 2022; Wang and Cheng, 2023).
Figure 6. Probability distribution of the health risk index of carcinogenic heavy metals in crops. Carcinogenic risk (CR) for (a), (b), (c), (d), and (e), and index for (f) total carcinogenic risk (TCR). (The red and blue dashed lines indicate the mean values, whereas black indicates the guideline value for carcinogenic risk (10−4). The green dashed lines present the acceptable carcinogenic risk value that of 10−6).
Sensitivity analysis identified the concentration (C) of heavy metals in rice as the most influential factor in health risk assessment for both adults and children (Supplementary Tables S5, S6). Exposure frequency significantly impacted HQ, contributing 19.8% of its variance in adults and 19.6% in children. The oral reference dose (RfD) showed a strong negative correlation with non-carcinogenic risk, whereas body weight exhibited weaker correlations—positive for adults and negative for children. For carcinogenic risks, the cancer slope factor contributed 57.7% and 57.8% of CR variance in adults and children, respectively, with exposure frequency accounting for 20.0% in adults and 20.9% in children. Body weight correlations with carcinogenic risks followed the same pattern as with non-carcinogenic risks but remained weak. Among the heavy metals, As contributed most to total non-carcinogenic risk, at 87.77% for adults and 87.44% for children. In contrast, Ni, Cr, and Cd collectively outweighed As in TCR, with Ni contributing 33.71% for adults and 35.47% for children, Cr at 35.07% for adults and 36.05% for children, and Cd at 36.11% for adults and 36.66% for children (Figure 7). As, Ni, Cr, and Cd were significant contributors to the total health risk associated with heavy metal exposure. Health risk assessments for heavy metals using Monte Carlo simulations involve considerable uncertainties. Regional, ethnic, and age-related differences often result in variations in model parameters, such as exposure frequency and body weight, leading to discrepancies in actual health risk levels (Jalali and Karimi Mojahed, 2020; Huang et al., 2021; Orosun, 2021). Sensitivity analysis, when integrated with Monte Carlo simulation, can identify critical exposure parameters and effectively evaluate health risks in diverse population groups. Notably, rice intake emerged as the most sensitive parameter in both non-carcinogenic and carcinogenic risk assessments, aligning with prior studies on rice heavy metal pollution in the Minxi region and vegetable heavy metal contamination in Chongqing (Lü et al., 2022; Shi et al., 2024).
Figure 7. Sensitivity analyses of heavy metals for (a) total hazard quotient and (b) total carcinogenic risk.
This study comprehensively assessed heavy metal pollution in the soil-rice system of Wanzhou District, Chongqing City. The study shows that in the soil - rice system of Wanzhou District, Chongqing, the average IICQ value is 3.60, with 92% of samples showing moderate or severe pollution. This indicates that the system is predominantly characterized by moderate pollution. Heavy metals in the soil were influenced by multiple sources, including natural sources from soil parent material, the use of pesticides, fertilizers, and manure, natural sources from rock weathering, agricultural activities related to fertilizers and pesticides, and coal combustion. For non - carcinogenic risks, the average total hazard quotient (THQ) is 1.222 for adults and 0.902 for children. This indicates that adults face 1.35 times the non - carcinogenic risk compared to children. Therefore, rice consumption may pose higher health risks to adults than to children. For carcinogenic risks, the average total carcinogenic risk (TCR) is 1.09 × 10−3 for adults and 8.06 × 10−4 for children. Both values exceed the threshold of 1.0 × 10−4, highlighting the high carcinogenic potential of heavy metals. As, Ni, Cr, and Cd are identified as posing significant health risks to both adults and children. Long-term consumption of rice grown in the region may present considerable health risks. To mitigate exposure, transitioning to alternative crop varieties is recommended. These findings provide critical insights into the agricultural environment’s quality and serve as a valuable reference for improving heavy metal pollution management, remediation, and control strategies.
The raw data supporting the conclusions of this article will be made available by the authors, without undue reservation.
LT: Formal Analysis, Funding acquisition, Methodology, Visualization, Writing – original draft. AY: Data curation, Formal Analysis, Software, Writing – original draft. MF: Data curation, Formal Analysis, Writing – original draft. DW: Methodology, Supervision, Writing – original draft. YL: Data curation, Investigation, Writing – original draft. XH: Investigation, Supervision, Writing – original draft. LX: Formal Analysis, Investigation, Resources, Writing – original draft. RF: Formal Analysis, Investigation, Resources, Writing – original draft. LC: Funding acquisition, Investigation, Writing – review and editing.
The author(s) declare that financial support was received for the research and/or publication of this article. Chongqing Municipal Education Commission Science and Technology Research Project (Grant No. KJQN202202709) should be revised to: the Science and Technology Research Program of Chongqing Municipal Education Commission (Grant No. KJQN202202709), Chongqing Municipal Public Health Key Special (Academic) Discipline Construction Fund should be revised to: Chongqing public health key specialty (discipline) project.
The authors declare that the research was conducted in the absence of any commercial or financial relationships that could be construed as a potential conflict of interest.
The author(s) declare that no Generative AI was used in the creation of this manuscript.
All claims expressed in this article are solely those of the authors and do not necessarily represent those of their affiliated organizations, or those of the publisher, the editors and the reviewers. Any product that may be evaluated in this article, or claim that may be made by its manufacturer, is not guaranteed or endorsed by the publisher.
The Supplementary Material for this article can be found online at: https://www.frontiersin.org/articles/10.3389/fenvs.2025.1558308/full#supplementary-material
Alam, M. N. E., Hosen, M. M., Ullah, A. K. M. A., Maksud, M. A., Khan, S. R., Lutfa, L. N., et al. (2023). Pollution characteristics, source identification, and health risk of heavy metals in the soil-vegetable system in two districts of Bangladesh. Biol. Trace Elem. Res. 201, 4985–4999. doi:10.1007/s12011-023-03558-7
Ali, W., Mao, K., Zhang, H., Junaid, M., Xu, N., Rasool, A., et al. (2020). Comprehensive review of the basic chemical behaviours, sources, processes, and endpoints of trace element contamination in paddy soil-rice systems in rice-growing countries. J. Hazard. Mater. 397, 122720. doi:10.1016/j.jhazmat.2020.122720
Anaman, R., Peng, C., Jiang, Z., Liu, X., Zhou, Z., Guo, Z., et al. (2022). Identifying sources and transport routes of heavy metals in soil with different land uses around a smelting site by GIS based PCA and PMF. Sci. Total Environ. 823, 153759. doi:10.1016/j.scitotenv.2022.153759
Baltas, H., Sirin, M., Gökbayrak, E., and Ozcelik, A. E. (2020). A case study on pollution and a human health risk assessment of heavy metals in agricultural soils around Sinop province, Turkey. Chemosphere 241, 125015. doi:10.1016/j.chemosphere.2019.125015
Baruah, S. G., Ahmed, I., Das, B., Ingtipi, B., Boruah, H., Gupta, S. K., et al. (2021). Heavy metal(loid)s contamination and health risk assessment of soil-rice system in rural and peri-urban areas of lower brahmaputra valley, northeast India. Chemosphere 266, 129150. doi:10.1016/j.chemosphere.2020.129150
Cai, L.-M., Wang, Q.-S., Luo, J., Chen, L.-G., Zhu, R.-L., Wang, S., et al. (2019). Heavy metal contamination and health risk assessment for children near a large Cu-smelter in central China. Sci. Total Environ. 650, 725–733. doi:10.1016/j.scitotenv.2018.09.081
Chen, C., Cheng, H., Jia, J., Wang, X., and Zhao, J. (2019). Use it or not: an agro-ecological perspective to flooded riparian land along the Three Gorges Reservoir. Sci. Total Environ. 650, 1062–1072. doi:10.1016/j.scitotenv.2018.09.062
Chen, R., Han, L., Liu, Z., Zhao, Y., Li, R., Xia, L., et al. (2022a). Assessment of soil-heavy metal pollution and the health risks in a mining area from southern shaanxi province, China. Toxics 10, 385. doi:10.3390/toxics10070385
Chen, Z., Xu, J., Duan, R., Lu, S., Hou, Z., Yang, F., et al. (2022b). Ecological health risk assessment and source identification of heavy metals in surface soil based on a high geochemical background: a case study in Southwest China. Toxics 10, 282. doi:10.3390/toxics10060282
Cheng, J. W., Cai, S. W., Huang, M. Q., and Yan, X. (2022). Heavy metal speciation migrationtransformation and risk assessement in plant rhizosphere soil of Zunyi manganese mineland, Guizhou. Environ. Chem. 41, 2833–2841. doi:10.7524/j.issn.0254-6108.2021050606
Delplace, G., Viers, J., Schreck, E., Oliva, P., and Behra, P. (2022). Pedo-geochemical background and sediment contamination of metal(loid)s in the old mining-district of Salsigne (Orbiel valley, France). Chemosphere 287, 132111. doi:10.1016/j.chemosphere.2021.132111
Deng, M., Zhu, Y., Shao, K., Zhang, Q., Ye, G., and Shen, J. (2020). Metals source apportionment in farmland soil and the prediction of metal transfer in the soil-rice-human chain. J. Environ. Manag. 260, 110092. doi:10.1016/j.jenvman.2020.110092
Ding, H., Liu, J., Liu, Q., Guo, L., Hang, Q., Zhang, Y., et al. (2024). Risk assessment and source tracing of heavy metals in major rice-producing provinces of Yangtze River Basin. J. Hazard. Mater. 480, 136206. doi:10.1016/j.jhazmat.2024.136206
Djahed, B., Taghavi, M., Farzadkia, M., Norzaee, S., and Miri, M. (2018). Stochastic exposure and health risk assessment of rice contamination to the heavy metals in the market of Iranshahr, Iran. Food Chem. Toxicol. 115, 405–412. doi:10.1016/j.fct.2018.03.040
Du, Y., Chen, L., Ding, P., Liu, L., He, Q., Chen, B., et al. (2019). Different exposure profile of heavy metal and health risk between residents near a Pb-Zn mine and a Mn mine in Huayuan county, South China. Chemosphere 216, 352–364. doi:10.1016/j.chemosphere.2018.10.142
El-Naggar, A., Ahmed, N., Mosa, A., Niazi, N. K., Yousaf, B., Sharma, A., et al. (2021). Nickel in soil and water: sources, biogeochemistry, and remediation using biochar. J. Hazard. Mater. 419, 126421. doi:10.1016/j.jhazmat.2021.126421
Faisal, M., Wu, Z., Wang, H., Hussain, Z., Azam, M. I., and Muzammil, M. (2022). Assessment and source apportionment of water-soluble heavy metals in road dust of Zhengzhou, China. Environ. Sci. Pollut. Res. 29, 68857–68869. doi:10.1007/s11356-022-20666-4
Fei, X., Lou, Z., Lv, X., Ren, Z., and Xiao, R. (2023). Pollution threshold assessment and risk area delineation of heavy metals in soils through the finite mixture distribution model and Bayesian maximum entropy theory. J. Hazard. Mater. 452, 131231. doi:10.1016/j.jhazmat.2023.131231
Gan, Y., Huang, X., Li, S., Liu, N., Li, Y. C., Freidenreich, A., et al. (2019). Source quantification and potential risk of mercury, cadmium, arsenic, lead, and chromium in farmland soils of Yellow River Delta. J. Clean. Prod. 221, 98–107. doi:10.1016/j.jclepro.2019.02.157
Gan, Y., Miao, Y., Wang, L., Yang, G., Li, Y. C., Wang, W., et al. (2018). Source contribution analysis and collaborative assessment of heavy metals in vegetable-growing soils. J. Agric. Food Chem. 66, 10943–10951. doi:10.1021/acs.jafc.8b04032
Guan, Q., Wang, F., Xu, C., Pan, N., Lin, J., Zhao, R., et al. (2018). Source apportionment of heavy metals in agricultural soil based on PMF: a case study in Hexi Corridor, northwest China. Chemosphere 193, 189–197. doi:10.1016/j.chemosphere.2017.10.151
Guo, X., Li, S., Zhang, Y., Wu, B., and Guo, W. (2022). Applications of dynamic simulation for source analysis of soil pollutants based on atmospheric diffusion and deposition model. Sci. Total Environ. 839, 156057. doi:10.1016/j.scitotenv.2022.156057
Han, J., Liang, L., Zhu, Y., Xu, X., Wang, L., Shang, L., et al. (2022). Heavy metal(loid)s in farmland soils on the Karst Plateau, Southwest China: an integrated analysis of geochemical baselines, source apportionment, and associated health risk. Land Degrad. Dev. 33, 1689–1703. doi:10.1002/ldr.4257
Han, L., Li, J., Xue, Q., Chen, Z., Zhou, Y., and Poon, C. S. (2020). Bacterial-induced mineralization (BIM) for soil solidification and heavy metal stabilization: a critical review. Sci. Total Environ. 746, 140967. doi:10.1016/j.scitotenv.2020.140967
Hao, H., Ge, D., Wen, Y., Lv, Y., and Chen, W. (2022). Probabilistic health risk assessment of inorganic arsenic and some heavy metals in rice produced from a typical multi-mining county, China. Environ. Sci. Pollut. Res. 29, 11510–11523. doi:10.1007/s11356-021-16583-7
HJ 803 (2016). Soil and sediment-Determination of aqua regia extracts of 12 metal elements-Inductively coupled plasma mass spectrometry. Available online at: https://english.mee.gov.cn/Resources/standards/Soil/Method_Standard4/201607/t20160704_357088.shtml (Accessed January 08, 2016).
HJ 923 (2017). Soil and sediment Determination of total mercury Catalytic pyrolysis-cold atomic absorption spectrophotometry. Available online at: https://english.mee.gov.cn/Resources/standards/Soil/Method_Standard4/201801/t20180126_430455.shtml (Accessed January 04, 2018).
HJ/T 166 (2004). The technical specification for soil environmental monitoring. Available online at: https://english.mee.gov.cn/Resources/standards/Soil/Method_Standard4/200710/t20071024_111895.shtml (Accessed December 09, 2016).
Hu, B., Shao, S., Fu, T., Fu, Z., Zhou, Y., Li, Y., et al. (2020). Composite assessment of human health risk from potentially toxic elements through multiple exposure routes: a case study in farmland in an important industrial city in East China. J. Geochem. Explor. 210, 106443. doi:10.1016/j.gexplo.2019.106443
Hu, X., Wang, J., Lv, Y., Liu, X., Zhong, J., Cui, X., et al. (2021a). Effects of heavy metals/metalloids and soil properties on microbial communities in farmland in the vicinity of a metals smelter. Front. Microbiol. 12, 707786. doi:10.3389/fmicb.2021.707786
Hu, Y., Wang, C., Song, Z., Chen, M., Ding, L., Liang, X., et al. (2021b). Heavy metal in rice and vegetable and human exposure near a large Pb/Zn smelter in Central China. IJERPH 18, 12631. doi:10.3390/ijerph182312631
Huang, J., Wu, Y., Sun, J., Li, X., Geng, X., Zhao, M., et al. (2021). Health risk assessment of heavy metal(loid)s in park soils of the largest megacity in China by using Monte Carlo simulation coupled with Positive matrix factorization model. J. Hazard. Mater. 415, 125629. doi:10.1016/j.jhazmat.2021.125629
Jalali, M., and Karimi Mojahed, J. (2020). Assessment of the health risks of heavy metals in soils and vegetables from greenhouse production systems in Iran. Int. J. Phytoremediation 22, 834–848. doi:10.1080/15226514.2020.1715917
Järup, L. (2003). Hazards of heavy metal contamination. Br. Med. Bull. 68, 167–182. doi:10.1093/bmb/ldg032
Jia, J., Xiao, B., Yu, Y., Zou, Y., Yu, T., Jin, S., et al. (2023). Heavy metal levels in the soil near typical coal-fired power plants: partition source apportionment and associated health risks based on PMF and HHRA. Environ. Monit. Assess. 195, 207. doi:10.1007/s10661-022-10823-0
Jiang, Y.-L., Yu, J., Wang, R., Wang, J.-B., Li, Y., Yu, F., et al. (2023). Source analysis and pollution assessment of soil heavy metals in typical geological high background area in southeastern chongqing. Huan Jing Ke Xue 44, 4017–4026. doi:10.13227/j.hjkx.202207074
Jiao, W., Niu, Y., Zhou, J., Zhang, H., Song, H., and Li, K. (2022). Source apportionment of bioavailable trace metals in soil based on chemical fractionation and its environmental implications. Environ. Sci. Pollut. Res. 29, 17062–17071. doi:10.1007/s11356-021-16987-5
Kan, X., Dong, Y., Feng, L., Zhou, M., and Hou, H. (2021). Contamination and health risk assessment of heavy metals in China’s lead–zinc mine tailings: a meta–analysis. Chemosphere 267, 128909. doi:10.1016/j.chemosphere.2020.128909
Kukusamude, C., Sricharoen, P., Limchoowong, N., and Kongsri, S. (2021). Heavy metals and probabilistic risk assessment via rice consumption in Thailand. Food Chem. 334, 127402. doi:10.1016/j.foodchem.2020.127402
Li, F., Yang, H., Ayyamperumal, R., and Liu, Y. (2022a). Pollution, sources, and human health risk assessment of heavy metals in urban areas around industrialization and urbanization-Northwest China. Chemosphere 308, 136396. doi:10.1016/j.chemosphere.2022.136396
Li, J., Li, K.-M., Jiao, L., Zang, F., Li, X., Yang, Y.-Q., et al. (2023a). Contamination, ecological-health risks, and sources of potentially toxic elements in road-dust sediments and soils of the largest urban riverfront scenic park in China. Environ. Geochem Health 45, 8169–8186. doi:10.1007/s10653-023-01715-4
Li, N., Chen, J., Liu, C., Yang, J., Zhu, C., and Li, H. (2022b). Cu and Zn exert a greater influence on antibiotic resistance and its transfer than doxycycline in agricultural soils. J. Hazard. Mater. 423, 127042. doi:10.1016/j.jhazmat.2021.127042
Li, Q., Li, X., Bu, C., and Wu, P. (2023b). Distribution, risk assessment, and source apportionment of heavy metal pollution in cultivated soil of a typical mining area in Southwest China. Enviro Toxic Chem. 42, 888–900. doi:10.1002/etc.5586
Li, W., Xu, B., Song, Q., Liu, X., Xu, J., and Brookes, P. C. (2014). The identification of ‘hotspots’ of heavy metal pollution in soil–rice systems at a regional scale in eastern China. Sci. Total Environ. 472, 407–420. doi:10.1016/j.scitotenv.2013.11.046
Li, X., Li, Z., Lin, C.-J., Bi, X., Liu, J., Feng, X., et al. (2018). Health risks of heavy metal exposure through vegetable consumption near a large-scale Pb/Zn smelter in central China. Ecotoxicol. Environ. Saf. 161, 99–110. doi:10.1016/j.ecoenv.2018.05.080
Liang, L., Zhu, Y., Xu, X., Hao, W., Han, J., Chen, Z., et al. (2023). Integrated insights into source apportionment and source-specific health risks of potential pollutants in urban park soils on the karst plateau, SW China. Expo. Health 15, 933–950. doi:10.1007/s12403-023-00534-3
Liang, T., Zhao, J.-K., Li, H.-M., Wang, Y., Cao, Z.-H., Zhang, W.-S., et al. (2024). Analysis on driving factors, reduction potential, and environmental effect of inorganic fertilizer input in chongqing. Huan Jing Ke Xue 45, 364–375. doi:10.13227/j.hjkx.202211280
Liao, Z., Chen, Y., Ma, J., Islam, Md. S., Weng, L., and Li, Y. (2019). Cd, Cu, and Zn accumulations caused by long-term fertilization in greenhouse soils and their potential risk assessment. IJERPH 16, 2805. doi:10.3390/ijerph16152805
Liu, J., Li, X., Zhang, P., Zhu, Q., Lu, W., Yang, Y., et al. (2023a). Contamination levels of and potential risks from metal(loid)s in soil-crop systems in high geological background areas. Sci. Total Environ. 881, 163405. doi:10.1016/j.scitotenv.2023.163405
Liu, L., Xu, X., Han, J., Zhu, J.-M., Li, S., Liang, L., et al. (2022). Heavy metal(loid)s in agricultural soils in the world’s largest barium-mining area: pollution characteristics, source apportionment, and health risks using PMF model and Cd isotopes. Process Saf. Environ. Prot. 166, 669–681. doi:10.1016/j.psep.2022.08.061
Liu, S., Zhao, H., Wu, K., Zhang, Z., Hou, Y., Chen, T., et al. (2020). Evaluation of heavy metal distribution characteristics of agricultural soil-rice system in a high geological background area according to the influence index of comprehensive quality (IICQ). Environ. Sci. Pollut. Res. 27, 20920–20933. doi:10.1007/s11356-020-08453-5
Liu, Z., Du, Q., Guan, Q., Luo, H., Shan, Y., and Shao, W. (2023b). A Monte Carlo simulation-based health risk assessment of heavy metals in soils of an oasis agricultural region in northwest China. Sci. Total Environ. 857, 159543. doi:10.1016/j.scitotenv.2022.159543
Liu, Z., Zhang, Q., Han, T., Ding, Y., Sun, J., Wang, F., et al. (2015). Heavy metal pollution in a soil-rice system in the Yangtze River region of China. IJERPH 13, 63. doi:10.3390/ijerph13010063
Long, Z., Zhu, H., Bing, H., Tian, X., Wang, Z., Wang, X., et al. (2021). Contamination, sources and health risk of heavy metals in soil and dust from different functional areas in an industrial city of Panzhihua City, Southwest China. J. Hazard. Mater. 420, 126638. doi:10.1016/j.jhazmat.2021.126638
Lü, Q., Xiao, Q., Guo, Y., Wang, Y., Cai, L., You, W., et al. (2022). Pollution monitoring, risk assessment and target remediation of heavy metals in rice from a five-year investigation in Western Fujian region, China. J. Hazard. Mater. 424, 127551. doi:10.1016/j.jhazmat.2021.127551
Lü, Q., Xiao, Q., Wang, Y., Wen, H., Han, B., Zheng, X., et al. (2021). Risk assessment and hotspots identification of heavy metals in rice: a case study in Longyan of Fujian province, China. Chemosphere 270, 128626. doi:10.1016/j.chemosphere.2020.128626
Lv, J. (2019). Multivariate receptor models and robust geostatistics to estimate source apportionment of heavy metals in soils. Environ. Pollut. 244, 72–83. doi:10.1016/j.envpol.2018.09.147
Ma, J., Lanwang, K., Liao, S., Zhong, B., Chen, Z., Ye, Z., et al. (2023). Source apportionment and model applicability of heavy metal pollution in farmland soil based on three receptor models. Toxics 11, 265. doi:10.3390/toxics11030265
Ministry of Health of the People's Republic of China. Tolerance limit of zinc in foods, GB 13106-1991,1991-06-07.
Ministry of Health of the People's Republic of China. Tolerance limit of copper in foods, GB 15199-1994, 1994-08-10.
Ministry of Ecology and Environment of the People's Republic of China and State Administration for Market Regulation. Soil environmental quality Risk control standard for soil contamination of agricultural land, GB 15618—2018, 2018-06-22.
Ministry of Agriculture of the People's Republic of China. Determination of pH in soil, NY/T 1377-2007, 2007-06-14.
National Health and Family Planning Commission of the People's Republic of China and the China Food and Drug Administration. Determination of multiple elements in food, GB 5009.268-2016, 2016-12-23. 5009.17–2021.
Mu, T., Wu, T., Zhou, T., Li, Z., Ouyang, Y., Jiang, J., et al. (2019). Geographical variation in arsenic, cadmium, and lead of soils and rice in the major rice producing regions of China. Sci. Total Environ. 677, 373–381. doi:10.1016/j.scitotenv.2019.04.337
National Health Commission of the People's Republic of China and State Administration for Market Regulation. Determination of total mercury and organic mercury in foods, GB 5009.17-2021, 2021-09-07.
Orosun, M. M. (2021). Assessment of arsenic and its associated health risks due to mining activities in parts of North-central Nigeria: probabilistic approach using Monte Carlo. J. Hazard. Mater. 412, 125262. doi:10.1016/j.jhazmat.2021.125262
Peng, H., Chen, Y., Weng, L., Ma, J., Ma, Y., Li, Y., et al. (2019). Comparisons of heavy metal input inventory in agricultural soils in North and South China: a review. Sci. Total Environ. 660, 776–786. doi:10.1016/j.scitotenv.2019.01.066
Peng, J., Zhang, S., Han, Y., Bate, B., Ke, H., and Chen, Y. (2022). Soil heavy metal pollution of industrial legacies in China and health risk assessment. Sci. Total Environ. 816, 151632. doi:10.1016/j.scitotenv.2021.151632
Qin, G., Niu, Z., Yu, J., Li, Z., Ma, J., and Xiang, P. (2021). Soil heavy metal pollution and food safety in China: effects, sources and removing technology. Chemosphere 267, 129205. doi:10.1016/j.chemosphere.2020.129205
Ren, Y., Lin, M., Liu, Q., Zhang, Z., Fei, X., Xiao, R., et al. (2021). Contamination assessment, health risk evaluation, and source identification of heavy metals in the soil-rice system of typical agricultural regions on the southeast coast of China. Environ. Sci. Pollut. Res. 28, 12870–12880. doi:10.1007/s11356-020-11229-6
Shen, G., Ru, X., Gu, Y., Liu, W., Wang, K., Li, B., et al. (2023). Pollution characteristics, spatial distribution, and evaluation of heavy metal(loid)s in farmland soils in a typical mountainous hilly area in China. Foods 12, 681. doi:10.3390/foods12030681
Shi, J., Yang, Y., Shen, Z., Lin, Y., Mei, N., Luo, C., et al. (2024). Identifying heavy metal sources and health risks in soil-vegetable systems of fragmented vegetable fields based on machine learning, positive matrix factorization model and Monte Carlo simulation. J. Hazard. Mater. 478, 135481. doi:10.1016/j.jhazmat.2024.135481
Singh, P. K., Shikha, D., and Saw, S. (2023). Evaluation of potential toxic heavy metal contamination in soil, fly ash, vegetables and grain crops along with associated ecological and health risk assessment of nearby inhabitants of a thermal power station in Jharkhand (India). Environ. Sci. Pollut. Res. 30, 7752–7769. doi:10.1007/s11356-022-22638-0
Tang, L., Deng, S., Tan, D., Long, J., and Lei, M. (2019). Heavy metal distribution, translocation, and human health risk assessment in the soil-rice system around Dongting Lake area, China. Environ. Sci. Pollut. Res. 26, 17655–17665. doi:10.1007/s11356-019-05134-w
Tian, J., Wang, L., Hui, S., Yang, D., He, Y., and Yuan, M. (2023). Cadmium accumulation regulated by a rice heavy-metal importer is harmful for host plant and leaf bacteria. J. Adv. Res. 45, 43–57. doi:10.1016/j.jare.2022.05.010
Wan, F., Jiang, N., Yu, L., Zang, K., Liu, S., He, W., et al. (2022). Heavy metal ecological-health risk assessment under wheat–maize rotation system in a high geological background area in eastern China. Sci. Rep. 12, 17912. doi:10.1038/s41598-022-22608-z
Wang, C.-C., Zhang, Q.-C., Yan, C.-A., Tang, G.-Y., Zhang, M.-Y., Ma, L. Q., et al. (2023a). Heavy metal(loid)s in agriculture soils, rice, and wheat across China: status assessment and spatiotemporal analysis. Sci. Total Environ. 882, 163361. doi:10.1016/j.scitotenv.2023.163361
Wang, J., Deng, P., Wei, X., Zhang, X., Liu, J., Huang, Y., et al. (2023b). Hidden risks from potentially toxic metal(loid)s in paddy soils-rice and source apportionment using lead isotopes: a case study from China. Sci. Total Environ. 856, 158883. doi:10.1016/j.scitotenv.2022.158883
Wang, J., Wang, B., Zhao, Q., Cao, J., Xiao, X., Zhao, D., et al. (2025). Sources analysis and risk assessment of heavy metals in soil in a polymetallic mining area in southeastern Hubei based on Monte Carlo simulation. Ecotoxicol. Environ. Saf. 290, 117607. doi:10.1016/j.ecoenv.2024.117607
Wang, S., Zhang, Y., Cheng, J., Li, Y., Li, F., Li, Y., et al. (2022a). Pollution assessment and source apportionment of soil heavy metals in a coastal industrial city, zhejiang, southeastern China. IJERPH 19, 3335. doi:10.3390/ijerph19063335
Wang, X., Li, X., Zhou, X., Sheng, W., Lin, K., and Ma, X. (2022b). Exploring the risk thresholds of soil heavy metals in carbonate and non-carbonate rock areas: the case of qianjiang district in chongqing, China. Bull. Environ. Contam. Toxicol. 109, 910–919. doi:10.1007/s00128-022-03580-w
Wang, X., Ma, S., Wang, X., Cheng, T., Dong, J., and Feng, K. (2022c). The mechanism of Cu2+ sorption by rice straw biochar and its sorption–desorption capacity to Cu2+ in soil. Bull. Environ. Contam. Toxicol. 109, 562–570. doi:10.1007/s00128-022-03538-y
Wang, Y., and Cheng, H. (2023). Soil heavy metal(loid) pollution and health risk assessment of farmlands developed on two different terrains on the Tibetan Plateau, China. Chemosphere 335, 139148. doi:10.1016/j.chemosphere.2023.139148
Wang, Y., Dong, A.-J., Yang, J.-F., Ma, Y.-B., Wang, Z.-J., and Yang, F.-Y. (2023c). Translocation, accumulation, and comprehensive risk assessment of heavy metals in soil-crop systems in an old industrial city, shizuishan, Ningxia, northwest China. Huan Jing Ke Xue 44, 4429–4439. doi:10.13227/j.hjkx.202209003
Wang, Y., Liu, C., Zhou, D., and Cheng, H. (2016). A new approach for evaluating soil heavy metal impact: a comprehensive index combined soil environmental quality and agricultural products quality. J. Agro-Environment Sci. 35, 1225–1232. doi:10.11654/jaes.2016.07.001
Xia, X., Ji, J., Zhang, C., Yang, Z., and Shi, H. (2022). Carbonate bedrock control of soil Cd background in Southwestern China: its extent and influencing factors based on spatial analysis. Chemosphere 290, 133390. doi:10.1016/j.chemosphere.2021.133390
Xiang, M., Ma, J., Cheng, J., Lei, K., Li, F., Shi, Z., et al. (2022). Collaborative evaluation of heavy metal pollution of soil-crop system in the southeast of Yangtze River Delta, China. Ecol. Indic. 143, 109412. doi:10.1016/j.ecolind.2022.109412
Xiao, N., Wang, F., Tang, L., Zhu, L., Song, B., and Chen, T. (2022). Recommended risk screening values for Cd in high geological background area of Guangxi, China. Environ. Monit. Assess. 194, 202. doi:10.1007/s10661-022-09802-2
Xu, H., Li, C., Wen, C., Zhu, S., Zhu, S., Li, N., et al. (2023). Heavy metal fraction, pollution, and source-oriented risk assessment in biofilms on a river system polluted by mining activities. Chemosphere 322, 138137. doi:10.1016/j.chemosphere.2023.138137
Xu, L., Dai, H., Skuza, L., Xu, J., Shi, J., Wang, Y., et al. (2022a). Integrated survey on the heavy metal distribution, sources and risk assessment of soil in a commonly developed industrial area. Ecotoxicol. Environ. Saf. 236, 113462. doi:10.1016/j.ecoenv.2022.113462
Xu, X., Luo, P., Li, S., Zhang, Q., and Sun, D. (2022b). Distributions of heavy metals in rice and corn and their health risk assessment in Guizhou province. Bull. Environ. Contam. Toxicol. 108, 926–935. doi:10.1007/s00128-021-03407-0
Xu, X., Xu, Z., Liang, L., Han, J., Wu, G., Lu, Q., et al. (2024). Risk hotspots and influencing factors identification of heavy metal(loid)s in agricultural soils using spatial bivariate analysis and random forest. Sci. Total Environ. 954, 176359. doi:10.1016/j.scitotenv.2024.176359
Yang, J., Sun, Y., Wang, Z., Gong, J., Gao, J., Tang, S., et al. (2022a). Heavy metal pollution in agricultural soils of a typical volcanic area: risk assessment and source appointment. Chemosphere 304, 135340. doi:10.1016/j.chemosphere.2022.135340
Yang, N., Hu, J., Yin, D., He, T., Tian, X., Ran, S., et al. (2023). Mercury and methylmercury in Hg-contaminated paddy soil and their uptake in rice as regulated by DOM from different agricultural sources. Environ. Sci. Pollut. Res. 30, 77181–77192. doi:10.1007/s11356-023-27712-9
Yang, Q., Li, Z., Lu, X., Duan, Q., Huang, L., and Bi, J. (2018a). A review of soil heavy metal pollution from industrial and agricultural regions in China: pollution and risk assessment. Sci. Total Environ. 642, 690–700. doi:10.1016/j.scitotenv.2018.06.068
Yang, S., Wang, Y., Ao, W., Bai, Y., and Li, C. (2018b). Prediction and analysis of CO2 emission in chongqing for the protection of environment and public health. IJERPH 15, 530. doi:10.3390/ijerph15030530
Yang, X., Zhang, Z., Sun, C., and Zeng, X. (2022b). Soil heavy metal content and enzyme activity in uncaria rhynchophylla-producing areas under different land use patterns. IJERPH 19, 12220. doi:10.3390/ijerph191912220
Yang, Z., Zhang, R., Li, H., Zhao, X., and Liu, X. (2022c). Heavy metal pollution and soil quality assessment under different land uses in the red soil region, southern China. IJERPH 19, 4125. doi:10.3390/ijerph19074125
Yu, Y., Yang, L., Yang, Y., Chen, H., Bo, L., Huang, B., et al. (2024). A collaborative approach for metal pollution assessment in production system of plastic-shed vegetables near industrial areas. Bull. Environ. Contam. Toxicol. 113, 7. doi:10.1007/s00128-024-03916-8
Zeng, F., Wei, W., Li, M., Huang, R., Yang, F., and Duan, Y. (2015). Heavy metal contamination in rice-producing soils of hunan province, China and potential health risks. IJERPH 12, 15584–15593. doi:10.3390/ijerph121215005
Zhang, H., Liang, P., Liu, Y., Wang, X., Bai, Y., Xing, Y., et al. (2022a). Spatial distributions and intrinsic influence analysis of Cr, Ni, Cu, Zn, as, Cd and Pb in sediments from the wuliangsuhai wetland, China. IJERPH 19, 10843. doi:10.3390/ijerph191710843
Zhang, L., Ruiz-Menjivar, J., Tong, Q., Zhang, J., and Yue, M. (2021). Examining the carbon footprint of rice production and consumption in Hubei, China: a life cycle assessment and uncertainty analysis approach. J. Environ. Manag. 300, 113698. doi:10.1016/j.jenvman.2021.113698
Zhang, M., Cheng, L., Yue, Z., Peng, L., and Xiao, L. (2024). Assessment of heavy metal(oid) pollution and related health risks in agricultural soils surrounding a coal gangue dump from an abandoned coal mine in Chongqing, Southwest China. Sci. Rep. 14, 18667. doi:10.1038/s41598-024-69072-5
Zhang, Q., Liu, H., Liu, F., Ju, X., Dinis, F., Yu, E., et al. (2022b). Source identification and superposition effect of heavy metals (HMs) in agricultural soils at a high geological background area of karst: a case study in a typical watershed. IJERPH 19, 11374. doi:10.3390/ijerph191811374
Zhang, T., Ni, J., and Xie, D. (2016). Assessment of the relationship between rural non-point source pollution and economic development in the Three Gorges Reservoir Area. Environ. Sci. Pollut. Res. 23, 8125–8132. doi:10.1007/s11356-016-6344-7
Zhao, G., Ma, Y., Liu, Y., Cheng, J., and Wang, X. (2022a). Source analysis and ecological risk assessment of heavy metals in farmland soils around heavy metal industry in Anxin County. Sci. Rep. 12, 10562. doi:10.1038/s41598-022-13977-6
Zhao, H., Lan, X., Yu, F., Li, Z., Yang, J., and Du, L. (2022b). Comprehensive assessment of heavy metals in soil-crop system based on PMF and evolutionary game theory. Sci. Total Environ. 849, 157549. doi:10.1016/j.scitotenv.2022.157549
Zhao, M., Wang, H., Sun, J., Cai, B., Tang, R., Song, X., et al. (2024). Human health risks of heavy metal(loid)s mediated through crop ingestion in a coal mining area in Eastern China. Ecotoxicol. Environ. Saf. 276, 116305. doi:10.1016/j.ecoenv.2024.116305
Keywords: heavy metals, IICQ index, PMF model, Monte Carlo simulation, health risks
Citation: Tong L, Yang A, Fan M, Wang D, Liu Y, Huang X, Xiang L, Fu R and Cheng L (2025) Assessment of the sources and health risks of heavy metals in the soil-rice system based on positive matrix factorization and Monte Carlo simulation. Front. Environ. Sci. 13:1558308. doi: 10.3389/fenvs.2025.1558308
Received: 10 January 2025; Accepted: 28 March 2025;
Published: 09 April 2025.
Edited by:
Oliver Matthias Wiche, Zittau/Görlitz University of Applied Sciences, GermanyCopyright © 2025 Tong, Yang, Fan, Wang, Liu, Huang, Xiang, Fu and Cheng. This is an open-access article distributed under the terms of the Creative Commons Attribution License (CC BY). The use, distribution or reproduction in other forums is permitted, provided the original author(s) and the copyright owner(s) are credited and that the original publication in this journal is cited, in accordance with accepted academic practice. No use, distribution or reproduction is permitted which does not comply with these terms.
*Correspondence: Longfeng Cheng, aGFpbmFzdGlva291QGhvdG1haWwuY29t
†These authors share first authorship
Disclaimer: All claims expressed in this article are solely those of the authors and do not necessarily represent those of their affiliated organizations, or those of the publisher, the editors and the reviewers. Any product that may be evaluated in this article or claim that may be made by its manufacturer is not guaranteed or endorsed by the publisher.
Research integrity at Frontiers
Learn more about the work of our research integrity team to safeguard the quality of each article we publish.