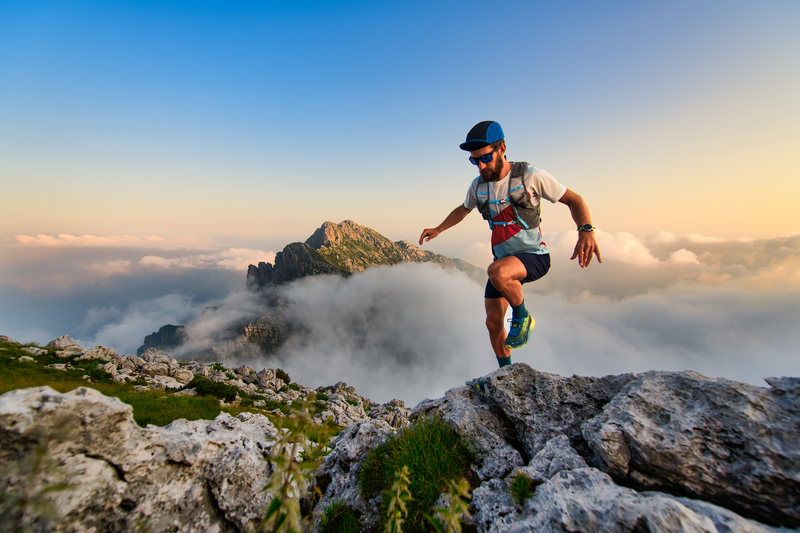
95% of researchers rate our articles as excellent or good
Learn more about the work of our research integrity team to safeguard the quality of each article we publish.
Find out more
ORIGINAL RESEARCH article
Front. Environ. Sci.
Sec. Big Data, AI, and the Environment
Volume 13 - 2025 | doi: 10.3389/fenvs.2025.1550745
The final, formatted version of the article will be published soon.
You have multiple emails registered with Frontiers:
Please enter your email address:
If you already have an account, please login
You don't have a Frontiers account ? You can register here
The rapid evolution of railway systems, driven by digitization and the proliferation of Internet-of-Things (IoT) devices, has resulted in an unprecedented volume of diverse and complex data. This Railway Big Data offers immense opportunities for advancing safety, efficiency, and sustainability in transportation but presents significant analytical challenges due to its heterogeneity, highdimensionality, and temporal dependencies. Existing approaches often fall short in fully exploiting these data characteristics, struggling with multi-source integration, real-time predictive capabilities, and adaptability to dynamic environments. To address these gaps, we propose a novel framework leveraging deep learning techniques tailored to Railway Big Data. Our method integrates temporal encoders and spatial graph neural networks, combined with domain-specific knowledge and contextual awareness, to achieve robust anomaly detection, predictive maintenance, and passenger demand forecasting. By capturing both spatial relationships and temporal patterns, the proposed framework ensures comprehensive insights into system behavior, enabling proactive decision-making and operational optimization. Experimental results on real-world railway datasets demonstrate superior performance in accuracy, scalability, and interpretability compared to traditional methods, underscoring the potential of our approach for next-generation intelligent railway systems. This work aligns with the goals of integrating Big Data and AI for environmental and operational improvements in railway transportation, contributing to a sustainable, resilient, and adaptive infrastructure capable of meeting future mobility demands.
Keywords: Railway Big Data, deep learning, Predictive maintenance, anomaly detection, Intelligent Transportation Systems
Received: 24 Dec 2024; Accepted: 01 Apr 2025.
Copyright: © 2025 Hang. This is an open-access article distributed under the terms of the Creative Commons Attribution License (CC BY). The use, distribution or reproduction in other forums is permitted, provided the original author(s) or licensor are credited and that the original publication in this journal is cited, in accordance with accepted academic practice. No use, distribution or reproduction is permitted which does not comply with these terms.
* Correspondence:
Lyubai Hang, China Construction Civil Construction Co., LTD, Beijing, China
Disclaimer: All claims expressed in this article are solely those of the authors and do not necessarily represent those of their affiliated organizations, or those of the publisher, the editors and the reviewers. Any product that may be evaluated in this article or claim that may be made by its manufacturer is not guaranteed or endorsed by the publisher.
Research integrity at Frontiers
Learn more about the work of our research integrity team to safeguard the quality of each article we publish.