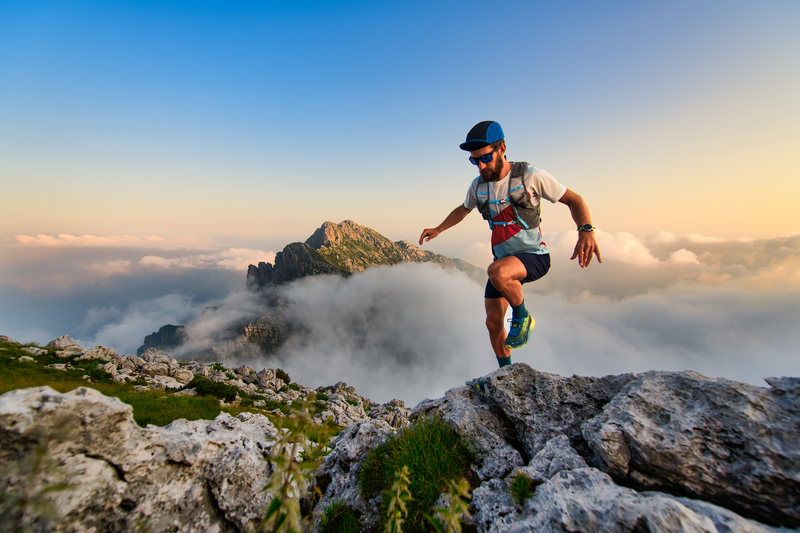
95% of researchers rate our articles as excellent or good
Learn more about the work of our research integrity team to safeguard the quality of each article we publish.
Find out more
ORIGINAL RESEARCH article
Front. Environ. Sci. , 17 January 2025
Sec. Environmental Economics and Management
Volume 13 - 2025 | https://doi.org/10.3389/fenvs.2025.1529946
This article is part of the Research Topic Environmental Resilience and Sustainable Agri-food System Management View all 13 articles
Introduction: The digital economy is reshaping economic structures and resource flows, but its role in enhancing resource allocation efficiency within the framework of green development has not yet been fully explored.
Methods: This paper analyzes panel data from 257 Chinese cities to investigate how the digital economy influences regional resource allocation efficiency, with a focus on the mediating role of green innovation.
Results: The results show that the digital economy significantly improves resource allocation efficiency, with nonlinear effects characterized by increasing marginal returns. Mechanism tests reveal that the digital economy optimizes resource allocation by fostering green innovation. Heterogeneity analysis indicates that in eastern regions and areas with strong environmental regulations, the digital economy promotes both substantive and strategic green innovations, leading to higher efficiency. In mid-western regions, only substantive green innovation has a significant effect, while regions with weak environmental regulations experience less pronounced impacts.
Discussion: This study offers insights into leveraging green innovation to achieve efficient resource allocation and strengthen environmental resilience in the digital era.
China’s economy has historically achieved rapid growth under a rough development model. However, this phase of GDP surge, heavily reliant on massive resource consumption, has inevitably triggered issues such as overcapacity, resource shortages, and environmental pollution (Yang et al., 2022). Given the evident negative externalities of pollution, numerous enterprises characterized by “three highs and one low” (excessive input, high energy consumption, significant pollution emissions, and low efficiency) have proliferated. This not only contravenes the principles of sustainable development but also consumes substantial economic resources, constrains the survival of clean and environmentally friendly enterprises, and generates severe factor mismatches (Bian et al., 2019; Hao et al., 2020), leading to a decline in overall productivity (Lyu et al., 2023; Wang and Cao, 2023). It implies that environmental pollution and resource allocation inefficiency are two closely related propositions, and promoting green innovation may be a feasible solution to the problem of resource mismatch.
Meanwhile, the advancement of the latest technological revolution has enabled the digital economy to exert a profound impact on the global economy and society. Thanks to the government’s forward-looking policies, as well as market and population advantages, China’s digital economy has gained remarkable progress. According to the 2023 White Paper on China’s Digital Economy Development, its value reached 50.2 trillion yuan in 2022, accounting for approximately 41.5% of the GDP. Theoretically, digital economy has the advantages of low consumption of natural resources, less pollution emissions and environmental friendliness in the course of factor mobility (Hao et al., 2023). The high penetrability of digital technologies can effectively mitigate information asymmetry, thus reducing resource misallocation (Xu et al., 2023). Nevertheless, some scholars concern that the application of digital technologies necessitates substantial investment and incurs high data storage costs, which will cause an unbalanced distribution of limited resources (Clarke, 2016). In addition, the rapid development of digitalization has increased market concentration, which contributes to industry monopolies and a regional “digital divide”, potentially causing new resource mismatches (Mariscal, 2005). Can the emerging digital economy provide a novel approach to addressing regional resource misallocation and advancing environmentally sustainable economic transformation? This study seeks to explore this question by examining the impact of the digital economy on regional green innovation. On one hand, digital technologies have the potential to accelerate the R&D process of green technologies and promote their broader adoption in practical applications (Guo et al., 2023). On the other hand, as environmental issues become increasingly prominent, regional resource allocation and utilization are shifting toward green, low-emission, and circular practices (Lu and Li, 2024). Recently, China has actively complied with the worldwide trend of green and low-carbon development, demonstrating extensive involvement in international efforts to reduce carbon emissions, and clearly puts forward the “dual-carbon” objective of striving for “carbon peak” by 2030 and “carbon neutrality” by 2060. Obviously, digital economy and green innovation have become typical facts and key variables in China’s economic green transformation. How to effectively unleash the dividend effect of the digital economy on resource allocation efficiency? How to give full play to enabling role of green innovation in allocating resources optimally? Addressing these questions can provide empirical evidence for evaluating the effects of the digital economy on regional resource allocation efficiency, offer insights into leveraging green innovation to optimize resource allocation and enhance environmental resilience, and guide policy-making toward achieving the “dual-carbon” objectives and sustainable economic development. In light of this, this study empirically investigates the influence and mechanisms of the digital economy on resource allocation efficiency, utilizing panel data from 257 prefecture-level and above cities in China from 2007 to 2021.
The main contributions of this study are reflected in three aspects. First, this study introduces a novel approach to measuring the digital economy by incorporating enterprise-level digital transformation data into the assessment framework for regional digital economy indicators. By recognizing enterprises as the micro-foundations of the macroeconomy, this method enables a more granular and comprehensive evaluation of urban digital economy development, bridging the gap between macroeconomic analysis and micro-level digital activities. Second, the study establishes an integrated analytical framework linking the digital economy, green innovation, and resource allocation efficiency. This framework provides a new perspective on the mechanisms through which digital transformation enhances regional resource allocation. By situating this relationship within the context of green development, the study broadens the understanding of these relationships and emphasizes the critical role of sustainability in driving economic efficiency. Moreover, the study explores the heterogeneous effects of urban green innovation by categorizing it into substantive and strategic types. This classification uncovers how different types of green innovation respond to varying environmental regulations and geographic conditions. By examining these dynamics, the study deepens the understanding of the transmission mechanism from “digital economy - green innovation - regional resource allocation efficiency”, advancing the understanding of the interplay between innovation types and regional characteristics.
Research closely associated with this topic primarily encompasses two major branches: the digital economy’s impact on resource allocation efficiency and its influence on green innovation.
The first branch pertains to the literature on the digital economy and its effect on regional resource allocation efficiency. Several scholars have investigated the impact of informatization and intelligence on factor misallocation (Colombo et al., 2019; Yu et al., 2023). While indicators such as the Internet and artificial intelligence reflect digital progress, they are not entirely synonymous with digital transformation. A smaller group of studies has directly examined how the digital economy affects resource allocation. For example, Lyu et al. (2023) used provincial panel data from China, finding that the digital economy significantly reduces capital misallocation and creates spatial spillover effects. Zhang, 2024 found that the digital economy positively influences resource allocation in both capital and labor, promoting regional balance through enhanced factor mobility. Wei et al. (2022) observed that digital advancement could enhance resource allocation efficiency in China’s service sector. Li et al. (2023) highlighted the pivotal role of digital empowerment in optimizing enterprise capital allocation in the digital age. Chen and Chen (2024) demonstrated that digitalization improves innovation factor allocation efficiency, especially for innovation capital, with limited effects in inland regions and areas of excessive allocation. Zhao et al. (2024) identified a nonlinear, inverted U-shaped impact of the digital economy on urban manufacturing, with higher thresholds in top-tier cities and potential negative spillover effects on neighboring areas. However, many studies have used resource allocation efficiency as a mediating variable to explore the impact of digitalization on economic quality development (Chen et al., 2023), urban-rural income gap (Jiang et al., 2022), and carbon emissions reduction (Li and Wang, 2022), without deeply analyzing how resource allocation efficiency itself is influenced.
The second branch of the literature investigates the influence of the digital economy on green innovation, revealing its dualistic effects. Numerous studies emphasize the supportive role of digitalization in fostering environmentally friendly innovation. Luo et al. (2023) concluded that digitalization advances green technology by enhancing trade freedom, modernizing industrial structures, and expanding market opportunities. Dong et al. (2024) observed that the digital economy boosts green innovation through marketization mechanisms and a dual-threshold effect, highlighting the importance of coordinated development between digitalization and market reforms. Similarly, Qiu et al. (2023) suggested that the digital economy directly fosters urban green innovation while also indirectly enhancing it by strengthening environmental regulation intensity. Studies by Bai et al. (2024) and Dian et al. (2024) further showed that digitalization significantly promotes green technological innovation in urban areas of China, accompanied by positive spillover effects. However, some studies caution that rapid digital expansion may introduce challenges, such as “information overload” and data security concerns (Tang and Veelenturf, 2019), where the costs of managing information surpass the benefits (Gebauer et al., 2020). These challenges can impose financial burdens on firms, thereby hindering green innovation (Ghasemaghaei and Calic, 2020). Furthermore, although the advancement of the digital economy holds promise for promoting green innovation, this impact often depends on enterprises’ resource bases and technological capabilities. Without sufficient R&D investment and technology infrastructure, the expected gains in green innovation may be constrained (Li et al., 2016). Therefore, some scholars argue that the influence of the digital economy on green innovation is nonlinear or even negative. For instance, Dai et al. (2022) noted a nonlinear relationship between digitalization and regional green innovation, emphasizing the pivotal role of R&D personnel and the necessity of aligning human capital with R&D funding. Ge et al. (2024) identified that the digital economy may hinder green technological innovation in less developed regions, including Tier 4 and Tier 5 cities and resource-dependent areas. These findings collectively suggest that the influence of the digital economy on green innovation is context-dependent, requiring careful consideration of regional and firm-level conditions.
In summary, the digital economy, green innovation, and resource allocation efficiency have emerged as central themes in contemporary economic and social development, attracting extensive scholarly attention and producing a substantial body of research. Existing studies provide valuable insights, such as the potential of the digital economy to mitigate factor misallocation, generate spatial spillovers, and drive environmentally friendly technological progress. However, most research either treats resource allocation efficiency as a mediating variable for broader outcomes, such as economic growth or emission reductions, or examines these topics in isolation. To the best of our knowledge, no studies have yet constructed a comprehensive analytical framework that integrates the digital economy, green innovation, and resource allocation efficiency. This paper aims to address this gap by examining these dynamics in an integrated manner, offering a deeper analysis of how the digital economy influences regional resource allocation efficiency through green innovation. This unified framework not only advances theoretical understanding but also provides actionable insights for policymakers striving to achieve balanced and sustainable economic growth.
The digital economy leverages data to optimize resource allocation and regeneration, thereby enhancing productivity. Its impact on regional resource allocation efficiency can be understood through its penetrative, integrative, and synergistic effects. Digital technologies, such as big data, cloud computing, and the Internet of Things (IoT), possess remarkable penetrative capabilities, extending across various sectors and reshaping traditional resource allocation methods (Wu and Yu, 2022; Lyu et al., 2023). This penetration breaks down industry barriers (Carlsson, 2004), supports cross-industry and cross-domain resource sharing, and enhances resource utilization efficiency (Zhang and Wang, 2023). As the digital economy advances, it blurs boundaries between industries, fostering industrial integration and promoting resource diversity and inclusiveness (Wang et al., 2023; Ren et al., 2022). For instance, the convergence of the Internet with manufacturing has given rise to smart manufacturing, while its integration with finance has expanded inclusive finance. These developments contribute to more flexible resource allocation and bolster regional economic growth. Furthermore, digital platforms unite key elements such as land, capital, labor, technology, and information into cohesive data value chains (Williams, 2021). By facilitating rapid information matching across economic entities (Goldfarb and Tucker, 2019), these platforms significantly improve resource allocation efficiency.
However, it is important to recognize the “network effect” inherent to the digital economy (Sturgeon, 2021). In regional resource allocation, this effect manifests as high development costs in areas with low digital technology levels, making it difficult to realize the digital economy’s optimization potential for resource allocation (Qiu et al., 2023). As the digital economy continues to expand, information dissemination and sharing accelerate, facilitating more efficient interregional and cross-industry resource flows. Consequently, the role of the digital economy in improving resource allocation efficiency becomes increasingly evident. These dynamics indicate that the impact of the digital economy on regional resource allocation efficiency is nonlinear, characterized by increasing marginal returns and a “Matthew effect” within the resource allocation system. Based on this analysis, we propose the following hypotheses.
Hypothesis 1. The digital economy enhances regional resource allocation efficiency.
Hypothesis 2. The impact of the digital economy on regional resource allocation efficiency is nonlinear, exhibiting a growing marginal effect.
The digital economy acts as a driving force for green innovation (Luo et al., 2023). At the micro level, digital technologies catalyze green technological advancements across various domains. For instance, intelligent manufacturing technologies in pollution control and recycling transform high-energy, high-emission processes into efficient, sustainable practices, achieving significant energy savings and emissions reductions (Shen and Zhang, 2023). Big data analytics enable companies to better understand market demands, guiding the development of eco-friendly products (Wu et al., 2016), while cloud computing provides platforms for resource sharing and collaboration in green innovation (Yang et al., 2017). Additionally, digital technologies foster the growth of emerging green industries and sustainable business models, promoting eco-friendly consumption patterns and encouraging high-emission industries to adopt sustainable practices (Yang et al., 2020). At the macro level, digital technologies accelerate the dissemination of regional innovation resources and the spillover of knowledge (Dai et al., 2022), enabling cities to assimilate advanced green technologies and enhance their green innovation capabilities (Zhu et al., 2020). Green innovation, in turn, optimizes regional resource allocation by enhancing resource efficiency and environmental quality. It helps enterprises reduce waste, minimize production-related environmental impacts (Ma and Zhu, 2022), lower costs, and improve economic benefits, thereby attracting high-quality resources to the region.
Overall, the digital economy transforms traditional production systems, improves supply-demand matching, and maximizes clean energy utilization through innovative green technologies, fostering high-quality development with minimal ecological impact (Hao et al., 2023). This positive feedback loop supports a more rational and efficient system of resource allocation, establishing a beneficial transmission pathway: “digital economy - green innovation - regional resource allocation efficiency.” The aforementioned analysis leads to the proposal of Hypothesis 3.
Hypothesis 3. The digital economy improves regional resource allocation efficiency by advancing green innovation.
Chinese cities exhibit significant regional heterogeneity in terms of economic development, topographical conditions, and resource endowments (Chen and Zhou, 2017). Furthermore, the persistent “digital divide” underscores disparities in regional digital economy development, necessitating further attention (Ma and Zhu, 2022). In this context, the interplay between the digital economy, green innovation, and resource allocation efficiency is likely to be shaped by regional heterogeneity. Addressing these disparities requires enhanced policy support and investment to strengthen digital economy growth and green innovation capacity, thereby mitigating constraints related to regional heterogeneity.
Environmental regulation also plays a pivotal role as a government-driven policy for environmental protection. It not only shapes regional green development but also inevitably influences patterns of economic resource allocation (Zhao et al., 2023). Scholars like Porter and Linde, 1995 argue that stringent environmental requirements imposed by governments drive enterprises to intensify R&D efforts and optimize production processes through sustained green technological innovation. Thus, environmental regulation acts as a catalyst for green innovation. However, some scholars present opposing perspectives, suggesting that excessive pollution control costs arising from stringent regulations may crowd out technological R&D activities and impede innovation (Clarkson et al., 2004; Zhao and Sun, 2016; Bel and Joseph, 2018). In summary, the strength of local government environmental regulation should affect the functioning of green innovation. Accordingly, we propose the hypotheses as follows.
Hypothesis 4. The transmission of green innovation between the digital economy and regional resource allocation efficiency varies by region.
Hypothesis 5. The transmission of green innovation between the digital economy and regional resource allocation efficiency varies by the environmental regulation intensity.
The theoretical framework of this paper is demonstrated in Figure 1.
First, to explore the impact of the digital economy on regional resource allocation efficiency, we set up a two-way fixed effects benchmark model as shown in Equation 1:
where the subscript c denotes the city, t denotes the year, Eff_Kct represents the city’s resource allocation efficiency, Digct represents the digital economy development index, and Xct represents a group of control variables. Additionally, we control for city fixed effects λc and year fixed effects μt, while employing robust standard errors to cluster at the city level. The main focus in this model is on the significance and sign of α1. A significantly positive α1 would indicate that the digital economy enhances resource allocation efficiency.
Second, to further investigate the nonlinear relationship between the digital economy and the efficiency of resource allocation, the quantile regression model is formulated as shown in Equation 2:
where Quantτ(Eff_Kct) denotes the value of Eff_Kct corresponding to the τth quantile; β1 denotes the marginal impact of each quantile.
Finally, to test the impact pathway of “digital economy - green innovation - regional resource allocation efficiency”, we add a cross-multiplier term of Dig and mechanism variable in the baseline model, and construct the following interaction term model for mechanism testing, as shown in Equation 3:
In the formula, Mct is the mechanism variable, which represents green innovation. γ1 is the regression coefficient reflecting the cross-multiplier term, if γ1 exhibits a significant positive value, it indicates that the digital economy fosters green innovation, which in turn enhances the regional resource allocation efficiency.
Resource allocation efficiency (Eff_K). Following Zhao et al. (2024), we use the covariance between productivity and factor share (OP covariance) to assess regional resource allocation efficiency. It is done by first calculating the resource allocation efficiency at the city-industry level, as shown in Equation 4:
where μcjit represents the productivity of firm i in industry j in city c and year t, ‾μcjt denotes the average productivity of firms in industry j in city c in year t. Similarly, ωcjit represents the factor share of firm i, while ‾ωcjt is the average factor share for firms in the same industry and city. We apply the OP method to compute firm productivity, using the capital share as a proxy for each firm’s factor share ωcjit, and calculate city-industry level resource allocation efficiency, denoted as Eff_Kcjt. A higher value of this indicator suggests that firms with higher productivity within the city-industry obtain a larger share of capital, indicating higher regional resource allocation efficiency.
To aggregate resource allocation efficiency at the city level, we use the capital share of each industry within the city (Scjt) as a weight, resulting in the city-level resource allocation efficiency, as shown in Equation 5:
In addition, we use the covariance between productivity and labor share, Eff_L, computed by the OP method, and the covariance between productivity and capital share, Eff_K_LP, computed by the LP method, as proxies for the explained variable to be used in the robustness tests later.
Digital economy development index (Dig). Numerous studies utilize regional indicators to establish comprehensive frameworks for assessing digital economy development (Li and Wang, 2022; Pan et al., 2022). However, as enterprises constitute the micro-foundation of the macroeconomy, their digital applications are pivotal in driving digital economy advancement. Therefore, we construct an evaluation framework that integrates macro-level digital development indicators with micro-level enterprise digital applications (Supplementary Appendix A.1). Macro-level digital development is assessed using four city-level indicators: per capita telecommunication service volume, the number of internet users per 100 population, the number of cellular subscribers per 100 population, and the proportion of employees engaged in information transmission, computer services, and software relative to total employment. Micro-level digital application is evaluated based on the degree of digital transformation within enterprises. Specifically, Python web scraping is employed to extract relevant keywords from the annual reports of publicly listed companies (Supplementary Appendix A.2). The number of words related to digital transformation keywords is used to evaluate the degree of enterprise digital transformation. All five indicators are standardized, and the entropy method is applied to compute the digital economy development index, denoted as Dig. For robustness testing, we further use keyword frequency related to digital transformation to remeasure the extent of enterprise digital transformation. The entropy method is then reapplied to construct an alternative Digital Economy Development Index, denoted as Dig_words.
Green innovation. The number of patents is a widely used metric for measuring innovation in existing studies (Luo et al., 2023; Yuan and Pan, 2023; Tian et al., 2024). In the context of urban green innovation practices, different motivations for green innovation can lead to varying effects of the digital economy. Drawing on Jiang and Bai (2022), who categorize urban green innovation based on its motivation, we classify it into two distinct types: substantive green innovation and strategic green innovation. Substantive green innovation refers to activities aimed at promoting technological progress, enhancing competitive advantages, and improving environmental performance. It is primarily reflected in green invention patents. In contrast, strategic green innovation represents activities driven by the pursuit of additional benefits, often aligning with government policies by emphasizing the quantity and speed of innovation. This is typically reflected in green utility model patents. Considering that patented technologies are likely to generate economic and social impacts during the application process, we use patent applications for green inventions per 10,000 population (GreenIno_apply) and patent applications for green new utility models per 10,000 population (GreenFm_apply) to characterize substantive green innovation and strategic green innovation, respectively. In order to enhance the robustness of the mechanism test, we also adopt patents granted for green inventions per 10,000 population (GreenIno_grant) and patents granted for green new utility models per 10,000 population (GreenFm_grant) as the alternative indicators of the two types of green innovations for the regression analysis, respectively.
Drawing on Wei et al. (2022) and Cao and Su (2024), we incorporate the following control variables into our regression model: Level of economic development (Pgdp). The enhancement of economic progress contributes to the efficient distribution of resources, but it may also trigger problems such as excessive concentration of resources and unfair distribution. Measured using the natural logarithm of GDP per capita. Industrial Structure (Sec). The evolution of industrial structure is the gradual substitution of high-productivity industries for low-productivity industries, and this process can optimize the allocation of factors in different industries. It is expressed as the proportion of the value added of the secondary industry in GDP. Urbanization level (Town). Excessive urbanization may cause resource shortage and ecological environment problems, forming resource mismatch and economic development gaps between urban and rural areas. It is calculated by the proportion of urban population to the total population. Government intervention (Gov). Reasonable government intervention in the economy helps optimize resource allocation as well as improve efficiency, but excessive intervention will distort market signals, cause factors of production to flow into enterprises with lower productivity, and exacerbate resource mismatch. Measurement using fiscal expenditure as a share of GDP. Level of financial development (Fina). Financial and credit policies and levels can guide the flow of funds, thus affecting the allocation of capital factors. It is measured by the sum of year-end deposit and loan balances of financial institutions as a share of GDP.
The research samples for this study include data from 2007 to 2021, covering 257 prefecture-level and above cities in China. Data sources include the China Urban Statistical Yearbook, the CNRDS database, the CSMAR database, and annual reports of listed companies, which were obtained via Python web scraping and analyzed using text analysis techniques. Missing values were imputed using linear interpolation, nominal variables were adjusted to 2007 prices based on relevant price indices, and continuous variables were winsorized at the 1% and 99% levels.
It is observed from Table 1 that the mean of regional resource allocation efficiency (Eff_K) is 0.267, accompanied by a standard deviation of 0.804, and the means of substantive green innovation (GreenIno_apply) and strategic green innovation (GreenFm_apply) are 0.527 and 0.543, with a standard deviation of 1.007 and 0.869, indicating that there is a large variation in resource allocation efficiency and green innovation levels among different cities. The mean of the digital economy development index (Dig) is 0.102, with a range of 0.018–0.290, suggesting varying levels of progress across different regions.
The results of the benchmark regression and quantile regression are reported in Table 2. Column (1) shows that the coefficient of Dig is positive at a significance level of 1%, confirming the facilitating effect of the digital economy on regional resource allocation efficiency. Column (2) demonstrates that this positive relationship remains significant even after controlling for fixed effects, supporting Hypothesis 1. Further, in order to investigate the evolutionary characteristics of the marginal effect of the digital economy on resource allocation efficiency, we apply a panel quantile model and report results for five quantiles in columns (3) to (7). We find that while there is no significant impact when Eff_K is low (q0.1), as it increases from 0.25 to 0.9 quantiles, so does the positive influence of Dig on resource allocation efficiency - an evolutionary trend similar to Matthew’s effect. This suggests that cities with competitive advantages are likely to gain more opportunities and resources as digitalization deepens, while those in less advantageous positions may encounter greater challenges in accessing them (Dian et al., 2024). Therefore, as the digital economy continues to advance, the difference in regional resource allocation efficiency will further expand, leading to an amplification of the Matthew effect. Hypothesis 2 is valid.
Given that both the explained and explanatory variables in this paper are city-level data, the baseline model may face two endogeneity issues. First, the problem of omitted variables arises, as some factors that influence both the digital economy and regional resource allocation efficiency may be unaccounted for. Second, there is the issue of reverse causality, suggesting that cities with high resource allocation efficiency may hold an advantage in developing the digital economy. An instrumental variable (IV) method is employed to mitigate endogeneity. Drawing on Lewbel’s (1997) heteroskedasticity-based IV construction method, the cube of the difference between Dig and the industry-province mean value of Dig is used as the IV (Dig_3), which is estimated using the two-stage least squares (2SLS) method. This choice of IV is justified as follows: in terms of relevance, Dig_3 is strongly correlated with Dig since it captures individual-specific deviations from regional and industry averages, amplified through the cube transformation; in terms of exogeneity, the deviation from regional means removes the influence of common shocks or unobserved factors that may directly affect the dependent variable, ensuring that Dig_3 affects regional resource allocation efficiency only through Dig. The IV test results can be shown in columns (1) and (2) of Table 3. In the first-stage regression, the coefficient of Dig_3 is highly significant and positive. Similarly, the second-stage regression reveals a significantly positive coefficient for Dig. Furthermore, both the underidentification test and weak identification test have been successfully passed, suggesting that Dig_3 is reasonable and valid. The regression findings demonstrate that the conclusion regarding the favorable influence of the digital economy on enhancing resource allocation efficiency remains robust.
For a prosperous digital economy, China has formulated its first pilot policy on the digital economy - the “National Comprehensive Experimental Zone for Big Data” (hereinafter referred to as the “experimental zone”). In February 2016, Guizhou was approved as the inaugural experimental zone, followed by Beijing, Tianjin, Hebei, Inner Mongolia, Liaoning, Henan, Shanghai, Chongqing and Guangdong in October of the same year. We leverage the establishment of these experimental zones as quasi-natural experiments and apply a difference-in-difference (DID) model to evaluate the impact of digital economy development on regional resource allocation efficiency from the perspective of an exogenous policy shock. The specific model setting is outlined below, as shown in Equation 6:
In the formula, Treatc is a dummy variable for whether city c belongs to the “experimental zone”, with a value of 1 for yes and 0 for no. Postt is a dummy variable for the period surrounding the implementation of the “experimental zone” policy, taking a value of 0 for years before 2016 and 1 for 2016 and onward.
The results of the DID regression are presented in column (3) of Table 3, where the coefficient of Treat*Post is significantly positive, suggesting that the “experimental zone” policy has enhanced resource allocation efficiency in the pilot areas (The results of equilibrium trend test and placebo test are shown in Supplementary Appendixs A.3, A.4). However, it is worth mentioning that the selection of the pilot cities was not entirely random. Factors like economic status, geographic location, and resource endowment were taken into account during policy formulation. These inherent differences may have varying impacts on factor allocation within cities over time, potentially invalidating our hypothesis identification. To control for inherent city characteristics’ effect on resource allocation efficiency, we add a cross-multiplier term between the degree of terrain relief and the time trend (Rdls), and a cross-multiplier term between the spherical distance of each city from Hangzhou and the time trend (Distance) to the control variables, which mitigates the estimation bias due to non-randomization in the selection of experimental groups to some extent. Furthermore, China designated a total of 120 cities as “Broadband China” demonstration cities in three batches between 2014-2016. These selected cities made concerted efforts to increase broadband user scale, enhance network speed, and expand coverage area - laying a solid foundation for digital infrastructure construction. There is some level of similarity between the cities involved in the “Broadband China” demonstration and the “experimental zone” analyzed in this study, which may have an influence on our regression results. Therefore, we include a dummy variable representing the “Broadband China” policy (Broadband) in Equation 6 to prevent interference. According to the results in columns (4) and (5) of Table 3, even after taking into account the non-randomized factors of pilot cities and the impact of contemporaneous policies, Treat*Post still shows a significant positive coefficient, indicating that the DID regression finding is robust.
(1) Replacement of the explained variable. Replace Eff_K with Eff_L and Eff_K_LP for regression, respectively. (2) Replacement of the explanatory variable. Replace Dig with Dig_words for regression. (3) Lag regression. Considering the possible lagged effect of digital economy, Dig is regressed again after one period of lagging. (4) Excluding the impact of epidemic. The COVID-19 pandemic has accelerated the progress of the digital economy (Rupeika-Apoga et al., 2022), disregarding the impact of the pandemic is likely to undermine the empirical findings. To mitigate this impact, we exclude data from 2020 to 2021, adjusting the sample period to 2007–2019 before conducting the regression. (5) Excluding municipalities. Compared with other cities, municipalities enjoy greater rights in administrative management and resource allocation, so we perform a repeated regression test after excluding the municipalities in the sample. The results, presented in Table 4, indicate that the coefficients of the core explanatory variable remain significantly positive across all tests, confirming the reliability of the findings in this study.
According to the previous theoretical analysis, green innovation serves as an indirect mechanism through which the resource allocation effect of the digital economy can be realized. We employ Equation 3 to empirically regress and verify the existence of this mechanism. The coefficients of the cross-multiplier terms between Dig and substantive green innovation in columns (1) and (3) of Table 5 are significantly positive; similarly, the results in columns (2) and (4) demonstrate that the coefficients of the cross-multiplier terms between Dig and strategic green innovation pass the significance test of at least 5%. These findings indicate that both substantive green innovation and strategic green innovation play a bridging role between the digital economy and regional resource allocation efficiency, thereby confirming the presence of green innovation mechanisms. Through the promotion of green innovation, the digital economy reduces resource consumption and environmental pollution, enabling more reasonable and effective utilization of resources, ultimately optimizing resource allocation. This establishes a positive pathway of influence from “digital economy - green innovation–regional resource allocation efficiency”, thus validating Hypothesis 3.
Based on the region of the city, the sample is divided into two types: eastern and mid-western1. As seen in Table 6, the coefficients of the cross-multiplier terms reveal that both substantive and strategic green innovation mechanisms demonstrate statistical significance in the eastern regions, while only substantive green innovation mechanism passes the significance test in the mid-western regions. As previously mentioned, substantive green innovation often requires high levels of technology and sustained R&D investment. The eastern region is more probable to carry out substantive green innovation and optimize resource allocation through such innovation due to its advanced economic development, abundant talent reserves, and well-developed industrial system, as well as the fact that the digital economy possesses a greater advantage in facilitating technological R&D, information sharing, and market expansion (Ma and Zhu, 2022). Strategic green innovation focuses on the quantity and short-term benefits of innovation, and this type of innovation is frequently employed by firms as a way to cater to investors and government regulation (Jiang and Bai, 2022). In the eastern regions, firms may adopt strategic green innovations to meet market and regulatory requirements in order to differentiate themselves from intense competition. However, the digital economy in the mid-western regions primarily focuses on addressing local practical challenges such as enhancing production efficiency and reducing logistics costs due to comparatively lower economic development levels, brain drain concerns, and a single industry structure. Therefore, the impact of the digital economy in these regions mainly lies in promoting substantial green innovation for resource allocation efficiency enhancement. This is because substantial green innovation places greater emphasis on technological R&D as well as product innovation, aligning more closely with the actual needs of the mid-western regions. Hypothesis 4 is confirmed.
Referring to Chen et al. (2018), we use the frequency of environment-related terms in each city’s Government Work Report as a proxy for environmental regulation (ER). To construct this measure, we manually collected, organized, and counted the frequency of key environment-related terms in local government work reports. These terms included “environmental protection,” “pollution control,” “energy consumption,” “emission reduction,” “waste discharge,” “ecology,” “green,” “low carbon,” “air quality,” “chemical oxygen demand,” “sulfur dioxide,” “carbon dioxide,” “PM10,” and “PM2.5.” Based on the median frequency, cities are divided into strong and weak ER groups for subgroup analysis. Results in Table 7 show that, in cities with strong ER, the interaction terms between substantive and strategic green innovation and Dig are both positive and statistically significant at the 1% level. This indicates that the digital economy can promote green innovation, enhancing factor allocation in cities with strong ER. Conversely, in cities with weak ER, the coefficients of these interaction terms are not significant, suggesting that green innovation does not mediate the impact of the digital economy on resource allocation efficiency in these regions. Strong ER enforces higher environmental standards and stricter emission limits, motivating firms to allocate more resources to green innovation. Additionally, the digital economy offers technical support and platforms that help firms achieve green transformation, which not only boosts firm competitiveness but also improves regional resource allocation efficiency (Qiu et al., 2023). In contrast, firms in weak ER regions face less pressure and incentive for green innovation (Ambec and Barla, 2002). Even with access to digital technologies and platforms, these firms may undervalue green innovation due to short-term considerations or lack of external incentives, limiting the digital economy’s role in advancing regional resource allocation efficiency. These findings support Hypothesis 5.
This study empirically examines how the digital economy influences regional resource allocation efficiency, focusing on 257 prefecture-level and higher cities in China from 2007 to 2021. It specifically investigates the impact pathway from the perspective of green innovation. The key findings are as follows: First, the digital economy significantly enhances resource allocation efficiency. However, as it develops further, disparities in allocation efficiency widen, exhibiting a non-linear trend with increasing marginal effects. This suggests a “Matthew effect” within regional resource allocation systems. Second, mechanism tests indicate that the digital economy improves resource allocation by fostering green innovation. This confirms a transmission pathway of “digital economy - green innovation - regional resource allocation efficiency.” Third, regional heterogeneity analysis reveals that in eastern regions, both substantive and strategic green innovation act as critical pathways for the digital economy’s influence on resource allocation. In contrast, in mid-western regions, this effect is primarily driven by substantive green innovation. Finally, the analysis of environmental regulation heterogeneity shows that in cities with strong environmental regulations, the digital economy promotes both substantive and strategic green innovation, thereby enhancing resource allocation efficiency. Conversely, in cities with weak environmental regulations, neither form of green innovation serves as a significant transmission mechanism.
Based on the findings, we propose the following policy recommendations: First, the government should emphasize the digital economy’s role in enhancing regional resource allocation efficiency. This includes providing additional support through favorable policies and reducing barriers and costs associated with digital transformation for enterprises. These measures will encourage broader digital adoption, boost productivity, and optimize resource allocation. Second, while the digital economy improves resource allocation efficiency, it has also exacerbated regional disparities. To address this issue, the government should enhance support for mid-western regions by promoting digital innovation and improving resource utilization efficiency. Meanwhile, the eastern region should be encouraged to leverage its digital economy advantages as a model for national development. Strengthening interregional information exchange and technical collaboration can dismantle barriers, promote rational resource distribution, and facilitate the flow of resources across regions. Third, both substantive and strategic green innovations are critical for efficient resource allocation, particularly given global resource scarcity and worsening environmental challenges. The government should prioritize green innovation by implementing policies such as establishing dedicated funding mechanisms, promoting technical cooperation and international exchanges, training specialized personnel, and creating robust incentive systems. Further, integrating the digital economy with green development should be a priority. Leveraging digital technologies for intelligent environmental management and energy conservation can foster a mutually beneficial relationship between economic growth and environmental resilience. Finally, regional differences in environmental regulations lead to varying impacts of green innovation mechanisms. Policies should be locally adapted to stimulate green innovation effectively. In cities with strong environmental regulations, policy incentives should encourage enterprises to engage in deeper green innovation. For instance, preferential support could be extended to firms adopting advanced environmental technologies, promoting technological and industrial upgrading. Conversely, in cities with weaker regulations, efforts should focus on strengthening these regulations and improving enforcement.
This study contributes theoretically by clarifying how the digital economy influences regional resource allocation efficiency through green innovation. It enriches the existing framework on the digital economy and resource distribution by considering regional and regulatory heterogeneity. Practically, it provides policy insights by emphasizing the role of the digital economy in optimizing resource allocation and advocating support for green innovation and digital transformation to promote balanced regional development and sustainability. However, this study has certain limitations. First, as the analysis focuses on Chinese cities, the findings may not fully generalize to other countries or regions with different levels of digitalization and regulatory environments. Future research could extend this framework to other regions to explore how local conditions shape the digital economy’s impact on resource allocation. Second, while green innovation is identified as the primary mechanism in this study, this perspective may be insufficient to capture the full complexity of the relationship. Future research could investigate additional mechanisms, such as regional innovation collaborations or improvements in digital infrastructure, to provide a more comprehensive understanding of how the digital economy enhances resource efficiency.
The original contributions presented in the study are included in the article/Supplementary Material, further inquiries can be directed to the corresponding author.
LJ: Conceptualization, Formal Analysis, Investigation, Project administration, Software, Writing–original draft. MZ: Supervision, Validation, Writing–review and editing. SH: Data curation, Methodology, Writing–review and editing.
The author(s) declare that financial support was received for the research, authorship, and/or publication of this article. This work was supported by the Major Research Project of Philosophy and Social Sciences of the Ministry of Education of China (No. 22JZD042).
The authors declare that the research was conducted in the absence of any commercial or financial relationships that could be construed as a potential conflict of interest.
The author(s) declare that no Generative AI was used in the creation of this manuscript.
All claims expressed in this article are solely those of the authors and do not necessarily represent those of their affiliated organizations, or those of the publisher, the editors and the reviewers. Any product that may be evaluated in this article, or claim that may be made by its manufacturer, is not guaranteed or endorsed by the publisher.
The Supplementary Material for this article can be found online at: https://www.frontiersin.org/articles/10.3389/fenvs.2025.1529946/full#supplementary-material
1The eastern region includes Jiangsu, Shanghai, Zhejiang, Fujian, Guangdong, Shandong, Hainan, Liaoning, Jilin, Heilongjiang, Hebei, Beijing, and Tianjin. The central and western regions include Henan, Hubei, Hunan, Shanxi, Jiangxi, Anhui, Inner Mongolia, Ningxia, Guangxi, Xinjiang, Chongqing, Sichuan, Guizhou, Yunnan, Shaanxi, Gansu, and Qinghai. Tibet is not included in the data for this study.
Ambec, S., and Barla, P. (2002). A theoretical foundation of the Porter hypothesis. Econ. Lett. 75 (3), 355–360. doi:10.1016/S0165-1765(02)00005-8
Bai, D., Li, M., Wang, Y., Mallek, S., and Shahzad, U. (2024). Impact mechanisms and spatial and temporal evolution of digital economy and green innovation: a perspective based on regional collaboration within urban agglomerations. Technol. Forecast. Soc. Change 207, 123613. doi:10.1016/j.techfore.2024.123613
Bel, G., and Joseph, S. (2018). Policy stringency under the European Union Emission trading system and its impact on technological change in the energy sector. Energy Policy 117, 434–444. doi:10.1016/j.enpol.2018.03.041
Bian, Y., Song, K., and Bai, J. (2019). Market segmentation, resource misallocation and environmental pollution. J. Clean. Prod. 228, 376–387. doi:10.1016/j.jclepro.2019.04.286
Cao, C., and Su, Y. (2024). Transportation infrastructure and regional resource allocation. Cities 155, 105433. doi:10.1016/j.cities.2024.105433
Carlsson, B. (2004). The Digital Economy: what is new and what is not? Struct. change Econ. Dyn. 15 (3), 245–264. doi:10.1016/j.strueco.2004.02.001
Chen, J., and Chen, Y. (2024). The impact of digitalization on the efficiency of innovation factor allocation: from the perspective of factor flow. Appl. Econ., 1–17. doi:10.1080/00036846.2024.2337817
Chen, J., and Zhou, Q. (2017). City size and urban labor productivity in China: new evidence from spatial city-level panel data analysis. Econ. Syst. 41 (2), 165–178. doi:10.1016/j.ecosys.2016.07.002
Chen, W., Du, X., Lan, W., Wu, W., and Zhao, M. (2023). How can digital economy development empower high-quality economic development? Technol. Econ. Dev. Econ. 29 (4), 1168–1194. doi:10.3846/tede.2023.18784
Chen, Z., Kahn, M. E., Liu, Y., and Wang, Z. (2018). The consequences of spatially differentiated water pollution regulation in China. J. Environ. Econ. Manag. 88, 468–485. doi:10.1016/j.jeem.2018.01.010
Clarkson, P. M., Li, Y., and Richardson, G. D. (2004). The market valuation of environmental capital expenditures by pulp and paper companies. Account. Rev. 79 (2), 329–353. doi:10.2308/accr.2004.79.2.329
Colombo, E., Mercorio, F., and Mezzanzanica, M. (2019). AI meets labor market: exploring the link between automation and skills. Inf. Econ. Policy 47, 27–37. doi:10.1016/j.infoecopol.2019.05.003
Dai, D., Fan, Y., Wang, G., and Xie, J. (2022). Digital economy, R&D investment, and regional green innovation-analysis based on provincial panel data in China. Sustainability 14 (11), 6508. doi:10.3390/su14116508
Dian, J., Song, T., and Li, S. (2024). Facilitating or inhibiting? Spatial effects of the digital economy affecting urban green technology innovation. Energy Econ. 129, 107223. doi:10.1016/j.eneco.2023.107223
Dong, X., Guo, K., and Zhao, X. (2024). Does digital economy promote regional green innovation? An empirical study based on the transmission effect and threshold effect of marketization. J. Digital Econ. 3, 47–61. doi:10.1016/j.jdec.2024.07.002
Ge, Y., Xia, Y., and Wang, T. (2024). Digital economy, data resources and enterprise green technology innovation: evidence from A-listed Chinese Firms. Resour. Policy 92, 105035. doi:10.1016/j.resourpol.2024.105035
Gebauer, H., Fleisch, E., Lamprecht, C., and Wortmann, F. (2020). Growth paths for overcoming the digitalization paradox. Bus. Horizons 63 (3), 313–323. doi:10.1016/j.bushor.2020.01.005
Ghasemaghaei, M., and Calic, G. (2020). Assessing the impact of big data on firm innovation performance: big data is not always better data. J. Bus. Res. 108, 147–162. doi:10.1016/j.jbusres.2019.09.062
Goldfarb, A., and Tucker, C. (2019). Digital economics. J. Econ. literature 57 (1), 3–43. doi:10.1257/jel.20171452
Guo, B., Wang, Y., Zhang, H., Liang, C., Feng, Y., and Hu, F. (2023). Impact of the digital economy on high-quality urban economic development: evidence from Chinese cities. Econ. Model. 120, 106194. doi:10.1016/j.econmod.2023.106194
Hao, X., Wen, S., Xue, Y., Wu, H., and Hao, Y. (2023). How to improve environment, resources and economic efficiency in the digital era? Resour. Policy 80, 103198. doi:10.1016/j.resourpol.2022.103198
Hao, Y., Gai, Z., and Wu, H. (2020). How do resource misallocation and government corruption affect green total factor energy efficiency? Evidence from China. Energy Policy 143, 111562. doi:10.1016/j.enpol.2020.111562
Jiang, L., and Bai, Y. (2022). Strategic or substantive innovation? —the impact of institutional investors’ site visits on green innovation evidence from China. Technol. Soc. 68, 101904. doi:10.1016/j.techsoc.2022.101904
Jiang, Q., Li, Y., and Si, H. (2022). Digital economy development and the urban–rural income gap: intensifying or reducing. Land 11 (11), 1980. doi:10.3390/land11111980
Lewbel, A. (1997). Constructing instruments for regressions with measurement error when no additional data are available, with an application to patents and R&D. Econometrica 65 (5), 1201–1213. doi:10.2307/2171884
Li, C., Liu, J., Liu, Y., and Wang, X. (2023). Can digitalization empowerment improve the efficiency of corporate capital allocation? —evidence from China. Econ. Analysis Policy 80, 1794–1810. doi:10.1016/j.eap.2023.11.014
Li, F., Nucciarelli, A., Roden, S., and Graham, G. (2016). How smart cities transform operations models: a new research agenda for operations management in the digital economy. Prod. Plan. and Control 27 (6), 514–528. doi:10.1080/09537287.2016.1147096
Li, Z., and Wang, J. (2022). The dynamic impact of digital economy on carbon emission reduction: evidence city-level empirical data in China. J. Clean. Prod. 351, 131570. doi:10.1016/j.jclepro.2022.131570
Lu, J., and Li, H. (2024). The impact of ESG ratings on low carbon investment: evidence from renewable energy companies. Renew. Energy 223, 119984. doi:10.1016/j.renene.2024.119984
Luo, S., Yimamu, N., Li, Y., Wu, H., Irfan, M., and Hao, Y. (2023). Digitalization and sustainable development: how could digital economy development improve green innovation in China? Bus. Strategy Environ. 32 (4), 1847–1871. doi:10.1002/bse.3223
Lyu, Y., Wang, W., Wu, Y., and Zhang, J. (2023). How does digital economy affect green total factor productivity? Evidence from China. Sci. Total Environ. 857, 159428. doi:10.1016/j.scitotenv.2022.159428
Ma, D., and Zhu, Q. (2022). Innovation in emerging economies: research on the digital economy driving high-quality green development. J. Bus. Res. 145, 801–813. doi:10.1016/j.jbusres.2022.03.041
Mariscal, J. (2005). Digital divide in a developing country. Telecommun. policy 29 (5-6), 409–428. doi:10.1016/j.telpol.2005.03.004
Pan, W., Xie, T., Wang, Z., and Ma, L. (2022). Digital economy: an innovation driver for total factor productivity. J. Bus. Res. 139, 303–311. doi:10.1016/j.jbusres.2021.09.061
Porter, M. E., and Linde, C. V. D. (1995). Toward a new conception of the environment-competitiveness relationship. J. Econ. Perspect. 9 (4), 97–118. doi:10.1257/jep.9.4.97
Qiu, Y., Liu, W., and Wu, J. (2023). Digital economy and urban green innovation: from the perspective of environmental regulation. J. Environ. Plan. Manag. 68, 267–289. doi:10.1080/09640568.2023.2244668
Ren, S., Li, L., Han, Y., Hao, Y., and Wu, H. (2022). The emerging driving force of inclusive green growth: does digital economy agglomeration work? Bus. Strategy Environ. 31 (4), 1656–1678. doi:10.1002/bse.2975
Rupeika-Apoga, R., Petrovska, K., and Bule, L. (2022). The effect of digital orientation and digital capability on digital transformation of SMEs during the COVID-19 pandemic. J. Theor. Appl. Electron. Commer. Res. 17 (2), 669–685. doi:10.3390/jtaer17020035
Shen, Y., and Zhang, X. (2023). Intelligent manufacturing, green technological innovation and environmental pollution. J. Innovation and Knowl. 8 (3), 100384. doi:10.1016/j.jik.2023.100384
Sturgeon, T. J. (2021). Upgrading strategies for the digital economy. Glob. strategy J. 11 (1), 34–57. doi:10.1002/gsj.1364
Tang, C., and Veelenturf, L. P. (2019). The strategic role of logistics in the industry 4.0 era. Transp. Res. Part E Logist. Transp. Rev. 129, 1–11. doi:10.1016/j.tre.2019.06.004
Tian, K., Zhai, D., and Han, S. (2024). Impact of carbon emission trading on urban green innovation: empirical evidence from China’s carbon emission trading pilot policy. Front. Environ. Sci. 12, 1419720. doi:10.3389/fenvs.2024.1419720
Wang, Q., and Cao, P. (2023). Evaluation and prediction on resource misallocation of energy industry in China. Environ. Dev. Sustain., 1–18. doi:10.1007/s10668-023-04265-y
Wang, X., Qin, C., Liu, Y., Tanasescu, C., and Bao, J. (2023). Emerging enablers of green low-carbon development: do digital economy and open innovation matter? Energy Econ. 127, 107065. doi:10.1016/j.eneco.2023.107065
Wei, Q., Liu, H., and Pan, F. (2022). Digital economy, industry heterogeneity, and service industry resource allocation. Sustainability 14 (13), 8020. doi:10.3390/su14138020
Williams, L. D. (2021). Concepts of digital economy and industry 4.0 in intelligent and information systems. Int. J. Intelligent Netw. 2, 122–129. doi:10.1016/j.ijin.2021.09.002
Wu, H. X., and Yu, C. (2022). The impact of the digital economy on China’s economic growth and productivity performance. China Econ. J. 15 (2), 153–170. doi:10.1080/17538963.2022.2067689
Wu, J., Guo, S., Li, J., and Zeng, D. (2016). Big data meet green challenges: big data toward green applications. IEEE Syst. J. 10 (3), 888–900. doi:10.1109/JSYST.2016.2550530
Xu, Y., Li, C., Wang, X., and Wang, J. (2023). Digitalization, resource misallocation and low-carbon agricultural production: evidence from China. Front. Environ. Sci. 11, 1117086. doi:10.3389/fenvs.2023.1117086
Yang, C., Huang, Q., Li, Z., Liu, K., and Hu, F. (2017). Big data and cloud computing: innovation opportunities and challenges. Int. J. Digital Earth 10 (1), 13–53. doi:10.1080/17538947.2016.1239771
Yang, F., Yuan, H., and Yi, N. (2022). Natural resources, environment and the sustainable development. Urban Clim. 42, 101111. doi:10.1016/j.uclim.2022.101111
Yang, J., Li, X., and Huang, S. (2020). Impacts on environmental quality and required environmental regulation adjustments: a perspective of directed technical change driven by big data. J. Clean. Prod. 275, 124126. doi:10.1016/j.jclepro.2020.124126
Yu, L., Ma, T., Wu, S., and Lyu, Z. (2023). How does broadband internet affect firm-level labor misallocation: the role of information frictions. China Econ. Rev. 82, 102067. doi:10.1016/j.chieco.2023.102067
Yuan, S., and Pan, X. (2023). Inherent mechanism of digital technology application empowered corporate green innovation: based on resource allocation perspective. J. Environ. Manag. 345, 118841. doi:10.1016/j.jenvman.2023.118841
Zhang, Y. (2024). A study on the correlation between the digital economy and resource allocation efficiency in the context of factor mobility. Appl. Math. Nonlinear Sci. 9 (1). doi:10.2478/amns-2024-1056
Zhang, Y., and Wang, J. (2023). Research on the impact of digital economy on resource misallocation: mechanism and empirical evidence. Contemp. Econ. Manag. 45 (11), 32–45. doi:10.13253/j.cnki.ddjjgl.2023.11.005
Zhao, X., Shang, Y., Magazzino, C., Madaleno, M., and Mallek, S. (2023). Multi-step impacts of environmental regulations on green economic growth: evidence in the lens of natural resource dependence. Resour. Policy 85, 103919. doi:10.1016/j.resourpol.2023.103919
Zhao, X., and Sun, B. (2016). The influence of Chinese environmental regulation on corporation innovation and competitiveness. J. Clean. Prod. 112, 1528–1536. doi:10.1016/j.jclepro.2015.05.029
Zhao, Y., Zheng, Y., and Fang, Z. (2024). The effects of digital economy on manufacturing resource allocation efficiency across Chinese cities: a spatial economic perspective. Appl. Econ., 1–16. doi:10.1080/00036846.2024.2423904
Keywords: digital economy, resource allocation efficiency, substantive green innovation, strategic green innovation, environmental regulation, green development
Citation: Jiang L, Zhang M and Hu S (2025) Digital economy, green innovation and regional resource allocation efficiency: evidence from 257 cities in China. Front. Environ. Sci. 13:1529946. doi: 10.3389/fenvs.2025.1529946
Received: 18 November 2024; Accepted: 02 January 2025;
Published: 17 January 2025.
Edited by:
Juan Lu, Nanjing Agricultural University, ChinaReviewed by:
He Li, Nanjing University of Posts and Telecommunications, ChinaCopyright © 2025 Jiang, Zhang and Hu. This is an open-access article distributed under the terms of the Creative Commons Attribution License (CC BY). The use, distribution or reproduction in other forums is permitted, provided the original author(s) and the copyright owner(s) are credited and that the original publication in this journal is cited, in accordance with accepted academic practice. No use, distribution or reproduction is permitted which does not comply with these terms.
*Correspondence: Shihui Hu, YmFieTY2NTI5QDE2My5jb20=
Disclaimer: All claims expressed in this article are solely those of the authors and do not necessarily represent those of their affiliated organizations, or those of the publisher, the editors and the reviewers. Any product that may be evaluated in this article or claim that may be made by its manufacturer is not guaranteed or endorsed by the publisher.
Research integrity at Frontiers
Learn more about the work of our research integrity team to safeguard the quality of each article we publish.