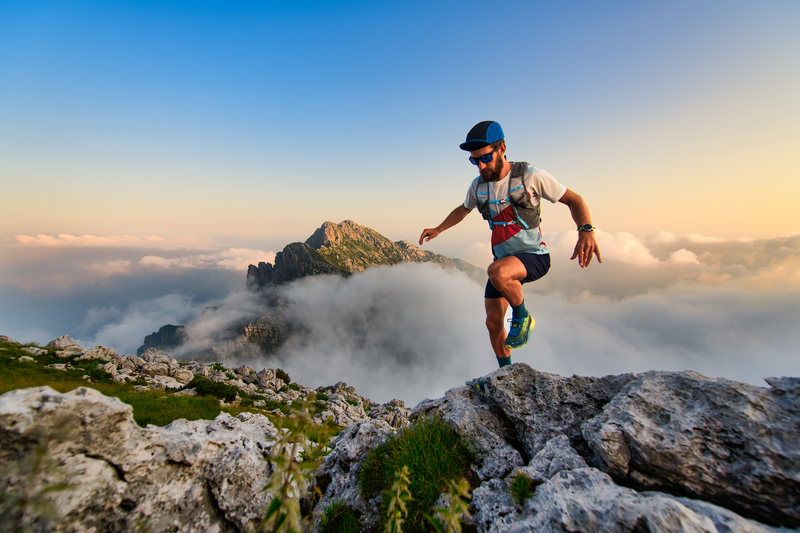
95% of researchers rate our articles as excellent or good
Learn more about the work of our research integrity team to safeguard the quality of each article we publish.
Find out more
ORIGINAL RESEARCH article
Front. Environ. Sci. , 24 January 2025
Sec. Freshwater Science
Volume 13 - 2025 | https://doi.org/10.3389/fenvs.2025.1524250
Nutrient pollution is a critical issue for lakes, leading to cultural eutrophication, which damages the ecosystem and prevents water uses. Proper watershed management practices must be put into action to effectively oppose eutrophication. Because of the complexities and the multiple layers of stakeholders involved in this process, the only factual way to evaluate improvements in watershed nutrient management is to directly estimate nutrient loads at watershed closing sections through available data from environmental monitoring. The Italian deep subalpine lakes (DSLs: Lake Como, Lake Garda, Lake Idro, Lake Iseo, Lake Maggiore) suffered from extensive eutrophication from the 1950s to the early 1980s. Their water quality improved in the 1980s and 1990s after national regulations were introduced to address nutrient pollution. After the turn of the century, increasingly pressing limits on nutrient disposal have been issued, yet steady if not worsening lake water quality conditions have been observed overall. To shed light on the issue, quantifying present and past external loads to these lakes based on direct measurements is essential. In this work, we focused on the main tributaries of the Italian DSLs, employing the observed time series of flowrate and nutrient concentrations to estimate the loading levels of nitrogen and phosphorus and their temporal evolution over a period ranging between 13 and 42 years according to data availability. The LOAD ESTimator (LOADEST) software was adopted to support the load quantification process. Results show that a general decrease in external nutrient loads is still occurring, albeit at a slow pace, the fastest reduction occurring for the Oglio River flowing into Lake Iseo, which has suffered from severe nutrient pollution till recently. Increasing loads have conversely been identified for the Sarca River flowing into Lake Garda. These outcomes highlight the beneficial actions carried out to reduce nutrient pollution, although a decorrelation analysis between external loads and flowrate is suggested for a clearer evaluation of its evolution across the DSLs.
The quality of large lakes has been considered with increasing concern by several communities around the world in the last decades (Jeppesen et al., 2005). In addition to pollution on both the lake surface and its catchment, climate change and uncontrolled water abstraction contribute to endangering the water balance and the entire ecosystems (Benejam et al., 2010; Bastanov et al., 2021). As a matter of fact, the water stored in lakes and their catchments represents a precious resource for human activities, such as agriculture, industry and civil uses (Payganeh et al., 2018). During the past decades, many surface freshwater resources have been jeopardized, the ensuing effects of water usage and pollution (Kumar and Anshumali, 2019; Ramadan and Sembiring, 2020) highlighting the necessity to preserve the availability of these precious resources.
Nutrient excess is the most common form of pollution which affects lakes (Davidson et al., 2011). Phosphorus (P) and nitrogen (N) are naturally present in water environments, being essential for algal growth and food-chain supply (Fong et al., 2003). However, increased concentrations can affect ecosystem balance and lead to critical water quality conditions. Urban sewage releases (Yang et al., 2023), agricultural emissions (Noll and Magee, 2009) and enhanced atmospheric depositions due to air pollution (Rogora et al., 2001) are the main factors contributing to nutrient excess, enhancing the possibility to trigger the eutrophication process (Hutchinson, 1973). The focus on lake eutrophication has further increased recently due to the upsurge of lake-water use for drinking purposes (Mezzanotte et al., 2011).
Input loads of nutrients into lakes from their catchments can originate either from diffuse riparian sources, such as rural nearshore areas, or from point sources, such as tributaries, wastewater-treatment plants or combined-sewer overflows (CSOs). Point inputs from tributaries collecting entire catchments represent by far the most relevant contribution for large lakes, the water conditions of these water bodies thus depending on the human activities over the whole watershed (Matzinger et al., 2006; Stackpoole et al., 2021; Yates et al., 2022; Yu et al., 2023). For this reason, regulations regarding urban and industrial sewage collection, farming and livestock breeding activities must be applied over the whole catchment to enhance lake water quality. This involves procedures that connect the network of actors, including both governmental proactive directives and local reactive actions to implement practical countermeasures, with the aim of enhancing water quality (Hill, 2013).
However, the complexity of these processes makes monitoring surveys of both lakes and their tributaries essential for effectively evaluating the success of watershed management efforts. This approach allows establishing a baseline and assessing changes in nutrient loads.
The Deep Subalpine Lakes of Northen Italy (DSLs: Salmaso et al., 2007) (in this study: Lake Como, Lake Garda, Lake Idro, Lake Iseo, Lake Maggiore), south of the Alps, are a relevant freshwater resource for multiple uses, endangered by pollution and climate change effects. They all suffered from severe eutrophication from the 1950s to the early 1980s. This pushed forward the activation of extensive countermeasures to limit the phenomenon (Rogora et al., 2018). This included the reduction of P in detergents, the activation of wastewater treatment plants and the improvement of sewer collection (Copetti et al., 2019). Different countermeasure efficiencies and implementation success have been obtained across lake watersheds, depending on anthropic pressures and intervention extents. The sensible improvement of lake water quality stopped in the early 2000s, after which steady if not slightly worsening water quality conditions were observed overall, also due to the effects of climate warming (Salmaso et al., 2007; Salmaso and Mosello, 2010; Rogora et al., 2018). This has been occurring despite the issuing of increasingly limiting nutrient emission regulations (Copetti and Erba, 2024). Many studies have addressed the re-oligotrophication path of the DSLs based on time series of in-lake nutrient concentrations (Mosello et al., 2001; Rogora et al., 2003; Salmaso and Mosello, 2010), also involving considerations on the existing nutrient sources in the catchments. However, the direct quantification of nutrient loads from the watersheds and lake main tributaries (MTs), including evaluation on their evolution, is a much less explored subject. This is due to the general lack of extensive monitoring campaigns on the tributaries of the DSLs in the past.
Therefore, in this study, an estimation of present and past P and N loads released by the MTs of the DSLs and of their evolution has been produced. The work focuses on the MTs for two reasons: 1) the catchments of the MTs cover a considerable part of the watersheds of the DSLs, making the quantification of their loads strongly significant for overall lake-scale evaluations (Nava et al., 2020; Valerio et al., 2022); 2) the monitoring activities prescribed by the Water Framework Directive 2000/60/EC of the European Commission (EU WFD, 2000)1 have resulted in a water quality database for the MTs with a minimum span of 15 years and a monthly resolution, suitable for scientific analyses, the same level of detail not being available for the minor tributaries (Fenocchi et al., 2023). This study is therefore a new step towards a comprehensive understanding of external nutrient loading across the DSLs, employing time series of nutrient loads long enough to draw comparisons among their catchments and make first evaluations on their evolution.
The Italian DSLs Lake Como, Lake Garda, Lake Idro, Lake Iseo and Lake Maggiore are a group of lakes of glacial origin at the southern slopes of the Alpine chain (Figure 1; Table 1). They are vital for the Alpine hydrological balance and for the water uses of the Po River plain in Northern Italy, one of the most anthropized areas in Europe. They collect extensive water runoff through large catchments, which include one or 2 MTs, whose watersheds cover most of the overall lake ones. The catchments of the MTs originate from small streams, mainly fed by nival and glacial fusion, which flow rapidly from high altitudes to low-sloping plains, where they combine into larger rivers which flow into the DSLs (Salmaso and Mosello, 2010; Terzi et al., 2021). The high-mountain areas where the MTs originate are pristine environments, whereas the downstream ones are made up of urban, industrial and rural areas.
Figure 1. Considered Italian Deep Subalpine Lakes (DSLs) and main tributaries (MTs): the catchments of the MTs are highlighted.
Table 1. Main hydrological and morphometric characteristics of the considered Deep Subalpine Lakes (DSLs) and hydrological features of their main tributaries (MTs) and their catchments (data from Salmaso and Mosello (2010); Dresti et al. (2021)).
The MTs considered in this study are the Adda River for Lake Como, the Sarca River for Lake Garda, the Toce River and the Ticino River for Lake Maggiore, the Chiese River for Lake Idro and the Oglio River for Lake Iseo (Figure 1; Table 1). Lake Lugano was not considered in the present study, despite being usually considered part of the DSLs (Salmaso and Mosello, 2010), because all its tributaries are small-sized, the largest one Creek Vedeggio having a mean discharge of 3.6 m3 s−1 in the 2004–2023 period (FOEN2), hence incomparable to the flowrate of the other MTs (Table 1).
As anticipated before, despite the high number of studies performed on the DSLs, direct experimental assessments of the input nutrient loads from their catchments have been scarce. A quantification of the total nutrient loads flowing into Lake Como in the 2000–2019 period was carried out by Fenocchi et al. (2023), adopting a regionalization procedure to estimate loads for minor unmonitored tributaries as well. For Lake Garda, the only study on nutrient load was undertaken by Decet and Salmaso (1997), encompassing only 14 months of sampling. Some considerations on nutrient mismanagement over the Chiese River catchment were published for chemically meromictic Lake Idro in Nizzoli et al. (2018) and Severini et al. (2023). Critical nutrient loading conditions also characterize Lake Iseo, the other chemically meromictic DSL, despite the recent efforts to reduce nutrient load inputs (Pilotti et al., 2021; Scibona et al., 2022; Valerio et al., 2022). In fact, nutrient inputs from the Oglio River over 2017–2018 were experimentally assessed by Valerio et al. (2022) determining that current loading levels are still too high. Last, studies on Lake Maggiore (Rogora et al., 2001; Rogora et al., 2003) determined that N concentrations in the lake and its tributaries are strongly associated with atmospheric deposition, this being related to the comparatively low anthropization of the catchment.
European national and regional authorities have focused on actions to reach higher water quality standards and reduce nutrient releases, conforming to the WFD3. Due to the monitoring and assessment requirements of the WFD itself, data series with a minimum duration of around 15 years and monthly resolution are available for nutrient concentrations in the MTs near their outlets into the DSLs, obtained from the analysis of water samples. Together with available daily discharge series in the same locations from gauging stations of the hydrological monitoring network (Supplementary Material S1), they allow studies on nutrient load quantification and evolution for the MTs.
Nutrient concentration and discharge series are available for Adda River and Oglio River from the local environmental protection agency ARPA Lombardia. The Oglio River is partially diverted 18 km upstream of its mouth to the Italsider industrial channel, which also flows into Lake Iseo with a comparable discharge to the residual one of the natural river, up to a 50 m3 s−1 hydraulic capacity threshold. Both the diverted discharge and the nutrient concentrations measured in such channel were considered, performing a weighted average of the resulting loads with those of the natural Oglio River at its mouth, for an overall assessment of the Oglio catchment. Daily discharges for the industrial channel Italsider were provided by Consorzio dell’Oglio4, whereas nutrient concentrations by ARPA Lombardia (yet sampled with quarterly frequency).
For the Sarca River and Chiese River, nutrient concentrations were provided by the local environmental protection agency APPA Trento5, whereas discharges are available from the Civil Protection Department of the Autonomous Province of Trento6.
For Lake Maggiore, monthly nutrient concentration sampling on the MTs Ticino River and Toce River started in 1981 within the framework of the International Commission for the Protection of Italian-Swiss Waters (CIPAIS7), work being performed by the Water Research Institute of the Italian National Research Council (CNR-IRSA) of Verbania, so that the available concentration series span more than 40 years. Daily discharges for the entire period were available for the Toce River from ARPA Piemonte, whereas for the Ticino River from the Swiss Federal Office for the Environment (FOEN). As both discharge and water quality of the Toce River are measured upstream of the junction with the Strona River, its largest tributary, which occurs 4 km upstream of Lake Maggiore, we also considered Strona River data for a weighted-average overall assessment of nutrient loads from the Toce catchment. Discharges and nutrient concentrations of the Strona River were obtained from ARPA Piemonte and CNR-IRSA, respectively.
The nutrient substances considered in this study are nitrate (N-NO3), ammonium (N-NH4) and total nitrogen (TN) for N, orthophosphate (P-PO4) and total phosphorus (TP) for P.
For this study, the LOAD ESTimator (LOADEST8) freeware software by the United States Geological Survey (USGS9) was employed to estimate daily nutrient load series from daily discharges and monthly concentration samples. LOADEST has been widely applied and validated over a multitude of case studies (e.g., Chen et al., 2015; Park and Engel, 2016; Yu et al., 2023; Song et al., 2024). This software infers daily load series through a regression analysis on input data, generating a model that fits them, and employing it to turn the available daily discharge series into a load series. LOADEST reports statistical indicators of the goodness-of-fit of the regression, as well as the regression parameters themselves.
The expression for observed load computation considered by LOADEST is reported in Equation 1, where Lτ is the measured transported load, τ is the considered integration period, equal to 1 day if daily discrete loads are produced, Q and C are the measured flowrate and the nutrient concentration, respectively.
LOADEST then applies the regression models on the series of discrete loads obtained from Equation 1, expressing them in the logarithmic form and considering the inferences of discharge, of seasonality and of temporal trends. LOADEST includes ten different fitting models, which include a variable portion of the terms on the right-hand-side of Equation 2 with increasing complexity. These span from the simplest model, which includes only the first two terms, leading to the well-known power-law dependence on discharge (Godsey et al., 2009), up to the most complicated possible regression model expressed by the full Equation 2.
As per Equation 2, through LOADEST dependence on discharge can be considered up to a quadratic regression, dependence on seasonality is expressed by the two terms including trigonometric functions, whereas the last two terms on the right-hand side of Equation 2 express possible temporal trends, up to a quadratic relationship. The variable dtime expresses time in a decimal form, the variable being centered on the midpoint of the considered time series.
LOADEST allows choosing among the ten different regression models, depending on the specific goal of the calibration, or it can select the best-performing model itself, according to the Akaike Information Criterion (AIC) (Mohammed et al., 2015). This last possibility was followed in this study, to obtain the most accurate possible load series. The software automatically computes the AIC value for all ten fitting models (which depends on likelihood, number of records and number of estimated parameters) and selects the one associated with the lowest AIC value. Depending on the features of the observed series, LOADEST selected different fitting models for different MTs and for different substances for the same MT, aiming at the best fit.
The Adjusted Maximum Likelihood Estimation (AMLE) method was herein selected to obtain the regression model parameters in LOADEST. The load estimation process was applied homogenously for all nutrient substances on all the MTs, obtaining daily series of nutrient loads starting from the daily discharge data series and adopting the obtained specific regression parameters.
Table 2 reports the mean and standard deviation, the maximum and the minimum annual discharges for the considered MTs, obtained from available time series. The annual flow variability which surfaces out of the statistics highlights the influence of interannual precipitation variation, resulting in an up to an order-of-magnitude difference between minimum and maximum annual values. This variability plays a fundamental role in nutrient delivery (Stackpoole et al., 2021; Yates et al., 2022).
As N and P concentrations can show either a dilution behavior (source-limited), a concentration behavior (transport-limited) or an uncertain behavior with discharge (Godsey et al., 2009; Fenocchi et al., 2023; Yu et al., 2023; Song et al., 2024), sampled concentration data series were analyzed in relation to the flowrates, to determine the specific Q-C (Flowrate–Concentration) behavior of each catchment for each considered nutrient substance (Table 3). The catchment of the Oglio River stands out as the only one in which the transport-limited concentration behavior is clearly observed from Q-C data. This is a signal of enduring nutrient pollution reaching the river waters during rainfall periods, being either excess fertilizers, resuspended sewer sediments, uncollected sewage or untreated sewage from the activation of CSOs, confirming the evaluations of Valerio et al. (2022).
Table 3. Q-C behavior of nutrient substances for the MTs in the available time series (DIL = clear dilution behavior; CONC = clear concentration behavior; NC = not clear on a visual interpretation).
An unclear behavior emerges for all nutrient substances for the Chiese River, potentially showing that nutrient pollution is still considerable on this catchment as well, as per the judgement of Nizzoli et al. (2018) and Severini et al. (2023). The other MTs instead display a clear dilution behavior, indicating a less dramatic anthropogenic nutrient pollution, with an essentially steady ordinary input being diluted by surface runoff in rainy periods.
As regards River Oglio and the industrial channel Italsider, nutrient concentration data sampled in the same day showed significant differences between the two streams (Supplementary Material S2). Such discrepancy should be attributed to different nutrient delivery to the two streams downstream of their fork, enforcing the weighted-average approach employed in this study for overall nutrient load estimation from the Oglio catchment.
The Pearson correlation coefficients (R) between the observed daily nutrient loads and those estimated with LOADEST for the MTs are reported in Table 4. All values are R ≥ 0.39, highest values with R > 0.98 resulting for N-NO3 and TN, likely due to pollution sources and transport dynamics for nitrates being simpler (TN is primarily made up by N-NO3 for the studied catchments). Lower correlation coefficients result for N-NH4, to which analytical errors due to the low observed concentrations surely contribute, and for P-PO4 and TP, which have multifaceted pollution sources and more complex transport dynamics.
Table 4. Pearson correlation coefficients between observed and estimated daily loads (all correlation coefficients are statistically significant with p-value <0.05).
Estimated loads of the considered nutrient substances for the studied MTs, cumulated over an annual basis, are reported in Figures 2–6, together with the linear trends of the series.
Figure 2. Time series of the cumulated estimated annual load of N-NO3 for: Adda River (A), Chiese River (B), Oglio River (with Italsider industrial channel) (C), Sarca River (D), Ticino River (E), Toce River (with Strona River) (F) (the red dashed lines report the linear trends of the load series; the trends for the Oglio River and Toce River do not consider the industrial channel Italsider and the Strona River, respectively).
Figure 3. Time series of the cumulated estimated annual load of N-NH4 for: Adda River (A), Chiese River (B), Oglio River (with Italsider industrial channel) (C), Sarca River (D), Ticino River (E), Toce River (with Strona River) (F) (the red dashed lines report the linear trends of the load series; the trends for the Oglio River and Toce River do not consider the industrial channel Italsider and the Strona River, respectively).
Figure 4. Time series of the cumulated estimated annual load of TN for: Adda River (A), Chiese River (B), Oglio River (with Italsider industrial channel) (C), Sarca River (D), Ticino River (E), Toce River (with Strona River) (F) (the red dashed lines report the linear trends of the load series; the trends for the Oglio River and Toce River do not consider the industrial channel Italsider and the Strona River, respectively).
Figure 5. Time series of the cumulated estimated annual load of P-PO4 for: Adda River (A), Chiese River (B), Oglio River (with Italsider industrial channel) (C), Sarca River (D), Ticino River (E), Toce River (with Strona River) (F) (the red dashed lines report the linear trends of the load series; the trends for the Oglio River and Toce River do not consider the industrial channel Italsider and the Strona River, respectively).
Figure 6. Time series of the cumulated estimated annual load of TP for: Adda River (A), Chiese River (B), Oglio River (with Italsider industrial channel) (C), Sarca River (D), Ticino River (E), Toce River (with Strona River) (F) (the red dashed lines report the linear trends of the load series; the trends for the Oglio River and Toce River do not consider the industrial channel Italsider and the Strona River, respectively).
Dependence of nutrient loads on rainfall is evident from Figures 2–6, as revealed by the high and very low values obtained for almost all nutrient substances and MTs in 2014, which was a very rainy year (Corsini et al., 2017), and in 2022, which was an extremely dry year (Koehler et al., 2022; Avanzi et al., 2024), respectively.
Such dependence is fully disclosed in Figure 7, in which the Pearson correlation coefficients between the series of annual mean discharges and of cumulated annual nutrient loads are displayed for all considered nutrient substances and MTs. All correlation coefficients are in fact positive, nutrient loads largely depending on flood events (Moatar et al., 2017; Torres and Baronas, 2021), this being due to discharges varying by more orders of magnitudes than concentrations (Godsey et al., 2009; Moatar et al., 2017).
Figure 7. Pearson correlation coefficients between the mean series of annual mean discharges and of cumulated annual nutrient loads for all considered nutrient substances and MTs (the circled point (Sarca river, N-NH4) is the only R coefficient with p-value >0.05)
Correlation coefficients close to unity mostly result in Figure 7 for N-NO3 and TN, echoing their more straightforward dependence on rainfall due to both atmospheric deposition and higher solubility from agricultural soil wash-off. A larger dispersion of correlation coefficients, with lower overall values, results for P-PO4 and TP, likely due to a more evident source-limited behavior, which affects also N-NH4. Similar results among nutrient substances were obtained for the correlation coefficients between mean annual discharge series and total nutrient loads delivered to Lake Como from its major and minor tributaries in Fenocchi et al. (2023).
The Toce River and the Oglio River show the highest correlation coefficients overall among the nutrient substances, highlighting a stronger dependence on discharge variations. This could be due to the higher side slope of the valley for the Toce River, favoring nutrient delivery to the stream with rainfall. For the Oglio River, instead, the cause could be a relevant amount of dispersed nutrients being stocked in the soil and sediments and being subsequently released with flood events, although this last hypothesis would need direct observations to go beyond the speculation stage.
The correlation coefficients for Adda River, Sarca River and Ticino River display a higher variability of the coefficients among the nutrient substances, still abiding to the general behavior with higher N-NO3 and TN values and lower N-NH4, P-PO4 and TP values. This could be due to higher withdrawal of P by vegetation for these basins, due to the higher relevance of agriculture inside them. Finally, the R values are lower for the combined Oglio River and Italsider channel than for the individual streams, the same happening for separate Toce River and Strona River. This issue must be ascribed to the intuitively more straightforward dependence of nutrient loads on rainfall over simpler catchments.
Past and present nutrient reduction interventions have been performed in the catchments of the MTs. However, the decreasing trends visible in Figures 2–6 must be considered in light of trends in mean annual discharges, as given in Supplementary Material S1, also given the outcomes in Figure 7.
Decreasing loads result for the Adda River and the Ticino River for all nutrient substances except N-NO3 and TN, even though with concurrent mean annual discharge decrease. For both basins, such increasing trends for N could be due to increasing agricultural pressures. Specifically, for the Adda River, the slight increase in estimated TN annual loads complies with the higher concentrations measured in recent years, which could be attributed to rising organic nitrogen from agricultural waste in the catchment.
For the Chiese River, estimated loads show a general increase for all nutrient substances in the first half of the time series, peaking on the rainy year 2014, followed by a decreasing trend. The cumulated annual loads for this MT markedly oscillate with discharge for all nutrient substances, with high correlation coefficients between them (Figure 7). This could be due to the steeper and smaller nature of the Chiese catchment compared to those of the other MTs (Table 1; Figure 1), which enforces a behavior which depends more on transport limitation (Torres et al., 2015; Fenocchi et al., 2023).
As regards the Oglio River, it should be noted first that the annual loads from the Italsider channel are in the same order of those from the natural river for all nutrient substances, being even higher overall for P-PO4 (Figure 6). This highlights the relevance of the industrial channel in terms of nutrient delivery to Lake Iseo, due to both discharges being slightly higher than those of the natural river under ordinary conditions and to the usually higher nutrient concentrations (Supplementary Material S2). Broadly speaking, external nutrient loading to Lake Iseo is known to be still relevant, leading together with chemically induced meromixis (Ambrosetti and Barbanti, 2005) to critical water quality conditions with deep-water anoxia and bottom nutrient accumulation (Scibona et al., 2022). Efforts to tackle this situation have been undertaken only recently on the watershed (Valerio et al., 2022), and a decreasing trend can be indeed seen from Figures 2–6, even though simultaneous with the decrease of mean annual discharge (Supplementary Material S1). It should be last mentioned that the water quality sampling location for the Oglio River is placed upstream of the Pisogne wastewater treatment plant outlet, so that a minor load underestimation is furthermore expected.
The cumulated annual loads of the Sarca River are the only ones which seem to increase through the time series, especially for TP and P-PO4 (Figures 5, 6), yet together with a rising trend in discharges (Supplementary Material S1). A longer time series and further assessments would be needed to fully state if such an increase in nutrient loading depends solely on discharges or if the pressures are indeed rising. Figure 7 reveals that the lowest correlation coefficient between annual loads and discharges results for N-NH4 in the Sarca River. This may highlight the presence of a steady, possibly rising, pollution source, more independent than others from discharge. As a matter of fact, increasing N-NH4 concentrations have been observed throughout the time series (Figure 3). This could also explain the comparatively low correlation coefficients obtained for TP and P-PO4 with flowrate in Figure 7.
The annual nutrient loads of the Toce River show a slow steady reduction over the last 40 years, still echoing the behavior of mean annual discharges (Supplementary Material S1). The Toce catchment is characterized by a lower degree of anthropisation compared to those of other MTs, especially in the riparian areas, which are largely occupied by natural vegetation, which also works as a buffer to nutrient pollution. The Toce valley is also quite narrow and steep, therefore urban development is limited by morphology (Bach et al., 2003). Furthermore, industrial activities in the Toce catchment are mostly of chemical nature (Riva et al., 2010), producing little nutrient pollution to the surrounding environment.
To properly compare the nutrient loads and their evolution among the considered MTs, the statistical features and the linear trends of cumulated annual loads for all nutrient substances and of mean annual discharges were computed over the common period of data availability 2008–2022 for all the MTs (Supplementary Material S3, Figure 8). To make trends truly comparable among catchments of different sizes, they have been normalized by the mean annual values over the common period 2008–2022.
Figure 8. Normalized trends of mean annual discharge and cumulated annual nutrient loads over the common period of data availability 2008–2022 for the considered nutrient substances over the MTs.
From Figure 8, it is clear that all MTs exhibited a decreasing nutrient load trend in the 2008–2022 period, except for the Sarca River, for which significantly increasing normalized trends were found for N-NH4, P-PO4 and TP. Mean annual discharges have been similarly decreasing over the same period for the MTs, the normalized reduction being negligible for the Sarca River, likely due to its basin being at the eastern boundary of the DSL region, hence being exposed to a slightly different meteorology. Despite this, such a result deserves further analyses and represents a reason of concern for catchment management, as the N-NH4, P-PO4 and TP nutrient substances for which the positive trend is present are those more closely associated to sewage pollution. On the opposite side, the most relevant decreasing normalized trends over 2008–2022 result for N-NH4 and especially P-PO4 and TP for the Oglio River, likely reflecting the awaited actions on wastewater collection and treatment improvement undertaken in the last decade on the catchment, as highlighted in Valerio et al. (2022). For the Oglio River, the relevance of interventions to reduce nutrient loading from the catchment is further proven by the reducing trend in normalized discharge not being the largest one among the MTs.
A timely study on the external nutrient loads delivered to the Italian DSLs by their MTs has been performed, making use of the available multidecadal experimental data, also originating from the growing water quality database from WFD monitoring. The assessment disclosed the present loading levels, their annual variability with rainfall and allowed drawing some considerations on nutrient load evolution in the MTs, making comparisons among them. The everlasting increase in the length of the available data series would allow even more solid assessments in the future. The LOADEST software has proven a valuable support for this kind of analyses, the external load series obtained in this study being also available as an input for water quality studies on the DSLs themselves, or for the calibration and validation of ecohydrological models of the catchments of the MTs.
The strong dependance of nutrient loads on discharges suggests that decorrelation of trends from the flowrate would allow more meaningful evaluations of nutrient pollution evolution, both over individual basins and on a comparative basis. The decorrelation procedure should correctly keep into account the Q-C relationships of each basin and nutrient substance. Future studies could also address how the estimated loads to the lakes compare against those generated on the catchments according to land use and occupation, determining the nutrient load abatement efficiencies across the catchments of the DSLs, also investigating their interannual variability. Finally, the nutrient abatement efficiencies of the DSLs themselves could be analyzed, comparing the inflowing and outflowing load series.
The data analyzed in this study is subject to the following licenses/restrictions: Data available on request from the authors. Requests to access these datasets should be directed to cGFvbG8uZGV6dWFubmlAcG9saW1pLml0.
PD: Conceptualization, Formal Analysis, Investigation, Methodology, Software, Validation, Visualization, Writing–original draft, Writing–review and editing. DC: Funding acquisition, Resources, Writing–review and editing. CD: Funding acquisition, Project administration, Resources, Supervision, Validation, Writing–review and editing. NP: Methodology, Software, Writing–review and editing. FB: Data curation, Writing–review and editing. AF: Conceptualization, Funding acquisition, Project administration, Resources, Supervision, Validation, Writing–review and editing.
The author(s) declare that financial support was received for the research, authorship, and/or publication of this article. Support by Regione Lombardia inside the “Modellazione idrodinamico-ecologica dei laghi lombardi a supporto dell’aggiornamento del Piano di Tutela delle Acque” research agreement with the University of Pavia and CNR-IRSA is deeply acknowledged.
We are grateful to all the institutions who provided the data series employed in this study, with special attention to Consorzio dell’Oglio and CIPAIS. We deeply thank Michela Rogora of CNR-IRSA Verbania for sharing the CIPAIS database and Gabriele Tartari, Paola Giacomotti and Arianna Orru for performing field sampling activities and laboratory analyses of such data over the decades.
The authors declare that the research was conducted in the absence of any commercial or financial relationships that could be construed as a potential conflict of interest.
The author(s) declared that they were an editorial board member of Frontiers, at the time of submission. This had no impact on the peer review process and the final decision.
The author(s) declare that no Generative AI was used in the creation of this manuscript.
All claims expressed in this article are solely those of the authors and do not necessarily represent those of their affiliated organizations, or those of the publisher, the editors and the reviewers. Any product that may be evaluated in this article, or claim that may be made by its manufacturer, is not guaranteed or endorsed by the publisher.
The Supplementary Material for this article can be found online at: https://www.frontiersin.org/articles/10.3389/fenvs.2025.1524250/full#supplementary-material
DSL, Deep Subalpine Lakes; MT, Main Tributary; N-NO3, Nitrate; N-NH4, Ammonium; TN, Total Nitrogen; P-PO4, Orthophosphate; TP, Total Phosphorus; ARPA, Regional Environmental Protection Agency; CIPAIS, International Commission for the Protection of Italian-Swiss Waters; AMLE, Adjusted Maximum Likelihood Estimation; AIC, Akaike Information Criterion; FOEN, Federal Office for the Environment; CSO, Combined-Sewer Overflow.
1https://eur-lex.europa.eu/legal-content/EN/TXT/?uri=CELEX:32000L0060
2https://www.hydrodaten.admin.ch/en/seen-und-fluesse/stations/2629
3https://environment.ec.europa.eu/topics/water/water-framework-directive_en
4https://www.bimoglio.it/hh/index.php
5https://www.appa.provincia.tn.it/
6https://www.floods.it/public/homepage.php
8https://water.usgs.gov/software/loadest/apps/
Ambrosetti, W., and Barbanti, L. (2005). Evolution towards meromixis of Lake Iseo (Northern Italy) as revealed by its stability trend. J. Limnol. 64, 1–11. doi:10.4081/jlimnol.2005.1
Avanzi, F., Munerol, F., Milelli, M., Gabellani, S., Massari, C., Girotto, M., et al. (2024). Winter snow deficit was a harbinger of summer 2022 socio-hydrologic drought in the Po Basin, Italy. Commun. Earth Environ. 5, 64. doi:10.1038/s43247-024-01222-z
Bach, H., Braun, M., Lampart, G., and Mauser, W. (2003). Use of remote sensing for hydrological parameterisation of Alpine catchments. Hydrol. Earth Syst. Sci. 7, 862–876. doi:10.5194/hess-7-862-2003
Bastanov, R. I., Shakirova, S. S., Gumenyuk, O. A., Derkho, A. O., and Krasnoperva, E. A. (2021). Assessment of ecological safety of fish from anthropogenically polluted freshwater reservoir. E3S Web Conf. 282, 03004. doi:10.1051/e3sconf/202128203004
Benejam, L., Benito, J., and García-Berthou, E. (2010). Decreases in condition and fecundity of freshwater fishes in a highly polluted reservoir. Water Air Soil Pollut. 210, 231–242. doi:10.1007/s11270-009-0245-z
Chen, D., Hu, M., Guo, Y., and Dahlgren, R. A. (2015). Reconstructing historical changes in phosphorus inputs to rivers from point and nonpoint sources in a rapidly developing watershed in eastern China, 1980-2010. Sci. Total Environ. 533, 196–204. doi:10.1016/j.scitotenv.2015.06.079
Copetti, D., and Erba, S. (2024). A bibliometric review on the Water Framework Directive twenty years after its birth. Ambio 53, 95–108. doi:10.1007/s13280-023-01918-0
Copetti, D., Tartari, G., Valsecchi, L., Salerno, F., Viviano, G., Mastroianni, D., et al. (2019). Phosphorus content in a deep river sediment core as a tracer of long-term (1962–2011) anthropogenic impacts: a lesson from the Milan metropolitan area. Sci. Total Environ. 646, 37–48. doi:10.1016/j.scitotenv.2018.07.256
Corsini, A., Ciccarese, G., Diena, M., Truffelli, G., Alberoni, P. P., and Amorati, R. (2017). Debris flows in val parma and val baganza (northern apennines) during the 13 october 2014 alluvial event in parma province (Italy). Italian J. Eng. Geol. Environ. 2017, 29–38. doi:10.4408/IJEGE.2017-01.S-03
Davidson, E. A., David, M. B., Galloway, J. N., Goodale, C. L., Haeuber, R., Harrison, J. A., et al. (2011) “Excess nitrogen in the U.S. environment: trends, risks, and solutions,” in Issues in ecology. doi:10.1149/ma2019-02/19/1023
Decet, F., and Salmaso, N. (1997). Indagini preliminari sulle caratteristiche chimiche dei principali affluenti e dell’emissario del Lago di Garda (Preliminary studies on the chemical features of the main tributaries and the outflow of Lake Garda) [in Italian]. Acqua Aria 7, 91–97.
Dresti, C., Fenocchi, A., and Copetti, D. (2021). Modelling physical and ecological processes in medium-to-large deep European perialpine lakes: a review. J. Limnol. 80. doi:10.4081/JLIMNOL.2021.2041
European Commission (2000). Directive 2000/60/CE of the European Parliament and of the Council establishing a framework for the Community action in the field of water policy.
Fenocchi, A., Buzzi, F., Dresti, C., and Copetti, D. (2023). Estimation of long-term series of total nutrient loads flowing into a large perialpine lake (Lake Como, Northern Italy) from incomplete discrete data by governmental monitoring. Ecol. Indic. 154, 110534. doi:10.1016/j.ecolind.2023.110534
Fong, P., Boyer, K. E., Kamer, K., and Boyle, K. A. (2003). Influence of initial tissue nutrient status of tropical marine algae on response to nitrogen and phosphorus additions. Mar. Ecol. Prog. Ser. 262, 111–123. doi:10.3354/meps262111
Godsey, S. E., Kirchner, J. W., and Clow, D. W. (2009). Concentration–discharge relationships reflect chemostatic characteristics of US catchments. Hydrol. Process 23, 1844–1864. doi:10.1002/HYP.7315
Hill, M. (2013). Adaptive capacity of water governance: cases from the Alps and the andes. Mt. Res. Dev. 33, 248–259. doi:10.1659/MRD-JOURNAL-D-12-00106.1
Hutchinson, G. E. (1973). Eutrophication. The scientific background of a contemporary practical problem. Am. Sci. 61, 269–273.
Jeppesen, E., Søndergaard, M., Jensen, J. P., Havens, K. E., Anneville, O., Carvalho, L., et al. (2005). Lake responses to reduced nutrient loading - an analysis of contemporary long-term data from 35 case studies. Freshw. Biol. 50, 1747–1771. doi:10.1111/j.1365-2427.2005.01415.x
Koehler, J., Dietz, A. J., Zellner, P., Baumhoer, C. A., Dirscherl, M., Cattani, L., et al. (2022). Drought in northern Italy: long earth observation time series reveal snow line elevation to Be several hundred meters above long-term average in 2022. Remote Sens. (Basel) 14, 6091. doi:10.3390/rs14236091
Kumar, B., and Anshumali, (2019). Phosphorus fractionation in surface water and sediments of industrially polluted freshwater reservoir, India. Chem. Ecol. 35, 219–234. doi:10.1080/02757540.2018.1554058
Matzinger, A., Spirkovski, Z., Patceva, S., and Wüest, A. (2006). Sensitivity of ancient lake ohrid to local anthropogenic impacts and global warming. Journal of Great Lakes Research 32, 158–179. doi:10.3394/0380-1330(2006)32[158:SOALOT]2.0
Mezzanotte, V., Bresciani, M., Canobbio, S., Giardino, C., Antonelli, M., Bortoluzzi, A., et al. (2011). Monitoring, environmental emergencies management and water treatment improvement of freshwater lakes in China: the Chao Lake case study. Water Sci. Technol. Water Supply 11, 490–496. doi:10.2166/ws.2011.071
Moatar, F., Abbott, B. W., Minaudo, C., Curie, F., and Pinay, G. (2017). Elemental properties, hydrology, and biology interact to shape concentration-discharge curves for carbon, nutrients, sediment, and major ions. Water Resour. Res. 53, 1270–1287. doi:10.1002/2016WR019635
Mohammed, E. A., Naugler, C., and Far, B. H. (2015) “Emerging business intelligence framework for a clinical laboratory through big data analytics,” in Emerging trends in computational biology, bioinformatics, and systems biology: algorithms and software tools, 577–602. doi:10.1016/B978-0-12-802508-6.00032-6
Mosello, R., Barbieri, A., Brizzio, M. C., Calderoni, A., Marchetto, A., Passera, S., et al. (2001). Nitrogen budget of Lago Maggiore: the relative importance of atmospheric deposition and catchment sources. J. Limnol. 60, 27–40. doi:10.4081/jlimnol.2001.27
Nava, V., Patelli, M., Bonomi, T., Stefania, G. A., Zanotti, C., Fumagalli, L., et al. (2020). Chloride balance in freshwater system of a highly anthropized subalpine area: load and source quantification through a watershed approach. Water Resour. Res. 56. doi:10.1029/2019WR026024
Nizzoli, D., Bartoli, M., Azzoni, R., Longhi, D., Castaldelli, G., and Viaroli, P. (2018). Denitrification in a meromictic lake and its relevance to nitrogen flows within a moderately impacted forested catchment. Biogeochemistry 137, 143–161. doi:10.1007/s10533-017-0407-9
Noll, M. R., and Magee, E. A. (2009). Quantification of phosphorus sources to a small watershed: a case study of Graywood Gully, Conesus Lake, NY. J. Gt. Lakes. Res. 35, 50–55. doi:10.1016/j.jglr.2008.09.005
Park, Y. S., and Engel, B. A. (2016). Identifying the correlation between water quality data and LOADEST model behavior in annual sediment load Estimations. WaterSwitzerl. 8, 368. doi:10.3390/w8090368
Payganeh, S., Knight, M. A., and Haas, C. T. (2018) “Analyzing conflicts over water extraction from great lakes of north America through game theory approaches,” in Pipelines 2018: utility engineering, surveying, and multidisciplinary topics - proceedings of sessions of the pipelines 2018 conference, 195–203. doi:10.1061/9780784481660.022
Pilotti, M., Barone, L., Balistrocchi, M., Valerio, G., Milanesi, L., and Nizzoli, D. (2021). Nutrient delivery efficiency of a combined sewer along a lake challenged by incipient eutrophication. Water Res. 190, 116727. doi:10.1016/j.watres.2020.116727
Ramadan, A. H., and Sembiring, E. (2020). Occurrence of microplastic in surface water of jatiluhur reservoir. E3S Web Conf. 148, 07004. doi:10.1051/e3sconf/202014807004
Riva, C., Binelli, A., Parolini, M., and Provini, A. (2010). The case of pollution of Lake Maggiore: a 12-year study with the bioindicator mussel dreissena polymorpha. Water Air Soil Pollut. 210, 75–86. doi:10.1007/s11270-009-0225-3
Rogora, M., Buzzi, F., Dresti, C., Leoni, B., Lepori, F., Mosello, R., et al. (2018). Climatic effects on vertical mixing and deep-water oxygen content in the subalpine lakes in Italy. Hydrobiologia 824, 33–50. doi:10.1007/s10750-018-3623-y
Rogora, M., Marchetto, A., and Mosello, R. (2001). Trends in the chemistry of atmospheric deposition and surface waters in the Lake Maggiore catchment. Hydrol. Earth Syst. Sci. 5, 379–390. doi:10.5194/hess-5-379-2001
Rogora, M., Marchetto, A., and Mosello, R. (2003). Modelling the effects of atmospheric sulphur and nitrogen deposition on selected lakes and streams of the Central Alps (Italy). Hydrol. Earth Syst. Sci. 7, 540–551. doi:10.5194/hess-7-540-2003
Salmaso, N., Morabito, G., Garibaldi, L., and Mosello, R. (2007). Trophic development of the deep lakes south of the Alps: a comparative analysis. Fundam. Appl. Limnol. 170, 177–196. doi:10.1127/1863-9135/2007/0170-0177
Salmaso, N., and Mosello, R. (2010). Limnological research in the deep southern subalpine lakes: synthesis, directions and perspectives. Adv. Oceanogr. Limnol. 1, 29–66. doi:10.1080/19475721003735773
Scibona, A., Nizzoli, D., Hupfer, M., Valerio, G., Pilotti, M., and Viaroli, P. (2022). Decoupling of silica, nitrogen and phosphorus cycling in a meromictic subalpine lake (Lake Iseo, Italy). Biogeochemistry 159, 371–392. doi:10.1007/s10533-022-00933-9
Severini, E., Magri, M., Soana, E., Bartoli, M., Faggioli, M., and Celico, F. (2023). Irrigation practices affect relationship between reduced nitrogen fertilizer use and improvement of river and groundwater chemistry. Agric. Water Manag. 289, 108564. doi:10.1016/j.agwat.2023.108564
Song, J. H., Her, Y., Park, Y. S., Yoon, K., and Kim, H. (2024). Investigating the applicability and assumptions of the regression relationship between flow discharge and nitrogen concentrations for load estimation. Heliyon 10, e23603. doi:10.1016/j.heliyon.2023.e23603
Stackpoole, S., Sabo, R., Falcone, J., and Sprague, L. (2021). Long-Term Mississippi river trends expose shifts in the river load response to watershed nutrient balances between 1975 and 2017. Water Resour. Res. 57, e2021WR030318. doi:10.1029/2021WR030318
Terzi, S., Sušnik, J., Schneiderbauer, S., Torresan, S., and Critto, A. (2021). Stochastic system dynamics modelling for climate change water scarcity assessment of a reservoir in the Italian Alps. Nat. Hazards Earth Syst. Sci. 21, 3519–3537. doi:10.5194/nhess-21-3519-2021
Torres, M., Joshua West, A., and Clark, K. E. (2015). Geomorphic regime modulates hydrologic control of chemical weathering in the Andes–Amazon. Geochim. Cosmochim. Acta 166, 105–128. doi:10.1016/J.GCA.2015.06.007
Torres, M. A., and Baronas, J. J. (2021). Modulation of riverine concentration-discharge relationships by changes in the shape of the water transit time distribution. Glob. Biogeochem. Cycles 35, e2020GB006694. doi:10.1029/2020GB006694
Valerio, G., Pilotti, M., Scibona, A., and Nizzoli, D. (2022). Monitoring phosphorus in the tributaries of a deep lake from the perspective of the receiving water body. Hydrol. Process 36. doi:10.1002/hyp.14612
Yang, F., Guo, J., Qi, R., and Yan, C. (2023). Isotopic and hydrochemical analyses reveal nitrogen source variation and enhanced nitrification in a managed peri-urban watershed. Environ. Pollut. 316, 120534. doi:10.1016/j.envpol.2022.120534
Yates, A. G., Brua, R. B., Friesen, A., Reedyk, S., and Benoy, G. (2022). Nutrient and suspended solid concentrations, loads, and yields in rivers across the Lake Winnipeg Basin: a twenty year trend assessment. J. Hydrol. Reg. Stud. 44, 101249. doi:10.1016/j.ejrh.2022.101249
Keywords: LOADEST regression model, DSL, phosphorus and nitrogen external loads, long-time series analysis, catchment nutrient pollution, flow discharge
Citation: Dezuanni P, Copetti D, Dresti C, Pella N, Buzzi F and Fenocchi A (2025) Evaluation of nutrient loads conveyed to the deep subalpine lakes of Northern Italy through their main tributaries. Front. Environ. Sci. 13:1524250. doi: 10.3389/fenvs.2025.1524250
Received: 07 November 2024; Accepted: 03 January 2025;
Published: 24 January 2025.
Edited by:
Qinghua Cai, Chinese Academy of Sciences (CAS), ChinaCopyright © 2025 Dezuanni, Copetti, Dresti, Pella, Buzzi and Fenocchi. This is an open-access article distributed under the terms of the Creative Commons Attribution License (CC BY). The use, distribution or reproduction in other forums is permitted, provided the original author(s) and the copyright owner(s) are credited and that the original publication in this journal is cited, in accordance with accepted academic practice. No use, distribution or reproduction is permitted which does not comply with these terms.
*Correspondence: Paolo Dezuanni, cGFvbG8uZGV6dWFubmlAcG9saW1pLml0
†Present address: Paolo Dezuanni, Department of Civil and Environmental Engineering, Politecnico di Milano, Milan, Italy
Disclaimer: All claims expressed in this article are solely those of the authors and do not necessarily represent those of their affiliated organizations, or those of the publisher, the editors and the reviewers. Any product that may be evaluated in this article or claim that may be made by its manufacturer is not guaranteed or endorsed by the publisher.
Research integrity at Frontiers
Learn more about the work of our research integrity team to safeguard the quality of each article we publish.