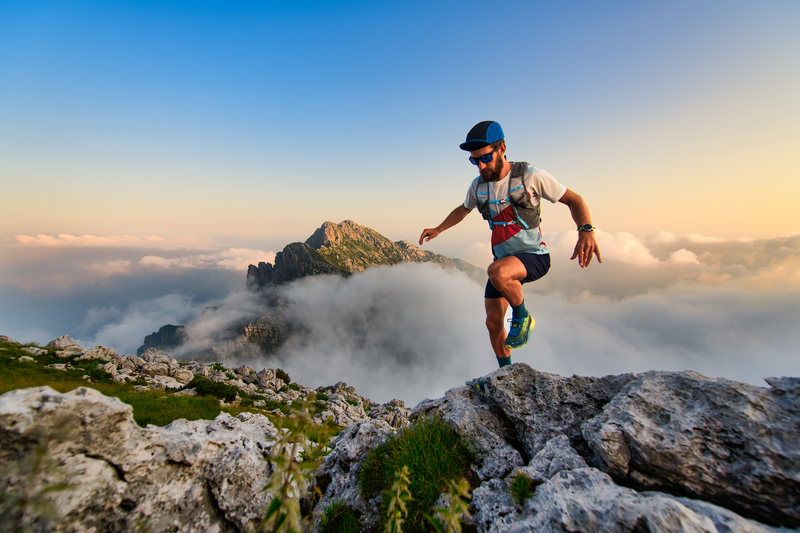
95% of researchers rate our articles as excellent or good
Learn more about the work of our research integrity team to safeguard the quality of each article we publish.
Find out more
ORIGINAL RESEARCH article
Front. Environ. Sci.
Sec. Land Use Dynamics
Volume 13 - 2025 | doi: 10.3389/fenvs.2025.1543524
The final, formatted version of the article will be published soon.
You have multiple emails registered with Frontiers:
Please enter your email address:
If you already have an account, please login
You don't have a Frontiers account ? You can register here
Water catchment areas are the key strategic water sources with a variety of ecological benefits. However, the trajectory of Land Cover and Land Use Changes (LULC-C(LULC) change poses a significant threat to water catchment areas, negatively affecting water quality. Thus, the adoption of remote sensing data and Machine Learning Algorithms (MLAs) is a novel approach that provides spatiotemporal data on the environmental changes resulting from LULC dynamics. Hence, this work harnessed Landsat imageries and the Random Forests (RF) classification as well as a hybrid model from the Multi-Layer Perceptron and Markov chain (MLPNN-Markov) to detect changes in LULC and forecast future changes.At every five years interval, the RF model generated more accurate maps for 2003-2023. The LULC prediction for 2019 also produced acceptable values for the kappa accuracy matrices, which were 65.50%, 58.4%, 90.90%, and 0.52 for overall accuracy, kappa location, kappa histogram, and kappa overall, respectively. The findings highlighted the decline of forest areas, with a strong negative correlation with built-up and mining areas. The secondary invasion of the abandoned cropland occupied by grassland members was observed. Thus, grassland displayed increasing trends between 2019 and 2023. Wetlands and water, however, exhibited a steady trend with minor variations. On the other hand, each of these trends persisted in the future, with the exception of grassland areas that displayed scaling-down behaviour in 2032.The outcomes of this work will offer a piece of updated information on the LULC-C and hints at the possible future direction for the trends by 2032. This is crucial to local bodies tasked to protect the integrity of the water catchment areas with the aim of improving the water quality.
Keywords: random forest, LULC, Landsat, change detection, uMngeni River Catchment, South Africa, MLPNN-Markov
Received: 11 Dec 2024; Accepted: 07 Apr 2025.
Copyright: © 2025 Bhungeni, Gebreslasie and Ramjatan. This is an open-access article distributed under the terms of the Creative Commons Attribution License (CC BY). The use, distribution or reproduction in other forums is permitted, provided the original author(s) or licensor are credited and that the original publication in this journal is cited, in accordance with accepted academic practice. No use, distribution or reproduction is permitted which does not comply with these terms.
* Correspondence:
Orlando Bhungeni, School of Agricultural, Earth and Environmental Sciences, Westville Campus, University of KwaZulu-Natal, Durban, South Africa
Disclaimer: All claims expressed in this article are solely those of the authors and do not necessarily represent those of their affiliated organizations, or those of the publisher, the editors and the reviewers. Any product that may be evaluated in this article or claim that may be made by its manufacturer is not guaranteed or endorsed by the publisher.
Research integrity at Frontiers
Learn more about the work of our research integrity team to safeguard the quality of each article we publish.