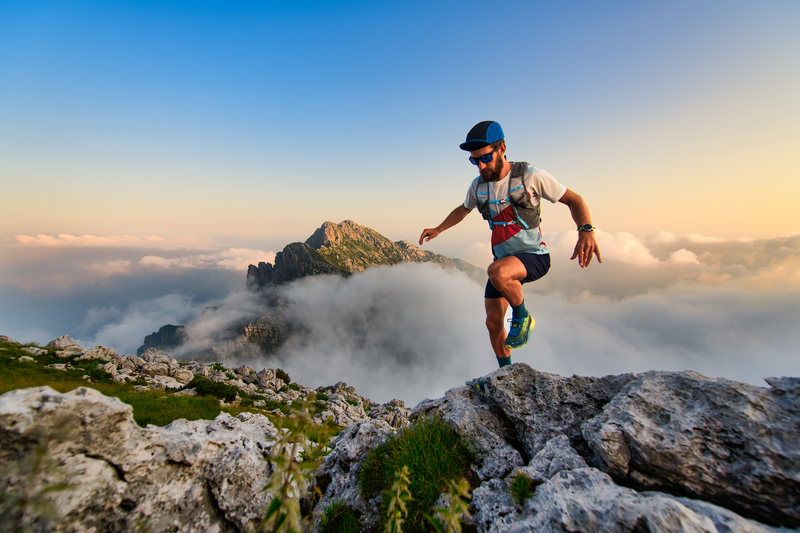
94% of researchers rate our articles as excellent or good
Learn more about the work of our research integrity team to safeguard the quality of each article we publish.
Find out more
ORIGINAL RESEARCH article
Front. Environ. Sci. , 31 January 2025
Sec. Environmental Economics and Management
Volume 13 - 2025 | https://doi.org/10.3389/fenvs.2025.1511469
Breakthroughs in green innovation (BGI) have become increasingly prominent in spearheading green technology, while intelligent manufacturing (IM) offers a fresh technical paradigm for the manufacturing industry’ green development. Yet, due to the limitations in measuring BGI, existing research on IM and BGI has been ignored. By devising a ground-breaking approach to BGI, this paper takes the IM pilot demonstration projects as an ideal quasi-natural experiment and investigates the influence of IM on BGI. Our findings indicate that IM can effectively enhance BGI which are further validated by a series of rigorous examinations. Further mechanism analysis reveals that crowding-in R&D resources, strengthening green open innovation, and alleviating agency conflict play potential pathways in bridging the nexus between IM and BGI. A heterogeneity analysis highlights that IM has the potential to disrupt the technological path dependency observed in high-pollution and high-energy consumption industries. Further research suggests that IM can form a joint effect with environmental regulations to promote BGI. BGI driven by IM can also improve both the firm’s economic and environmental, social, and governance (ESG) performance, leading to a “win-win” scenario for economic performance and green development. Our study confirms that promoting IM in emerging countries is indispensable for enhancing BGI, which serves as the new impetus for green development.
As the key driving force of green technological innovation, a breakthrough in green innovation (BGI) is an important breaching point in driving the green transformation, as well as represents a significant focus of China’s sustainable development (Shahzad et al., 2022). Unlike traditional green innovation, BGI exhibits three notable characteristics. First, BGI places a greater emphasis on the novelty of green technology, aiming to break away from the path dependence on traditional technology (Datta and Srivastava, 2023) and to reshape and create new competitive nodes for green technologies. The realization of this process requires the continuous disruption of the company’s original technological paradigm, leading to longer innovation cycles and higher risks, and thus necessitating greater R&D investment. Second, BGI emphasizes the diversity of knowledge sources and has a greater demand for external innovation resources (West and Bogers, 2014). BGI entails the assimilation and creation of new knowledge, and firms may face greater challenges in relying solely on their own knowledge base to achieve breakthroughs in cutting-edge technologies (Chesbrough, 2003). They often require the integration of more diverse external knowledge to provide the foundation for novel green technologies and products. Third, the process of BGI is more complex and may involve more significant information asymmetry (Feller et al., 2009). In contrast to incremental innovations, which merely repeat or make only minor expansions from the original (Maslach, 2016; Wang et al., 2021), BGI represents a higher level of green innovation behavior that can genuinely drive technological change. It necessitates extensive communication and cooperation across multiple sectors and segments. The innovation process of BGI is more tedious and complex, characterized by higher levels of information asymmetry. In the green economy era, BGI not only fosters green development but also enhances the core competitiveness of enterprises. In this context, how to improve the BGI of enterprises is a frontier issue worthy of in-depth study.
As the heart of the 4th industrial revolution, intelligent manufacturing (IM) represents a novel production model characterized by capabilities in self-perception, self-learning, self-decision-making, self-execution, and self-adaptation. IM may transform the traditional production mode of companies, significantly impacting their production and operation (Shang and You, 2019). In the context of vigorously promoting intelligent transformation and green development (Wei et al., 2024), how will IM affect the BGI of enterprises? Research on this issue is at the frontier of environmental and innovation economics and holds significant practical importance for manufacturing powers’ green transformation (Yang et al., 2022), nevertheless, there is little discussion about the relationship between IM and BGI. Three related research branches on breakthrough innovation, green innovation, and IM provide an important reference for our study.
First, research on breakthrough innovation within the field of innovation economics offers a crucial framework for the exploration of BGI. To our knowledge, there are limited studies on the topic of BGI. However, breakthrough innovation represents a leading area in innovation economics (Esposito, 2023; Wu et al., 2024), on which scholars have made significant progress and have produced fruitful results (Byun et al., 2021; Liang et al., 2023). Breakthrough innovation is frequently regarded as a disruptive technology that incorporates both novel and highly impactful elements (Berkes and Gaetani, 2021). Currently, studies of breakthrough innovation can be broadly categorised into two main branches. On the one hand, many scholars have studied its economic performance. Breakthrough innovation can boost the competitive advantage of businesses (Cho and Kim, 2017) while also acting as a crucial driver for social progress and economic growth (Baba and Walsh, 2010; Sharif, 2012). On the other hand, with the increasing prominence of breakthrough innovation, exploring the driving factors behind it has become a key research focus in innovation economics (Fabra et al., 2023). Some studies have indicated that factors like industry competition (Davis and Tomoda, 2018), technological environment dynamics (Esposito, 2023), and government policies (Zhang et al., 2023) can affect breakthrough innovation. Unfortunately, there is a notable dearth of systematic studies on BGI. In this study, we integrate the research methodologies of breakthrough innovation into the realm of green innovation. Our study broadens the horizons of innovation and environmental economics.
Second, another strand of literature closely related to BGI is green innovation. The positive externalities of knowledge spillover and the negative externalities of pollution may lead companies to be unwilling to participate in green innovation (Rennings, 2000). Numerous studies on green innovation focus on the negative punishment policies represented by environmental protection tax (Wang et al., 2024a), and the positive incentive policies represented by government tax incentives (Wang C. et al., 2022) and financial support (Han et al., 2024). With the development of the digital economy, numerous scholars have found that the new generation of information technology, represented by digital transformation, the Internet, and IM, not only enhances green total factor productivity (Yang and Shen, 2023; Ge et al., 2024) but also optimizes the allocation of factor resources (Gao et al., 2023), thereby promoting corporate green innovation (Qi et al., 2024; Zhang et al., 2024; Ma and Lin, 2024). However, it is worth noting that these studies tend to examine the impact of IM on regional green production or include IM within the context of digitization to explore the effect of the digital economy on firms’ green innovation. This approach may overlook the unique characteristics of IM itself (Zhong et al., 2017; Zhou et al., 2018) and thus underestimate its role in fostering green innovation in manufacturing firms. As a new production methodology that emphasizes the deep integration of new-generation information and communication technologies with advanced manufacturing technologies1, IM is the fusion and transcendence of digitization and automation (Zhou et al., 2018). It aims to improve the quality and efficiency of the entire manufacturing process (Zhong et al., 2017) and to enhance the greening of the manufacturing industry through the use of smart technologies (Yang and Shen, 2023; Shen and Zhang, 2023; Jin and Chen, 2024). Based on the characteristics of manufacturing firms, extracting IM from a broad range of digital technologies and exploring its impact on corporate green innovation can more precisely identify the green innovation effects of new production methods on micro firms. Some scholars have confirmed the positive role of IM in promoting corporate green innovation (Jin and Chen, 2024; Wei et al., 2024), which provides valuable insights for our study. However, these studies often view corporate green innovation as a homogeneous concept, using the number of green patents as a measure of corporate green innovation (Jin and Chen, 2024). This approach may fail to capture the variability in corporate green patents, particularly their technological breakthroughs, and therefore overlook how IM specifically influences BGI in the manufacturing industry. Based on this, we use the newly entry category of green invention patents to measure the BGI of enterprises and further examine the impact of IM on BGI. This not only provides new insights into exploring the influence of new production methods on the green innovation of micro-enterprises in the digital economy era but also offers valuable references for accelerating the green transformation of manufacturing country.
Third, the discussion of IM also provides valuable reference for this paper. IM is a pivotal element of the new round of technological revolution, which can fundamentally transform the paradigm of production technology (Acemoglu and Restrepo, 2020). Some studies suggests that IM not only boosts the operational efficiency of enterprises (Li et al., 2023) but also improves their environment performance (Lyu et al., 2023). Additionally, some scholars have investigated the connection between IM and innovation (Lee, 2023; Liu et al., 2020). With the popularization of IM application and increasingly prominent environmental problems, research on the green innovation effects of IM has gradually attracted extensive attention from scholars (Han and Mao, 2023; Lee et al., 2022). Xu et al. (2023) highlighted that enterprises can leverage artificial intelligence to facilitate green transformation in heavily polluting industries, thereby achieving a significant advancement in green innovation. Jin and Chen (2024) discovered that IM fosters green innovation through easing financing constraints, encouraging green employment, boosting environmental subsidies, and strengthening the disclosure of environmental information. However, because BGI remains a weak link in index measurement, discussions on IM and BGI are scarce in existing research. BGI is a form of green innovation way that emphasizes breaking the traditional technical paths and reflecting substantive innovation, which has a far-reaching impact on advancing green development. Therefore, it is vital to explore the relationship between IM and BGI.
As a key strategy to accelerate the digital, intelligent, and green transformation of the manufacturing sector, IM pilot demonstration projects (IMPP), an exogenous event, provides a suitable opportunity for our research. Utilizing panel data from Chinese A-share manufacturing companies, we regard IMPP as a quasi-natural experiment and apply time-varying difference-in-differences (TDID) to assess the impact of IM on BGI. Our findings suggest that IM can promote corporate BGI by crowding-in R&D resources, enhancing green open innovation (GOPI), and mitigating agency conflict. Furthermore, our study explores the heterogeneous characteristics promoted by IM in terms of industrial pollution, energy consumption, and regional environmental regulation. Though our analysis, we demonstrate that BGI can improve both the economic and environmental, social, and governance (ESG) performance of companies.
There are three significant contributions in this study.
First, we meticulously construct a BGI index by focusing on the technical aspects of International Patent Classification (IPC). The method is a new perspective for research on green innovation. While there has been extensive exploration on green innovation (Yan et al., 2024) and breakthrough innovation (Capponi et al., 2022; Esposito, 2023), the scarcity of empirical studies on BGI may be attributed to the constraints in its construction. We integrated the cutting-edge domain of innovation economics, specifically breakthrough innovation, into the realm of green innovation. Our findings broaden the scope of green innovation and enrich the research of environmental economics.
Second, based on the three characteristics of BGI, our study delves into a comprehensive analysis of the intricate relationship between IM and BGI, and finds that R&D resources, GOPI, and agent conflict are the mechanisms through which IM affects BGI. Numerous studies have explored the correlation among IM, technological innovation (Liu et al., 2020), and green development (Yang and Liu, 2024). However, in contrast to traditional green innovation, BGI not only needs to break the traditional technical track but also has a higher demand for diversified knowledge, which leads to greater risks and stronger information asymmetry in BGI. This paper incorporates IM and BGI into the same research framework, thereby enriching the current literature on the economic performance of IM and offering new perspectives into the nexus between technology paradigms and green innovation in the digital economy era.
Third, our study also examines the heterogeneity surrounding the impact of IM on BGI performance, thereby offering valuable empirical evidence to enhance the effectiveness of IM. On one hand, our study highlights that IM can enhance BGI in high-pollution and high-energy consumption industries. This conclusion adds a fresh perspective on breaking path dependence within the manufacturing department. On the other hand, we find that IM will synergize with environmental regulations to enhance BGI, which enriches Porter’s theory in the digital economy era.
The rest of this paper is structured as follows: Section 2 elaborates a theoretical analysis and proposes the research hypotheses. Section 3 outlines the construction of indicators, data, and models. Section 4 exhibits the regression results, covering the benchmark regression, robustness tests, mechanism analysis, heterogeneity analysis, and consequence analysis. Section 5 details the conclusions and implications.
In recent years, emerging digital technologies such as artificial intelligence, blockchain, cloud computing, and big data have flourished, and IM is increasingly becoming the core strategic direction of global technological transformation. The advanced manufacturing industry of the United States, Germany’s Industry 4.0, France’s New Industrial France Plan, Japan’s robotics strategy, South Korea’s information technology integration and development strategy, among others, all emphasize the importance of industrial intelligence. The utilization of the Internet and other new-generation information technologies to drive the transformation of the manufacturing industry has become a common choice among countries worldwide. To encourage enterprises to adopt IM and achieve the status of a strong manufacturing country, China’s Ministry of Industry and Information Technology (MIIT) has continuously issued several policy documents between 2015 and 2018, including the “Circular of the MIIT on Announcing the List of IMPP,” the “Development Plan of IM (2016–2020),” and the “IM Project Implementation Guidelines (2016–2020),” all of which clearly outline the implementation of the IMPP.
Regarding the implementation of the IMPP, China employs a method that involves first piloting the program and then promoting it through exemplary practices. The specific process is as follows: initially, local governments recommend enterprises to the MIIT based on whether they meet the threshold conditions of the IMPP; subsequently, after obtaining the approval from the relevant departments, MIIT formally publishes the list of enterprises participating in the IMPP. The policy requires that following the implementation of the IMPP, enterprises must substantially enhance their productivity and resource utilization. Concurrently, it explicitly emphasizes that traditional manufacturing enterprises should intensify their green transformation and advance the greening of critical manufacturing technologies. As a significant initiative in response to the IM, the MIIT has selected 305 pilot enterprises between 2015 and 2018, encompassing 31 provinces and 92 industries across China. This initiative has accumulated substantial practical experience, which is vital for the exploration of IM models. According to the summary of initiatives related to the “14th Five-Year Plan” for IM development, following the implementation of the IMPP, the production efficiency of IM pilot enterprises has risen by an average of 45%, defective products have decreased by an average of 35%, and the product development cycle has notably shortened. Consequently, the level of IM within these enterprises has been significantly enhanced.
BGI is a key driving force for the manufacturing department to optimize resource allocation and achieve environmental governance. However, compared to traditional green innovation, BGI may entail greater innovation risks, require more knowledge sources, and have stronger information asymmetry in the green innovation process. As a new mode of production, IM can provide technical support for enterprise production by integrating big data, large computing power and strong algorithms (Wang J. et al., 2022), which is conducive to promoting BGI.
Based on the resource-based theory (Wernerfelt, 1984), firms’ innovation activities are characterized by long input-output cycles, high risk, and the non-recoverability of initial investments. These activities typically require substantial amounts of capital, human resources, experimental equipment, and other resources to maintain a competitive advantage (Barney, 1991; Peteraf, 1993; Bharadwaj, 2000). In particular, compared with traditional green innovation, BGI emphasizes exploration and breakthroughs in new technological fields. They typically entail higher levels of risk and uncertainty, and the implementation of its R&D activities faces greater constraints in terms of innovation resources, such as capital and talent. However, IM has the potential to transform firms’ traditional production models and innovation processes (Ribeiro-Navarrete et al., 2021) and to invigorate green innovation of firms (Jin and Chen, 2024). This will crowd in corporate R&D resources, thereby providing ample resource support for enterprises to break the path dependence of technology choice and foster BGI.
First, IM can drive enterprise’s digital transformation (Zhou et al., 2018), aiding in the stimulation of their green innovation power potential. On the one hand, IM has created a lot of data and changed the innovation model of enterprises (Gao et al., 2020; Kusiak, 2017). In the industrial economy era, the corporate green innovation process primarily relies on a linear chain model of knowledge accumulation, research, and application. With the support of intelligent technology, big data, as a new production factor, participates in the innovation process (Chatterjee et al., 2022). This can not only promote the digitalization of enterprise production processes but also facilitate the digitalization of consumer information. New data from the production department, as well as new green ideas and demands from consumers, will be transmitted to the R&D department in a more convenient way. Under these circumstances, the boundaries between R&D and production within enterprises, as well as between manufacturers and consumers, will become increasingly blurred, and the process of innovation will gradually integrate (Nambisan et al., 2017). The data generated by IM have revolutionized the traditional innovation mode, creating a notable “multiplier effect” in green innovation. These shifts will drive companies to boost their investment in green innovation, helping them to acquire a competitive advantage by advancing BGI.
On the other hand, IM can also enhance enterprises’ big data mining capabilities and stimulate their innovation potential (Mikalef et al., 2019). In the IM mode, powerful computing power and accurate algorithms have become an important support for enterprises to quickly analyse massive data. In the face of scattered and complex data with different structures, big data can strengthen enterprises’ process ability for unstructured and non-standardized data, and fully mine high-value data elements through coding and integration of data (Akter et al., 2019). On the production side, IM brings an organic combination of digital technology and traditional means of production, enabling production data within the enterprise to be fully mined and helping the enterprise to discover the hidden information among data elements, thus providing more original data elements for green innovation activities. On the consumer side, intelligent technology can mine customers’ green data using machine learning and data mining (Hamilton and Sodeman, 2020), realize real-time interaction between enterprises and customers (Cappa et al., 2021), form a customer-centered design network and design thinking, and depict the ‘user portraits’ of consumers from multiple angles. By processing and analysing valuable user information that supports product innovation (Shukla et al., 2019), enterprises can promptly transform it into efficient and accurate green innovation behaviour, thereby identify the optimal plan during the green innovation process. This will stimulate their green innovation vitality, and enhance their investment in green innovation, thereby promote enterprises’ BGI.
Second, IM can optimize the labor structure of enterprises and contribute to enhancing the quality of human capital. IM has a substitution effect on the production personnel who do repetitive and programmed work (Acemoglu and Restrepo, 2019; Acemoglu and Restrepo, 2020; Bessen, 2019), but it has a complementary effect on non-repetitive and non-programmed R&D personnel (Furman and Seamans, 2019). The combined influence of these two factors has significantly promoted the upgrading of the labor skill structure in enterprises. According to the theory of human capital (Becker, 2009), a highly skilled labor force with stronger knowledge reserves and professionalism is not only facilitates innovation (Diebolt and Hippe, 2019), which can promote breakthroughs in core green technologies, but also enhances enterprises’ understanding of sustainable development concepts, such as green and environmental protection, thereby promoting enterprises to pursue long-term green development. Therefore, the upgrading of labor structure will help enterprises increase their investment in green innovation and promote BGI.
BGI places a strong emphasis on the development and creation of new technologies, which require the continual search, acquisition, and accumulation of new knowledge. This process aims to achieve transformations from quantitative to qualitative levels and ultimately yield breakthrough outcomes. It is challenging for enterprises to rely solely on their traditional closed green innovation approaches to achieve these objectives; therefore, they often need to engage in GOPI with multi-agent cooperation. According to the open innovation theory (Chesbrough, 2003), effectively integrating internal and external innovation resources (Teece, 2007) and promoting the exchange of diverse knowledge and ideas across organizational boundaries (Chesbrough and Bogers, 2014) are crucial for enhancing breakthrough innovation within enterprises (Wang et al., 2024b). IM can facilitate GOPI and enrich the diversity of external knowledge sources. With the assistance of intelligent platforms, the inflow of a large number of heterogeneous resources helps expand the boundaries of enterprise knowledge search and continuously optimizes the existing knowledge infrastructure. This process enhances companies’ capabilities to tackle complex green technologies, thereby fostering the emergence of BGI.
First, IM can reduce the cost of cooperation among various green innovation entities. IM can use digital technology to convert vast amounts of information into binary digital resources, facilitating the liquefaction of these resources. Liquefied resources can accelerate the speed and frequency of knowledge and information flowing among various green innovative entities, break the spatial limitations of knowledge spillover in the traditional sense, and promote the deep integration and complementary advantages of green innovative resources between enterprises, as well as between enterprises and universities and relevant research institutes (Fernández-Mesa et al., 2014). Diversified knowledge, technology, and information are interconnected among innovation entities (Perez-Arostegui et al., 2012), further expanding the network boundary of GOPI and strengthening the connections among green innovation entities. IM reduces the cost of cooperation between enterprises and other green innovative entities, and facilitates the development of BGI.
Second, IM will reduce the cost of searching for green innovative partners. According to the search friction theory, due to the heterogeneity of demand among innovation entities (Tödtling et al., 2009), there are a large number of demand and supply entities in the green innovation market, and it is often necessary to pay a non-negligible search cost to achieve cooperation between innovation entities (Salge et al., 2013). However, IM can store, organize and mine the production and R&D data of green innovative entities, and establish a data pool. Further, through intelligent technologies such as matching algorithms and cluster analysis, IM can find the best partner for enterprise green innovation activities. IM will reduce the search cost among the innovative partners and improve the matching efficiency of innovation cooperation (Van Alstyne et al., 2016). This will help enterprises establish an open and efficient GOPI network, and then optimize green innovation resources and enhance BGI’s capabilities.
IM will reduce the internal information asymmetry and alleviate the agency conflict. The “hidden action” caused by moral hazard is an important obstacle to enterprise innovation (Holmstrom, 1989; Manso, 2011). Unlike traditional green innovation, BGI involves the exploration and breakthrough of high-quality innovations, making the innovation process more complex. This may lead to severe moral hazards. On the one hand, the “Type I agency conflict” between shareholders and management is more pronounced (Zardkoohi et al., 2017). Although BGI is crucial for corporate green development, the long-term nature and high level of difficulty in its R&D process may lead management to adopt short-term strategies that ultimately hinder BGI. On the other hand, the agency conflict between management and the R&D department is more salient. BGI not only involves communication and collaboration among several departments within the enterprise but also necessitates deep knowledge of market dynamics and user needs, characterized by stronger information asymmetry. Based on performance evaluations, R&D departments may exhibit caution and reluctance in taking initiatives within BGI. However, IM can optimize internal management processes (Kretschmer and Khashabi, 2020), effectively mitigate agent conflicts (Ortega-Argiles et al., 2005), and create a favorable internal environment for enhancing BGI.
First, IM can enhance the oversight of management behaviours and help mitigate “Type I agency conflict” between shareholders and management. The separation of ownership and control in enterprises results in serious information asymmetry between shareholders and management. Since management has more information during the innovation process, they may prefer short-term actions that benefit performance appraisal, but have limited motivation to pursue BGI, which would enhance corporate long-term competitiveness. However, IM can collect and monitor the behaviour and decision-making information of management in real time with the help of a big data platform, thereby to a certain extent strengthen the scope and intensity of supervision over management. This process will effectively restrain the opportunistic behaviours of enterprise management by violating rules and discipline and excessively pursuing short-term economic benefits (Menon, 2018), thus alleviating the “Type I agency conflict,” which will promote BGI.
Second, IM will change the management process and green innovation chain, which will aiding in diminishing the information asymmetry between the management and the R&D department. In the traditional mode, the enterprise organization structure is hierarchical and linear. But IM can promote the transformation of the enterprise management structure to networking and flattening (Kretschmer and Khashabi, 2020). The reduction of organizational hierarchy decreases the internal information asymmetry, promotes the efficient communication between management and the R&D department, and helps enhance the breakthrough of green technology. Moreover, IM’s dynamic tracking of the green innovation chain will further strengthen the supervision of the R&D department and reduce the strategic green innovation behaviours adopted in response to assessments. This will guide the R&D department to take more substantive innovative activities, thereby promoting BGI.
In summary, IM can promote BGI, which involves higher risk, greater requirements for knowledge integration, and stronger information asymmetry. Therefore, four research hypotheses are proposed as follows.
Hypothesis 1. IM can positively promote BGI.
Hypothesis 2. IM can promote BGI by crowding-in R&D resources.
Hypothesis 3. IM can promote BGI by strengthening GOPI.
Hypothesis 4. IM can promote BGI by alleviating agency conflict.
Figure 1 displays the theoretical framework of IM’s on BGI.
Our initial sample consists of all industrial firms listed on Chinese A-shares from 2010 to 2022. To ensure the reliability of data, we process the samples according to the following steps: 1) we exclude companies designated as “ST,” “*ST,” “suspension of listing,” or “termination of listing.” 2) firms in the financial and insurance sectors are excluded. 3) we exclude companies with a significant number of missing values in the main variables. 4) all continuous variables are winsorized at the 1% level of each tail. After filtering, we obtain 14230 firm–year observations.
Financial and corporate governance data are obtained from CSMAR databases. Patent data are sourced from incoPat databases. Significantly, our focus revolves around green invention patents, selected from the International Intellectual Property Organization (WIPO) list, which includes listed companies, their subsidiaries, and their associates.
BGI is the explained variable. Based on two streams of studies on green innovation and breakthrough innovation (Yan et al., 2024; Capponi et al., 2022), we use the technology categories and the number of patents for new entrants in green invention patents to construct the BGI (Balsmeier et al., 2017; Gao et al., 2021). The basic logic behind the selection of BGI is that IPC subclass codes are frequently used to identify firms’ capabilities to engage in or explore new technological areas (Gao et al., 2021). According to Balsmeier et al. (2017), the categories and numbers of new green patent entries represent critical breakthroughs in existing technological capabilities and can indicate a firm’s level of BGI. On the one hand, the more newly green patent categories an enterprise enters, the broader its exploration and expansion in new technology areas. This indicates that firms have broken through existing technological paradigms, resulting in higher novelty of technological innovations (Lin et al., 2021). On the other hand, a greater number of green patent applications under the category of new entrants by firms implies that firms are more abundant in green innovations within new technologies and products. This suggests that firms have invested more resources in innovation and have more diverse sources of external knowledge. Moreover, it is worth noting that we select green invention patents as the base data for constructing the BGI. Compared with green utility model patents, the application and examination processes of green invention patents are more complex and stringent (Jia et al., 2019), and the information asymmetry within the innovation process is higher. Therefore, the selection of green invention patents as the base data for the BGI can better reflect the complexity of the innovation processes of enterprises. In summary, the higher the novelty, the more diverse the knowledge sources, and the more complex the innovation process of an enterprise’s green technology, the higher level of BGI.
Specifically, based on the information of the IPC main group (Gao et al., 2021), we constructed BGI by following these steps. To begin with, we take the number of newly entered categories of green invention patents over the past 5 years as the primary indicator of BGI, named as IPC5. IPC5 is distinguished by the revolutionary ability of green knowledge or technical methodologies into emerging domains (Lin et al., 2021). Additionally, based on the calculation of the index of IPC5, we calculate the number of patents applications of green invention entering new fields in the past 5 years as the second indicator of BGI, regarded as Newpatent5. Newpatent5 is featured by the quantity of existing green invention patents to break through the boundaries of technology and knowledge.
Simultaneously, taking into account that the dimension of time may influence the accuracy of BGI, we also calculate the number of newly entered categories and corresponding applications of green invention patents over the past four and 3 years, which we consider additional indices for conducting robustness tests on the BGI. To more effectively evaluate the characteristics of BGI’s knowledge diversity, we utilize text analysis techniques and mine the abstract information of green invention patent to construct the cosine similarity (Arts et al., 2019). We consider this index as an additional metric for measuring the BGI. The lower the similarity between the summary information of enterprises’ green invention patents, the richer the knowledge base involved in green innovation, and thus the wider the boundary of expansion and the higher the level of enterprises’ BGI. Furthermore, we also examined the technical novel characteristics of BGI. The frequency of patent citations is frequently considered as an index to measure the influence of patents (Hall et al., 2001), and commonly used to measure breakthrough innovation (Verhoeven et al., 2016). The frequency with which a green patent is cited correlates with the breadth of its technological spillover, which, to a certain extent, reflects the enterprise’s departure from the original technological paradigm and the novelty of green technology. From this perspective, we employ a proxy indicator by calculating the top 20% of highly cited green invention patents to gauge BGI, which is further regarded as an indicator for a robustness assessment.
We take the IMPP released by the Ministry of Industry and Information Technology (MIIT) as a quasi-natural experiment to investigate the impact of IM on corporate BGI (Wei et al., 2024; Lyu et al., 2023). To advance the creation of a powerful industrial nation, the MIIT selected 305 enterprises to implement IMPP from 2015 to 2018. The specific situation of enterprises implementing the IMPP in terms of time and quantity is as follows: 46 in 2015, 63 in 2016, 97 in 2017, and 99 in 2018. IMPP provides valuable practical experience for the country to explore IM models and drive the intelligent advancement of the manufacturing sector. We regard the policy of annually launching and publishing the list of pilot enterprises as an obvious exogenous event. This method can overcome the endogenous problem in selecting the IM index to some extent, and provide an opportunity to accurately identify the green innovation impacts of IM.
When it comes to determining the list of IMPP enterprises, we consulted the research conducted by Wei et al. (2024) and extracted information on firms that have adopted IMPP by analysing relevant documents2. First, we referred to the list of IMPP released by the MIIT between 2015 and 2018. Second, we cross-referenced using tools like Qichacha and Cninfo to verify the pilot companies, thereby compiling a comprehensive list of IMPP companies. Lastly, we ensured the accuracy of the list by conducting meticulous manual searches and individual verifications. As a result, we identified a final roster of 105 companies that have successfully implemented IMPP. For enterprises that were repeatedly selected for IMPP, this paper defines the dummy variable for IM based on the year they were first selected. In addition, we also employ text analysis methods to identify IM-related keywords in the annual reports of companies and regard them as proxy variables for IM to conduct robustness checks.
Apart from the variables previously mentioned, we also consider the following enterprise characteristics that might influence BGI: company size (Size), company age (Age), net profit rate of total assets (Roa), book-to-market value ratio (Btm), cash flow status (Cfp), total compensation of the top three managers (Tec3), dual roles of CEO and chairman (Dual), and proportion of fixed assets (Pfa). Table 1 represents descriptions of these variables.
The DID model is well suited for identifying causation and is frequently used in policy evaluations due to its numerous advantages. We constructed the following model to examine the impact of IM on BGI:
Table 2 provides descriptive statistics. The results indicate that in the manufacturing enterprise samples from 2010 to 2022, the means of IPC5 and Newpatent5 are 0.946 and 1.646, respectively, with standard deviations of 3.501 and 8.707, respectively, indicating that BGI among Chinese enterprises is still in its nascent stages and that noticeable disparities exist. The mean of IM is 0.034, with a standard deviation of 0.181, suggesting that the implementation of IMPP remains limited, with a large number of enterprises yet to transition to intelligent production, resulting in substantial disparities in the degree of implementation among manufacturing companies. The averages for Size, Age, Roa, Btm, Cfp, Tec3, Dual, and Pfa are 22.21, 2.05, −3.24, −1.19, −2.82, 14.62, 0.29, and−1.63, respectively. These statistics align with previous findings in the literature.
The distribution of BGI yields valuable insights into the emerging landscape of BGI in China’s manufacturing industry. Based on the Guidelines for Industry Classification of Listed Companies (Revised in 2012), we investigate the temporal trends and industry-specific distributions of BGI levels within manufacturing samples. Panels A and B illustrate the temporal evolution of cumulative BGI, as shown in Supplementary Figure SA1.
Panel A of Supplementary Figure SA1 shows there has been a gradual increase in the number of IPC5s within manufacturing enterprises in recent years. However, it is worth noting that the upward trend changed in 2020 and has since shown a decline. The emergence of the COVID-19 epidemic may have influenced the green innovation activities of manufacturing companies, resulting in a reduction in IPC5. Further confirmation of these findings is observed through another indicator, Newpatent5 (Panel B), which reinforces the consistency of the results.
The distribution of BGI sum in the manufacturing industry is presented in Panels C and D of Supplementary Figure SA2. Panel C reveals that within the manufacturing industry, the top five industries with the highest IPC5 total rankings are electrical machinery and equipment, computers, automobiles, special equipment, and chemical raw materials and chemicals. This finding suggests that within these industries, green innovation activities are more active and the BGI level is higher. Similar outcomes are replicated in another BGI indicator (Newpatent5), as presented in Panel D.
According to Hausman and Taylor (1981) and Agresti (2012), the explanatory variable, which is the number of firms’ green patents, is a typical discrete non-negative integer count data. Poisson or negative binomial models are commonly employed in empirical studies to estimate it. However, the use of Poisson regression necessitates the satisfaction of the assumption that variances and means are approximately equal. The descriptive statistics presented in this manuscript indicate that the variance (squared standard deviation) of both IPC5 and Newpatent5 exceeds their means, suggesting that the number of green patents in our sample exhibits over-dispersion. In this context, if we use Poisson regression to analyze the effect of these events, it will underestimate the standard errors of the model parameter estimates. To eliminate individual differences within the sample data and achieve more robust regression results, we select the negative binomial regression model with fixed effects to investigate the impact of IM on BGI.
Table 3 lists the results of Equation 1, which reflect the impact of IM on BGI. As IM is a binary variable equal to 1, its coefficient indicates the impact of IM on the BGI compared to those without IMPP. Columns (1) and (2) denote results without controlling control variables. Columns (3) and (4) display results including various fixed effects and control variables. Specifically, in Column (3), the regression coefficient of IM is 0.278, which is significantly positive at the 5% level. This implies that, compared with companies that did not implement IMPP, the level of IPC5 of companies that implemented IMPP improved significantly. In Column (4), the regression coefficient of IM is 0.264, which is significantly positive at the 10% level. This implies that, compared with companies that did not implement IMPP, the level of Newpatent5 of companies that implemented IMPP improved significantly. These findings suggest that IM positively enhances BGI. This verifies hypothesis 1.
Benchmark regression results demonstrate that IM can promote the BGI of manufacturing enterprises. Nevertheless, factors such as sample selection, missing variables, and other potential sources of endogeneity might have influenced our findings. To address this, we conducted a series of robustness tests.
The basic results show that after the implementation of IMPP, the BGI of enterprises has been impacted. However, an important premise for the conclusion is the parallel trend test, which requires that in the absence of exogenous impacts, the changes in BGI between the treatment group and the control group are similar. Based on this, we employ a dynamic difference-in-differences model3 to explore the dynamic influence of IM on BGI.
Based on Equation 2, we re-estimate the model and plot the dynamic graphs of the parallel trend. Supplementary Figures SA3, A4 represent the results of parallel trend test. These findings indicate that prior to the implementation of IMPP, there is no systematic difference in BGI among enterprises. After the implementation of IMPP, the treatment group exhibits a considerable rise in BGI compared to the control group, supporting the parallel trend test.
Although the aforementioned research found that IM positively impacts BGI, the results may be affected by other factors. To ensure that the impact of IM on BGI is not affected by other random variables, we use a placebo test to examine the random nature of the IM effect. Based on the distribution of the IM variable in the benchmark regression, we performed 500 random samples and created a “pseudo-policy dummy variable,” and then regressed it again using Equation 1 to test the coefficient value and P - value distribution. From the test results depicted in Supplementary Figures SA5, A6, we observe that the influence of IM on BGI is not due to other random factors. These findings bolster the credibility of our estimation results.
Given the potential sample selection issues during the implementation of IMPP, we utilize the propensity score matching method (PSM-DID) for verification. We regard the control variables as covariates. Furthermore, we employ two matching methods, namely, kernel matching and nearest neighbor matching, to ensure robustness in our analysis. To more evaluate the net effect of IM on corporate BGI, we re-estimate the regression using Equation 1, excluding unmatched samples. Table 4 indicates that IM can enhance BGI.
According to Goodman-Bacon (2021), the two-way fixed-effects estimator in the estimation of the TDID model is equivalent to the weighted average of all possible two-period DID estimators in the sample. This approach may make the heterogeneity of treatment effects unrobust, for instance, due to issues such as negative weights. As each firm was selected for IMPP at a distinct point in time, the following three controls will be included in our study: ①New entrants to the IMPP (Treatment) and those who have never been selected for the IMPP (Never Treated); ②New entrants to the IMPP (Earlier Group Treatment) and those who have not yet joined the IMPP (Later Group Comparison); ③New entrants to the IMPP (Later Group Treatment) and those who are already participating in the IMPP (Earlier Group Comparison). Among these three controls, the first two serve as ‘good controls’ in that they assess the impact of whether or not to enter the IMPP on firms’ BGI. However, the treatment effect in the third control is not necessarily homogeneous. If the weights assigned to the control mean estimator of the third are greater, this can influence the results of the two-way fixed effects estimator, thereby biasing the results (Goodman-Bacon, 2021; Sun and Abraham, 2021).
Based on this observation, we employed the Goodman-Bacon decomposition to examine whether the third control was over-represented. Supplementary Figures SA7, A8 present the TDID Goodman-Bacon decomposition results for IPC5 and Newpatent5, respectively. These results demonstrate that the percentage of the third control is smaller in this study, implying that the effect of IM on BGI is not attributable to the third control. Additionally, we also report decomposition results specifically for IPC5 and Newpatent5, and these results are presented in Table 5. Based on the Goodman-Bacon decomposition results of IPC5, it is evident that the primary contributor to the overall DID estimation results in this study is the estimation results from the sample that was never exposed to the IMPP, serving as the control group, with a weight as high as 98.9% (Treatment vs. Never Treated). In contrast, the sample with earlier treated individuals as the control group carries a weight of only 0.3% (Later Group Treatment vs. Earlier Group Comparison), which has a minimal impact on the overall estimation results and does not lead to an overestimation of the effect of IM on BGI. Newpatent5 follows a similar judgment process. The above analyses demonstrate that the results of the TDID estimation we employed are reliable.
The relationship between IM and BGI may be affected by endogeneity issues. To address this, we employed the instrumental variable approach to eliminate potential endogeneity. Specifically, we selected the interaction term between the number of landline telephones in each city in 1984 and the time variable (IV1), as well as the interaction term between the number of post offices in each city in 1984 and the time variable (IV2), as instrumental variables for IM (Nunn and Qian, 2014). The selection of these variables is justified on two grounds. First, regional landline telephones and post offices can influence the spread and development of information by shaping the technological and usage habits of Internet use, thereby satisfying the relevance requirement of the instrumental variable. Second, the number of landline telephones and post offices in 1984 does not directly contribute to firms’ current BGI, thereby satisfying the exogeneity assumption of the instrumental variable.
Table 6 presents the estimation results of the Two-Stage Least Squares (2SLS) method, with Columns (1)-(3) indicating the results for IV1 and Columns (4)-(6) indicating the results for IV2. The results of the LM test show that IV1 and IV2 exhibit a significant correlation with IM; the F-values for both IV1 and IV2 are significantly greater than 10, thereby rejecting the original hypothesis of weak instruments. Our central conclusions remain robust.
To investigate the correlation between IM and BGI, it is critical to exclude the influence of other policies. Given that other pilot cities and regional policies may interfere with the identification of IMPP effects, we construct dummy variables to exclude the influence of other related policies within the same period. These policies encompass the Made in China 2025 IM Pilot Project (Li and Branstetter, 2024), the National Big Data Comprehensive Pilot Zone (Wang X. et al., 2024), the Broadband China Policy (Wang and Wang, 2024), and the Smart City Policy (Qi et al., 2024). We label them as Policy1, Policy2, Policy3, and Policy4, respectively. Subsequently, we systematically incorporate the aforementioned four policies as control variables in Equation 1, and the Columns (1)-(8) of Table 7 report the estimation results. The results indicate that the estimated coefficients of IM are significantly positive, following the incorporation of the aforementioned policies as control variables. Moreover, we consider the joint influence of Policy1 - Policy4, and Columns (9) and (10) present the regression results. The results show that the regression coefficients of IM on IPC5 and Newpatent5 remain positive and statistically significant. These results align with our findings.
(1) Replacing the explained variables. First, referring to Lin et al. (2023), we gauge the novelty of innovation outcomes by calculating the cosine similarity of green invention patent abstracts4, name it BGI1, and regard it as the first indicator of BGI, Second, referring to Aghion et al. (2019) and Chang et al. (2019), we exploit the top 20% of green invention patent citations as the second indicator for measuring BGI, and name it BGI2. Last, following Makri and Scandura (2010), we apply the dispersion of green invention patent technology5 as the third indicator to measure BGI, and name it BGI3. We also calculate the number of newly entered categories of green invention patents and their applications by the company over the past four and 3 years. We employ them as alternative indicators for BGI in the robustness test and name them IPC4, Newpatent4, IPC3, and Newpatent3, respectively. Table 8 represents the estimation results.
(2) Replacing the explanatory variable. Drawing upon Verhoef et al. (2021), we exploit intelligent transformation (IM_R) based on text analysis methods to replace IM6. Among them, IM_R1 denotes the total number of words related to IM_R, and IM_R2 represents the ratio of the total number of words related to IM_R to the total number of words in the annual report. The results of the alternative explanatory variables are displayed in Table 9. These findings demonstrate that whether substituting the explained variables or explanatory variables, IM can significantly enhance BGI.
To ensure the reliability of the regression conclusions, referring to Jin and Chen (2024), we also selected the fixed effects model for the robustness test. Table 10 displays the regression results after replacing the econometric model. The coefficients of the IM remain significantly positive, indicating that the conclusions of our study are robust.
Theoretical analyses suggest that IM can promote BGI through three channels: crowding in R&D resources, strengthening GOPI, and mitigating agency conflict. Based on this, we selected the mediation effect model to test these mediating variables, and the model is set as follows:
In addition, considering the endogeneity of the three-stage mediation effect model, we further selected the moderating effect model to test the robustness of these mediating variables, and the model is set as follows:
Where
IM will crowd in the R&D resources, then fully stimulate the BGI vitality of enterprises. Based on this, we employ the ratio of a company’s R&D expenditure to its total assets to quantify R&D resources. A higher proportion of investment in R&D will lead to a larger pool of green innovative resources, ultimately facilitating BGI. Table 11 presents the results of the mechanism test for R&D resources. Columns (1)-(3) display the regression results for the mediation effect model. Column (1) demonstrates that the coefficient of IM is significantly positive, indicating that IM contributes to the enhancement of R&D resource. In Columns (2) and (3), the coefficients of IM exhibit a notably positive correlation, and the coefficients of R&D also show a significantly positive relationship. These findings imply that IM has the ability to enhance BGI by facilitating a growth in R&D resources.
To ensure the reliability of the regression results for R&D resources, we constructed an interaction term of IM and R&D (IM
IM can foster the establishment of GOPI network by connecting enterprises with other enterprises, universities, and research institutes. Generally speaking, the greater the extent to which enterprises engage in green collaboration, the more abundant the resources of knowledge and technology required for green innovation become. Within the framework of the GOPI network, the communication cost among enterprises will be effectively reduced, thereby release the full potential of BGI (Laursen and Salter, 2006). Hence, we aim to study whether IM impacts BGI via GOPI. Drawn on Hong and Su (2013), we calculate the number of innovation collaborative partners involved in green invention patents to measure GOPI7. The greater the GOPI, the more universities and research institutes engage in green collaborative innovation with the enterprise, indicating a more robust and comprehensive GOPI network. Table 12 presents the results of the mechanism test for GOPI. Columns (1)-(3) display the regression results for the mediation effect model. Specifically, Column (1) reveals that the coefficient of IM is saliently positive, suggesting that IM facilitates the expansion of the green innovation partner network and significantly reinforces GOPI. Furthermore, in Columns (2) and (3), the coefficients of GOPI are significantly positive. These findings imply that IM has the capability to promote BGI by enhancing GOPI.
To ensure the reliability of the regression results for GOPI, we constructed an interaction term of IM and GOPI (IM
IM has the ability to enhance resource allocation, internal management efficiency, and alleviate information asymmetry. These advantages can assist enterprises minimize agency conflict, resulting in enhance BGI. Therefore. we investigate the possibility of agency conflict acting as a mediator between IM and BGI. Following Ang et al. (2000), we select the management expense ratio to gauge agency conflict. It is determined by dividing management expenses by operating income. A higher ratio indicates larger agency conflict. Table 13 presents the results of the mechanism test for agency conflict. Columns (1)-(3) display the regression results for the mediation effect model. Specifically, Column (1) indicates that the coefficient of IM is notably negative, suggesting that IM contributes to reducing the management cost of enterprises. In Columns (2) and (3), the coefficients of IM are notably positive, whereas the coefficients of agency conflict are significantly negative. These findings indicate that IM can enhance BGI by alleviating agency conflict.
To ensure the reliability of the regression results for agency conflict, we constructed an interaction term of IM and agency conflict (IM
Manufacturing, especially high-pollution and high-energy consumption industries, exhibit a strong path dependence concerning technological innovation. The existing inventory of polluting technologies within enterprises may impede the development of clean technologies (Aghion et al., 2016). In particular, China, as the representative of emerging manufacturing countries, relies heavily on high-pollution and high-energy consumption industries, which act as both the cornerstone of national economic growth and a major source of pollution discharge. Therefore, it is of great significance for emerging manufacturing countries to expedite the achievement of green development by finding ways to overcome the path dependence of these industries. In this context, we examine the influence of IM on BGI within high-pollution and high-energy consumption industries.
In this part, based on the industry’s pollution intensity and energy consumption levels, we divide the total sample into four parts: high-pollution (HP) industries and non-high-pollution industries (NHP)8, high-energy consumption (HEC) industries and non-high-energy consumption industries (NHEC)9. Table 14 displays that the coefficients of IM are significant positive in Columns (1), (2), (5), and (6) for the HP and HEC sub-samples, whereas the coefficients of IM in the NHP and NHEC sub-samples are not significant. Therefore, we infer that IM can disrupt the path dependence of technological innovation in HP and HEC industries, thereby promote BGI of these industries.
There are several plausible explanations for these results. First, IM highlights the integration of artificial intelligence technology into the production process, which helps break the inertia of green innovation. What’s more, the characteristics of IM also can facilitate precise control and management throughout the green innovation process, thereby providing a new production paradigm for HP and HEC industries. Second, the production networks of enterprise based on artificial intelligence technology are beneficial for breaking the ‘locking effect’ of traditional production networks on traditional technology paths. Third, the transformation and upgrading of machinery equipment facilitated by IM could alleviate the “sunk cost” barrier to adopting green technology to some extent. Therefore, IM can disrupt the path dependence of enterprise technology selection by altering the production paradigm of the HP and HEC industries, and drive these industries to promote BGI.
The correlation between environmental regulation and green innovation has been a topic of academia. For a considerable time, two opposing views have existed regarding the effect of environmental regulation on green innovation. Based on neoclassical ‘cost-following’ theory, some research argues that environmental regulation raises pollution control costs and decreases R&D inputs (Conrad and Wastl, 1995). From the perspective of Porter’s theory, some scholars assert that environmental regulation significantly raises the opportunity cost of green innovation, compelling enterprises to proactively pursue green innovation initiatives (Du et al., 2021; Porter and Linde, 1995). What is the impact of environmental regulation on the relationship between IM and BGI? Currently, there is little academic discussion on this issue.
Based on this, we explore the influence of IM on BGI under different levels of environmental regulations. First, from the viewpoint of environmental governance, we calculate the proportion of pollution control investment for each province to measure the intensity of environmental regulation at the regional level (Liu et al., 2019). Based on the median, this paper divides the samples into high environmental regulation (HER) and low environmental regulation (LER). Second, we regard the evaluation standard of the environmental protection model city as another measure of environmental regulation. The underlying rationale is that the areas selected as model cities for environmental protection are subject to more rigorous environmental evaluations. Within this constraint, these cities intensify their commitment to environmental regulation. From this perspective, using information from the official website of the Ministry of Ecology and Environment of the People’s Republic of China10, we categorise the samples into two groups based on strong environmental protection (SEP) and weak environmental protection (WEP). A value of 1 is assigned when the company is situated in a model city for environmental protection and 0 otherwise. Table 15 displays the results. For the sub-sample of HER and SEP in Columns (1), (2), (5), and (6), the coefficients of IM exhibit a significant positive relationship. However, for the sub-sample of LER and WEP in Columns (3), (4), (7), and (8), the coefficients of IM do not exhibit a significant relationship. This empirical evidence suggests that as regional environmental regulations become stricter, the promoting effect of IM on BGI becomes more pronounced.
The above conclusions may be attributed to the following two factors. First, IM can decrease enterprise costs and form a joint force with environmental regulations to enhance BGI. On the one hand, environmental regulations could potentially increase the opportunity costs associated with pollution, thereby creating an incentive for green innovation within the enterprise. However, environmental regulations may also raise the costs of corporate governance and reduce the resources available for green innovation within enterprises. In the above two situations, the dominant cost factor under the aforementioned situations is also the subject of debate between the cost-following theory and Porter theory. Nevertheless, IM enhances efficiency of green innovation and contributes to lowering the cost of environmental governance to some extent. In areas with strict environmental regulations, as enterprises face higher opportunity costs, the impact of IM on boosting BGI becomes more pronounced. Second, environmental regulations can drive the formation of GOPI, which is beneficial for enhancing the mechanism of IM in promoting BGI. As a crucial method for environmental improvement, BGI requires advanced, extensive, and fundamental technical support. Universities and research institutes often have innovative and advanced green technologies, in which case, the growing stringency of regional environmental regulations compels enterprises to bolster their collaboration with universities and research institutes, thereby enhancing the GOPI network formation. In some respects, the above analysis also indicates that the stricter the regional environmental regulations, the more perfect the GOPI network, and the more effectively the IM promotes BGI.
As a novel production model, IM has immense potential to promote BGI in the manufacturing sector. However, will BGI bring a “win-win” outcome for both economic and environmental protection? Current research has yet to provide a concise answer. Based on this, we further investigate the economic and ESG performance of BGI as promoted by IM. We conducted an examination based on the following model:
Table 16 presents the influence of BGI on financial performance. The coefficients show that IPC5 and Newpatent5 have notably positive effects on TQ, signifying that IM have the potential to enhance economic performance. The impact of BGI on the company’s ESG performance are shown in Table 17. These findings suggest that BGI enhances corporate ESG.
The empirical results suggest that IM can enhance the dual performance of a company’ economy and ESG by promoting BGI. One possible explanation is that BGI has the potential to send green signals. These green signals help companies to attract market investors and financial institutions, secure financial support, and drive advancements in environmental (E), social (S), and governance dimensions (G). We also perform furth tests on the three corporate ESG sub-indicators: E, S, and G. Notably, in comparison to G, BGI exhibits a notable capacity to enhance corporate performance in the E and S dimensions (as represented in Columns (3) to (8) of Table 17). In this study, our findings reveal that IM can foster a “win-win” outcome for both economic and environmental performance by advancing BGI.
The BGI emphasises the novelty of knowledge technology and focuses on breaking the path of existing technologies so that manufacturing countries can achieve green development. As a key element of the new round of industrial revolution, IM provides a foundation for enterprises to engage in BGI activities. The primary conclusions can be summarized as follows.
First, IM plays a vital role in fostering BGI in the manufacturing industry. The studies conducted by Jin and Chen (2024), Han et al. (2024), and Yang and Shen (2023) provide valuable insights by demonstrating that IM can promote green innovation and facilitate the green transformation of the industry. Our findings extended these studies by applying the breakthrough innovation indicator construction methodology to the domain of green innovation for the first time, thereby constructing BGI. Our analysis ultimately revealed that IM can significantly enhance BGI. This not only enriches the discussion on the economic implications of IM but also offers novel insights and directions for future research on green innovation.
Second, having confirmed the facilitating effect of IM on BGI, we further examined the various mechanisms through which IM influences BGI. In contrast to existing studies, such as those by Jin and Chen (2024) and Han and Mao (2023), which explored the mechanisms of IM on green innovation with respect to financing constraints, green employment, environmental subsidies, and information sharing, our study, drawing upon the resource-based theory, open innovation theory, and information asymmetry, identifies R&D investment, GOPI, and agency conflict as pivotal mechanisms through which IM fosters BGI. Through these mechanisms, IM is able to address and alleviate the financial constraints, knowledge limitations, and higher information asymmetry that firms encounter in facilitating BGI. Our findings enrich the study of the mechanisms through which IM improves green development.
Third, IM has a greater promoting effect on BGI in HP and HEC industries, effectively breaking the path of technology selection within these industries. David (1985) contends that path dependence in technological innovation signifies that prior technological innovations influence subsequent technological trajectories. Specifically, in heavy chemical industries, the existing inventory of pollutive technologies within firms can potentially impede the choice of cleaner technologies (Aghion et al., 2016), thereby hindering green innovation. However, few scholars have explored on the phenomenon of path dependency in green innovation within the context of intelligence. Our research reveals that IM has a significant promoting effect on BGI in the HP and HEC industries, effectively breaking the path of technology selection in these sectors. Additionally, we also demonstrated that IM plays a pronounced role in regions with stricter environmental regulations, suggesting that it can synergize with environmental regulations to advance BGI. These findings align with the studies conducted by Zhao and Qian (2024) and Song et al. (2024). This conclusion enriches Porter’s theory in the digital economy era.
Fourth, our consequential test demonstrates that BGI driven by IM, can significantly contribute to a dual enhancement in both the economic and ESG performance of enterprises. Specifically, IM can facilitate ‘win-win’ outcomes for corporate economic and environmental performance through BGI. Numerous scholars have emphasized the positive role of green innovation in economic development (Kunapatarawong and Martínez-Ros, 2016) and environmental governance (Liu et al., 2024; Shen and Zhang, 2023). However, limited attention has been devoted to the consequences of BGI. Our analysis enriches the existing studies on the impact of BGI on economic and environmental performance.
Our conclusions have valuable policy implications for the advancement of IM and the promotion of BGI in emerging economies.
First, governments need to promote intelligent transformation to enhance BGI through IM. Our research indicates that IM can promote BGI in manufacturing firms. On the one hand, it is essential for the government to strengthen the top-level design of industrial intelligence through a multi-level and systematic policy framework to create an effective policy environment for enterprises to boost IM. On the other hand, the government can provide the necessary financial support, including subsidies, direct financial assistance, and tax incentives, to alleviate the financial pressure on enterprises and to offer favorable institutional support for the implementation of IM and the promotion of R&D and transformation within BGI. In particular, heavily polluting enterprises should capitalize on the development of IM, actively exploring transformation paths that align with their own conditions. This approach aims to break the path dependence of traditional technologies and thereby enhance BGI.
Second, the government should effectively streamline the channels for implementing IM to promote BGI. Mechanism analysis indicates that IM can enhance corporate BGI through crowding-in R&D investment and strengthening GOPI. On the one hand, the government should increase R&D investment and encourage enterprises to boost their R&D spending and actively engage in green innovation activities through funds allocated to green projects, policy-based loans, and other preferential measures. This will facilitate providing effective support for enterprises to achieve breakthroughs in cutting-edge green technologies. On the other hand, the government should also focus on fostering close collaboration among industry, academia, and research in the age of intelligence, actively encouraging universities, research institutions, and enterprises to establish a broader array of green partnerships. This initiative aims to clear pathways for IM to advance BGI. For example, universities and research institutions are encouraged to establish research institutes dedicated to intelligent technologies, collaborative laboratories involving industry, academia, and research, and intelligent green innovation platforms. This initiative aims to foster a strong ecosystem of green collaboration, thereby creating a conducive environment for advancing BGI within enterprises.
Third, governments should focus on strengthening environmental regulations, thereby fostering synergy with IM to promote BGI. Empirical results demonstrate that IM significantly enhances BGI in regions with stricter environmental regulations. This suggests that under stronger environmental regulations, intelligent firms are capable of reducing the costs associated with corporate environmental governance, compelling firms to input more R&D resources, thereby stimulating the green innovation effect of IM. Consequently, the government should enact stricter environmental regulations, augment the severity of treatment and penalties for environmental violations, and effectively enhance the enforceability of regional environmental regulations. For example, the government can appropriately increase the amount of regional investment in pollution control and formulate local environmental regulatory policies to internalize the external costs of polluting enterprises, thereby stimulating their green innovation effect. The stricter regulations will synergize with IM to jointly promote the advancement of BGI.
This paper has some limitations and requires further exploration. First, regarding index measurement, drawing on the frontier of innovation economics (Balsmeier et al., 2017; Gao et al., 2021), we use IPC information from green invention patents to measure BGI. Although we have constructed several indicators to test the robustness of BGI from different angles, such as cosine similarity, citation times, and the technical dispersion of green invention patents, the method of index measurement has not reached a broad consensus in academic circles. The theory of breakthrough innovation is still at the exploratory stage in innovation economics. With its development, we also need to learn the latest methods to construct BGI in the future, to more accurately reflect corporate BGI. Second, this paper examines the influence of IM on BGI. However, the research sample primarily focus on the intelligent development of China’s manufacturing sector. It has not considered other emerging manufacturing powers. In future research, we need to collect more detailed data on IM development in different manufacturing countries for comparative analysis to provide more comprehensive empirical evidence for the green innovation effect of IM.
The original contributions presented in the study are included in the article/Supplementary Material, further inquiries can be directed to the corresponding author.
XD: Formal Analysis, Supervision, Writing–review and editing. YD: Conceptualization, Data curation, Formal Analysis, Writing–original draft. MZ: Writing–review and editing, Methodology, Project administration, Software. XX: Writing–review and editing, Funding acquisition, Investigation, Supervision.
The author(s) declare that financial support was received for the research, authorship, and/or publication of this article. This study was funded by the R&D Program of Beijing Municipal Education Commission: Research on Beijing-Tianjin-Hebei Cooperative Development Strategy and Total Factor Productivity in Manufacturing Industry(No:SM202110038014).
The authors declare that the research was conducted in the absence of any commercial or financial relationships that could be construed as a potential conflict of interest.
The author(s) declare that no Generative AI was used in the creation of this manuscript.
All claims expressed in this article are solely those of the authors and do not necessarily represent those of their affiliated organizations, or those of the publisher, the editors and the reviewers. Any product that may be evaluated in this article, or claim that may be made by its manufacturer, is not guaranteed or endorsed by the publisher.
The Supplementary Material for this article can be found online at: https://www.frontiersin.org/articles/10.3389/fenvs.2025.1511469/full#supplementary-material
1Definition of IM as per the IM Development Plan (2016–2020).
2The specific documents also include: Implementation Plan of IMPP in 2015. Notice on the Recommendation of IMPP in 2015. Notice on the Implementation of IMPP in 2016 by the MIIT. Notice on the Recommendation of IMPP in 2016 by the Office of the MIIT. Notice on the Recommendation of IMPP in 2017 by the Office of the MIIT and Notice on the Recommendation of IMPP in 2018 by the Office of the MIIT, etc.
3Among them,
4The specific construction steps for the cosine similarity index are as follows: Firstly, preprocess the green patent abstract content. This includes removing stop words, punctuation marks, and special characters that have no substantive meaning for text analysis and processing to reduce the interference of noise on index calculation. Next, use “Jieba” segmentation to process the text. Divide the text content into several words or terms, count the frequency of the words in the text content, and construct the corresponding word frequency vector. Then, assign corresponding weights to each word applying the ‘Term Frequency-Inverse Document Frequency (TF-IDF)’ method, accurately characterising the importance of words in the text content by combining term frequency (TF) and inverse document frequency (IDF). Finally, calculate the cosine similarity of the green invention patent abstract content for each company in year t and year t-1. The calculation formula of cosine similarity is as follows:
5The calculation formula is:
6First, select the keywords for IM_R. Based on the information from official documents, reports, and international frontier literature related to intelligence manufacturing, we can derive and organize the keywords related to IM_R. Next, we use synonym expansion to enrich the keywords for IM_R, and finally get 85 related words. Finally, the total count of words related to the IM_R is used as the proxy variable for IM.
7The green innovation collaborative partners include universities, research institutes, and joint laboratories (such as research centers, R&D centers, and technology centers).
8Based on the 2012 revision of the ‘Industry Classification Guidelines for Listed Companies’ by the China Securities Regulatory Commission (CSRC), industry codes C17, C19, C22, C25, C26, C28, C29, C30, C31, and C32 are identified as heavily polluting (HP) sectors. Other industries fall under the non-heavily polluting (NHP) category.
9Refer to Tanaka et al. (2014), we calculated the ratio of SO2 emissions in the national emissions in 2010, and considered it as the indictor of the energy consumption of industries. According to the median of this value, our study sample were divided into two groups: HEC and NHEC industries.
10The Notice on Launching the Activities of Creating a National Model City for Environmental Protection announced that the activities of creating a national model city for environmental protection would be launched in cities all over the country. In the evaluation process of environmental protection model cities, the relevant institutions need to assess various environmental quality and environmental construction indicators. For example, the annual average concentration of major pollutants in urban air is required to reach the national second-class standard, and the number of days when their daily average concentration reaches the secondary standard needs to account for more than 85% of the total number of days in the whole year. In particular, there are also stricter requirements for cleaner production of key industrial enterprises in these cities (Information Source: Assessment Indicators and Implementation Rules for National Environmental Protection Model Cities (Sixth Stage).
Acemoglu, D., and Restrepo, P. (2019). Automation and new tasks: how technology displaces and reinstates labor. J. Econ. Perspect. 33 (2), 3–30. doi:10.1257/jep.33.2.3
Acemoglu, D., and Restrepo, P. (2020). Robots and jobs: evidence from US labor markets. J. Political Econ. 128 (6), 2188–2244. doi:10.1086/705716
Aghion, P., Akcigit, U., Bergeaud, A., Blundell, R., and Hémous, D. (2019). Innovation and top income inequality. Rev. Econ. Stud. 86 (1), 1–45. doi:10.1093/restud/rdy027
Aghion, P., Dechezleprêtre, A., Hemous, D., Martin, R., and Van Reenen, J. (2016). Carbon taxes, path dependency, and directed technical change: evidence from the auto industry. J. Political Econ. 124 (1), 1–51. doi:10.1086/684581
Akter, S., Bandara, R., Hani, U., Wamba, S. F., Foropon, C., and Papadopoulos, T. (2019). Analytics-based decision-making for service systems: a qualitative study and agenda for future research. Int. J. Inf. Manag. 48, 85–95. doi:10.1016/j.ijinfomgt.2019.01.020
Ang, J. S., Cole, R. A., and Lin, J. W. (2000). Agency costs and ownership structure. J. Finance 55 (1), 81–106. doi:10.1111/0022-1082.00201
Arts, S., Hou, J., Carlos Gomez, J., Catalano, G., Daraio, C., Gregori, M., et al. (2019). Text mining to measure novelty and diffusion of technological innovation.
Baba, Y., and Walsh, J. P. (2010). Embeddedness, social epistemology and breakthrough innovation: the case of the development of statins. Res. Policy 39 (4), 511–522. doi:10.1016/j.respol.2010.01.016
Balsmeier, B., Fleming, L., and Manso, G. (2017). Independent boards and innovation. J. Financial Econ. 123 (3), 536–557. doi:10.1016/j.jfineco.2016.12.005
Barney, J. (1991). Firm resources and sustained competitive advantage. J. Manag. 17 (1), 99–120. doi:10.1177/014920639101700108
Becker, G. S. (2009). Human capital: a theoretical and empirical analysis, with special reference to education. University of Chicago press.
Berkes, E., and Gaetani, R. (2021). The geography of unconventional innovation. Econ. J. 131 (636), 1466–1514. doi:10.1093/ej/ueaa111
Bessen, J. (2019). Automation and jobs: when technology boosts employment. Econ. Policy 34 (100), 589–626. doi:10.1093/epolic/eiaa001
Bharadwaj, A. S. (2000). A resource-based perspective on information technology capability and firm performance: an empirical investigation. MIS Q. 24, 169–196. doi:10.2307/3250983
Byun, S. K., Oh, J. M., and Xia, H. (2021). Incremental vs. breakthrough innovation: the role of technology spillovers. Manag. Sci. 67 (3), 1779–1802. doi:10.1287/mnsc.2019.3507
Cappa, F., Oriani, R., Peruffo, E., and McCarthy, I. (2021). Big data for creating and capturing value in the digitalized environment: unpacking the effects of volume, variety, and veracity on firm performance. J. Prod. Innovation Manag. 38 (1), 49–67. doi:10.1111/jpim.12545
Capponi, G., Martinelli, A., and Nuvolari, A. (2022). Breakthrough innovations and where to find them. Res. Policy 51 (1), 104376. doi:10.1016/j.respol.2021.104376
Chang, X., Chen, Y., Wang, S. Q., Zhang, K., and Zhang, W. (2019). Credit default swaps and corporate innovation. J. Financial Econ. 134 (2), 474–500. doi:10.1016/j.jfineco.2017.12.012
Chatterjee, S., Chaudhuri, R., Shah, M., and Maheshwari, P. (2022). Big data driven innovation for sustaining SME supply chain operation in post COVID-19 scenario: moderating role of SME technology leadership. Comput. and Industrial Eng. 168, 108058. doi:10.1016/j.cie.2022.108058
Chesbrough, H., and Bogers, M. (2014). “Explicating open innovation: clarifying an emerging paradigm for understanding innovation,” in New frontiers in open innovation. Forthcoming: Oxford University Press, 3–28.
Chesbrough, H. W. (2003). Open innovation: the new imperative for creating and profiting from technology. Brighton, MA, USA: Harvard Business Press.
Cho, S. Y., and Kim, S. K. (2017). Horizon problem and firm innovation: the influence of CEO career horizon, exploitation and exploration on breakthrough innovations. Res. Policy 46 (10), 1801–1809. doi:10.1016/j.respol.2017.08.007
Conrad, K., and Wastl, D. (1995). The impact of environmental regulation on productivity in German industries. Empir. Econ. 20, 615–633. doi:10.1007/bf01206060
Datta, A. A., and Srivastava, S. (2023). (Re)conceptualizing technological breakthrough innovation: a systematic review of the literature and proposed framework. Technol. Forecast. Soc. Change 194, 122740. doi:10.1016/j.techfore.2023.122740
David, P. A. (1985). Clio and the economics of QWERTY. Am. Econ. Rev. 75 (2), 332–337. Available at: https://www.jstor.org/stable/1805621
Davis, C., and Tomoda, Y. (2018). Competing incremental and breakthrough innovation in a model of product evolution. J. Econ. 123, 225–247. doi:10.1007/s00712-017-0568-y
Diebolt, C., and Hippe, R. (2019). The long-run impact of human capital on innovation and economic development in the regions of Europe. Appl. Econ. 51 (5), 542–563. doi:10.1080/00036846.2018.1495820
Du, G., Yu, M., Sun, C., and Han, Z. (2021). Green innovation effect of emission trading policy on pilot areas and neighboring areas: an analysis based on the spatial econometric model. Energy Policy 156, 112431. doi:10.1016/j.enpol.2021.112431
Esposito, C. R. (2023). The geography of breakthrough invention in the United States over the 20th century. Res. Policy 52 (7), 104810. doi:10.1016/j.respol.2023.104810
Fabra, I. T., Ghobadian, A., Troise, C., and Bresciani, S. (2023). Antecedents of successful diffusion of breakthrough innovations past the formative phase: perceptions of innovation-engaged practitioners. Technovation 127, 102851. doi:10.1016/j.technovation.2023.102851
Feller, J., Finnegan, P., Hayes, J., and O’Reilly, P. (2009). Institutionalising information asymmetry: governance structures for open innovation. Inf. Technol. and People 22 (4), 297–316. doi:10.1108/09593840911002423
Fernández-Mesa, A., Luis Ferreras-Mendez, J., Alegre, J., and Chiva, R. (2014). IT competency and the commercial success of innovation. Industrial Manag. and Data Syst. 114 (4), 550–567. doi:10.1108/imds-09-2013-0389
Furman, J., and Seamans, R. (2019). AI and the economy. Innovation Policy Econ. 19 (1), 161–191. doi:10.1086/699936
Gao, Q., Cheng, C., and Sun, G. (2023). Big data application, factor allocation, and green innovation in Chinese manufacturing enterprises. Technol. Forecast. Soc. Change 192, 122567. doi:10.1016/j.techfore.2023.122567
Gao, R. X., Wang, L., Helu, M., and Teti, R. (2020). Big data analytics for smart factories of the future. CIRP Ann. 69 (2), 668–692. doi:10.1016/j.cirp.2020.05.002
Gao, Y., Hu, Y., Liu, X., and Zhang, H. (2021). Can public R&D subsidy facilitate firms’ exploratory innovation? The heterogeneous effects between central and local subsidy programs. Res. Policy 50 (4), 104221. doi:10.1016/j.respol.2021.104221
Ge, L., Zhao, H., Liu, J., He, T., Zhang, X., and Liu, Y. (2024). Towards green production: how big a role does digital economic contribution play in China? Pol. J. Environ. Stud. 33 (5), 5677–5692. doi:10.15244/pjoes/183795
Goodman-Bacon, A. (2021). Difference-in-differences with variation in treatment timing. J. Econ. 225 (2), 254–277. doi:10.1016/j.jeconom.2021.03.014
Hall, B. H., Jaffe, A. B., and Trajtenberg, M. (2021). The NBER patent citation data file: lessons, insights and methodological tools.
Hamilton, R. H., and Sodeman, W. A. (2020). The questions we ask: opportunities and challenges for using big data analytics to strategically manage human capital resources. Bus. Horizons 63 (1), 85–95. doi:10.1016/j.bushor.2019.10.001
Han, F., and Mao, X. (2023). Impact of intelligent transformation on the green innovation quality of Chinese enterprises: evidence from corporate green patent citation data. Appl. Econ. 56, 5342–5359. doi:10.1080/00036846.2023.2244256
Han, F., Mao, X., Yu, X., and Yang, L. (2024). Government environmental protection subsidies and corporate green innovation: evidence from Chinese microenterprises. J. Innovation and Knowl. 9 (1), 100458. doi:10.1016/j.jik.2023.100458
Hausman, J. A., and Taylor, W. E. (1981). Panel data and unobservable individual effects. Econ. J. Econ. Soc. 49, 1377–1398. doi:10.2307/1911406
Holmstrom, B. (1989). Agency costs and innovation. J. Econ. Behav. and Organ. 12 (3), 305–327. doi:10.1016/0167-2681(89)90025-5
Hong, W., and Su, Y. S. (2013). The effect of institutional proximity in non-local university–industry collaborations: an analysis based on Chinese patent data. Res. Policy 42 (2), 454–464. doi:10.1016/j.respol.2012.05.012
Jia, N., Huang, K. G., and Man Zhang, C. (2019). Public governance, corporate governance, and firm innovation: an examination of state-owned enterprises. Acad. Manag. J. 62 (1), 220–247. doi:10.5465/amj.2016.0543
Jin, M., and Chen, Y. (2024). Has green innovation been improved by intelligent manufacturing? evidence from listed Chinese manufacturing enterprises. Technol. Forecast. Soc. Change 205, 123487. doi:10.1016/j.techfore.2024.123487
Kretschmer, T., and Khashabi, P. (2020). Digital transformation and organization design: an integrated approach. Calif. Manag. Rev. 62 (4), 86–104. doi:10.1177/0008125620940296
Kunapatarawong, R., and Martínez-Ros, E. (2016). Towards green growth: how does green innovation affect employment? Res. Policy 45 (6), 1218–1232. doi:10.1016/j.respol.2016.03.013
Kusiak, A. (2017). Smart manufacturing must embrace big data. Nature 544 (7648), 23–25. doi:10.1038/544023a
Laursen, K., and Salter, A. (2006). Open for innovation: the role of openness in explaining innovation performance among UK manufacturing firms. Strategic Manag. J. 27 (2), 131–150. doi:10.1002/smj.507
Lee, C. C., Qin, S., and Li, Y. (2022). Does industrial robot application promote green technology innovation in the manufacturing industry? Technol. Forecast. Soc. Change 183, 121893. doi:10.1016/j.techfore.2022.121893
Lee, H. (2023). Converging technology to improve firm innovation competencies and business performance: evidence from smart manufacturing technologies. Technovation 123, 102724. doi:10.1016/j.technovation.2023.102724
Li, G., and Branstetter, L. G. (2024). Does “Made in China 2025” work for China? Evidence from Chinese listed firms. Res. Policy 53 (6), 105009. doi:10.1016/j.respol.2024.105009
Li, K., Zhang, L., Fu, H., and Liu, B. (2023). The effect of intelligent manufacturing on remanufacturing decisions. Comput. and Industrial Eng. 178, 109114. doi:10.1016/j.cie.2023.109114
Liang, R., Wen, X., and Zhu, S. (2023). The impact of emission charges on the quality of corporate innovation: based on the perspective of breakthrough technological innovation. J. Clean. Prod. 404, 136830. doi:10.1016/j.jclepro.2023.136830
Lin, C., Liu, S., and Manso, G. (2021). Shareholder litigation and corporate innovation. Manag. Sci. 67 (6), 3346–3367. doi:10.1287/mnsc.2020.3626
Lin, W., Yu, W., and Xiao, R. (2023). Measuring patent similarity based on text mining and image recognition. Systems 11 (6), 294. doi:10.3390/systems11060294
Liu, J., Chang, H., Forrest, J. Y. L., and Yang, B. (2020). Influence of artificial intelligence on technological innovation: evidence from the panel data of China’s manufacturing sectors. Technol. Forecast. Soc. Change 158, 120142. doi:10.1016/j.techfore.2020.120142
Liu, X., Huang, N., Su, W., and Zhou, H. (2024). Green innovation and corporate ESG performance: evidence from Chinese listed companies. Int. Rev. Econ. and Finance 95, 103461. doi:10.1016/j.iref.2024.103461
Liu, Y., Luo, N., and Wu, S. (2019). Nonlinear effects of environmental regulation on environmental pollution. Discrete Dyn. Nat. Soc. 2019, 1–10. doi:10.1155/2019/6065396
Lyu, W., Wang, T., Hou, R., and Liu, J. (2023). Going green and profitable: the impact of smart manufacturing on Chinese enterprises. Comput. and Industrial Eng. 181, 109324. doi:10.1016/j.cie.2023.109324
Ma, R., and Lin, B. (2024). Internet development, information availability, and Chinese enterprises’ cooperative green technology innovation. J. Environ. Manag. 367, 121972. doi:10.1016/j.jenvman.2024.121972
Makri, M., and Scandura, T. A. (2010). Exploring the effects of creative CEO leadership on innovation in high-technology firms. Leadersh. Q. 21 (1), 75–88. doi:10.1016/j.leaqua.2009.10.006
Manso, G. (2011). Motivating innovation. J. Finance 66 (5), 1823–1860. doi:10.1111/j.1540-6261.2011.01688.x
Maslach, D. (2016). Change and persistence with failed technological innovation. Strategic Manag. J. 37 (4), 714–723. doi:10.1002/smj.2358
Menon, N. M. (2018). Information spillover and semi-collaborative networks in insurer fraud detection. MIS Q. 42 (2), 407–426. doi:10.25300/misq/2018/14433
Mikalef, P., Boura, M., Lekakos, G., and Krogstie, J. (2019). Big data analytics capabilities and innovation: the mediating role of dynamic capabilities and moderating effect of the environment. Br. J. Manag. 30 (2), 272–298. doi:10.1111/1467-8551.12343
Nambisan, S., Lyytinen, K., Majchrzak, A., and Song, M. (2017). Digital innovation management: reinventing innovation management research in a digital world. MIS Q. 41 (1), 223–238. doi:10.25300/misq/2017/41:1.03
Nunn, N., and Qian, N. (2014). US food aid and civil conflict. Am. Econ. Rev. 104 (6), 1630–1666. doi:10.1257/aer.104.6.1630
Ortega-Argiles, R., Moreno, R., and Caralt, J. S. (2005). Ownership structure and innovation: is there a real link? Ann. Regional Sci. 39, 637–662. doi:10.1007/s00168-005-0026-6
Perez-Arostegui, M. N., Benitez-Amado, J., and Tamayo-Torres, J. (2012). Information technology-enabled quality performance: an exploratory study. Industrial Manag. and Data Syst. 112 (3), 502–518. doi:10.1108/02635571211210095
Peteraf, M. A. (1993). The cornerstones of competitive advantage: a resource-based view. Strategic Manag. J. 14 (3), 179–191. doi:10.1002/smj.4250140303
Porter, M. E., and Linde, C. V. D. (1995). Toward a new conception of the environment-competitiveness relationship. J. Econ. Perspect. 9 (4), 97–118. doi:10.1257/jep.9.4.97
Qi, Y., Tang, Y., and Bai, T. (2024). Impact of smart city pilot policy on heterogeneous green innovation: micro-evidence from Chinese listed enterprises. Econ. Change Restruct. 57 (2), 67. doi:10.1007/s10644-024-09654-5
Rennings, K. (2000). Redefining innovation—eco-innovation research and the contribution from ecological economics. Ecol. Econ. 32 (2), 319–332. doi:10.1016/s0921-8009(99)00112-3
Ribeiro-Navarrete, S., Botella-Carrubi, D., Palacios-Marqués, D., and Orero-Blat, M. (2021). The effect of digitalization on business performance: an applied study of KIBS. J. Bus. Res. 126, 319–326. doi:10.1016/j.jbusres.2020.12.065
Salge, T. O., Farchi, T., Barrett, M. I., and Dopson, S. (2013). When does search openness really matter? A contingency study of health-care innovation projects. J. Prod. Innovation Manag. 30 (4), 659–676. doi:10.1111/jpim.12015
Shahzad, M., Qu, Y., Rehman, S. U., and Zafar, A. U. (2022). Adoption of green innovation technology to accelerate sustainable development among manufacturing industry. J. Innovation and Knowl. 7 (4), 100231. doi:10.1016/j.jik.2022.100231
Shang, C., and You, F. (2019). Data analytics and machine learning for smart process manufacturing: recent advances and perspectives in the big data era. Engineering 5 (6), 1010–1016. doi:10.1016/j.eng.2019.01.019
Sharif, M. N. (2012). Technological innovation governance for winning the future. Technol. Forecast. Soc. Change 79 (3), 595–604. doi:10.1016/j.techfore.2011.12.004
Shen, Y., and Zhang, X. (2023). Intelligent manufacturing, green technological innovation and environmental pollution. J. Innovation and Knowl. 8 (3), 100384. doi:10.1016/j.jik.2023.100384
Shukla, N., Tiwari, M. K., and Beydoun, G. (2019). Next generation smart manufacturing and service systems using big data analytics. Comput. and Industrial Eng. 128, 905–910. doi:10.1016/j.cie.2018.12.026
Song, Y., Zhang, L., Dong, X., and Zhang, M. (2024). Can environmental regulation foster incremental enhancement and quality improvement in green technological innovation under the background of the digital development? Environ. Dev. Sustain., 1–17. doi:10.1007/s10668-024-04862-5
Sun, L., and Abraham, S. (2021). Estimating dynamic treatment effects in event studies with heterogeneous treatment effects. J. Econ. 225 (2), 175–199. doi:10.1016/j.jeconom.2020.09.006
Tanaka, S., Yin, W., and Jefferson, G. H. (2014). Environmental regulation and industrial performance: evidence from China. Work. Pap. Medford, MA: Tufts Univ.
Teece, D. J. (2007). Explicating dynamic capabilities: the nature and microfoundations of (sustainable) enterprise performance. Strategic Manag. J. 28 (13), 1319–1350. doi:10.1002/smj.640
Tödtling, F., Lehner, P., and Kaufmann, A. (2009). Do different types of innovation rely on specific kinds of knowledge interactions? Technovation 29 (1), 59–71. doi:10.1016/j.technovation.2008.05.002
Van Alstyne, M. W., Parker, G. G., and Choudary, S. P. (2016). Pipelines, platforms, and the new rules of strategy. Harv. Bus. Rev. 94 (4), 54–62.
Verhoef, P. C., Broekhuizen, T., Bart, Y., Bhattacharya, A., Dong, J. Q., Fabian, N., et al. (2021). Digital transformation: a multidisciplinary reflection and research agenda. J. Bus. Res. 122, 889–901. doi:10.1016/j.jbusres.2019.09.022
Verhoeven, D., Bakker, J., and Veugelers, R. (2016). Measuring technological novelty with patent-based indicators. Res. Policy 45 (3), 707–723. doi:10.1016/j.respol.2015.11.010
Wang, C., Chen, P., Hao, Y., and Dagestani, A. A. (2022a). Tax incentives and green innovation—the mediating role of financing constraints and the moderating role of subsidies. Front. Environ. Sci. 10, 1067534. doi:10.3389/fenvs.2022.1067534
Wang, C., and Wang, L. (2024). Does broadband infrastructure promote urban innovation? Evidence from “Broadband China” demonstration policy. Struct. Change Econ. Dyn. 69, 349–362. doi:10.1016/j.strueco.2024.01.005
Wang, H., Hao, Y., and Fu, Q. (2024a). Data factor agglomeration and urban green finance: a quasi-natural experiment based on the National Big Data Comprehensive Pilot Zone. Int. Rev. Financial Analysis 96, 103732. doi:10.1016/j.irfa.2024.103732
Wang, H., Zheng, L. J., Zhang, J. Z., Kumar, A., and Srivastava, P. R. (2024b). Unpacking complementarity in innovation ecosystems: a configurational analysis of knowledge transfer for achieving breakthrough innovation. Technol. Forecast. Soc. Change 198, 122974. doi:10.1016/j.techfore.2023.122974
Wang, J., Xu, C., Zhang, J., and Zhong, R. (2022b). Big data analytics for intelligent manufacturing systems: a review. J. Manuf. Syst. 62, 738–752. doi:10.1016/j.jmsy.2021.03.005
Wang, N., Xiao, M., and Savin, I. (2021). Complementarity effect in the innovation strategy: internal R&D and acquisition of capital with embodied technology. J. Technol. Transf. 46 (2), 459–482. doi:10.1007/s10961-020-09780-y
Wang, X., Wang, S., Wu, K., Zhai, C., and Li, Y. (2024c). Environmental protection tax and enterprises’ green technology innovation: evidence from China. Int. Rev. Econ. and Finance 96, 103617. doi:10.1016/j.iref.2024.103617
Wei, X., Jiang, F., Chen, Y., and Hua, W. (2024). Towards green development: the role of intelligent manufacturing in promoting corporate environmental performance. Energy Econ. 131, 107375. doi:10.1016/j.eneco.2024.107375
Wernerfelt, B. (1984). A resource-based view of the firm. Strategic Manag. J. 5 (2), 171–180. doi:10.1002/smj.4250050207
West, J., and Bogers, M. (2014). Leveraging external sources of innovation: a review of research on open innovation. J. Prod. Innovation Manag. 31 (4), 814–831. doi:10.1111/jpim.12125
Wu, F. S., Tsai, C. C., Wu, C. H., and Lo, T. H. (2024). An exploratory study of breakthrough innovations in digital businesses: the case of the Perfect Corporation. Technol. Forecast. Soc. Change 201, 123233. doi:10.1016/j.techfore.2024.123233
Xu, Y., Yang, C., Ge, W., Liu, G., Yang, X., and Ran, Q. (2023). Can industrial intelligence promote green transformation? New insights from heavily polluting listed enterprises in China. J. Clean. Prod. 421, 138550. doi:10.1016/j.jclepro.2023.138550
Yan, Z., Yu, Y., Du, K., and Zhang, N. (2024). How does environmental regulation promote green technology innovation? Evidence from China’s total emission control policy. Ecol. Econ. 219, 108137. doi:10.1016/j.ecolecon.2024.108137
Yang, H., Li, L., and Liu, Y. (2022). The effect of manufacturing intelligence on green innovation performance in China. Technol. Forecast. Soc. Change 178, 121569. doi:10.1016/j.techfore.2022.121569
Yang, S., and Liu, F. (2024). Impact of industrial intelligence on green total factor productivity: the indispensability of the environmental system. Ecol. Econ. 216, 108021. doi:10.1016/j.ecolecon.2023.108021
Yang, Z., and Shen, Y. (2023). The impact of intelligent manufacturing on industrial green total factor productivity and its multiple mechanisms. Front. Environ. Sci. 10, 1058664. doi:10.3389/fenvs.2022.1058664
Zardkoohi, A., Harrison, J. S., and Josefy, M. A. (2017). Conflict and confluence: the multidimensionality of opportunism in principal–agent relationships. J. Bus. Ethics 146, 405–417. doi:10.1007/s10551-015-2887-7
Zhang, H., Wu, J., Mei, Y., and Hong, X. (2024). Exploring the relationship between digital transformation and green innovation: the mediating role of financing modes. J. Environ. Manag. 356, 120558. doi:10.1016/j.jenvman.2024.120558
Zhang, N., You, D., Tang, L., and Wen, K. (2023). Knowledge path dependence, external connection, and radical inventions: evidence from Chinese Academy of Sciences. Res. Policy 52 (4), 104738. doi:10.1016/j.respol.2023.104738
Zhao, X., and Qian, Y. (2024). Does digital technology promote green innovation performance? J. Knowl. Econ. 15 (2), 7568–7587. doi:10.1007/s13132-023-01410-w
Zhong, R. Y., Xu, X., Klotz, E., and Newman, S. T. (2017). Intelligent manufacturing in the context of industry 4.0: a review. Engineering 3 (5), 616–630. doi:10.1016/j.eng.2017.05.015
Keywords: intelligent manufacturing, breakthrough in green innovation, green open innovation, information asymmetry, ESG performance
Citation: Dong X, Du Y, Zhao M and Xiao X (2025) Does intelligent manufacturing promote a breakthrough in green innovation? Evidence from China. Front. Environ. Sci. 13:1511469. doi: 10.3389/fenvs.2025.1511469
Received: 15 October 2024; Accepted: 08 January 2025;
Published: 31 January 2025.
Edited by:
Irfan Ali, Aligarh Muslim University, IndiaCopyright © 2025 Dong, Du, Zhao and Xiao. This is an open-access article distributed under the terms of the Creative Commons Attribution License (CC BY). The use, distribution or reproduction in other forums is permitted, provided the original author(s) and the copyright owner(s) are credited and that the original publication in this journal is cited, in accordance with accepted academic practice. No use, distribution or reproduction is permitted which does not comply with these terms.
*Correspondence: Xiang Xiao, Z2FueXU4ODg4QDEyNi5jb20=
Disclaimer: All claims expressed in this article are solely those of the authors and do not necessarily represent those of their affiliated organizations, or those of the publisher, the editors and the reviewers. Any product that may be evaluated in this article or claim that may be made by its manufacturer is not guaranteed or endorsed by the publisher.
Research integrity at Frontiers
Learn more about the work of our research integrity team to safeguard the quality of each article we publish.