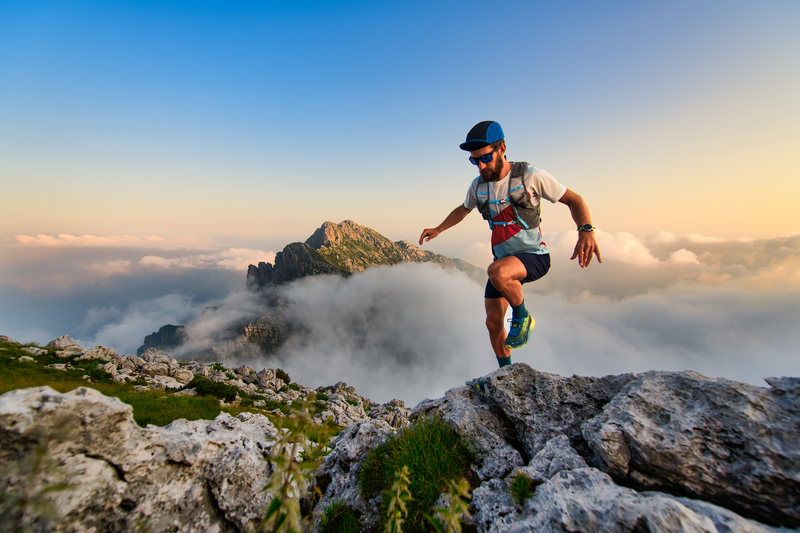
95% of researchers rate our articles as excellent or good
Learn more about the work of our research integrity team to safeguard the quality of each article we publish.
Find out more
ORIGINAL RESEARCH article
Front. Environ. Sci.
Sec. Environmental Systems Engineering
Volume 13 - 2025 | doi: 10.3389/fenvs.2025.1553121
This article is part of the Research Topic Municipal Solid Waste Landfills: Environmental Effects and Pollution Management View all articles
The final, formatted version of the article will be published soon.
You have multiple emails registered with Frontiers:
Please enter your email address:
If you already have an account, please login
You don't have a Frontiers account ? You can register here
Municipal Solid Waste Generation (MSWG) presents a significant challenge for sustainable urban development, with waste production escalating at alarming rates worldwide. To address this issue, accurate predictive models are essential for optimizing waste management strategies. This study utilizes a comprehensive dataset of 4,343 records from municipal waste management, incorporating variables such as population density, urbanization indices, and waste composition. Advanced machine learning algorithms, including Decision Trees (DT), Random Forest (RF), LightGBM, and XGBoost, are employed, with XGBoost being introduced as a novel approach for MSWG prediction. Its ability to model complex nonlinear relationships, handle missing data and outliers robustly, and prevent overfitting through advanced regularization techniques sets it apart from other models. The study finds that XGBoost outperforms the other algorithms, achieving an R² value of 0.985 and an RMSE of 0.056, making it the most accurate predictor of MSWG. The flexibility and scalability of XGBoost further enhance its applicability in managing diverse datasets, and its feature-ranking capability is instrumental in identifying key factors influencing waste generation. The results demonstrate that incorporating XGBoost into waste management frameworks can significantly improve resource allocation, reduce operational costs, and contribute to environmental sustainability. This approach not only advances predictive methodologies in MSWG management but also provides actionable insights for urban planners and policymakers in effectively tackling the growing waste management crisis. The findings highlight the potential of machine learning, particularly XGBoost, as a transformative tool for strategic decision-making in environmental management.
Keywords: Municipal Solid Waste Generation (MSWG), machine learning algorithms, XGBoost, Urban sustainability, Predictive Modeling
Received: 30 Dec 2024; Accepted: 25 Mar 2025.
Copyright: © 2025 Liu, Zhi and Akhundzada. This is an open-access article distributed under the terms of the Creative Commons Attribution License (CC BY). The use, distribution or reproduction in other forums is permitted, provided the original author(s) or licensor are credited and that the original publication in this journal is cited, in accordance with accepted academic practice. No use, distribution or reproduction is permitted which does not comply with these terms.
* Correspondence:
Abed Akhundzada, Faculty of Engineering, Kardan University, Kabul, Afghanistan, Kabul, Afghanistan
Disclaimer: All claims expressed in this article are solely those of the authors and do not necessarily represent those of their affiliated organizations, or those of the publisher, the editors and the reviewers. Any product that may be evaluated in this article or claim that may be made by its manufacturer is not guaranteed or endorsed by the publisher.
Research integrity at Frontiers
Learn more about the work of our research integrity team to safeguard the quality of each article we publish.