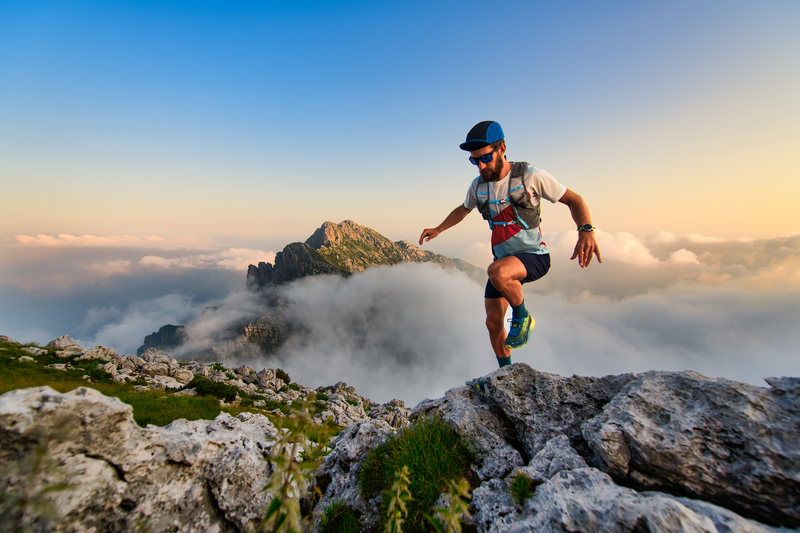
94% of researchers rate our articles as excellent or good
Learn more about the work of our research integrity team to safeguard the quality of each article we publish.
Find out more
ORIGINAL RESEARCH article
Front. Environ. Sci.
Sec. Environmental Economics and Management
Volume 13 - 2025 | doi: 10.3389/fenvs.2025.1511025
The final, formatted version of the article will be published soon.
You have multiple emails registered with Frontiers:
Please enter your email address:
If you already have an account, please login
You don't have a Frontiers account ? You can register here
Industrial carbon emission reduction is not only the need to cope with climate change and environmental pollution, but also an important way to achieve sustainable economic development. This paper first constructs an evaluation system of urban green development index from four dimensions: economy, society, resources and environment. Then, the undesirable super-efficiency SBM model is used to measure the static industrial carbon emission efficiency, and the spatiotemporal characteristics of the dynamic industrial carbon emission efficiency are analyzed by combining the Malmquist index. Finally, the urban green development index was incorporated into the Tobit regression model, and the impact of energy intensity, urban structure and other factors on industrial carbon emission efficiency was considered. This paper cited 18 cities in Sichuan Province from 2015 to 2022 as an example for analysis. The results show that the overall green development level of Sichuan from 2015 to 2022 shows a downward trend, and there is great room for improvement in the level of green development. The average industrial carbon emission efficiency in Sichuan is 0.740, and the difference in industrial carbon emission efficiency is mainly due to the difference in pure technical efficiency. From 2015 to 2022, Sichuan's industrial carbon emission efficiency showed a trend of first stable and then decreasing. There is a significant positive correlation between the level of green development and the efficiency of industrial carbon emissions. Altogether, the industrial carbon emission efficiency model established in this paper is of vital magnitude to the low-carbon development of regional industry.
Keywords: Industrial carbon emission efficiency, Undesirable super-efficiency SBM model, Urban green development, tobit model, Influencing factors
Received: 14 Oct 2024; Accepted: 10 Mar 2025.
Copyright: © 2025 Yang, Feng and Zhao. This is an open-access article distributed under the terms of the Creative Commons Attribution License (CC BY). The use, distribution or reproduction in other forums is permitted, provided the original author(s) or licensor are credited and that the original publication in this journal is cited, in accordance with accepted academic practice. No use, distribution or reproduction is permitted which does not comply with these terms.
* Correspondence:
Tingting Feng, Chengdu University of Technology, Chengdu, China
Disclaimer: All claims expressed in this article are solely those of the authors and do not necessarily represent those of their affiliated organizations, or those of the publisher, the editors and the reviewers. Any product that may be evaluated in this article or claim that may be made by its manufacturer is not guaranteed or endorsed by the publisher.
Research integrity at Frontiers
Learn more about the work of our research integrity team to safeguard the quality of each article we publish.