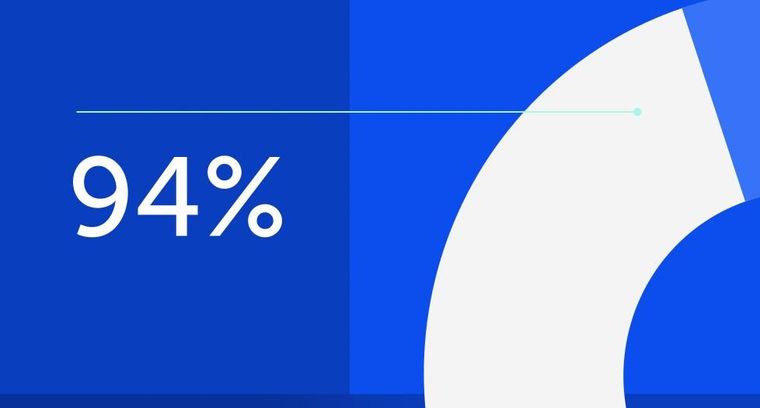
94% of researchers rate our articles as excellent or good
Learn more about the work of our research integrity team to safeguard the quality of each article we publish.
Find out more
ORIGINAL RESEARCH article
Front. Environ. Sci., 12 March 2025
Sec. Environmental Economics and Management
Volume 13 - 2025 | https://doi.org/10.3389/fenvs.2025.1514403
In the context of global concerns about climate change and environmental pollution, green economy is a key path to sustainable development. This study focuses on the impact of FinTech (FI) on China’s green economic growth (GEG) and regional differences to support green economic development. The panel data of 30 provinces in China from 2011 to 2021 are selected to analyse the relationship between fintech innovation and green economic growth with the help of spatial Durbin model, and spatial factors are taken into account to ensure that the study is scientific and reliable. Fintech innovation significantly promotes green economic growth, with positive spatial spillover effects. However, there are obvious regional differences, as the eastern part of China has developed its economy and financial technology, and its financial technology promotes green economic growth much more than that of the central and western parts of China, showing a transitional situation between the east and the west, and the central part of China. Fintech promotes China’s green economic growth, but regional development is uneven. While the eastern part of the country makes good use of fintech, the central and western parts of the country need to improve financial infrastructure and cultivate talents. This study provides empirical evidence on the development of green economy in Chinese cities, which will help coordinate the development of science and technology with economic development, formulate differentiated policies, and contribute Chinese experience to global green development.
The escalating urgency of the climate crisis has prompted global economies to adopt sustainable, low-carbon growth models. As the concept of a green economy gains wider acceptance, the demand for robust financial mechanisms to facilitate this transition has become increasingly evident. However, traditional financial systems often exhibit limitations in providing adequate funding and support for green initiatives, primarily due to their risk-averse nature and the inherent complexities involved in evaluating and financing environmentally sustainable projects. Within this context, financial technology (FinTech) has emerged as a pivotal instrument to bridge the gap between sustainable development objectives and the financial resources required to achieve them (Khoshnava et al., 2020).
As the global economy transitions towards greener models, it is essential to investigate innovative financial tools that can support sustainable development (Wang et al., 2021). FinTech offers a unique opportunity to address many of the conventional challenges associated with green finance, including enhancing accessibility, improving efficiency, and promoting transparency and innovation (Ding et al., 2022). A systematic study of FinTech’s impact on green economic development is both timely and necessary to ensure that the financial sector can effectively facilitate the transition to a sustainable, low-carbon future. Such research is critical to unlocking the full potential of financial technology as a catalyst for both economic growth and environmental sustainability.
In this research endeavour, we endeavour to illuminate the intricate interplay between fintech and the progression of green economic growth (GEG). Our investigation delves into the question of whether the augmentation of information technology (IT) resources allocated to financial institutions fosters or impedes the proliferation of regional green economic development. Our study aligns closely with the pioneering works of Zhou et al. (2022a) and Nenavath and Mishra (2023), which have collectively underscored the salutary effects of fintech on fostering green economy development, employing green finance as a pivotal mediating mechanism.
Based on the transition of global climate governance and the practice of China’s “dual-carbon” strategy, this study focuses on the spatial and temporal effect mechanism of fintech-driven green economic growth. Through the spatial Durbin model, it is found that there is a significant spatial spillover effect of fintech innovation on green total factor productivity, with a gradient difference of east > central > west, revealing the ternary synergy law of technology-finance-environment in the era of digital economy. At the practical level, it provides differentiated paths for deepening the integration of science and technology industries in the east and breaking through the bottleneck of digital infrastructure in the central and western parts of China, and at the same time builds a synergistic framework of “technological spillover in the east, industrial undertaking in the central part of China, and ecological compensation in the western part of the country”.
Due to the availability and completeness of the data, this paper selects the panel data of 30 provinces in China from 2011-2021 and uses the SDM model to explore the impact of fintech on the development of the green economy. It further divides the regions into East, Central, and West and conducts heterogeneity analyses to explore the differences in the impacts between different regions. Through empirical evidence, it is found that: 1. The green economy development and green finance level of the 30 provinces in China show an upward trend and present a situation of strong in the east, weak in the west, and transition in the centre; 2. Fintech promotes the development of the local green economy, and at the same time there is a spatial spillover effect on the neighbouring regions; 3. There is a regional heterogeneity of the impact of fintech on the development of the green economy.
The sections of the study are arranged accordingly: Section 2 comprehends the existing literature, Section 3 analyses the influencing mechanism, and Section 4 presents the data and models. Section 5 carries out the empirical research. Section 6 summarizes the empirical results and puts forward the relevant policy recommendations.
The concept of a green economy emerges as a guiding principle, encapsulating the core objective of elevating human wellbeing and promoting social justice to pursue a sustainable future (Lin and Zhou, 2022). Technological innovation has a key role in driving the transition to a green economy (Cheng et al., 2024). The innovation and optimization of green technologies are identified as crucial factors in fostering sustainable development and mitigating adverse externalities. This confirms that the convergence of digital technologies with green innovation empowers businesses to enhance competitiveness. The convergence of digital technologies and green innovation can help businesses become more competitive (Yin and Zhao, 2024). Manufacturing enterprises can further promote their digital green innovation performance through external knowledge cooperation (Yin and Yu, 2022). China’s green economy is booming, and innovation is a key driver of economic growth (Zhao et al., 2022b). Yu et al. argue that there is an inverted U-shape relationship between green technological innovation and economic growth pressures (Yu et al., 2023).
The Environmental Kuznets Curve (EKC) depicts an inverted U-shaped relationship between environmental pollution and economic growth. Scholars have extensively explored the factors influencing carbon emissions within this theoretical framework. Some research indicates a diminishing impact of economic growth on carbon emissions (Li et al., 2024). Yu et al. discovered that Foreign Direct Investment (FDI) positively impacts green economic development in Chinese urban areas through technology transfer and agglomeration effects (Yu and Liu, 2024). As economies allow foreign investment and adopt advanced technologies, a positive spillover effect occurs. Furthermore, advanced technologies tend to mitigate the inhibitory effect on GEG (Yu et al., 2021). However, trade exhibits heterogeneity in its impact on carbon emissions. While trade openness may promote carbon emissions, diversification of trade can serve to inhibit carbon emissions (Wang, Zhang and Li, 2024). Moreover, human capital (EDU) exerts a positive and enduring influence on economic growth (Doré and Teixeira, 2023). The level of human capital also influences environmental quality. An increase in green human capital initially leads to a deterioration in environmental quality, but in the long run, it results in an improvement in the environment (Ahakwa et al., 2023). Wang et al. (2023) concluded that the effect of human capital on carbon emissions varies before and after the EKC turning point, with a more pronounced inhibitory effect observed after the turning point (Wang et al., 2023).
The policies of green economy and globalization have had a combined effect on the economic value of natural resources, specifically the decrease in the form of resource rent (Xuan et al., 2023). This result underscores the importance of developing green growth policies. It acknowledges that natural resources are finite and cannot be exploited or substituted without limit. The study further points out that policies to promote green growth have a crucial role to play in achieving sustainable, inclusive and robust economic growth. In terms of environmental benefits, green economy policies can significantly increase the efficiency of resource use and play an important role in significantly reducing environmental pollution (Ma et al., 2023).
Fintech, the fusion of financial services with technological innovation, is a pivotal force propelling the global digital economy forward. Green technology innovation (GTI) is characterized by external economics, high investment, and high risk. Fintech can empower traditional financial institutions to tackle green identification, reduce the cost of green credit and guarantee GTI activities (Liu et al., 2023). Some studies assert that fintech not only facilitates technological innovation but also accelerates the growth of the digital economy. It diminishes the role of local governments in fiscal decentralization (Chen et al., 2022). Fintech reduces financial risk and promotes sustainable development. It also carries out investment activities in digital green innovation projects and selects co-investment partners which will help companies avoid financial risks and achieve sustainable economic development (Yin et al., 2022).
The transformative potential of fintech extends to reshaping industry structures and redirecting capital flows (Dong et al., 2024). Regional economies also have the potential to significantly benefit as they foster growth and prosperity in the area. According to financial geography, distance increases transaction costs, and information asymmetry hinders risk control. Fintech makes use of information technology to overcome geographic restrictions, and “information asymmetry”. This eases the contradiction between demand and supply, enterprises and banks, and reduces transaction costs and financing constraints (Guo et al., 2023). It completes the process of reducing costs and controls risks through technical identification. Moreover, it aids in the reduction of geographical barriers in financial services which often lead to disparities and inequalities. Since geographical distance affects the accessibility of financial resources, human capital, and other factors, fintech hubs tend to be established in already existing financial centers. FinTech companies tend to cluster in central cities, where higher salary levels and employment environments attract talent inflows. It intensifies competition within the industry and also eases financing constraints and other issues. This accelerates corporate innovation and increases total factor productivity, thereby promoting green urban developmen (Huang, 2022). Fintech companies in central cities are more likely to play a radiation-driven role in geographically neighboring cities. Geographical proximity ensures a reduced transactional cost, transport costs and control risks. It also facilitates the fintech in the central city to play a “technological spillover effect” to reduce the green credit constraints faced by enterprises and thus promote cities’ green development (Liao and Li, 2022). Therefore, fintech can have a beneficial impact on the local economy.
As finance and technology become more integrated, understanding the impact of financial investment on GEG is crucial for promoting China’s sustainable development. The role of FinTech in driving regional economic growth has been widely recognized (Zhou and Wang, 2024). Studies have shown that China’s GEG shows a fluctuating trend and further found that financial development and TI promote green economy fluctuations (Cao et al., 2021).
In fintech innovations, traditional financial service constraints are breached to promote sustainable regional development by reducing pressure on resources (Teng and Shen, 2023). Moreover, energy efficiency improvements can curb carbon emissions in the transport sector (Li et al., 2022). Fintech uses artificial intelligence (AI) to provide smarter and more personalized means of service. This brings financial services closer to user needs and market changes (Almansour, 2023). In the context of trade openness, AI can effectively curb carbon emissions as trade openness increases (Wang et al., 2024). Fintech has the effect of governing the environment and promoting the traditional financial services industry to move from emptiness to reality. It further alleviates the problem of enterprise financing constraints by assisting the enterprise’s innovation, production and business activities. This ensures high-quality and sustainable development (Guo et al., 2023). Fintech enables online and digital payment and withdrawal. The electronic service model directly reduces the consumption of physical resources in the process of financial business, thus reducing carbon emissions and environmental pollutant emissions (Wang et al., 2024). Concurrently, it has brought about an information revolution in the financial sector, reduced the degree of informational asymmetry, and promoted the optimization of resource allocation efficiency. The introduction of big data technology directly promotes the collection, collation, and analysis of information on green projects by financial institutions and assists in decision-making and management (Liu et al., 2024). Therefore, this paper makes the case that fintech can influence the GEG. According to neoclassical growth theory, the marginal returns to production inputs diminish, and because of resource constraints, sustainable economic growth cannot rely solely on inputs of factors of production. However, technological innovation is also required to achieve this. Green technology innovation (GTI) can advance the study and creation of environmentally friendly products. It can also help reduce waste and pollutants and improve the efficiency of resource utilization (Dabbous and Aoun Barakat, 2023; Zhang et al., 2023). The innovation of green technology can help the government or institutions to complete green strategic planning and effectively improve the quality of the environment (Ni et al., 2023).
The Environmental Kuznets Curve (EKC) theory posits an inverted U-shaped relationship between economic growth and environmental degradation, suggesting an eventual shift towards environmental protection as economies mature. With the pursuit of high-quality economic development, balancing growth with environmental sustainability has become paramount.
Fintech, especially the application of artificial intelligence (AI) and big data, has brought significant changes to energy management, strongly promoting energy conservation and emission reduction. Through real-time collection and analysis of massive data on energy production, transmission, distribution, and consumption, AI algorithms can accurately predict energy demand and optimize scheduling programs (Liu et al., 2022). The smart grid analyses the user’s power consumption habits with big data, reasonably distributes power resources, and effectively reduces energy loss; in industrial production, AI monitors the operating conditions of equipment in real time, predicts failures in advance, and dramatically improves the efficiency of energy use, providing a solid energy guarantee for the development of the green economy.
In terms of green investment decision-making, financial and technological innovation provides a more scientific basis. Big data widely integrates corporate environmental performance data, sustainable development reports and market dynamics and other sources of information to build a comprehensive green investment assessment system. Artificial intelligence machine learning algorithms conduct in-depth mining and analysis of these data to accurately assess the investment value and risk level of green projects. Taking the investment evaluation of new energy projects as an example, with the help of AI models for comprehensive consideration, it can help investors screen out more potential projects, guide the flow of social capital to the green industry, and provide sufficient financial support for the development of the green economy (Huang et al., 2024).
Financial and technological innovation has also injected a strong impetus for the development of the green industry. Big data and artificial intelligence help enterprises gain precise insight into market demand, optimise product design by analysing consumers' environmental preferences, enhance the green attributes of products and strengthen market competitiveness (Yu and Liu, 2024). Meanwhile, the rise of fintech platforms has effectively lowered the financing threshold of green enterprises, and crowdfunding platforms have opened up financing channels for small-scale green entrepreneurial projects, which has greatly promoted the R&D and application of green technologies and facilitated the flourishing development of the green industry in a diversified direction.
Fintech promotes interregional financial cooperation through cross-border payments and international crowdfunding. This accelerates the flow of funds to a wide range of regions, opens up more financing channels for green projects and enhances capital efficiency. This model directly promotes the green development of the project area and attracts the attention of other regions. It also stimulates imitation and competition and creates a spatial spillover effect of green economic growth. Fintech tools such as crowdfunding platforms act as knowledge dissemination channels, facilitating the widespread sharing of green technologies and encouraging innovators from all over the world to participate in the green economy. The emergence of Fintech has eased the transfer of technology across borders. For instance, sustainable agricultural technology can now be spread to other regions via the internet to promote green transformation. In addition, the regional dissemination of fintech success stories and policy innovations promotes the harmonization of policies and regulations. This also provides a favourable policy environment for the green economy and further stimulates positive interregional spillover effects.
Figure 1 illustrates the mechanism of fintech’s impact on GEG. In this view, the following hypotheses of this research are proposed.
H1. Fintech innovation is crucial to promoting green growth.
H2. Fintech innovation has obvious regional heterogeneity in terms of green economic growth.
One crucial metric for assessing how well the green development model is working is the Green Economic Growth Index (GEG). This paper empirically analyzes the connection between FI and GEG based on relevant research on green economic growth by Lin and Zhou (2022). In addition to economic development, GEG needs to be studied in terms of ecological protection, social progress, and technological innovation. As such, based on Hu and Lin (Hu and Zheng, 2023; Lin and Zhou, 2022), this study constructs a green development indicator system that is more comprehensive and more in line with China’s national conditions. The indicators include the four secondary indicators mentioned above and 21 tertiary indicators (Table 1). The GEG indicators were constructed using the entropy weight method. The data were logarithmically processed for accuracy. The study presents the measured green economic growth indices of 30 provinces and cities in China from 2011 to 2021 in Figure 2.
The data results (Figure 2) show that the overall GEG of China’s provinces shows a year-on-year increasing trend from 2011 to 2021, with Beijing, Shanghai, Jiangsu, Zhejiang and Guangdong having higher overall levels. This means that economically developed regions also have greater options for transforming their economic growth patterns. They can more effectively promote high-quality, low-pollution development patterns. The development mode of high quality and low pollution can be promoted more effectively.
From a regional perspective, GEG is high in the eastern seaboard region with its superior economic and financial ecological advantages. It has also demonstrated excellent performance for the green economy in terms of demand and financial support. Along with the promotion of green financial policies, the Eastern Seaboard is rapidly becoming a national and international meeting point for high-quality capital, advanced green technologies and professional talents. The underdevelopment of central and western regions can be attributed to several factors. Firstly, these regions exhibit a lower level of economic development, which hinders the achievement of a balance between economic growth and environmental sustainability, thus impeding the transition to a green economy. Secondly, the central and western regions are characterized by a higher concentration of resource-based industries. These industries, which were historically associated with high pollution and energy consumption, have not undergone comprehensive upgrades. This results in continued adverse impacts on ecological and environmental protection efforts. Consequently, GEG in these regions remains relatively low. This regional disparity highlights the existence of variations in green economic growth (GEG) across different geographical areas.
Financial technology (FinTech) is still in its infancy and there is no standardized definition of it in academia. The Financial Stability Board noted that FinTech (FI) is the term for new financial innovation brought about by blockchain and big data, creating new products, applications, business models, and processes in the financial industry.
Fintech innovation (FI) was replaced by the Digital Finance Indicator (DFI), which was selected to reflect the development of inclusive digital finance in China’s 30 inter-provincial regions (Guo et al., 2020). Underneath the DFI, there are three auxiliary indicators. The first is the discussion on the coverage of DFI, which largely reflects the breadth of coverage of FI. The second indicator is the depth of use. The interpenetration of finance and technology is not only reflected in the breadth of application but also in the depth of consideration, which is measured specifically through credit and payments. The third is the degree of digitalization. Financial services are characterized by multi-level and diversified development. The mutual integration of finance and technology has greatly improved the convenience and mobility of financial services. There are also 33 tertiary indicators under the secondary indicators.
This paper combines the existing relevant studies and takes other relevant variables affecting GEG as control variables. These are as follows: Urbanization rate (UR) is measured by the proportion of urban residents to the total population of the degree of urbanization in China’s provincial administrative regions. Total foreign investment as a proportion of regional GDP is used to represent the indicator of FDI. The structure is the tertiary industry’s added value divided by the secondary industries. Human capital level (Edu) is replaced by the university students’ number per 10,000 population in persons. The ratio of the entire postal and telecommunications industry to the regional GDP is used to indicate the amount of information (Tech).
The 2011–2021 period marks China’s economic transition from high-speed to high-quality growth, encompassing the 12th and 13th Five-Year Plans. Green economy trends flourished during this time. China has a vast territory, and there are significant differences among provinces in terms of economic development, resource endowment and ecological environment. Choosing 30 provinces as the study object can cover different types of regions and improve the representativeness and universality of the study. Special administrative regions differ from other provinces in terms of systems, policies and management regimes; at the same time, there are differences in the calibers and sources of data statistics, which affect the comparability and quality of data. Therefore, this paper selects annual panel data for 30 Chinese provinces (excluding Tibet) from 2011 to 2021. Data were obtained from the National Bureau of Statistics, provincial statistical yearbooks, the China Statistical Yearbook and the Environmental Statistics Yearbook (Table 2).
In real economic societies, geographically adjacent countries or regions strengthen their economic ties and cooperation. They coordinate their regional economic policies to safeguard their common economic interests, which means that regional economic activities cannot exist independently. Green economic growth has obvious spatial spillover (Song et al., 2019). The spatial econometric model relaxes the assumption that spatial units are independent of each other. This means the model considers an interrelationship between the region and its neighbours. Thus, changes in variables in the region directly affect the region and its neighbours. Therefore, this paper uses spatial econometric modelling in its study.
In the field of spatial economics, spatial autocorrelation analysis is used to explore the extent to which the same variables in different regions are spatially dependent. It is also implemented to reflect more comprehensively and realistically the direction and degree of influence among the studied variables. Before conducting the baseline regression, the spatial autocorrelation of GEG and financial technology innovation is tested using the Moran index to determine the spatial econometric model. The equation for calculating the Global Moran Index (Equation 1) is as follows:
Where:
The spatial weight matrix forms the basis for constructing spatial models which can be divided into adjacency matrices and distance matrices according to type. The 0–1 matrix has been chosen as the matrix of choice when analyzing spatial spillovers. As shown in Equation 2, 0–1 matrices assign a value of 1 to adjacent areas and 0 to non-adjacent areas. This means that the correlation between spatial units depends on whether the two areas are adjacent or not, with certain regional restrictions. Therefore, the economic distance matrix Equation 3 was chosen for comparative analysis, taking into account the spillover range.
Adjacency weight matrix
Economic distance weighting matrix
As seen from Table 3, under the weight matrix of adjacency weight and weight matrix of economic distance, the Moran index of China’s GEG during 2011–2021 is positive and significant at the 1% statistical level.
To observe the contribution of provinces to spatial autocorrelation, we used Moran scatter plots to describe spatial agglomeration. The study takes 30 provinces and selects the green economic growth index data for 3 years, 2011, 2016 and 2021, in the form of cross-sectional data and demonstrates the spatial autocorrelation using a Moran scatter plot. As shown in Figure 3, the 2021 green index of economic growth of the local Moran scatter diagram, we can find that the local Moran Green Growth Index is mainly located in quadrants 1 and 3. Moreover, most provinces share the same characteristics in terms of their level of GEG. A province with a weaker green economic growth level has a weaker GEG in its neighbouring provinces. The above indicates that the regional GEG has spatial correlation and severe geographical spatial imbalance, corroborating the findings of Zhao et al. (Zhao et al., 2022a).
Further identification of the patterns of spatial econometric models is required. Without considering any spatial correlation, the LM test was used to determine whether to choose the SAR or SEM model and if both were possible, the SDM model was chosen. The Wald and LR tests were then used to determine whether the SDM model could be reduced to a SAR or SEM model (Table 4). The Housman test was used to select either a fixed effects model or a random effects model. Immediately following this, the joint significant test was used to further determine which fixed model (time fixed, spatial fixed and double fixed) to choose.
Referring to Elhorst’s test idea (Elhorst, 2012), the Wald test, LM test and LR test pass the 1% significance test, indicating that the SDM is more suitable for the analysis in this paper.
With the above tests, this paper constructs the SDM model for empirical analysis. The general expressions (4) for the models are as follows:
where
In the adjacency matrix (Table 5 (1)) and economic distance matrix (Table 5 (2)), the regression coefficients of the level of fintech are both positive. This indicates that the development of local FinTech has a significant contribution to the local GEG. Innovative developments in financial technology have broken cost barriers to financial services. It has also expanded the audience of financial services and enabled more people to use financial products conveniently through digital technologies. In this way, it will raise consumption levels in the region and promote GEG. In the meantime, it can be observed that fintech innovation in this region has a positive contribution to GEG in neighbouring regions. However, in regions with close economic ties, Fintech has a negative spatial spillover effect on GEG. Innovation in financial technology mainly refers to the use of advanced, sophisticated, and cutting-edge technologies, such as big data analysis, to achieve inter-regional linkage and dissemination of TI. FI is caused by the spread effect of internet technology, which has the basic characteristics of technical knowledge in essence. In addition, with the help of remote communication and interaction of the Internet, it can effectively break the regional limitations of inter-regional technology exchange, information transmission, and knowledge transmission. This will help to realize the spread and linkage of remote knowledge. Regions with close economic ties can also strengthen the learning and imitation of advanced technologies. This will help promote cooperation and competition between enterprises so that technological progress can be reflected in improving work efficiency and the effective control of unknown risks.
Under the two spatial weighting matrices, FDI, Tech and Urban all contribute significantly to GEG. FDI spreads through technology and advanced management experience, which in turn affects resource allocation. Observing the Wx term, with the higher local urbanization rate, there is a significant positive spatial spillover effect on the GEG of neighbouring regions. This may be related to the population movement between neighbouring regions. From the accelerating effects of technology and finance upon the movement of human resources, FI will inevitably promote the spatial movement of talent across regions. In a conventional sense, human capital is the basic power source that ensures the normal economic operation and healthy operation of enterprises. Scientific and technological development, as well as the steady advancement of the economy, lies in human resources. It is necessary to allocate human resources management technology reasonably since it is an important hub to accelerate the inter-regional realization of information exchange and technology interaction. Moreover,it considers the spatial spillover effect for invisible knowledge, thus promoting the regional economy to achieve rapid growth. Previous studies have shown that FDI can bring managerial skills and advanced production technologies to the Chinese economy (Pan et al., 2017). The “bottom-up competition” hypothesis suggests that to attract more FDI inflows, countries or regions will attempt to use reduced environmental protection to gain more market share by relaxing relevant environmental regulations (Luo et al., 2021). Such behaviour can boost economic growth in the short term as FDI increases but also degrade the environment due to the investment construction with the lack of corresponding environmental constraints. Overall, it can hinder green economic growth (Feng et al., 2019). A possible explanation for the significantly positive regression coefficient for industrial structure in neighbouring regions is that the differentiation and complementarity of industrial structure between regions could provide potential conditions for cross-regional industrial specialization and regional specialization. The industrial structure differences drive the green economy, “fluid factor resources ”, spatial aggregation and diffusion effect.
The spillover effects of the spatial Durbin model are decomposed and analyzed specifically (Table 6). From the perspective of direct effect, under both spatial weight matrices, fintech innovation is significantly positively related to green economic growth. This suggests that both can aid in the local province’s GEG. Tech, Structure and Urban play a significant role in GEG in both weighting matrices, while Edu and FDI only play a significant role in GEG in the neighbourhood matrix.
In terms of indirect effects, the level of FI is significantly negative under the economic distance weighting matrix. This suggests that local FinTech have a negative spillover effect on the GEG of provinces with close economic ties. Fintech has the characteristics of technological knowledge. Edu possesses positive spatial spillover effects and promotes GEG in surrounding provinces. In terms of the overall effect, under both spatial weight matrices, FI possesses a positive spatial spillover effect on the GEG within both local and surrounding provinces. Economic expansion and social advancement are driven primarily by breakthroughs in technology.
Based on the results of the analyses of the spatial Durbin model mentioned above, the potential drivers of regional differences can be analysed in depth from multiple dimensions. Differences in the foundation of fintech development is one of the important factors leading to regional divergence. With their strong economic strength and advanced technological infrastructure, developed regions are able to invest a lot of resources in fintech R&D, attract high-end technical talents and innovative enterprises to cluster, and build a perfect fintech ecosystem. This first-mover advantage allows developed regions to take the lead in the use of financial technology to promote green economic growth, and the development of green economy has been effective. In contrast, due to the obvious shortcomings in the investment in technology research and development, talent reserves and the construction of financial technology infrastructure, less developed regions face many obstacles in the use of financial technology to help the development of the green economy, resulting in the development of the green economy is relatively lagging behind, and thus widening the gap between the development of the green economy between the regions.
The suitability of the industrial structure of each region to the green economy also has a key impact on regional differences. Regions dominated by traditional industries and with a high proportion of heavily polluting industries face great challenges in the transition to a green economy. Although the demand for fintech in such regions is urgent, the transition is difficult due to the complex technical, financial and employment issues involved in industrial restructuring. Fintech is constrained by industrial structure in promoting green economic growth in such regions, making it difficult to give full play to its effectiveness. On the other hand, those regions with mainly service and high-tech industries, whose industrial structure is more in line with the concept of green economy development, can quickly and deeply integrate with fintech and accelerate the development of green industries with the help of the power of fintech to realise the rapid growth of green economy. This difference in industrial structure makes different regions show obvious regional heterogeneity in the process of fintech-driven green economic growth.
Policy support and orientation are also potential driving factors that cannot be ignored. Some regions have actively formulated and implemented a series of targeted policies and measures, such as tax incentives, financial subsidies and special development plans, to encourage financial institutions to carry out green fintech business and guide social capital investment in green industries. These policies have created a favourable policy environment for the integration and development of fintech and green economy, stimulated the enthusiasm and creativity of market players, and strongly promoted the development of green economy. However, in some regions, due to insufficient policy support or unclear guidance, financial institutions and enterprises cannot be effectively mobilised to participate in the development of the green economy, limiting the application and innovation of fintech in the field of the green economy, leading to the slow development of the green economy and further aggravating the imbalance of development between regions.
The fintech-related keyword search index can reflect the fintech (Ripberger, 2011). Therefore, we refer to Lyons to construct the fintech index (Lyons et al., 2022), replace the original explanatory variables, and conduct robustness tests (Table 7). Under both weighting matrices, local FinTech still significantly promotes local GEG. The results are stable. Observing the spatial spillover effect of fintech on GEG, under both matrices, the development of fintech shows negative spatial spillover to neighbouring regions and regions with close economic ties. This is because the fintech indicators used in the robustness test are statistically quantified for keywords on the Internet, and digital finance attracts talent to the local area, thus dampening the GEG of the neighbouring region.
Add the time lag term GEG lagged by one period to construct a dynamic spatial Durbin model (DSDM) to test the long-term dynamic effects and spatial spillover persistence of fintech innovation. The results (Table 8) show that the first-order lag term of green economic development will promote the local GEG, reflecting the dynamic and continuous characteristics of China’s provincial GEG. The estimation results of the dynamic spatial panel model also further support the reliability of the previous regression analyses; that is, there is indeed a facilitating effect of fintech on the green economy.
Different regions have diverse mechanisms of linking and influencing technological innovation and ecosystem resources due to differences in economic development. Therefore, a ‘unilateral' effort to build a green economy may not achieve the desired results (Liu and Dong, 2021). As such, this paper analyses regional heterogeneity by categorizing the employed provinces into three regions: central, eastern and western. Under the neighbourhood matrix, except for the western region, which fails the significance test, the Fintech innovations in the other two regions contribute significantly to the regional GEG (Table 9). Combining the aforementioned findings, it may be concluded that the influence of FI on GEG is significantly regionally heterogeneous, meaning that hypothesis H2 holds. Regional heterogeneity is caused by variations in the three regions' economic standing. Geographically, the eastern region has a distinct resource endowment, a faster economic growth rate and a higher degree of fintech innovation. In addition, urban agglomerations such as the Yangtze River Delta have laid the groundwork for regional development, putting them ahead of other regions in terms of FI and GEG. The contribution of fintech in promoting GEG is not evident in the Western region. These factors include a lack of resources, a low level of economic development, a lack of municipal support in promoting financial innovation and green economy development, a lack of motivation, and inefficiency.
In addition, the study of the eastern region shows that fintech innovation has a positive spatial spillover effect on the GEG of neighbouring regions. The eastern region has an inherent advantage in fintech, and the policy measures formulated by the eastern regional governments have a certain demonstration effect on the neighbouring regions, thus generating a positive spillover effect.
In terms of specific provinces, Beijing, Shanghai, Jiangsu and Zhejiang, as one of the most economically developed regions in China, have abundant capital resources and advanced financial service systems. The application of fintech has made it easier for green economy projects in these regions to obtain financial support. Fintech has helped to develop new types of financial instruments, such as carbon trading and green insurance, to further promote the GEG. The governments of Shanghai, Guangdong, Beijing and Zhejiang have provided policy support for the development of green economy and fintech, including tax incentives, capital subsidies and policy guidance. These policies have encouraged the integration and development of fintech and green economy. Concurrently, residents in these regions are more aware of environmental protection and have a growing demand for green products and services, providing a favourable market environment for the green economy.
Western provinces, such as Sichuan, are dominated by agriculture and heavy industry, with relatively weak green economy industries. Although Sichuan Province has been more active in the development of fintech, it has mainly focused on traditional finance, with less support for the green economy. Yunnan Province is dominated by agriculture and tourism, and GEG is relatively lagging. Environmental awareness in Yunnan Province is relatively low, and there is limited market demand for green consumption and green industry development, which also affects the role of fintech in GEG.
To reduce regional inequalities in green economy opportunities, innovation in financial technology can be leveraged in a number of ways. In terms of strengthening financial infrastructure, the Government, in conjunction with financial institutions, should set up a special fund for regions with weak financial infrastructure, such as the central and western regions, and increase investment in key areas such as network communications and payment and clearing systems, so as to improve the coverage and quality of financial technology services, reduce the difficulty of accessing services for green economy projects, and narrow the gap with the east in terms of the accessibility of financial services. In terms of enhancing the application capacity of fintech, fintech knowledge popularisation activities have been carried out online and offline in the central and western regions, and special training has been conducted for green enterprises and entrepreneurs to enhance their knowledge and application capacity of fintech products and services. At the same time, colleges and vocational schools are encouraged to set up relevant majors to cultivate local talents, and to attract talents from the eastern region for exchange or attachment to enrich the talent pool. In terms of promoting regional fintech cooperation, establish a fintech cooperation mechanism between developed regions in the east and less developed regions in the central and western parts of the country, whereby fintech enterprises in the east can set up branches in the central and western parts of the country or export their technologies to achieve technological sharing, set up a cross-regional green fintech service platform, integrate regional resources, and help green projects in the central and western parts of the country to dock with the national and even global funds and technologies, so as to promote the synergistic development of the green economy.
The spatial econometric model shows that there is a significant spatial spillover effect of fintech on the development of green economy. Positive spillovers are evident in economically developed regions with a good foundation in fintech, such as Guangdong, which provides low-interest loans to neighbouring green enterprises and promotes the development of green industries by virtue of its many fintech firms and big-data risk-control technology; whereas in economically weak regions with lagging fintechs, such as some of China’s western regions, the spillovers are insignificant or even negative, due to the scarcity of professional financial talents and the difficulty in transforming scientific and technological achievements.
Policy-wise, green finance subsidies interact with tax incentives to reduce business costs, stimulate green financial service participation, and promote innovative product R&D. Subsidies incentivize fintech firms to develop financing products for green enterprises (Zhou et al., 2022b). Fintech firms use technology to accurately identify compliant projects, enhancing policy effectiveness and achieving synergistic development. Industrial structure also affects regional differences. Regions with high pollution industries face transformation difficulties, limiting fintech’s role. Service and high-tech-dominated regions integrate quickly with fintech, promoting green growth (Guan et al., 2022). Policy support and orientation are crucial; regions with active policies create favorable environments (Wu et al., 2020), while those with insufficient support face fintech application and innovation limitations, slowing green economy development and exacerbating imbalances.
Less developed regions show greater inclusivity in leveraging financial and tech innovation for green finance. Governments introduce preferential policies like tax breaks and subsidies to encourage green financial tech innovation, creating a supportive environment. Market demand for green industry is rising, with a capital-tech gap. Fintech innovation meets this demand by accurately matching green enterprises' financing needs via big data and developing supply chain finance (Gao et al., 2023). Eager to narrow gaps with developed regions, less developed areas actively collaborate with fintech firms and research institutions. Despite challenges, concerted efforts are expected to enhance fintech innovation tolerance and achieve high-quality green finance development.
In terms of data acquisition, although data from multiple sources such as statistical yearbooks and financial databases were used, there is still the problem of incomplete data. It is difficult to obtain data for some fintech segments, such as the missing data for some emerging green fintech application scenarios, which affects the comprehensiveness of the study. In terms of model setting, although the commonly used spatial econometric models are chosen, they may fail to fully consider the impact of some complex economic and social factors, such as cultural differences and social networks, on the spatial spillover effect of fintech. The scope of the study mainly focuses on the domestic regional level, and there are fewer studies on the spatial spillover effect of FinTech on the green economy among international countries, which limits the universality of the research results.
China’s unique green finance policies, such as green bonds and carbon trading schemes, may reshape the spatial spillover effects of financial and technological innovations on green economic growth (GEG) and the mechanism of regional heterogeneity through multidimensional pathways. Green bonds strengthen the “demonstration effect” of cleantech innovation in the eastern region through targeted capital injection, and their cross-regional industrial chain synergy and technological diffusion under the economic distance matrix make up for the underestimation of the spillover effect of the western region by the traditional adjacency matrix. The national carbon market, on the other hand, through the reconstruction of price signals, has given rise to the innovation of carbon financial instruments, and promoted the transfer of low-carbon technologies in the east to the central and western regions through the quota trading network, thus forming a dual spillover corridor of market-technology. In addition, policy instruments structurally reshape the path of control variable impacts: green certification standards guide FDI to cluster high-tech clean industries in the east, while the carbon account system accelerates the cross-regional replication of low-carbon models in smart cities. However, policy implementation may trigger parameter shifts in spatial econometric models. Future research can quantify the non-linear effect of carbon price threshold on the marginal contribution of central technology through dynamic spatial Durbin models, so as to more precisely analyse the spatio-temporal evolution of GEG driving mechanism under policy intervention.
This paper develops a comprehensive framework to assess GEG in each province and applies the SDM to empirically investigate financial innovation and its spatial spillover effect on GEG in China. It also utilizes the DFI compiled by Peking University to examine the heterogeneity of the impact of FI on GEG in terms of regional heterogeneity. This paper finds that: (1) According to the adjacency matrix and the economic distance matrix, fintech innovation has made a significant contribution to GEG with spatial spillovers. The result remains robust after robustness testing. (2) Regarding the regional impacts of the control variables, both FDI, Tech and Urban significantly contribute to the GEG. (3) The influence of FI on GEG varies significantly by area. Under the adjacency matrix, fintech innovation in all three regions significantly contributes to the regional GEG, except the Western region. Financial technology innovation in neighbouring regions, specifically the eastern region, has a positive spatial spillover effect on the regional GEG, with an obvious “demonstration effect”.
The Government should establish a special fund for green financial science and technology innovation to support financial technology enterprises in their green technology research and development projects. This fund could provide financial support in the form of venture capital and research grants to incentivize enterprises to engage in innovative practices in the field of green finance.
The government can promote the establishment of a green financial data-sharing platform to integrate various types of green economic and financial data. It can also provide data support and decision-making references for financial institutions and enterprises and improve the operational efficiency of green financial businesses. The government should enhance the development of unified technical standards for green financial technology applications. It should also improve the interoperability and compatibility of various financial technology products, reduce the cost of enterprise applications, and encourage the rapid development of green financial technology.
The Government can formulate a series of green finance subsidy policies to provide certain financial incentives or tax concessions to financial institutions and enterprises involved in green economy projects. This will encourage them to invest more in the field of green finance. It can create a green finance development fund to support the development of green financial products and projects, including green bonds and green credit, to provide a stable source of funding for the green economy.
By establishing a training system for fintech talents, the government can cooperate with universities, research institutes and other institutions to establish a comprehensive training system for fintech talent. The training system will offer relevant professional courses and training programs and cultivate more composite talents who are familiar with finance and technology. The government can encourage enterprises to increase their investment in employee training in the field of fintech by giving them tax incentives for talent training, etc. This will improve the technical level and professional ability of their employees.
The raw data supporting the conclusions of this article will be made available by the authors, without undue reservation.
HM: Conceptualization, Data curation, Formal Analysis, Methodology, Resources, Validation, Writing–original draft. YZ: Data curation, Formal Analysis, Investigation, Methodology, Validation, Writing–original draft, Writing–review & editing. YP: Formal Analysis, Investigation, Visualization, Writing–review & editing. RD: Formal Analysis, Visualization, Writing–review & editing. DT: Formal Analysis, Project administration, Supervision, Writing–review & editing.
The author(s) declare that no financial support was received for the research, authorship, and/or publication of this article.
The authors declare that the research was conducted in the absence of any commercial or financial relationships that could be construed as a potential conflict of interest.
The author(s) declare that no Generative AI was used in the creation of this manuscript.
All claims expressed in this article are solely those of the authors and do not necessarily represent those of their affiliated organizations, or those of the publisher, the editors and the reviewers. Any product that may be evaluated in this article, or claim that may be made by its manufacturer, is not guaranteed or endorsed by the publisher.
Ahakwa, I., Xu, Y., and Tackie, E. A. (2023). Greening human capital towards environmental quality in Ghana: insight from the novel dynamic ARDL simulation approach. Energy Policy 176, 113514. doi:10.1016/j.enpol.2023.113514
Almansour, M. (2023). Artificial intelligence and resource optimization: a study of Fintech start-ups. Resour. Policy 80, 103250. doi:10.1016/j.resourpol.2022.103250
Cao, J., Law, S. H., Samad, A., Mohamad, W., Wang, J., and Yang, X. (2021). Impact of financial development and technological innovation on the volatility of green growth-evidence from China. Environ. Sci. Pollut. Res. Int. 28 (35), 48053–48069. doi:10.1007/s11356-021-13828-3
Chen, X., Teng, L., and Chen, W. (2022). How does FinTech affect the development of the digital economy? Evidence from China. North Am. J. Econ. Finance 61, 101697. doi:10.1016/j.najef.2022.101697
Cheng, M., Wang, J., Yang, S., and Li, Q. (2024). The driving effect of technological innovation on green development: from the perspective of efficiency. Energy Policy 188, 114089. doi:10.1016/j.enpol.2024.114089
Dabbous, A., and Aoun Barakat, K. (2023). The road towards environmental sustainability: investigating the role of information and communication technologies and green technology innovations. J. Clean. Prod. 432, 139826. doi:10.1016/j.jclepro.2023.139826
Ding, N., Gu, L., and Peng, Y. (2022). Fintech, financial constraints and innovation: evidence from China. J. Corp. Finance 73, 102194. doi:10.1016/j.jcorpfin.2022.102194
Dong, H., Zheng, Y., and Tang, Y. (2024). Impact of FinTech on the industrial structural transformation: evidence from China's resource-based cities. Resour. Policy 91, 104833. doi:10.1016/j.resourpol.2024.104833
Doré, N. I., and Teixeira, A. A. C. (2023). The role of human capital, structural change, and institutional quality on Brazil's economic growth over the last two hundred years (1822–2019). Struct. Change Econ. Dyn. 66, 1–12. doi:10.1016/j.strueco.2023.04.003
Elhorst, J. P. (2012). Matlab software for spatial panels. Int. Regional Sci. Rev. 37 (3), 389–405. doi:10.1177/0160017612452429
Feng, Y., Wang, X., Du, W., Wu, H., and Wang, J. (2019). Effects of environmental regulation and FDI on urban innovation in China: a spatial Durbin econometric analysis. J. Clean. Prod. 235, 210–224. doi:10.1016/j.jclepro.2019.06.184
Gao, Q., Cheng, C., and Sun, G. (2023). Big data application, factor allocation, and green innovation in Chinese manufacturing enterprises. Technol. Forecast. Soc. Change 192, 122567. doi:10.1016/j.techfore.2023.122567
Guan, S., Liu, J., Liu, Y., and Du, M. (2022). The nonlinear influence of environmental regulation on the transformation and upgrading of industrial structure. Int. J. Environ. Res. Public Health 19 (14), 8378. doi:10.3390/ijerph19148378
Guo, F., Wang, J. I., Wang, F., Kong, T., Zhang, X., and Cheng, C. Y. (2020). Measuring the development of digital inclusive finance in China: indexing and spatial characterization. Econ. Q. 19 (04), 1401–1418. doi:10.13821/j.cnki.ceq.2020.03.12
Guo, J., Fang, H., Liu, X., Wang, C., and Wang, Y. (2023). FinTech and financing constraints of enterprises: evidence from China. J. Int. Financial Mark. Institutions Money 82, 101713. doi:10.1016/j.intfin.2022.101713
Hu, N., and Zheng, B. (2023). Natural resources, education, and green economic development. Resour. Policy 86, 104053. doi:10.1016/j.resourpol.2023.104053
Huang, S. (2022). Does FinTech improve the investment efficiency of enterprises? Evidence from China’s small and medium-sized enterprises. Econ. Analysis Policy 74, 571–586. doi:10.1016/j.eap.2022.03.014
Huang, Y., Xiong, N., and Liu, C. (2024). Renewable energy technology innovation and ESG greenwashing: evidence from supervised machine learning methods using patent text. J. Environ. Manag. 370, 122833. doi:10.1016/j.jenvman.2024.122833
Khoshnava, S. M., Rostami, R., Zin, R. M., Kamyab, H., Abd Majid, M. Z., Yousefpour, A., et al. (2020). Green efforts to link the economy and infrastructure strategies in the context of sustainable development. Energy 193, 116759. doi:10.1016/j.energy.2019.116759
Li, R., Li, L., and Wang, Q. (2022). The impact of energy efficiency on carbon emissions: evidence from the transportation sector in Chinese 30 provinces. Sustain. Cities Soc. 82, 103880. doi:10.1016/j.scs.2022.103880
Li, R., Wang, Q., and Guo, J. (2024). Revisiting the environmental kuznets curve (EKC) hypothesis of carbon emissions: exploring the impact of geopolitical risks, natural resource rents, corrupt governance, and energy intensity. J. Environ. Manag. 351, 119663. doi:10.1016/j.jenvman.2023.119663
Liao, B., and Li, L. (2022). Spatial division of labor, specialization of green technology innovation process and urban coordinated green development: evidence from China. Sustain. Cities Soc. 80, 103778. doi:10.1016/j.scs.2022.103778
Lin, B., and Zhou, Y. (2022). Measuring the green economic growth in China: influencing factors and policy perspectives. Energy 241, 122518. doi:10.1016/j.energy.2021.122518
Liu, J., Zhang, Y., and Kuang, J. (2023). Fintech development and green innovation: evidence from China. Energy Policy 183, 113827. doi:10.1016/j.enpol.2023.113827
Liu, Y., and Dong, F. (2021). How technological innovation impacts urban green economy efficiency in emerging economies: a case study of 278 Chinese cities. Resour. Conservation Recycl. 169, 105534. doi:10.1016/j.resconrec.2021.105534
Liu, Y., Wang, Z., and Mai, S. (2024). Big data development and enterprise green innovation: text analysis of listed companies’ annual reports. Int. Rev. Econ. and Finance 96, 103703. doi:10.1016/j.iref.2024.103703
Liu, Z., Sun, Y., Xing, C., Liu, J., He, Y., Zhou, Y., et al. (2022). Artificial intelligence powered large-scale renewable integrations in multi-energy systems for carbon neutrality transition: challenges and future perspectives. Energy AI 10, 100195. doi:10.1016/j.egyai.2022.100195
Luo, Y., Salman, M., and Lu, Z. (2021). Heterogeneous impacts of environmental regulations and foreign direct investment on green innovation across different regions in China. Sci. Total Environ. 759, 143744. doi:10.1016/j.scitotenv.2020.143744
Lyons, A. C., Kass-Hanna, J., and Fava, A. (2022). Fintech development and savings, borrowing, and remittances: a comparative study of emerging economies. Emerg. Mark. Rev. 51, 100842. doi:10.1016/j.ememar.2021.100842
Ma, R., Xie, X. q., Liu, B., Zhou, F., and Samsurijan, M. S. b. (2023). Transmission to green economic development and the dependence on natural resources in China. Resour. Policy 86, 104211. doi:10.1016/j.resourpol.2023.104211
Nenavath, S., and Mishra, S. (2023). Impact of green finance and fintech on sustainable economic growth: empirical evidence from India. Heliyon 9 (5), e16301. doi:10.1016/j.heliyon.2023.e16301
Ni, L., Ahmad, S. F., Alshammari, T. O., Liang, H., Alsanie, G., Irshad, M., et al. (2023). The role of environmental regulation and green human capital towards sustainable development: the mediating role of green innovation and industry upgradation. J. Clean. Prod. 421, 138497. doi:10.1016/j.jclepro.2023.138497
Pan, X., Zhang, J., Li, C., Quan, R., and Li, B. (2017). Exploring dynamic impact of foreign direct investment on China’s CO $$_{2}$$ 2 emissions using markov-switching vector error correction model. Comput. Econ. 52 (4), 1139–1151. doi:10.1007/s10614-017-9745-x
Ripberger, J. T. (2011). Capturing curiosity: using internet search trends to measure public attentiveness. Policy Stud. J. 39 (2), 239–259. doi:10.1111/j.1541-0072.2011.00406.x
Song, X., Zhou, Y., and Jia, W. (2019). How do economic openness and R&D investment affect green economic growth? evidence from China. Resour. Conservation Recycl. 146, 405–415. doi:10.1016/j.resconrec.2019.03.050
Teng, M., and Shen, M. (2023). Fintech and energy efficiency: evidence from OECD countries. Resour. Policy 82, 103550. doi:10.1016/j.resourpol.2023.103550
Wang, Q., Hu, S., and Li, R. (2024). Could information and communication technology (ICT) reduce carbon emissions? The role of trade openness and financial development. Telecommun. Policy 48 (3), 102699. doi:10.1016/j.telpol.2023.102699
Wang, Q., Zhang, F., and Li, R. (2023). Revisiting the environmental kuznets curve hypothesis in 208 counties: the roles of trade openness, human capital, renewable energy and natural resource rent. Environ. Res. 216, 114637. doi:10.1016/j.envres.2022.114637
Wang, Q., Zhang, F., and Li, R. (2024). Free trade and carbon emissions revisited: the asymmetric impacts of trade diversification and trade openness. Sustain. Dev. 32 (1), 876–901. doi:10.1002/sd.2703
Wang, Q., Zhang, F., Li, R., and Sun, J. (2024). Does artificial intelligence promote energy transition and curb carbon emissions? The role of trade openness. J. Clean. Prod. 447, 141298. doi:10.1016/j.jclepro.2024.141298
Wang, Y., Hu, H., Dai, W., and Burns, K. (2021). Evaluation of industrial green development and industrial green competitiveness: evidence from Chinese urban agglomerations. Ecol. Indic. 124, 107371. doi:10.1016/j.ecolind.2021.107371
Wu, H., Li, Y., Hao, Y., Ren, S., and Zhang, P. (2020). Environmental decentralization, local government competition, and regional green development: evidence from China. Sci. Total Environ. 708, 135085. doi:10.1016/j.scitotenv.2019.135085
Xuan, D., Jiang, X., and Fang, Y. (2023). Can globalization and the green economy hedge natural resources? Functions of population growth and financial development in BRICS countries. Resour. Policy 82, 103414. doi:10.1016/j.resourpol.2023.103414
Yin, S., Dong, T., Li, B., and Gao, S. (2022). Developing a conceptual partner selection framework: digital green innovation management of prefabricated construction enterprises for sustainable urban development. Buildings 12 (6), 721. doi:10.3390/buildings12060721
Yin, S., and Yu, Y. (2022). An adoption-implementation framework of digital green knowledge to improve the performance of digital green innovation practices for industry 5.0. J. Clean. Prod. 363, 132608. doi:10.1016/j.jclepro.2022.132608
Yin, S., and Zhao, Y. (2024). Digital green value co-creation behavior, digital green network embedding and digital green innovation performance: moderating effects of digital green network fragmentation. Humanit. Soc. Sci. Commun. 11 (1), 228. doi:10.1057/s41599-024-02691-5
Yu, D., Li, X., Yu, J., and Li, H. (2021). The impact of the spatial agglomeration of foreign direct investment on green total factor productivity of Chinese cities. J. Environ. Manage 290, 112666. doi:10.1016/j.jenvman.2021.112666
Yu, G., and Liu, K. (2024). Foreign direct investment, environmental regulation and urban green development efficiency—an empirical study from China. Appl. Econ. 56, 2738–2751. doi:10.1080/00036846.2023.2200232
Yu, H., Wang, J., Hou, J., Yu, B., and Pan, Y. (2023). The effect of economic growth pressure on green technology innovation: do environmental regulation, government support, and financial development matter? J. Environ. Manag. 330, 117172. doi:10.1016/j.jenvman.2022.117172
Zhang, Z., Zhou, Z., Zeng, Z., and Zou, Y. (2023). How does heterogeneous green technology innovation affect air quality and economic development in Chinese cities? Spatial and nonlinear perspective analysis. J. Innovation and Knowl. 8 (4), 100419. doi:10.1016/j.jik.2023.100419
Zhao, X., Ma, X., Shang, Y., Yang, Z., and Shahzad, U. (2022a). Green economic growth and its inherent driving factors in Chinese cities: based on the Metafrontier-global-SBM super-efficiency DEA model. Gondwana Res. 106, 315–328. doi:10.1016/j.gr.2022.01.013
Zhao, X., Mahendru, M., Ma, X., Rao, A., and Shang, Y. (2022b). Impacts of environmental regulations on green economic growth in China: new guidelines regarding renewable energy and energy efficiency. Renew. Energy 187, 728–742. doi:10.1016/j.renene.2022.01.076
Zhou, B., and Wang, Q. (2024). FinTech matters in sustainable finance: does it redistribute the supply of financial services? J. Int. Financial Mark. Institutions Money 91, 101913. doi:10.1016/j.intfin.2023.101913
Zhou, G., Zhu, J., and Luo, S. (2022a). The impact of fintech innovation on green growth in China: mediating effect of green finance. Ecol. Econ. 193, 107308. doi:10.1016/j.ecolecon.2021.107308
Keywords: fintech innovation, green economic growth, spatial spillover effects, spatial durbin model, policy implications
Citation: Ma H, Zhou Y, Pan Y, Dong R and Tang D (2025) Spatial spillover of fintech innovation on green economic growth based on 30 provinces in China. Front. Environ. Sci. 13:1514403. doi: 10.3389/fenvs.2025.1514403
Received: 20 October 2024; Accepted: 17 February 2025;
Published: 12 March 2025.
Edited by:
Mobeen Ur Rehman, Keele University, United KingdomReviewed by:
Sandeep Poddar, Lincoln University College, MalaysiaCopyright © 2025 Ma, Zhou, Pan, Dong and Tang. This is an open-access article distributed under the terms of the Creative Commons Attribution License (CC BY). The use, distribution or reproduction in other forums is permitted, provided the original author(s) and the copyright owner(s) are credited and that the original publication in this journal is cited, in accordance with accepted academic practice. No use, distribution or reproduction is permitted which does not comply with these terms.
*Correspondence: Yuhan Pan, MjAyMjEyMjQwMDQwQG51aXN0LmVkdS5jbg==; Decai Tang, dGFuZ2RlY2FpQG51aXN0LmVkdS5jbg==
Disclaimer: All claims expressed in this article are solely those of the authors and do not necessarily represent those of their affiliated organizations, or those of the publisher, the editors and the reviewers. Any product that may be evaluated in this article or claim that may be made by its manufacturer is not guaranteed or endorsed by the publisher.
Research integrity at Frontiers
Learn more about the work of our research integrity team to safeguard the quality of each article we publish.