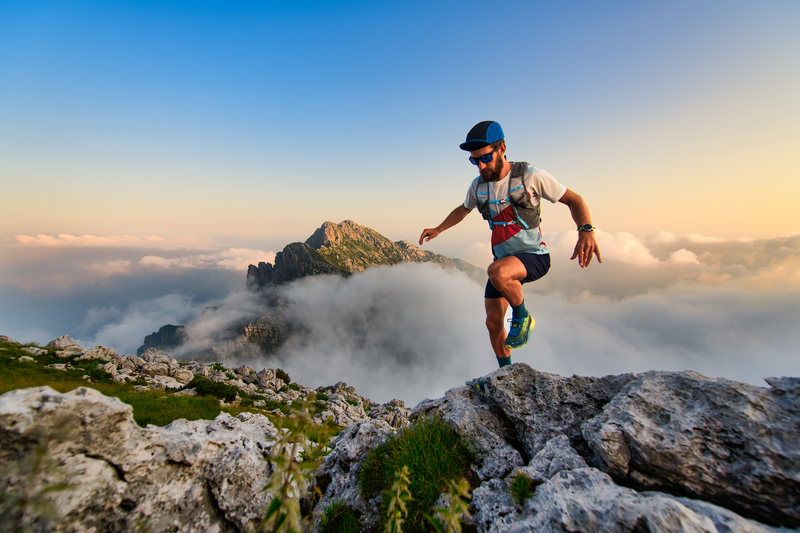
94% of researchers rate our articles as excellent or good
Learn more about the work of our research integrity team to safeguard the quality of each article we publish.
Find out more
ORIGINAL RESEARCH article
Front. Environ. Sci.
Sec. Environmental Economics and Management
Volume 13 - 2025 | doi: 10.3389/fenvs.2025.1542363
This article is part of the Research Topic Intelligence and Big Data for Sustainable Urban Built Environment View all 5 articles
The final, formatted version of the article will be published soon.
You have multiple emails registered with Frontiers:
Please enter your email address:
If you already have an account, please login
You don't have a Frontiers account ? You can register here
The role of digital economy (DE) in improving urban ecological development (UED) has attracted scholarly attention. Additionally, traditional causal inference models encounter several challenges, such as model misspecification and the "curse of dimensionality." In response to these problems, the double machine learning method is applied to assess the effect of DE on UED. Leveraging data from 282 Chinese cities in 2006-2021, several valuable conclusions emerge. First, DE improves UED and positively contributes to ecological resilience and recovery. Second, promoting green innovation, enhancing environmental efficiency, and optimizing industrial structures are the pathways through which DE contributes to UED. Third, the influence of DE on UED displays heterogeneity. Based on the results, this work proposes relevant recommendations grounded in empirical research.
Keywords: digital economy, Urban ecological development, Double machine learning, Causal mediation model, causal inference model
Received: 10 Dec 2024; Accepted: 19 Mar 2025.
Copyright: © 2025 Jiang, Li and Xu. This is an open-access article distributed under the terms of the Creative Commons Attribution License (CC BY). The use, distribution or reproduction in other forums is permitted, provided the original author(s) or licensor are credited and that the original publication in this journal is cited, in accordance with accepted academic practice. No use, distribution or reproduction is permitted which does not comply with these terms.
* Correspondence:
Yue Xu, Suzhou City University, Suzhou, China
Disclaimer: All claims expressed in this article are solely those of the authors and do not necessarily represent those of their affiliated organizations, or those of the publisher, the editors and the reviewers. Any product that may be evaluated in this article or claim that may be made by its manufacturer is not guaranteed or endorsed by the publisher.
Research integrity at Frontiers
Learn more about the work of our research integrity team to safeguard the quality of each article we publish.