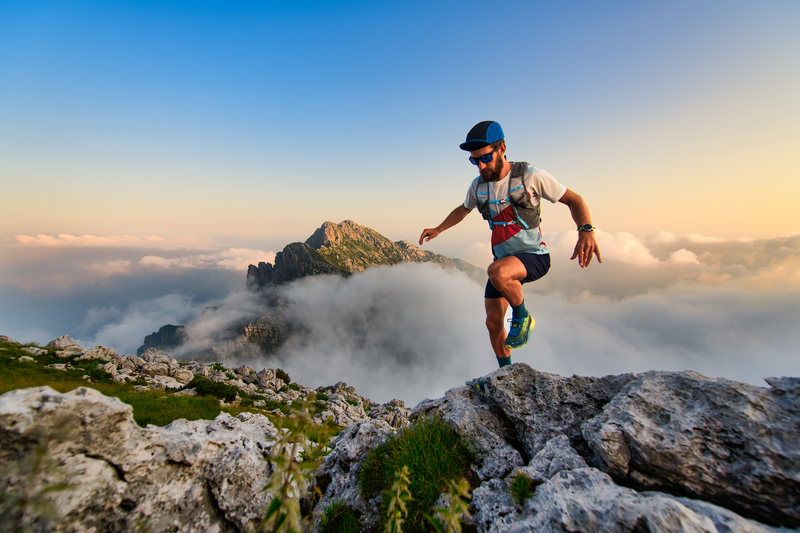
95% of researchers rate our articles as excellent or good
Learn more about the work of our research integrity team to safeguard the quality of each article we publish.
Find out more
ORIGINAL RESEARCH article
Front. Environ. Sci. , 31 March 2025
Sec. Environmental Economics and Management
Volume 13 - 2025 | https://doi.org/10.3389/fenvs.2025.1506794
This article is part of the Research Topic Innovative Approaches to Smart and Sustainable Urban Development in the Global South View all articles
Introduction: In the context of escalating global climate change, cities play a crucial role in promoting green transformation, ecological construction, and achieving sustainable development goals. This study aims to explore the impact of the digital economy and environmental governance on urban green development.
Methods: Based on data from 280 prefecture-level cities in China from 2007 to 2022, we construct a new indicator system to measure urban green development. Using a spatial error model, we analyze the relationship between the digital economy, environmental governance, and green urban innovation.
Results: The findings indicate that the digital economy significantly promotes urban green development, with its impact influenced by geographical location and human capital. Environmental governance further enhances this positive effect, making the digital economy an essential driver of green urban innovation. Additionally, the implementation of China’s “Broadband China” policy has strengthened digital infrastructure, fostering green urban innovation and intercity cooperation.
Discussion: The study highlights the need for policymakers to consider geographical heterogeneity and human capital disparities when formulating digital and governance strategies. These insights provide valuable guidance for urban management and environmental protection efforts.
In the tide of globalization and industrialization, environmental issues such as climate change, resource exhaustion, and ecological deterioration are growing increasingly acute, compelling us to re - evaluate our development paradigms. As the refinement and actualization of the concept of sustainable development, gurban reen development endeavors to forge a novel framework of harmonious coexistence between the economy and the environment. It not only underscores the reduction of pollution emissions and the efficient utilization of resources but also champions the attainment of a positive interplay between economic growth and environmental conservation through innovative technologies and policy directives. To instigate profound transformations in the economic structure and development model, it is imperative to discover new economic growth drivers. For example, vigorously promoting the development of sectors such as the digital economy, artificial intelligence, biotechnology, and new energy. These sectors can not only infuse new vigor into the economy but also effectively address the environmental challenges in traditional economic development. As the world’s largest developing nation, China is assuming an increasingly significant role in global climate governance, actively exploring strategies to realign its economic strategy towards a more environmentally - friendly and low - carbon growth trajectory. For instance, China is vigorously advancing the development of clean energy to facilitate the adjustment of the energy structure. Meanwhile, the government encourages industrial upgrading and impels traditional high - pollution and high - energy - consuming industries to undergo a transformation towards a more eco - friendly and energy - efficient orientation. Additionally, China has intensified environmental protection and supervision. In 2023, a series of measures were introduced, such as the “Action Plan for the Sustained Improvement of Air Quality” and the “Administrative Measures for Ecological and Environmental Statistics”, to foster a more environmentally - conscious and sustainable economic development model. Nevertheless, currently, China’s foundation for urban green development remains tenuous. The inefficient industrial structure and the high - pollution energy structure are arduous to alter (Lu et al., 2017; Zhao et al., 2021), and the structural and underlying contradictions between economic development and environmental protection cannot be fundamentally mitigated. Therefore, exploring and implementing the aforementioned new economic growth drivers are of paramount importance for the long - term and healthy development of China’s economy.
In recent years, with the rapid development of new-generation information technologies, the digital economy has become a core force driving changes in the social and economic structure. It not only accelerates the transformation from traditional industrial economies to digital and intelligent economic models but also promotes deep integration and innovation across industries, giving rise to a series of new business forms and models. The digital economy optimizes resource allocation, improves production efficiency (Baoping and Peiwei, 2022), enhances enterprise competitiveness, and injects new momentum into economic growth. At the same time, it drives the intelligent upgrading of social services, improves the quality of life for the public, and makes economic development more inclusive and sustainable. According to the “Global Digital Economy White Paper” released by the China Academy of Information and Communications Technology, the digital economy of 51 countries worldwide reached $41.4 trillion in 2022, accounting for 46.1% of global GDP, marking the digital economy as a key factor in reshaping the global economic landscape. Moreover, China, with its large population base, advanced infrastructure, favorable institutional environment, and abundant data resources, has achieved significant success in the digital economy and has become a global focal point. China’s digital economy reached $7.47 trillion in 2022, accounting for 41.5% of GDP. The national “14th Five-Year Plan” clearly states that by 2025, the added value of core digital industries should account for 10% of GDP, establishing the digital economy as a new driver of economic growth. Therefore, studying the impact of the digital economy on China’s green development has become crucial.
Research into the unbalanced development across regions within the realm of spatial economic theory lays a groundwork for comprehending the distinctive challenges and opportunities that diverse regions encounter on the journey of sustainable development. For example, certain regions might occupy an advantageous stance in the green transition owing to propitious natural conditions or robust technological innovation capabilities. The development paradigm advocated by the theory of sustainable transformation can proffer novel perspectives for tackling the inequality quandaries in spatial economy. For instance, by fostering renewable energy technologies, it can diminish the reliance on non - renewable resources and ameliorate the issue of regional decline triggered by resource exhaustion. Moreover, in the course of promoting a more equitable spatial development pattern on a global scale or within a nation, it is also requisite to depend on policy design steered by the theory of sustainable transformation to guarantee that the green development trajectories of all regions are practicable and all - encompassing.
Based on this background, this paper uses panel data from 280 prefecture-level cities in China from 2007 to 2022, employs a spatial error model to empirically test the impact of the digital economy on urban green development and its mechanism of action, and deeply analyzes how environmental governance influences the relationship between the digital economy and urban green development. Additionally, the study focuses on green urban innovation and changes in the urban environment, as well as discussing the heterogeneity of urban human capital and geographical location. This research aims to provide theoretical support and policy recommendations for urban-level green transformation under the deepening reform and opening-up context and to achieve the “dual carbon” goals.
In recent times, the academic realm has carried out a meticulous exploration of the pre - mechanisms for propelling green development. It not only underscores the significance of governmental policies, the transformation of economic paradigms, alterations in social conduct, and ecological conservation but also devotes special attention to how digital technologies can endow urban green development with impetus. First, from the vantage point of institutional economics, research accentuates the function of government policies, regulations, and institutional frameworks in fostering urban green development. For instance, environmental regulations exert an influence on urban green development via avenues such as innovation compensation, structural optimization, and green credit (Wu et al., 2020; Chen et al., 2022; Wang L. et al., 2022). Moreover, the selection of government policy instruments, like subsidies, tax incentives, and emission trading systems, assumes a pivotal role in spurring enterprises to invest in environmental protection and technological innovation (Yu et al., 2022; Jiang et al., 2023; Benkhodja et al., 2023). Second, research regarding economic model transformation and technological advancement reveals that these factors have made substantial contributions to urban green development. This encompasses the greening of energy, the implementation of novel technologies, and their impacts on enhancing resource efficiency and curbing emissions (Yang et al., 2020; Madaleno et al., 2022). Particularly, technological innovation is deemed a significant impetus for elevating the quality of economic growth (Liu et al., 2018; Shen et al., 2021). Third, the transformation of social behavior patterns and the augmentation of public awareness are acknowledged as momentous forces for propelling urban green development. Cultivating green consumption habits and heightening public involvement in environmental protection endeavors contribute to the establishment of sustainable consumption patterns and the overall shift of society towards a green trajectory (Ogiemwonyi et al., 2020; Zameer and Yasmeen, 2022; Lopes et al., 2023; Gomes and Lopes, 2024). Fourth, the significance of safeguarding and restoring natural ecosystem services is extensively recognized as an integral part of supporting human wellbeing and development. Research in this domain entails the evaluation of ecological carrying capacity and the formulation of biodiversity conservation strategies to guarantee that development does not jeopardize the health and service capabilities of ecosystems (Kong et al., 2010; Wei et al., 2015; Pera, 2020; Wu et al., 2021). Ultimately, the digital - technology - empowered urban green development has emerged as a novel research domain, delving into how big data, cloud computing, the Internet of Things, and artificial intelligence can foster green urban innovation by enhancing the efficiency of resource allocation and optimizing production and supply - chain management. For instance, investigated how big data and cloud computing can assist cities in more effectively monitoring and managing environmental pollution, thereby offering data support for formulating more scientific and rational environmental protection policies. This data - driven decision - making process contributes to enhancing the transparency and enforceability of policies, thus facilitating urban green development deliberated on how the Internet of Things and artificial intelligence technologies can be applied in energy management and industrial production to attain the objective of energy conservation and emission reduction. By optimizing resource allocation and augmenting production efficiency, these technologies not only propel the transformation and upgrading of traditional industries into green industries but also furnish technological support for the sustainable development of cities. The research conducted by Si et al. (2024) manifested how social media and mobile applications can stimulate public participation in environmental protection activities. Through these platforms, information regarding green lifestyles can be effectively disseminated, and more individuals can be motivated to engage in resource - saving and environment - protecting behaviors. Additionally, some scholars have also explored the utilization of remote sensing technology and geographic information systems (GIS) to monitor alterations in natural ecosystems, which is of paramount importance for promptly detecting and resolving ecological problems (Abbass et al., 2022; Begum et al., 2022). This implies that digital technology can also play a significant role in safeguarding and restoring natural ecosystem services.
In essence, be it policy innovation from the vantage point of institutional economics or specific technological implementations like the Internet of Things and artificial intelligence, digital technology assumes an inalienable role in invigorating urban green development. Simultaneously, this also mirrors the intimate interconnection among the aforementioned five dimensions, which jointly constitute a comprehensive framework for propelling urban green development.
In recent years, academic research has extensively explored the antecedent driving mechanisms of urban green development, covering multiple dimensions such as policy and institutional levels, economic and technological levels, social and cultural levels, as well as ecological and environmental levels. However, there are still deficiencies in existing studies regarding the relationship between digital economy and urban green development. Particularly, discussions are lacking on how environmental governance within specific spatial ranges affects the process by which the digital economy influences urban green development. This paper aims to fill this research gap by constructing a comprehensive analytical framework that includes digital economy, environmental governance, and urban green development, to deeply explore the interactive relationships among the three. Specifically, this paper uses detailed datasets and advanced econometric methods to systematically examine the actual impact of the digital economy on urban green development under different scenarios; at the same time, it introduces environmental governance as a key variable to explore how it alters or strengthens the urban green development effects brought about by the digital economy. This study not only provides new perspectives for understanding the challenges China faces in achieving its dual carbon goals in a global context but also contributes valuable insights for formulating more precise and effective urban green development strategies.
Some studies have posited that the advancement of the digital economy can result in a substantial rise in electricity consumption, consequently amplifying carbon emissions (Li et al., 2021; Solarin et al., 2021; Salahuddin and Alam, 2015). The development of the digital economy can curb carbon emissions by improving energy efficiency and promoting industrial intelligence and green transformation. Specifically, the digital industry not only helps reduce carbon emissions itself but also drives other industries to optimize production processes and reduce energy consumption; at the same time, the advancement of information and communication technology and the Internet has reduced dependence on traditional high-carbon emission methods, thus promoting low-carbon economic development as a whole (Lyu et al., 2023; Si et al., 2024). Therefore, the promotion and technological penetration of the digital economy can effectively reduce regional carbon emissions and accelerate industrial upgrading and green transformation. Furthermore, the extensive implementation of digital technology amplifies the efficacy and precision of eco-friendly urban management. By constructing intelligent sensory networks and large data analytics platforms, municipalities can surveil environmental statuses and resource deployment in real-time, thereby escalating resource utilization efficiency and curtailing ecological contamination (Wan and Shi, 2022). The advancement of digital infrastructure contributes to mitigating pollutant discharges through the advocacy of green urban innovation (Nie et al., 2023). Additionally, the harnessing and exploitation of big data can dismantle informational barriers, amalgamate environmental data to engender a scientific surveillance system, and supply a foundation for pollution reduction and efficient governance (Gan et al., 2023; He et al., 2024). The digital economy has also engendered green urban innovation and technological progression. The consistent metamorphosis and deployment of digital technology have catalyzed the swift advancement of environmentally sustainable industries. The ubiquitous application of green technologies renders cities more ecologically benign and energy-efficient across domains such as energy, transportation, and construction, thereby imparting a fresh impetus to urban green development. The digital economy exerts an influence on the green urban innovation capabilities of cities, and the evolution of digital technology can invigorate technological innovation endeavors and augment innovation efficiency (He et al., 2024). This can propel the development of green urban innovation prowess. At the stage of refining digital economic development with a high level of dynamism, the digital economy’s role in stimulating green urban innovation becomes more pronounced (Luo et al., 2023). Hence, we proposed the following hypothesis:
H1:. The local development of the digital economy promotes the improvement of urban green developments.
From a global vantage point, local governments, as the executors of the central government’s environmental governance policies, assume a pivotal role in propelling the urban green development. By establishing a rational institutional framework and strategic blueprint, local governments can fully unleash the potential of the digital economy in facilitating the green transformation of cities. The advancement of the digital economy not only presents enterprises with opportunities for technological innovation but also fosters the efficient allocation of resources and enhances the efficiency of information dissemination (Lu et al., 2023). An effective environmental regulatory regime is one of the linchpin factors in attaining this objective. Firstly, against diverse national backdrops, there exist substantial disparities in the form and intensity of environmental regulatory policies, which directly influence the innovation capabilities and technological choices of enterprises. For example, in certain developed nations, incentive policies are amalgamated with stringent environmental criteria, spurring enterprises to actively explore data - driven energy - saving and emission - reduction technologies. Conversely, some developing countries may place greater emphasis on employing lenient yet guiding policies to promote the application of digital solutions (Wang L. P. et al., 2022). This differentiated strategy exerts an impact on the trajectories of digital - driven environmental transformation on a global scale. Moreover, more rigorous environmental laws, regulations, and standards exert varying degrees of influence on enterprises’ adoption of clean technologies within different national contexts, thereby augmenting the demand for digital solutions. For instance, the European Union’s General Data Protection Regulation (GDPR) not only reinforces the protection of personal data but also sets exacting standards for data application in the environmental protection domain, propelling the development of relevant digital technologies. In emerging economies such as China, the government actively promotes smart city and big data strategies to expedite the green transformation of the economic structure (Hao et al., 2023). Finally, regarding the impact of environmental regulation on green total factor productivity, an appropriate degree of environmental regulation contributes to enhancing green total factor productivity, yet the specific circumstances vary from one country to another. Therefore, when formulating an appropriate regulatory framework, it is imperative to take local actualities into account to ensure that advanced technological means can be utilized efficiently to enhance the efficiency of environmental management while averting over - suppressing the enthusiasm of enterprises and the adaptability of the market (Zhan et al., 2023). In conclusion, an in-depth analysis of how environmental regulatory policies in different countries affect digital-driven environmental transformation is of utmost significance for formulating more scientific and rational urban green development strategies (Figure 1). Consequently, we posit the following hypothesis:
H2:. Environmental governance plays a moderating role in the impact of the digital economy on urban green development.
In the realm of geographical spatial analysis, regions with comparatively proximate physical distances typically manifest more analogous characteristics. This phenomenon is termed spatial autocorrelation. To corroborate and conduct a more in - depth analysis of this spatial correlation, this study concurrently employed the Global Moran’s I index and the Local Moran’s I index. The significance of performing spatial correlation verification and analysis resides in discerning whether the data distribution pattern is random, clustered, or uniform, thereby providing a foundation for subsequent spatial modeling and facilitating the identification of potential spatial processes or mechanisms. The Global Moran’s I index serves as a means to gauge whether variables across the entire study area display a clustering tendency. It aids us in comprehending whether the observations within the data are randomly dispersed, uniformly distributed, or exhibit a certain degree of clustering. Through the calculation of the Global Moran’s I index, we can appraise the extent of spatial autocorrelation of a particular variable throughout the entire study area and ascertain whether a significant spatial clustering phenomenon exists. This step is of paramount importance for validating the existence of a general spatial association and lays the groundwork for further in - depth exploration. Conversely, the Local Moran’s I index centers on each individual observation point or region to detect spatial autocorrelation at the local level. It can not only uncover which regions form “hot spots” or “cold spots” clusters, namely, regions where high values are contiguous to high values or low values are adjacent to low values, but also pinpoint the outliers where high values are in proximity to low values. The Local Moran’s I index can offer more meticulous insights, indicating which specific regional relationships make substantial contributions to the overall pattern, which holds great significance for formulating targeted policy intervention measures. In conclusion, the Global Moran’s I index is primarily concerned with detecting spatial autocorrelation on a macro - scale, whereas the Local Moran’s I index focuses on analyzing spatial heterogeneity at the micro - level. The combined utilization of the two can comprehensively elucidate the spatial structural characteristics of the data and the possible mechanisms underlying them. The specific calculation formula is shown in Equations 1, 2:
In the formula,
The weight matrix is used to measure the spatial distance between cities, reflecting the spatial proximity condition. The distance between region i and region j is
Various types of spatial weights exist. This study used the spatial weight matrix
We used the inverse of the distance between spatial units, where
Adjacency Matrix: We use the spatial proximity matrix as a robustness test, adjacency matrix was shown in Matrix 5:
Tobler’s First Law of Geography emphasizes the importance of spatial relationships, stating that everything is related to everything else, but things closer in proximity are generally more strongly related than things farther apart (Tobler, 1970). When analyzing spatial data, relying solely on traditional econometric methods may not adequately account for this proximity effect, potentially leading to biased estimation results. This is because traditional econometrics assumes independence between observations, whereas in reality, observations within a region may be influenced by those in neighboring regions, constituting spatial interdependence. To more accurately interpret and analyze spatial data, spatial econometric models offer an improved approach. These models can not only capture spatial dependencies but also quantify the extent of these relationships, leading to more accurate parameter estimation. By introducing spatial lag terms, spatial econometric models can account for spatial correlation variables that are crucial to the outcome but overlooked by non-spatial models, thereby reducing estimation error (Yang et al., 2018). Moreover, spatial econometric models are also significant for policy evaluation. They can help us understand how policy changes implemented in one area may influence other regions through spatial interactions and assess differences in regional responses. Therefore, when dealing with data that exhibits clear spatial characteristics, it is essential to adopt spatial econometric methods, as they can provide more authentic and reliable analysis results, offering a scientific basis for policymakers (Dubé et al., 2014).
When delving into the synergistic influence of the digital economy and environmental governance on the urban green development, the Spatial Error Model (SEM) is primarily chosen as this model can effectively address the data error issues engendered by spatial dependence. The urban green development represents a complex process. Cities engage with one another via diverse channels, such as the economy, technology, and policies. This interaction might give rise to spatial autocorrelation errors in the observed data. SEM centers on capturing and rectifying the correlation among error terms resulting from the influence of neighboring regions, which is especially significant when analyzing cross -urban green development matters. In comparison to the Spatial Lag Model (SLM) or the Spatial Durbin Model (SDM), SEM is more appropriate for scenarios where the direct impact of intra - regional factors is the principal concern and potential errors owing to spatial spill - over effects need to be controlled. Hence, the utilization of SEM contributes to a more precise assessment of the actual effects of the digital economy and environmental governance measures on the urban green development and offers more dependable research conclusions. The model construction is shown in Equation 6:
The model for the regulatory effect of environmental governance, denoted as
where i is the city; t is the year;
When studying the impact of digital economy development (DE) on urban ground space development (UGD), scholars often encounter difficulties in determining causal relationships, which is mainly due to potential endogeneity issues. To more accurately assess the specific impact of DE growth on urban green development and overcome the challenge of endogeneity, this study uses the “Broadband China” initiative as an exogenous variable for analysis. The “Broadband China” policy, as a national-level strategy, provides an opportunity for this study with its pilot implementation, offering a quasi-natural experiment. By selecting the policy as an exogenous shock source, researchers can observe changes in urban green development brought about by DE development under relatively controlled conditions, thereby effectively reducing the potential endogeneity interference between the dependent variable (UGD) and the independent variable (DE). This methodological choice is based on the following considerations: Firstly, the implementation of “Broadband China” is independent of local economic activities, thus it can be considered a relatively exogenous factor; secondly, the gradual rollout of the policy makes it possible to adopt the Difference-in-Differences (DID) model, which can better separate the policy effect from other time trend factors; finally, considering the interactions between geographically adjacent areas, this study also introduces methods from spatial econometrics to further correct for spatial correlations and spillover effects. See Equations 10, 11 for details.
According to the studies conducted by (Li and Wang, 2023; Wang et al., 2021), urban green development can be bifurcated into two facets: green technology and the ecological environment. Among these, green technology is gauged by the quantity of green invention applications in the current year, for the number of green inventions can directly mirror the innovation capacity and activity level of a region in environmental protection and sustainable development technologies. The ecological environment encompasses environmental quality, air - polluting gases, and air pollutants, as these are all pivotal indicators for assessing the ecological wellbeing status of a region and are directly associated with human health and ecological equilibrium. The specific indicator system is presented in Table 1. Given that the entropy weight method can objectively ascertain the weight of each indicator in accordance with the degree of data variation, it can effectively circumvent the deviation engendered by subjective weighting. Moreover, by quantifying the degree of information disorder, this method can more scientifically and equitably reflect the genuine contribution of each green indicator to the overall urban green development level, enhancing the precision and reliability of the evaluation results. Consequently, the entropy weight method is employed to measure the urban green development level. The specific calculation process of the entropy weight method is shown in Formulas 12–18:
Initially, standardization processing is necessary. This process is vital in data analysis as it guarantees the comparability of data across different indicators. By removing the discrepancies in measurement units and equalizing the weights of variables, it allows indicators of diverse scales to be compared on an equal footing, which is key to enhancing model performance and analysis accuracy. Once standardized, a higher value of a positive indicator is more favorable, whereas for a negative indicator, the situation is reversed. This approach not only addresses the issues stemming from data scale differences but also speeds up the algorithm convergence. It ensures that every indicator takes part in the analysis fairly, thus establishing a robust basis for subsequent data handling and model building. Assuming the original matrix is
Positive indicator:
Negative indicator:
where xij represents the initial value of indicator j for sample i, vij represents the standard value of indicator j for sample i, and i = 1,2 … m; j = 1,2 … n.
a. Secondly, we analyze the disparities among indicators within each sample. Based on this analysis, we quantify the information quantity that each indicator offers and subsequently decide its weight. In the overall evaluation framework, an indicator’s weight represents its relative significance. Specifically, a greater weight implies a more substantial contribution of the indicator to the overall assessment. This approach can objectively mirror the function of each indicator. It sidesteps the biases introduced by subjective weight - assignment methods, leading to more rational and precise evaluation outcomes. Ensuring the authenticity and reliability of data analysis results hinges significantly on this approach. The calculation of the indicator weights can be found in Formula 14:
b. Thirdly, we computed the information entropy of the indicators to gauge their discriminative power and significance. A lower information entropy indicates greater variability of the indicator across different evaluation targets, meaning it conveys more information and thus should be assigned a higher weight. In contrast, a higher information entropy implies weaker discrimination ability of the indicator, leading to a lower weight. Through the quantification of the information entropy for each indicator, we can objectively and precisely set its weight. This approach offers a scientific foundation for comprehensive assessment and guarantees the fairness and reasonableness of the evaluation outcomes. This procedure effectively captures the relative importance of each indicator within the overall evaluation framework. The formula for calculating the information entropy is presented in Formula 15:
c. Fourth, we computed the redundancy of information entropy to assess the effectiveness of the information that each indicator offers. When the redundancy is low, it suggests that the indicator has less information entropy, more significant data differences, and a greater amount of useful information. As a result, it should be assigned a higher weight. On the contrary, high redundancy implies a large amount of repeated information, which contributes little to decision - making. By calculating the redundancy and then converting it into weights, we can objectively measure the significance of each indicator. This provides a scientific basis for comprehensive evaluations. This approach guarantees that the evaluation system becomes more rational and precise. The formula for calculating the redundancy is presented in Formula 16:
d. Fifth, we computed the weights of individual indicators to measure their relative significance within the overall assessment framework. By calculating the information entropy, we can gauge the variability of each indicator’s data and thereby establish its contribution to the overall assessment. An indicator with a higher weight conveys more information and has a greater impact on the final decision. Proper weight distribution enables a more objective and precise reflection of the comprehensive status of each evaluated entity, improving the scientific basis and dependability of decision - making. This method circumvents the inaccuracies stemming from subjective weight assignment, and it enhances the fairness and rationality of the assessment system. The calculation of these indicator weights is presented in Formula 17:
e. Lastly, once the weights are determined via the entropy weight method, we calculate the comprehensive score of an individual sample. This is achieved by combining the scores of each indicator with their respective weights, enabling us to quantify the sample’s overall performance. This approach not only aggregates data from all evaluation aspects but also applies weighting according to the real - world contribution of each indicator. As a result, it guarantees that the evaluation outcome is more objective and all-encompassing. The comprehensive score precisely represents the overall standing of each sample in relation to all the evaluated indicators. It serves as a scientific benchmark for comparing and ordering different samples, which in turn helps in making more informed and precise decisions. The formula for calculating the comprehensive score is presented in Formula 18:
Taking into account data accessibility and adhering to the methodology employed by (Shi et al., 2023; Zhao et al., 2023), four specific indices were meticulously selected: the count of employees engaged in the information transmission, computer services, and software sectors; the quantity of international Internet users; the per - capita volume of telecommunications services; and the number of mobile phone users at year - end. These indices were chosen as they can comprehensively and directly mirror the core constituents of the digital economy. The number of employees in the information transmission, computer services, and software industries directly reflects the foundation of professional expertise within the digital economy realm. The quantity of international Internet users not only showcases the prevalence of network infrastructure but also reflects the global footprint of digital economic activities. The per - capita volume of telecommunications services comprehensively takes into account individuals’ proficiency in utilizing digital communication technologies and serves as a crucial dimension for gauging the standard of digital life. The number of mobile phone users at year - end, being the most widely adopted indicator of smart device utilization, reveals the extent of public involvement in the digital economy. In comparison to other potential alternative indices, these four indices can more precisely capture the pivotal aspects of urban digital economic development. Through principal component analysis, they are effectively integrated, guaranteeing the validity and scientific rigor of the evaluation system, and thereby enabling a more accurate assessment of the overall level of urban digital economic development. The results are presented in Table 2.
The moderating effect denotes the extent of governmental focus on sustainable development. Environmental governance metrics previously established by scholars exhibit a robust correlation with urban green development, thereby engendering endogenous challenges. Consequently, this study, emulating Liu et al., utilized the ratio of word frequency pertaining to environmental protection in local government reports to the total word count in all reports as an instrumental variable for environmental governance (EG) (Liu et al., 2023). Local government reports are typically compiled at the outset of the year, while economic activities are ongoing throughout the year. This approach aids in alleviating endogeneity concerns arising from indicators and measurements of local governmental efforts towards environmental governance. Centering the environmental governance variable is conducted to diminish multicollinearity issues (Dalal and Zickar, 2012; Fairchild and McQuillin, 2010).
The study incorporated control variables to elucidate their influence on urban green development (Jin et al., 2019). Drawing from the research of (Zhou et al., 2020), we selected the following control variables: industry structure (IS), population size (PS), urban innovation ability (UIA), education expenditures (EE), and technology expenditures (TE). The industry structure (IS) is quantified by the ratio of the tertiary sector to the secondary sector. Population size (PS) is expressed as the logarithm of the urban permanent population. Urban innovation ability (UIA) is assessed by the number of new businesses established per 100 inhabitants. Education expenditures (EE) are represented by the proportion of government spending on education relative to GDP. Similarly, technology expenditures (TE) are measured by the proportion of government spending on technology in relation to GDP.
This research concentrates on 280 prefecture-level cities spanning from 2007 to 2022. Green patent information was derived from the National Intellectual Property Administration (2007–2022), while data pertaining to air pollution gases and pollutants were obtained from the China Environmental Monitoring Station. The ecological environment quality index is adapted from the work of (Xu et al., 2021). Details regarding the digital economy and control variables were sourced from the China City Statistical Yearbook (2007–2022). Regions with incomplete data, such as Tibet, Taiwan, Hong Kong, and Macau, have been excluded from the analysis, and gaps in the data have been addressed through interpolation techniques. To counteract the adverse effects of heteroscedasticity on equation estimation, the variables have been subjected to logarithmic transformation. A comprehensive summary of the statistics is provided in Table 3.
From a temporal vantage point, the green transformation in urban hubs generally manifests a positive and upward trajectory (Figure 2). During the period from 2007 to 2022, the urban green development of Chinese cities has witnessed profound metamorphoses. In the initial phase, spanning from 2007 to 2012, the urban green development in the central region trailed behind that in the eastern and western regions, which mirrors the influence of the unbalanced regional economic development on environmental governance investment. Nevertheless, since 2015, with the implementation of the Action Plan for the Prevention and Control of Air Pollution, the urban green development level across the nation has achieved a remarkable leap. This plan is widely esteemed as one of the most stringent environmental protection measures in Chinese history, with the aim of curbing industrial emissions, enhancing air quality, and propelling the optimization and upgrading of the energy structure. The plan not only establishes explicit emission - reduction targets but also reinforces the supervision of polluting enterprises and fosters the application and development of clean energy, thereby spurring a substantial upsurge in the urban green development index of each region. Moreover, the successful execution of the Action Plan for the Prevention and Control of Air Pollution has spurred the introduction of a series of subsequent policies, encompassing but not restricted to those in the domains of water resource protection, soil remediation, and the establishment of ecological protection areas. These measures, acting in concert, have laid a robust foundation for the sustainable development of Chinese cities. Particularly in the central and eastern regions, local governments have actively heeded the call of the central government, augmenting environmental protection investment, promoting green buildings, developing public transportation, and advocating the circular economy, leading to remarkable headway in urban green development in these regions.
Figure 2. The spatiotemporal evolution trend of urban green development (UDG) level in (a) 2007, (b) 2012, (c) 2017, and (d) 2022.
This research employed Moran’s I to elucidate the spatial correlation of the interval population pertaining to urban green development. As delineated in Table 4, the Moran’s I value for urban green development remains positively significant across all years, underscoring the tendency of spatial units exhibiting analogous attributes to aggregate, thus fulfilling the essential conditions requisite for spatial econometric examination. Furthermore, the Z value of Moran’s I has exhibited a descending trajectory from 2007 to 2022.
Figure 3 presents Moran’s I scatter plot, illustrating that in 2011 and 2022, urban green development is predominantly situated in the first and third quadrants, indicating spatial characteristics of high-high clustering and low-low clustering. This observation aligns with the findings of the Global Moran’s I, underscoring pronounced spatial clustering features. Over the period from 2007 to 2022, the spatial agglomeration of green development has diminished, and the distribution of regions with varying levels of urban green development has become more dispersed, no longer manifesting the significant clustering observed in earlier years.
Prior to undertaking a spatial panel regression, we ascertained the necessity of a spatial panel analysis. As illustrated in Table 5, both Lagrange Multiplier (LM) tests for spatial lag effects rejected the null hypothesis of no spatial dependence. Additionally, one of the two LM tests for spatial error effects also refuted the null hypothesis of no spatial dependence.
In this investigation, we initially employed the Stata software and carried out a benchmark regression analysis grounded in Model 10 to appraise the influence of the local digital economy’s development on the urban green development the results are shown in Table 6. As the data in Table 6 (1) indicates, after taking the two-way fixed effects into account, the estimated coefficient of the digital economy stands at 0.0119, signifying that a 1% increment in the digital economy will result in an approximate 1.19% enhancement in the urban green development. This discovery not only validates the statistical significance of the digital economy’s development but also precisely quantifies its economic impact. Namely, for policymakers, fostering the development of the digital economy can be regarded as one of the efficacious strategies to facilitate green growth.
Moreover, the spatial error coefficient λ is also significantly positive. This result suggests that there is a distinct spatial agglomeration phenomenon in the urban green development of Chinese cities. Based on the above benchmark regression results, we further explored the impact of environmental governance (EG) as a moderating variable on the relationship between Digital economy and urban green development. To achieve this, we introduced a spatial error-based moderating effect model on the basis of the baseline regression model. As shown in Table 6 (2), the results of this model indicate that Digital economy still maintains a significant positive impact at the 1% significance level. More importantly, the coefficient of the term representing the interaction between Digital economy and, EG is also significantly positive, meaning that as the intensity of environmental governance increases, the positive impact of Digital economy on urban green development is enhanced. It is worth noting that when incorporating environmental governance as a moderating variable, we adopted a centralized processing method to reduce the potential issue of multicollinearity, ensuring the accuracy and stability of the model estimation (Dalal and Zickar, 2012; Fairchild and McQuillin, 2010). For instance, China’s “Internet Plus” action plan and the smart city initiative both exemplify how to harness digital technologies to foster environmental protection and the attainment of sustainable development goals. By fortifying data sharing, optimizing resource distribution, and augmenting public engagement, these policy measures not only expedite the development of the digital economy but also create a propitious environment for green urban innovation. Hence, the findings of this study imply that policymakers ought to attach significance to the amalgamation of digital economy construction and environmental governance, and proactively explore urban green development strategies tailored to local peculiarities, so as to impel cities to evolve in a more sustainable trajectory. This will not only enrich the theoretical framework but also offer robust guidance for practical operations.
Certain cities reap greater rewards from the impetus of the digital economy on urban green development, primarily owing to the synergistic effect of multiple elements, encompassing but not restricted to government efficacy, regional economic prowess, and digital literacy proficiency. Specifically, cities boasting high - efficiency governance can more adeptly formulate and execute relevant policies, facilitating the efficient allocation of resources and effective environmental conservation. Cities with robust regional economic might typically possess more financial means to underpin the implementation of urban green development initiatives and can draw in more investments. Urban denizens with a high degree of digital literacy are better equipped to adapt to and capitalize on the opportunities presented by the digital economy, spurring technological innovation and application, thus expediting urban green development. Moreover, the resource endowments and developmental phases of diverse regions also dictate the disparities in their potential and trajectories for fostering the green economy in the era of the digital economy. Hence, these factors collectively influence the extent to which each city benefits from the advancement of the digital economy. To conduct a more precise regression analysis, China is partitioned into eastern, central, and western regions after a comprehensive consideration of these factors, with the aim of delving deeply into the distinctive paths and accomplishments of each region in propelling urban green development through the growth of the digital economy. This can not only enable us to comprehend the specific challenges and opportunities in each region in greater detail but also offer more targeted decision - making support for policymakers.
Table 7 presents the results of the geographical heterogeneity analysis. It reveals that the impacts of the digital economy, interaction effects, and the spatial error coefficient λ on urban green development vary distinctly across different regions. In the eastern region, the digital economy has a significantly positive impact on urban green development at the 1% significance level. This clearly shows that the development of the digital economy has notably boosted the urban green development in this area. However, in the central and western regions, although the impact is positive, it is not as pronounced as in the east (Figure 4). This variance can be ascribed to multiple factors. Firstly, the Internet penetration rate is low, and digital infrastructure construction lags behind (Huang et al., 2023). Moreover, there are deeper - seated issues such as low policy efficiency, regulatory loopholes, and an irrational industrial structure. Specifically, the central and western regions have relatively low policy implementation efficiency. As a result, it is challenging to effectively carry out policy measures that support the development of the digital economy. Additionally, compared with the east, the regulatory frameworks in these regions are not yet complete, with rather large regulatory gaps. This fails to create a stable, transparent, and fair-competition market environment for the digital economy. Lax or underdeveloped regulatory policies may cause market disorder, dampen the confidence of enterprises and consumers, and thereby restrain the growth impetus of the digital economy. Simultaneously, the industrial structures in the central and western regions are inclined towards traditional industries. There is insufficient attention and support for emerging industries like the digital economy, which curbs the in - depth integration of the digital economy and urban green development. The scarcity of high - tech talents is also a crucial factor impeding the development of the digital economy in these regions. Owing to the lack of effective talent attraction and cultivation mechanisms, technological innovation and application capabilities are restricted, further influencing the overall development level of the digital economy. Consequently, to promote the urban green development of the central and western regions, it is essential to overcome the above - mentioned obstacles. Policy design needs to be optimized, market supervision should be enhanced, and the industrial structure should be adjusted to fit the requirements of the digital economy era. By enhancing policy efficiency, reducing regulatory gaps, and improving industrial layout, the digital economy can better play its role in promoting urban green development (Zhou et al., 2024).
Human capital profoundly shapes the dynamics of the Digital Economy in the context of urban green development. Utilizing the proportion of the population possessing a bachelor’s degree or higher relative to the total permanent urban populace as an indicator of human capital, data from China were stratified into categories of high and low human capital to facilitate a nuanced heterogeneity analysis.
The findings, encapsulated in Table 8 (Figure 5), reveal that as human capital escalates, the direct impact of the Digital economy on urban green development manifests an upward trajectory. Correspondingly, the affirmative regulatory influence of Economic Growth also ascends. High human capital signifies that a region is endowed with a surfeit of erudite professionals, technical virtuosos, and pioneering innovators. These individuals are better equipped to comprehend, assimilate, and propel digital technologies forward. When the government underscores and advocates for the symbiosis of the Digital economy with urban green development, the conclave of high-caliber talent can furnish indispensable technical substantiation and operational efficacy for pertinent policies and initiatives, thereby expediting the infusion of digital technology into environmental stewardship, resource allocation optimization, and green urban innovation endeavors (Wang M. et al., 2022). Furthermore, communities steeped in high education and specialization exhibit a heightened receptivity and propensity to engage with governmental green directives, offering robust support and impetus and ensuring the more efficacious enforcement of urban green development strategies. Lastly, enterprises and institutions situated in regions with robust human capital are more inclined to under green technology research and investment under the auspices of governmental encouragement (Jin et al., 2023).
In this academic manuscript, a bidirectional fixed-effect spatial error model is utilized, incorporating multiple control variables to mitigate issues of endogeneity, particularly those arising from omitted variables. Recognizing the potential for mutual causation between the digital economy and urban green development, which could introduce endogeneity problems, several strategies are employed to address this concern.
Firstly, to account for the spatial dependencies and potential endogeneity, the study adopts a spatial proximity weight matrix. Additionally, to further address the endogeneity arising from simultaneity, the variable representing the digital economy is lagged by one period. This approach helps to alleviate the issue of reverse causality, assuming that the digital economy influences urban green development with a time lag. Moreover, to ensure the robustness of the findings, the dataset undergoes trimming by excluding the top 1% and bottom 1% outliers. This truncation aims to reduce the influence of extreme values that might skew the regression results. Additionally, an alternative spatial weight matrix based on adjacency is utilized in the robustness checks, providing a different perspective on spatial interactions and allowing for a comparison of results under varying specifications. Furthermore, to account for other unobserved factors that may simultaneously influence both DE and urban green development, the model is adjusted accordingly. The inclusion of these controls helps to isolate the specific effect of Digital economy on urban green development.
Table 9 presents the regression results from these endogeneity tests. Panel (1) of the table displays the outcomes using the spatial proximity matrix. Given that reliance on a single spatial weight matrix could bias the results, an adjacency matrix is used as an alternative in Panel (2) for comparative analysis. This dual approach enhances the credibility of the findings by demonstrating consistency across different spatial specifications.
Panel (3) of Table 9 reports the results after applying a one-stage lag to the Digital economy variable. By using lagged data, the analysis aims to reduce simultaneity bias, thereby providing a more accurate estimation of Digital economy’s impact on urban green development.
Panels (4) and (5) present results from further robustness tests. Panel (4) excludes data from the year 2022, possibly to account for any atypical conditions or events that occurred in that year which could distort the results. Panel (5) employs a Spatial Difference-in-Differences (DID) model, which is a powerful method for assessing the causal impact of policies or interventions over time and across different regions.
To validate the assumption conditions of the spatial Difference - in - Differences model regarding China’s “Broadband China” policy, it is imperative to conduct a parallel trends test. This is due to the fact that the DID method is founded upon a core assumption that, in the absence of policy implementation, the trends of the treatment group and the control group would have evolved in an identical manner. Should this assumption prove invalid, the results of the DID estimation may be skewed, as it becomes infeasible to clearly distinguish the actual impact engendered by the policy from the alterations caused by other factors.
Moreover, the parallel trends test furnishes empirical evidence for the study, suggesting that prior to policy implementation, the disparity in urban green development between the treatment group and the control group was nearly negligible. The outcomes of the parallel trends test are presented in Figure 6. This figure depicts the variations in changes between the treatment group and the control group before and after the implementation of the “Broadband China” policy. As is discernible from the figure, prior to policy implementation, the difference in the level of urban green development between the two groups was scarcely perceptible, indicating that the trends of the two groups were analogous at the baseline, fulfilling the pre - requisite of the parallel trends assumption requisite for DID analysis. Subsequent to policy implementation, particularly commencing from the second year, this difference attained statistical significance, and this alteration is consonant with the anticipated impact of the policy. In this fashion, we can more cogently demonstrate the applicability of the spatial DID model in assessing the effects of the “Broadband China” policy.
Overall, the results from these robustness tests indicate that the key variables of interest, EG and Interact, remain statistically significant at the 10% level, and the spatial autoregressive parameter λ is significantly positive at the 1% level. These findings suggest that the initial conclusions hold even when accounting for endogeneity concerns, thereby reinforcing the reliability and robustness of the study’s results.
This study aims to bridge critical gaps in understanding the synergistic effects of digital economy and environmental governance in promoting urban green development. Unlike previous research primarily focusing on the impacts of digital economic growth and independent environmental policies, this study integrates these two factors into a spatial framework, providing a more comprehensive perspective. We utilized a spatial error model to reflect interdependencies among cities, thereby deepening our understanding of how digital technological advancements and management strategies jointly contribute to green urban innovation. Furthermore, this study introduces a new set of indicators based on Chinese environmental quality geographic data, which not only broadens the dimensions for measuring urban green development but also offers new insights for academia in assessing urban sustainability, emphasizing the importance of digital infrastructure and environmental governance as drivers of ecological innovation.
In light of the latent impetus that the digital economy can offer for the sustainable development of cities, we propose that policymakers accord top priority to fortifying digital infrastructure construction, including high - speed internet and data centers. Concurrently, environmental governance should be incorporated as a core consideration into the digital economy’s development trajectory. Specifically, through formulating and implementing well - targeted environmental protection regulations, spurring green urban innovation, and facilitating cross - departmental data sharing, it is imperative to ensure that the digital economy’s development is congruent with green objectives. Moreover, green financial incentives, such as green bonds and carbon trading markets, ought to be introduced to proffer financial backing for environmental protection initiatives and green enterprises, thereby enhancing their market competitiveness. For cities boasting a robust digital foundation yet relatively feeble environmental policies, the existing environmental governance system should be either established or optimized. Digital technologies like big data analysis and the Internet of Things should be harnessed to augment the efficiency of environmental monitoring and resource management. The smart city framework should be adopted to integrate diverse service and information platforms, thus enhancing the efficiency and responsiveness of urban management and services. Pilot programs should be encouraged to explore the optimal integration model of digital technologies and environmental governance. Drawing upon these practical experiences, more extensive adjustments to urban development strategies can be guided. Ultimately, it can assist urban administrators in devising urban development pathways that are both sustainable and resource - efficient. This all - encompassing approach of leveraging green financial incentives and the smart city framework can not only expedite the attainment of urban sustainable development goals but also catalyze more innovation and cooperation prospects.
Alleviating Redundancy: Notwithstanding the invaluable contributions of this study, it is not devoid of certain limitations, which, in turn, pave the way for a wealth of opportunities for subsequent research. Firstly, while the study employed specific environmental quality data from China to devise novel indicators for urban green development, the applicability of these research findings in other countries or regions is circumscribed owing to the substantial disparities in economic and environmental conditions among different countries or regions. To address this lacuna in cross - national generalizability, we propose that subsequent research endeavors formulate a well - defined agenda. For instance, analogous models can be tested within diverse geographical and institutional frameworks to validate the universality of these research findings. Secondly, this study centers on macro - level urban statistical data, which might inadvertently veil the intricate micro - level interactions within individual industries or corporate entities. Future research can undertake industry - specific analyses to elucidate how digital transformation and environmental management propel green urban innovation at the organizational level. Finally, this study has not probed into the potential long - term ramifications of emerging digital technologies such as artificial intelligence and blockchain on urban sustainable development. Researchers are urged to explore the impacts of these technologies in future work, as they are anticipated to unearth new aspects of the evolving interplay between digitalization and ecological protection. By meticulously addressing these research gaps and presenting specific research agendas, future research can build upon the groundwork laid in this paper and attain a more profound comprehension of the role of the digital economy and governance in fostering urban sustainable development in various contexts.
The original contributions presented in the study are included in the article/supplementary material, further inquiries can be directed to the corresponding author.
RY: Conceptualization, Data curation, Writing – original draft, Writing – review and editing.
The author(s) declare that no financial support was received for the research and/or publication of this article.
The authors declare that the research was conducted in the absence of any commercial or financial relationships that could be construed as a potential conflict of interest.
The author(s) declare that no Generative AI was used in the creation of this manuscript.
All claims expressed in this article are solely those of the authors and do not necessarily represent those of their affiliated organizations, or those of the publisher, the editors and the reviewers. Any product that may be evaluated in this article, or claim that may be made by its manufacturer, is not guaranteed or endorsed by the publisher.
Abbass, K., Qasim, M. Z., Song, H. M., Murshed, M., Mahmood, H., and Younis, I. (2022). A review of the global climate change impacts, adaptation, and sustainable mitigation measures. Environ. Sci. Pollut. Res. 29, 42539–42559. doi:10.1007/s11356-022-19718-6
Baoping, R., and Peiwei, L. (2022). The mechanism and path for digital economy to foster new drivers for high-quality economic development in China. Front. Econ. China 17 (4). doi:10.3868/s060-015-022-0028-4
Begum, H., Abbas, K., Alam, A., Song, H. M., Chowdhury, M. T., and Ghani, A. B. A. (2022). Impact of the COVID-19 pandemic on the environment and socioeconomic viability: a sustainable production chain alternative. Foresight 24, 456–475. doi:10.1108/fs-02-2021-0053
Benkhodja, M. T., Fromentin, V., and Ma, X. F. (2023). Macroeconomic effects of green subsidies. J. Clean. Prod. 410, 137166. doi:10.1016/j.jclepro.2023.137166
Chen, H. X., Yang, Y. P., Yang, M. T., and Huang, H. (2022). The impact of environmental regulation on China's industrial green development and its heterogeneity. Front. Ecol. Evol. 10. doi:10.3389/fevo.2022.967550
Dalal, D. K., and Zickar, M. J. (2012). Some common myths about centering predictor variables in moderated multiple regression and polynomial regression. Organ. Res. Methods 15, 339–362. doi:10.1177/1094428111430540
Dubé, J., Legros, D., Thériault, M., and Rosiers, F. D. (2014). A spatial Difference-in-Differences estimator to evaluate the effect of change in public mass transit systems on house prices. Transp. Res. Part B-Methodological 64, 24–40. doi:10.1016/j.trb.2014.02.007
Fairchild, A. J., and McQuillin, S. D. (2010). Evaluating mediation and moderation effects in school psychology: a presentation of methods and review of current practice. J. Sch. Psychol. 48, 53–84. doi:10.1016/j.jsp.2009.09.001
Gan, C., Yu, J. L., Zhao, W. S., and Fan, Y. (2023). Big data industry development and carbon dioxide emissions: a quasi-natural experiment. J. Clean. Prod. 422, 138590. doi:10.1016/j.jclepro.2023.138590
Gomes, S., and Lopes, J. M. (2024). Unlocking the potential of circular consumption: the influence of circular habits, environmental concerns and the search for pro-sustainable information on circular consumption. Bus. Strategy Dev. 7. doi:10.1002/bsd2.327
Hao, F., Zang, Y., Fan, B. K., and Zhang, Y. (2023). Environmental regulation promotes green development in China: from the perspective of technological innovation. Front. Energy Res. 11. doi:10.3389/fenrg.2023.1209650
He, W. W., He, S. L., and Hou, H. L. (2024). Digital economy, technological innovation, and sustainable development. Plos One 19, e0305520. doi:10.1371/journal.pone.0305520
Huang, L., Zhang, H. F., Si, H. Y., and Wang, H. Y. (2023). Can the digital economy promote urban green economic efficiency? Evidence from 273 cities in China. Ecol. Indic. 155, 110977. doi:10.1016/j.ecolind.2023.110977
Jiang, Z. S., Xu, C. H., and Zhou, J. (2023). Government environmental protection subsidies, environmental tax collection, and green innovation: evidence from listed enterprises in China. Environ. Sci. Pollut. Res. 30, 4627–4641. doi:10.1007/s11356-022-22538-3
Jin, P. Z., Peng, C., and Song, M. L. (2019). Macroeconomic uncertainty, high-level innovation, and urban green development performance in China. China Econ. Rev. 55, 1–18. doi:10.1016/j.chieco.2019.02.008
Jin, W., Gao, S. H., and Pan, S. F. (2023). Research on the impact mechanism of environmental regulation on green total factor productivity from the perspective of innovative human capital. Environ. Sci. Pollut. Res. 30, 352–370. doi:10.1007/s11356-022-22120-x
Kong, F. H., Yin, H. W., Nakagoshi, N., and Zong, Y. G. (2010). Urban green space network development for biodiversity conservation: identification based on graph theory and gravity modeling. Landsc. Urban Plan. 95, 16–27. doi:10.1016/j.landurbplan.2009.11.001
Li, L. H., and Wang, H. M. (2023). Influence of green investment on China's sustainable development. Sustainability 15, 9804. doi:10.3390/su15129804
Li, X. Y., Liu, J., and Ni, P. J. (2021). The impact of the digital economy on CO2 emissions: a theoretical and empirical analysis. Sustainability 13, 7267. doi:10.3390/su13137267
Liu, J., Tang, J., Zhou, B., and Liang, Z. (2018). The effect of governance quality on economic growth: based on China’s provincial panel data. Economies 6 (4), 56–23. doi:10.3390/economies6040056
Liu, Z., Tang, Y., Wilson, J., Tao, X. L., Lv, B. J., Wang, Z. Y., et al. (2023). Influence of government attention on environmental quality: an analysis of 30 provinces in China. Environ. Impact Assess. Rev. 100, 107084. doi:10.1016/j.eiar.2023.107084
Lopes, J. M., Pinho, M., and Gomes, S. (2023). Green to gold: consumer circular choices may boost circular business models. Environ. Dev. Sustain. doi:10.1007/s10668-023-03930-6
Lu, M., Li, D. X., Peng, W., and Ma, R. Q. (2023). Heterogeneous environmental regulation tools and green economy development: evidence from China. Environ. Res. Commun. 5, 015007. doi:10.1088/2515-7620/acb1f9
Lu, Z. N., Chen, H. Y., Hao, Y., Wang, J. Y., Song, X. J., and Mok, T. M. (2017). The dynamic relationship between environmental pollution, economic development and public health: evidence from China. J. Clean. Prod. 166, 134–147. doi:10.1016/j.jclepro.2017.08.010
Luo, S. Y., Yimamu, N., Li, Y. R., Wu, H. T., Irfan, M., and Hao, Y. (2023). Digitalization and sustainable development: how could digital economy development improve green innovation in China? Bus. Strategy Environ. 32, 1847–1871. doi:10.1002/bse.3223
Lyu, Y., Zhang, L. F., and Wang, D. (2023). Does digital economy development reduce carbon emission intensity? Front. Ecol. Evol. 11. doi:10.3389/fevo.2023.1176388
Madaleno, M., Dogan, E., and Taskin, D. (2022). A step forward on sustainability: the nexus of environmental responsibility, green technology, clean energy and green finance. Energy Econ. 109, 105945. doi:10.1016/j.eneco.2022.105945
Nie, C. F., Zhong, Z. H., and Feng, Y. (2023). Can digital infrastructure induce urban green innovation? New insights from China. Clean Technol. Environ. Policy 25, 3419–3436. doi:10.1007/s10098-023-02605-0
Ogiemwonyi, O., Bin Harun, A., Alam, M. N., and Othman, B. A. (2020). Do we care about going green? Measuring the effect of green environmental awareness, green product value and environmental attitude on green culture. An insight from Nigeria. Environ. Clim. Technol. 24, 254–274. doi:10.2478/rtuect-2020-0015
Pera, A. (2020). Assessing sustainability behavior and environmental performance of urban systems: a systematic review. Sustainability 12, 7164. doi:10.3390/su12177164
Salahuddin, M., and Alam, K. (2015). Internet usage, electricity consumption and economic growth in Australia: a time series evidence. Telematics Inf. 32, 862–878. doi:10.1016/j.tele.2015.04.011
Shen, F., Liu, B., Luo, F., Wu, C. C., Chen, H., and Wei, W. D. (2021). The effect of economic growth target constraints on green technology innovation. J. Environ. Manag. 292, 112765. doi:10.1016/j.jenvman.2021.112765
Shi, Y. F., Zhang, T. L., and Jiang, Y. F. (2023). Digital economy, technological innovation and urban resilience. Sustainability 15, 9250. doi:10.3390/su15129250
Si, H. C., Tian, Z., Guo, C. Y., and Zhang, J. (2024). The driving effect of digital economy on green transformation of manufacturing. Energy and Environ. 35, 2636–2656. doi:10.1177/0958305x231155494
Solarin, S. A., Shahbaz, M., Khan, H. N., and Bin Razali, R. (2021). ICT, financial development, economic growth and electricity consumption: new evidence from Malaysia. Glob. Bus. Rev. 22, 941–962. doi:10.1177/0972150918816899
Tobler, W. R. (1970). A computer movie simulating urban growth in the Detroit region. Econ. Geogr. 46, 234–240. doi:10.2307/143141
Wan, Q. Q., and Shi, D. Q. (2022). Smarter and cleaner: the digital economy and environmental pollution. China and World Econ. 30, 59–85. doi:10.1111/cwe.12446
Wang, L., Dilanchiev, A., and Haseeb, M. (2022). The environmental regulation and policy assessment effect on the road to green recovery transformation. Econ. Analysis Policy 76, 914–929. doi:10.1016/j.eap.2022.10.006
Wang, L. P., Long, Y., and Li, C. (2022). Research on the impact mechanism of heterogeneous environmental regulation on enterprise green technology innovation. J. Environ. Manag. 322, 116127. doi:10.1016/j.jenvman.2022.116127
Wang, M., Xu, M., and Ma, S. J. (2022). Corrigendum to ’The effect of the spatial heterogeneity of human capital structure on regional green total factor productivity’ [Structural Change and Economic Dynamics 59 (2021) 427–441]. Struct. Change Econ. Dyn. 61, 463–466. doi:10.1016/j.strueco.2022.02.006
Wang, Y. L., Zhao, N., Lei, X. D., and Long, R. Y. (2021). Green finance innovation and regional green development. Sustainability 13, 8230. doi:10.3390/su13158230
Wei, Y. G., Huang, C., Lam, P. T. I., and Yuan, Z. Y. (2015). Sustainable urban development: a review on urban carrying capacity assessment. Habitat Int. 46, 64–71. doi:10.1016/j.habitatint.2014.10.015
Wu, H. T., Hao, Y., and Ren, S. Y. (2020). How do environmental regulation and environmental decentralization affect green total factor energy efficiency: evidence from China. Energy Econ. 91, 104880. doi:10.1016/j.eneco.2020.104880
Wu, M. W., Wu, J. Q., and Zang, C. F. (2021). A comprehensive evaluation of the eco-carrying capacity and green economy in the Guangdong-Hong Kong-Macao Greater Bay Area, China. J. Clean. Prod. 281, 124945. doi:10.1016/j.jclepro.2020.124945
Xu, D., Yang, F., Yu, Y., Zhou, Y. Y., Li, H. X., Ma, J. J., et al. (2021). Quantization of the coupling mechanism between eco-environmental quality and urbanization from multisource remote sensing data. J. Clean. Prod. 321, 128948. doi:10.1016/j.jclepro.2021.128948
Yang, L., Wang, K. L., and Geng, J. C. (2018). China's regional ecological energy efficiency and energy saving and pollution abatement potentials: an empirical analysis using epsilon-based measure model. J. Clean. Prod. 194, 300–308. doi:10.1016/j.jclepro.2018.05.129
Yang, Y. C., Nie, P. Y., and Huang, J. B. (2020). The optimal strategies for clean technology to advance green transition. Sci. Total Environ. 716, 134439. doi:10.1016/j.scitotenv.2019.134439
Yu, X., Xu, Y. D., Zhang, J., and Sun, Y. (2022). The synergy green innovation effect of green innovation subsidies and carbon taxes. Sustainability 14. doi:10.3390/su14063453
Zameer, H., and Yasmeen, H. (2022). Green innovation and environmental awareness driven green purchase intentions. Mark. Intell. and Plan. 40, 624–638. doi:10.1108/mip-12-2021-0457
Zhan, L., Guo, P., and Pan, G. Q. (2023). The effect of mandatory environmental regulation on green development efficiency: evidence from China. Environ. Sci. Pollut. Res. 30, 9782–9792. doi:10.1007/s11356-022-22815-1
Zhao, C. Y., Liu, Z. Q., and Yan, X. F. (2023). Does the digital economy increase green TFP in cities? Int. J. Environ. Res. Public Health 20, 1442. doi:10.3390/ijerph20021442
Zhao, J., Zhao, Z. R., and Zhang, H. (2021). The impact of growth, energy and financial development on environmental pollution in China: new evidence from a spatial econometric analysis. Energy Econ. 93, 104506. doi:10.1016/j.eneco.2019.104506
Zhou, L., Zhang, S. N., Zhou, C. J., Yuan, S., Jiang, H., and Wang, Y. F. (2024). The impact of the digital economy on high-quality agricultural development--Based on the regulatory effects of financial development. Plos One 19, e0293538. doi:10.1371/journal.pone.0293538
Keywords: digital economy, urban green development, environment governance, moderation effects, spatial error model
Citation: Yuan R (2025) The synergistic effect of digital economy and environmental governance on urban green development. Front. Environ. Sci. 13:1506794. doi: 10.3389/fenvs.2025.1506794
Received: 06 October 2024; Accepted: 18 March 2025;
Published: 31 March 2025.
Edited by:
Dillip Das, University of KwaZulu-Natal, South AfricaReviewed by:
Irina Georgescu, Bucharest Academy of Economic Studies, RomaniaCopyright © 2025 Yuan. This is an open-access article distributed under the terms of the Creative Commons Attribution License (CC BY). The use, distribution or reproduction in other forums is permitted, provided the original author(s) and the copyright owner(s) are credited and that the original publication in this journal is cited, in accordance with accepted academic practice. No use, distribution or reproduction is permitted which does not comply with these terms.
*Correspondence: Rongze Yuan, YmlkNTk0MkAxNjMuY29t
Disclaimer: All claims expressed in this article are solely those of the authors and do not necessarily represent those of their affiliated organizations, or those of the publisher, the editors and the reviewers. Any product that may be evaluated in this article or claim that may be made by its manufacturer is not guaranteed or endorsed by the publisher.
Research integrity at Frontiers
Learn more about the work of our research integrity team to safeguard the quality of each article we publish.