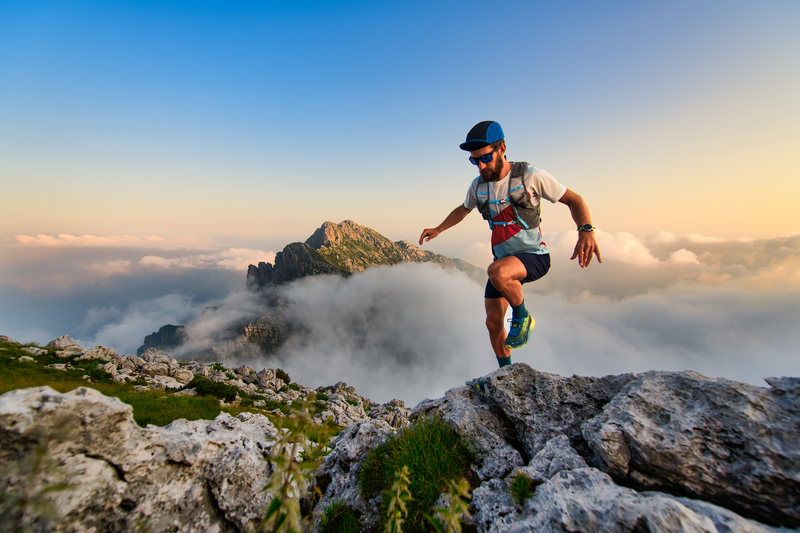
94% of researchers rate our articles as excellent or good
Learn more about the work of our research integrity team to safeguard the quality of each article we publish.
Find out more
ORIGINAL RESEARCH article
Front. Environ. Sci.
Sec. Freshwater Science
Volume 13 - 2025 | doi: 10.3389/fenvs.2025.1557004
This article is part of the Research Topic Nitrate from Field to Stream: Characterization and Mitigation View all 11 articles
The final, formatted version of the article will be published soon.
You have multiple emails registered with Frontiers:
Please enter your email address:
If you already have an account, please login
You don't have a Frontiers account ? You can register here
Nitrogen pollution in aquatic ecosystems, primarily from agricultural sources, presents significant environmental challenges. At the land management decision level, reducing nitrate leaching requires knowledge of nitrate loading over time and location, the complexity of which is amplified by limited data availability, especially in poorly gauged watersheds. This issue is particularly pronounced in cold and humid regions where water quality data are often collected during the growing season only. Large data gaps result in systematic errors when estimating nitrogen load based on traditional regression methods. In this study, we explore the feasibility of using processbased hydrologic model to estimate nitrate loads from sparse temporal water quality data in a coastal agricultural watershed in Atlantic Canada and compared its performance with three regression methods. We found that the absence of the available 16% non-growing season data during the 10-year study period can lead to significant biases (as high as 21%) in load estimation by regression methods. In contrast, nitrate load estimates obtained with the Soil and Water Assessment Tool (SWAT) were less sensitive to systematic data gaps. The results suggest that process-based models like SWAT can be a viable alternative for nitrate load estimation when limited data is available. As agri-environmental water quality issues become more pressing, it is crucial to use appropriate methods based on data quality and availability to avoid misleading results.
Keywords: Nitrate load, Sparse data, Water Quality, Regression model, Physical-based model
Received: 07 Jan 2025; Accepted: 13 Feb 2025.
Copyright: © 2025 Liang, Jiang, Fuller, Cordeiro, Zhang, Qi, Geng, Liu, Zhang, Azimi and Meng. This is an open-access article distributed under the terms of the Creative Commons Attribution License (CC BY). The use, distribution or reproduction in other forums is permitted, provided the original author(s) or licensor are credited and that the original publication in this journal is cited, in accordance with accepted academic practice. No use, distribution or reproduction is permitted which does not comply with these terms.
* Correspondence:
Kang Liang, Oak Ridge Institute for Science and Education (ORISE), Oak Ridge, 117, Tennessee, United States
Disclaimer: All claims expressed in this article are solely those of the authors and do not necessarily represent those of their affiliated organizations, or those of the publisher, the editors and the reviewers. Any product that may be evaluated in this article or claim that may be made by its manufacturer is not guaranteed or endorsed by the publisher.
Research integrity at Frontiers
Learn more about the work of our research integrity team to safeguard the quality of each article we publish.