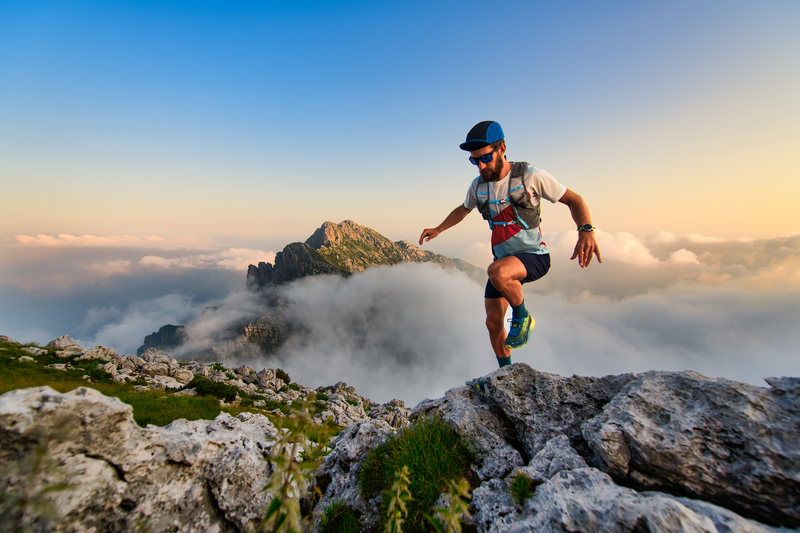
95% of researchers rate our articles as excellent or good
Learn more about the work of our research integrity team to safeguard the quality of each article we publish.
Find out more
ORIGINAL RESEARCH article
Front. Environ. Sci. , 24 January 2025
Sec. Environmental Economics and Management
Volume 12 - 2024 | https://doi.org/10.3389/fenvs.2024.1526681
This article is part of the Research Topic Climate Risk and Green and Low-Carbon Transformation: Economic Impact and Policy Response View all 18 articles
In line with the Sustainable Development Goals (SDGs) proposed by the United Nations and the general direction of global green and low-carbon transformation, China has formulated a dual-carbon target. It aims to peak carbon dioxide emissions by 2030 and strive for carbon neutrality by 2060. Therefore, carbon emission intensity (CEI) has gradually become a research focus. This study investigates the effect of ESG performance on CEI, and explores the moderating effect of climate policy uncertainty (CPU) and the digital economy (DE) on ESG performance and CEI. Provincial-level data in China from 2011 to 2020 was used under the panel data analysis method. The analysis reveals that ESG performance significantly alleviates CEI. In addition, CPU weakens the efficiency of ESG initiatives in decreasing CEI due to regulatory unpredictability, while the digital economy’s expansion, although innovative, may exacerbate CEI in regions dependent on fossil fuels. The study contributes to the literature by highlighting the need for integrated policies that harmonize digital growth with sustainability goals. Additionally, it underscores the significance of considering CPU and DE as critical moderating factors in ESG-CEI dynamics, offering insights for policymakers aiming to balance technological advancement with environmental responsibility.
In the global pursuit of sustainable development, the role of Environmental, Social, and Governance (ESG) performance has become increasingly critical (Keeley et al., 2022; Li et al., 2024), especially in the context of mitigating climate change and promoting sustainable communities as outlined in the United Nations Sustainable Development Goals (SDGs), specifically SDG 11. SDG 11 aims to make cities and human settlements inclusive, safe, resilient, and sustainable, underscoring the importance of reducing carbon emissions and enhancing the quality of life through responsible governance and environmental stewardship (Akuraju et al., 2020). The actions of China, the largest carbon emitter, are crucial for the success of climate change mitigation in light of the globalization of the world’s economy. Despite the significant progress China has made in reducing carbon emission intensity (CEI), there is a growing urgency for absolute reductions (James and Menzies, 2022). To attain the cohesion of continuous economic advancement and reduction in carbon emissions, China, as a developing nation deems it an inherent necessity to achieve SDG 11 (Liu et al., 2024).
Despite the growing emphasis on ESG performance as a driver of low-carbon development, critical gaps remain in understanding the mechanisms through which ESG initiatives influence CEI across varying policy and technological landscapes (Su and Xue, 2023). ESG advocates green development concepts such as environmental protection, social responsibility and scientific governance, in line with current low-carbon development goals. Previous studies have shown that high ESG performance is conducive to promoting firm green transformation and upgrading, thus contributing to low-carbon development (Chen G. et al., 2022; Persakis, 2023). On the one hand, external capital markets prioritize the environmental performance of listed firms and are more inclined to invest in firms with long-term growth potential. To prevent losing access to resources due to weak ESG performance, management will proactively adopt green production practices to decrease CEI (Kong et al., 2024). On the other hand, as the public promotes eco-friendly and sustainable consumption, consumers are increasingly interested in purchasing products that are environmentally friendly and recyclable (Shu and Tan, 2023). Firms that excel in ESG performance are more likely to succeed in market competition, motivating them to implement eco-friendly initiatives and offer sustainable products and services, ultimately leading to a decline in CEI (Ren et al., 2024; Guo et al., 2023). Figure 1 illustrates the average trends in ESG scores and CEI at the provincial level from 2011 to 2020, showing a steady improvement in ESG performance accompanied by declining CEI. Over time, ESG performance demonstrates a consistent upward trajectory, whereas CEI displays a contrary direction.
Figure 1. ESG scores and CEI in China.1
ESG performance serves as a comprehensive measure of corporate responsibility, encompassing environmental protection, social equity, and robust governance practices. These factors are crucial in the fight against climate change, particularly in reducing carbon emissions, which are a significant contributor to global warming (Zakari et al., 2022; Christensen et al., 2021). However, the effectiveness of ESG initiatives in achieving lower carbon emissions is not uniform across all regions and is influenced by various external factors. Two key moderating factors that have garnered attention in recent years are the climate policy uncertainty (CPU) and digital economy (DE) (Zhong et al., 2022). CPU, which arises from inconsistent government regulations, shifting international agreements, and economic fluctuations, poses significant challenges to the successful implementation of ESG strategies (Ma et al., 2023a). When different regions face uncertainty in climate policies, their ability to invest in long-term sustainable practices may be compromised, leading to a potential increase in carbon emissions despite strong ESG performance (Dubash, 2020). Conversely, DE driven by the rapid advancement of digital technologies and data analytics, offers new opportunities for regional to innovate and optimize resource use, potentially enhancing the impact of ESG practices on carbon reduction (Wang and Shao, 2023). Digital technologies can improve efficiency, reduce waste, and enable more transparent and accountable governance, all of which are vital for achieving the targets set by SDG 11. By exploring the interactive dynamics among ESG performance, CPU, and DE, this study provides new insights into how digitalization and policy environments influence corporate sustainability efforts. This study makes a novel contribution by bridging these research gaps and examining the moderating roles of CPU and DE in the relationship between ESG performance and CEI. The findings offer theoretical and practical contributions by extending the understanding of ESG’s role in carbon reduction and by demonstrating how leveraging digital technologies while managing policy risks can accelerate low-carbon transitions. These insights are critical for policymakers and business leaders aiming to design effective strategies that align with SDG 11 and broader climate goals.
This study contributes to the understanding of how digitalization and policy environments interact with corporate sustainability efforts, particularly in the context of China’s regional disparities. The research evaluates the ESG score of listed companies to obtain the provincial ESG index, and investigate the impact of ESG performance on regional CEI. On this basis, the role of CPU and DE in the relationship between ESG performance and CEI is further investigated. Using provincial-level data from China, this research constructs an ESG index based on the performance of listed companies, evaluates its impact on regional CEI, and investigates how CPU and DE shape this relationship. Unlike prior studies, this research is among the first to integrate CPU and DE as moderating factors, addressing the dual challenges of policy uncertainty and technological innovation in the low-carbon transition. The findings will provide valuable insights for policymakers and business leaders, helping them design strategies that leverage the digital economy while managing policy risks to achieve the carbon reduction goals essential for sustainable communities.
Regional development plays a crucial role in attaining the dual-carbon objective, and China’s accomplishment of reaching the highest level of carbon emissions will contribute to advancing sustainable development, aligning with the United Nation proposed SDG11. ESG performance, a novel assessment tool for measuring enterprise development, covers three key dimensions: environment, social responsibility, and governance. It complies well with the principles of environmentally friendly and sustainable development worldwide and serves as an effective means to achieve China’s goals for green development. As the concept of ESG has gained popularity, it has been widely investigated, implemented, and advocated in several fields, attracting the attention of scholars worldwide. They focus on ESG and globally responsible institutional investment (Matos, 2020; Avramov et al., 2022), the impact of ESG scores on the measurement of corporate sustainability performance (Chen H. et al., 2023), and the importance and role decision-making process of ESG factors in finance (Ziolo et al., 2019). Regarding the relationship between ESG performance and regional CEI, few scholars have studied it (Li T. T. et al., 2021).
By examining the fundamental principles of Sustainable Development Theory and Economic Externalities Theory, this exploratory approach can assist in addressing the issue of environmental pollution and ESG performance amid rapid regional economic growth. The United Nations Summit on Sustainable Development took place in New York in 2015. During the summit, member states officially accepted 17 SDGs, aiming to move towards sustainable development over the next 15 years by comprehensively addressing the social, economic, and environmental aspects of development in a coordinated manner. Sustainable Development Theory has had a profound impact on ESG (Chen H.-M. et al., 2022). In the context of carbon neutrality, greater demands have been placed on reconciling economic development and carbon emissions, and Sustainable Development Theory has been extended to develop a framework for an inclusive ‘environment-society-governance’ relationship. Dual-carbon targets are essential for achieving sustainable development. China is currently in a new phase of constructing its ecological civilization, with every region engaged towards achieving a harmonious balance between reducing pollution and reducing carbon emissions (Luo and Tang, 2022). The dual-carbon initiative is a significant strategic choice made by China, driven by its responsibility to advance the establishment of a global community and the essential need to achieve sustainable development.
Economic Externalities Theory provides valuable insights into the concept of ESG. Firstly, as public resources, ecological and environmental issues necessitate government intervention through administrative or market mechanisms. Second, to ensure the completeness of the information given, ESG reports should include both positive and negative externality actions of an enterprise. Finally, for ESG reports to fully and accurately disclose carbon emissions data, it is a prerequisite that the spatial extent of environmental externalities is clearly defined (Edmans, 2023). The increase in carbon emissions has evolved into a matter of global concern in terms of governance, since the issue of carbon emissions has become widely recognized and acknowledged by countries in the ongoing discussion, leading to worldwide efforts towards environmental sustainability (Azam et al., 2022). The carbon emission reduction activities, formulated based on the concept of economic externalities, serves as a method of governance (Persakis, 2023). An effective technique currently being implemented is a governance mechanism that operates from the bottom-up and is devoted to ESG principles in responsible investing. This mechanism has the potential to strengthen the advancement of a low-carbon economy.
Existing research suggests that strong ESG performance implies that firms are aware of sustainability, are actively engaged in practical actions at the ESG level and are achieving fruitful benefits at this stage (Qian and Liu, 2024). Firms, as major participants in the economic market, play a main part in low-carbon development. In terms of the environment, enterprises will aim to decrease waste emissions, specifically nitrogen oxides and greenhouse gases, in order to enhance their ESG performance (Shu and Tan, 2023). This action will result in a reduction of their own carbon emissions, which will subsequently impact the carbon emissions of the surrounding area. An important prerequisite for reducing emissions is energy conservation, and firms need to enhance energy efficiency, diminish energy consumption and cultivate cleaner energy sources. This will facilitate its own transition to environmentally friendly and low-carbon practices, enhance green technology advancements, and thus decrease the corporate carbon emissions (Kong et al., 2024). Socially, ESG ratings can enhance employee satisfaction and empower employees to actively fulfil their social responsibilities as employees, resulting in increased economic benefits for firms (Li and Xu, 2024). This, in turn, can provide financial support for energy-saving and emission-reduction initiatives and impact the carbon emissions of the region. In terms of governance, firms should integrate energy saving and emission reduction in business operations as well as all aspects of management (Persakis, 2023), strengthen the compliance management of suppliers in the supply chain, and achieve co-operation with supply chain firms to reduce emissions, thus influencing the regional carbon emission situation. In summary, enhanced ESG performance leads to decreased carbon emissions across all three levels, establishing the basis for carbon reduction and sustainable progress in the firm’s operating region. Thus, the subsequent hypothesis is established.
H1:. ESG performance of firms in the region dampens regional CEI.
Climate change is a worldwide problem that concerns the entire international community. Climate change has grown increasingly noticeable in recent years, as seen by the frequent occurrence of extreme weather events. Severe weather anomalies serve as factors that exacerbate threats, promoting us to prioritize low-carbon transition and sustainable development. Under the advocacy of the SDGs, it has become a mainstream in the world today for countries to join hands to address climate change and jointly promote green and low-carbon development. The CPU exposes carbon risks that are inherent to the climate. Climate policy primarily aims to address carbon emissions, and its main focus should be assisting organizations in identifying strategies to decrease their carbon emissions (He et al., 2024). Despite the public’s acknowledgement of the significance of climate policy in a low-carbon economy and sustainable development, there is a scarcity of literature on CEI and CPU in current research. Furthermore, this study is the initial one to suggest the CPU as a moderator on the association between ESG and CEI.
Weather problems and climate policies are deeply involved in the formation of low-carbon markets, directly affecting the current state of traditional business and the prospects for green markets. Moreover, increasing concern of global warming is closely connected to the ambiguity caused by drastic climate measures (Ma et al., 2023a). Based on Efficient Market Theory, when facing an increase in CPU, business operators and investors will maintain rational choices to avoid the impact of climate policy risks. Consequently, corporate managers are expected to modify their portfolio strategies, leading businesses to enhance their industrial structure, increase the efficiency of green resource utilization (Ren et al., 2023), and decrease their own carbon emissions, thereby affecting the regional CEI. In this process, the CPU will realize a moderating influence on firm ESG performance and the regional CEI. In particular, the presence of uncertain climate policies has an impact on the interest and attitude of investors towards green markets when there is a growth in CPU, which leads corporate managers to place more emphasis on the utilization of green and clean energy (Adams et al., 2020). Driving operational decisions for firms and their supply chains in terms of R&D investment, energy allocation, and green technology innovation (Liang et al., 2022). Development trend will promote an increase in the government’s attempts to regulate the environment, thereby compelling firms to enhance the beneficial impact of the transition towards environmental practices. It will relieve pressure of environment and contribute significantly to the region’s carbon reduction activities and the achievement of sustainable development.
To summarize, climate policies are suggested as a means to mitigate the hazards associated with climate change. The CPU is a primary contributor to greenhouse gas emissions and acts an indispensable role in improving corporate energy efficiency and promoting green transformation (Ren et al., 2022). Additionally, it serves as an essential bridge in aligning corporate ESG with regional CEI. Studying the impact of CPU on the relationship between ESG and CEI helps enterprises and regions to successfully complete the green development goals of low-carbon transition and carbon neutrality. Therefore, the second hypothesis is proposed.
H2:. CPU plays a significant moderating role between ESG and CEI.
The digital economy, which encompasses digital infrastructure, digital industry development, and digital financial inclusion, plays a critical role in shaping economic and environmental outcomes. Digital infrastructure includes essential technologies such as broadband networks, which facilitate connectivity and access to digital services (Barrett et al., 2023; Di Vaio et al., 2022). Digital industry development focuses on the growth of sectors driven by digital technologies, such as e-commerce and fintech (Afjal et al., 2023). Digital financial inclusion refers to the accessibility of financial services through digital platforms, enabling broader economic participation, particularly in underserved regions (Berry et al., 2018; Johri et al., 2024). These components of the DE significantly influence the relationship between ESG performance and CEI, as they drive both innovation and energy consumption, impacting sustainability outcomes (Sun et al., 2023; Kong et al., 2024). Additionally, DE characterized by digital infrastructure, industry development, and financial inclusion, significantly influences environmental outcomes, particularly within Sustainable Development Theory (Yi et al., 2022). This theory, emphasizing balanced growth, social equity and environmental protection, provides a framework to understand the effect of the digital economy on ESG performance and CEI.
On one hand, DE drives innovation and efficiency, potentially reducing CEI through improved resource management and energy optimization (Gu et al., 2023; Kishor et al., 2024). Digital tools like smart grids and Internet of Things (IOT) devices enable companies to operate more sustainably, aligning with Sustainable Development Theory. However, the expansion of digital infrastructure often leads to increased energy demand, exacerbating carbon emissions, especially in regions relying on fossil fuels (Gu et al., 2023). This paradox is concerning in areas where digital growth outpaces green energy adoption, leading to misalignment between digital expansion and sustainability (Liu et al., 2024). Digital transformation is beneficial for improving the transparency and sharing of enterprise information. On the one hand, it increases external legitimacy pressure, makes it easier to attract media supervision and analyst attention, and increases social supervision power (Chen et al., 2020). Conversely, reducing the level of information asymmetry can help alleviate financing constraints and improve the ESG performance. Additionally, pertinent research indicate that digital transformation can encourage companies to disclose their ESG practices by improving green technology innovation and enhancing the efficiency of decision-making and operational management (Kwilinski et al., 2023; Wang et al., 2023; Guo et al., 2023; Cheng et al., 2024). While digitalization supports sustainability, its benefits depend on renewable energy adoption and sustainable practices. In advanced digital economies without corresponding green energy, digital growth could increase emissions, influence the ESG governance goals (Barrett et al., 2023; Liu et al., 2024). Hence, the third hypothesis is proposed.
H3:. DE plays a significant moderating role between ESG and CEI.
Figure 2 describes the framework of the study.
This study investigates the correlation between ESG and CEI. Additionally, it assesses the impact of CPU and DE on this relationship, hence emphasizing the significance of CPU and DE in enhancing CEI. The research focuses on Chinese provinces and is conducted as follows: (1) Provinces with significant missing data are excluded; (2) missing variables are addressed using the interpolation method. Finally, the data from 30 provinces in China from 2011 to 2020 was selected as the study sample. Because there is a lack of data for Tibet, Taiwan, Hong Kong, and Macau, these regions were excluded from the empirical analysis, resulting in a total of 300 observations. In 2011, China initiated the process of investigating and creating a carbon trading market. This event signified the initiation of regional aviators and the creation of a structure for a nationwide carbon market in China. Therefore, the study period begins in 2011 and ends in 2020 to eliminate the influence of the pandemic period. Considering the development of the ESG and DE assessment systems and CPU, selecting the sample from all provinces in China, which ensured that the data were representative.
The study computed the overall ESG scores of listed businesses in each province as reported by Bloomberg (https://www.bloombergchina.com/solution/sustainable-finance/). Bloomberg, an internationally renowned and esteemed provider of financial information, ensures the accuracy and credibility of the data. The CEI related indicators are obtained from the China Environmental Statistics Yearbook (https://www.mee.gov.cn/hjzl/sthjzk/sthjtjnb/) and the China Energy Statistics Yearbook (https://www.shujuku.org/china-energy-statistical-yearbook.html). China’s provincial CPU index is provided by Figshare Dataset (https://figshare.com/). The data for DE indicators are taken from the Digital Finance Research Centre of Peking University (https://idf.pku.edu.cn/yjcg/zsbg/index.htm), as well as the China Industrial Statistical Yearbook (https://www.shujuku.org/china-industry-statistical-yearbook.html) and China Statistical Yearbook (https://www.stats.gov.cn/sj/ndsj/) from previous years. Data on the control variables were obtained from the National Statistical Office database (https://data.stats.gov.cn/index.htm). Data were collected and processed using mainly SPSS software and EXCEL. Model regression analyses were conducted through Stata software.
To develop a transparent and thorough methodology for evaluating carbon neutrality, the CEI was identified as a representative indicator in this study. The United Nations-supported “Getting to Zero” initiative has been joined by more than 4,500 non-governmental participants from 92 economies around the world, working together to obtain the target of halving carbon emissions by 2030 (Chen G. et al., 2022). China, along with other countries, has established a definitive objective of achieving peak carbon emissions by 2030 and carbon neutrality by 2060 (Cheng et al., 2018; Wang et al., 2020). The Chinese government proposes to strengthen the governance of CEI to better utilise the economic, social and ecological benefits of energy conservation and carbon reduction, which will lay a solid foundation for achieving the goal of carbon neutrality. It emphasizes the essential value of conducting research on CEI. CEI serves as the principal indicator linking the greenhouse gas emissions generated by economic activities. By measuring the quantity of carbon emitted per unit of product or economic value, this approach evaluates both carbon efficiency and the integration of environmental and economic factors (Li Y. et al., 2021). The integration shows the extensive advantages it offers in evaluating low-carbon development, precisely reflecting the advancements made by different regions in attaining their dual-carbon objectives (Dopp, 2016). Therefore, CEI is a dependable and transparent approach that adheres to international standards and best practices for evaluating carbon neutrality.
Drawing on the measurement method of Zhang et al. (2017), the study adopts the IPCC emission factor method to calculate the total carbon emissions from regional energy consumption in each province. Additionally, CEI is determined by dividing the total carbon emissions by the GDP of each province in the same year (Xiong et al., 2016). Specifically, the computation of the CEI involves three primary stages. First, the scope of carbon accounting is established. Regional carbon accounting borders can be categorized in numerous ways based on different criteria. The World Resources Institute initially offered the concept of preventing double counting by defining the boundaries of emission sources in its guidelines for creating business greenhouse gas emission inventories. The boundary definition of carbon accounting in this study draws on this idea and can be divided into three main areas: Scope 1 refers to all direct emissions within a city’s jurisdiction, including mainly greenhouse gas emissions from energy activities within cities and towns, industrial processes, agriculture, land-use change and forestry, and waste disposal activities; Scope 2 encompasses the energy-related emissions that occur outside of metropolitan regions, including primarily emissions from purchased secondary energy sources; Scope 3 covers other indirect emissions caused by activities within the town that occur outside the jurisdiction but are not covered by Scope 2. Secondly, the total carbon emissions are estimated. The total carbon emissions are calculated by summing the carbon emissions within the three ranges, as defined by the carbon accounting scope in the first step. Finally, the carbon intensity per unit of GDP is computed by dividing the total carbon emissions of each Chinese province by its GDP. This indicator reflects the carbon efficiency of economic activity by utilizing GDP data. Specific formulas for the CEI are shown in Equation 1:
where
This study acquired empirical CEI data for 30 Chinese provinces spanning from 2011 to 2020 through the process of these computations. The aforementioned approach aligns with the principle of sustainable development and can accurately evaluate the effectiveness of each province in both reducing carbon emissions and achieving GDP growth. Therefore, it offers concrete evidence and a scientific foundation for regions in the process of achieving their dual-carbon goals.
Within the framework of sustainable development, ESG has progressively garnered public scrutiny. ESG provides a tangible expression of the emerging development idea in the capital market, with a significant and far-reaching influence on the management and functioning of businesses (Tarmuji et al., 2016). ESG advocates the adoption of environmentally friendly development principles, including protecting the environment, fulfilling social responsibilities, and implementing scientific governance, which are in line with the requirements of the current low-carbon development goals. ESG ratings have been shown to be an important measure of corporate ESG performance (Conen and Hartmann, 2019). Rating agencies evaluate a firm’s performance with regards to its environmental impact, social responsibility, and corporate governance by examining disclosed information and third-party data through qualitative and quantitative methods (Vannoni and Ciotti, 2020). ESG ratings for firms were collected from Bloomberg for this research. The Bloomberg ESG Disclosure Score is a rating system launched by Bloomberg to assess the extent of ESG disclosure by publicly traded firms worldwide. The score is calculated based on a firm’s publicly accessible documents, such as annual reports and sustainability reports, evaluating the company’s level of transparency and comprehensiveness in reporting. Figure 3 demonstrates the ESG assessment indicator system.
The research goes further by investigating the relationship between the ESG scores of businesses in different provinces and the CEI of those specific regions. The overall ESG performance score for each province is calculated by averaging the ESG scores of all the companies operating in that province, in line with the aims of this study, which draws on Xie’s (2024) research. This rating enables provinces to understand the strengths and room for improvement of their listed firms in terms of ESG disclosure, and thereby enhance their disclosure policies. The specific calculations are demonstrated in Equation 2:
where
The study employed CPU and DE counts as moderating variables to evaluate their impact on the association between ESG performance and CEI. In 2020, China has proposed the ambitious aim of achieving carbon peaking and carbon neutrality by 2030 in response to the escalating issue of climate change (Li T. T. et al., 2021). Specific goals have been established to promote the dual-carbon purpose and engage in the worldwide management of climate change (Lee and Cho, 2023). Given the externality of the climate problem, governments tend to put in place climate policies to guide the allocation of resources to internalise the cost of the externality. However, climate policy is also characterised by significant uncertainty, as the timing, intensity and details of policies are difficult to fully anticipate by micro agents.
Lee et al. (2022) have observed a significant increase in China’s CPU in recent years. It implies that although China’s existing climate policy has yielded certain favourable outcomes, the frequent changes in policy also create a degree of uncertainty regarding carbon emissions and corporate performance. Given the importance of the topic of climate change and sustainable development, the study introduces the CPU into the research framework of ESG performance and CEI, which is crucial for further exploration. Examining the level of uncertainty around climate policies is equally substantial to analysing the policies themselves. The study adopts the algorithm of Ma et al. (2023a), whose study calculates the CPU index for each province by constructing the MacBERT model.
DE has emerged as the primary driving force behind a fresh wave of technological revolution and industrial transformation. At the recent 2024 Global Digital Economy Conference, the Chinese government aims to capitalize on the potential of DE to advance regional green development. This endeavour holds immense importance in driving China’s economic and social progress, fostering a harmonious coexistence between humans and nature, and achieving comprehensive green transformation, which holds immense practical importance. It highlights the significance of examining the digital economy in economic activities.
The research uses the entropy method to assess the degree of DE advancement in each province. The assessment based on three dimensions are shown in Table 1.
To guarantee the accuracy and effectivity of model estimation outcomes, this research incorporates four additional variables to analyse the impact on the link between CEI and ESG: labour force level, social consumption level, foreign direct investment, and openness level.
Labour force level (LAB): With the rapid aging of the population, China’s labour market is experiencing significant transformations in the current economic landscape (Zhu et al., 2021). Structural imbalances between labour supply and demand are increasingly evident, leading to potential repercussions on economic activities and the ecological environment. This study employs the logarithmic measure to quantify the number of individuals employed in each province.
Social Consumption Level (CON): This indicator reflects the aggregate quantity of consumer products provided to the population and society through different channels of commodity distribution by various industries (Wang et al., 2019). It is a crucial metric that measures the level of economic prosperity. Hence, the ratio of gross social consumption goods to GDP is utilized.
Foreign Direct Investment (FDI): As to the International Monetary Fund (IMF), FDI is when an investor from one country invests funds in another country for production or operation purposes, while also having a certain degree of control over the operation. Studies have shown that FDI can have significant long-term and short-term effects on carbon emissions (Shao, 2018). This study expresses the ratio of total FDI to GDP.
Degree of openness (OPN): Increased regional participation enables the integration of modern foreign technology and development ideals, hence promoting international collaboration in the field of the green economy. Azam et al. (2022) showed that trade openness has an impact on carbon intensity. This dimension is represented by the ratio of total exports and imports of goods to GDP.
The variable descriptions are shown in the Table 2.
This study investigates how ESG performance and CEI are related in Chinese provinces through a two-way fixed effects panel regression model. As shown in Equation 3, the model explains the effects of geographic location and temporal variation by creating province and year fixed effects. The two-way stationary model provides a more precise estimation of the mechanism by which ESG influences CEI.
The study delves deeper into the moderating effect of CPU and DE on the relationship between ESG and CEI. By introducing an interaction term between CPU and DE with ESG, respectively, this study explores how CPU and DE affect the interrelationship between ESG and CEI. Thus, Equations 4 and 5 provide models that demonstrate this moderating effect. It takes into account time-series dependence and data heterogeneity, and emphasises the moderating role of CPU and DE. It offers new perspectives to advance research on CEI and ESG.
where
The outcomes of the descriptive statistics can be seen from Table 3. A total of 300 sets of data are included in the analysis. The variables CEI and ESG demonstrate a higher standard deviation in comparison to the other variables. This implies there is a substantial gap in numbers between ESG performance and CEI within the 30 provinces.
Table 4 presents the correlation coefficient between variables. It appears that there is no significant multicollinearity, considering the correlation coefficient between the key variable is below 0.7. Furthermore, the research hypothesis H1 is supported by a notable negative correlation between ESG performance and CEI (r = −0.302, p < 0.01).
Table 5 reflects Variance Inflation Factor (VIF) values for the key variables. It is evident that the VIF value of all variables is below 5 and the average value of that points to 1.66, reflecting the absence of significant problems related to multicollinearity.
Table 6 demonstrates the regression outcomes. It can be obviously perceived from Table 6 that in the column (1) without control variables, ESG exerts a significant negative impact on CEI (β = −0.172, p < 0.01). Column (2) displays the regression findings with control variables, revealing that the notable negative effect remains evident (β = −0.172, p < 0.01). Likewise, it can be observed from column (3) that the ESG lagged by one period has a notable adverse effect on CEI (β = −0.139, p < 0.01). All of the results above verify hypothesis H1 and indicate a causal connection between ESG performance and CEI.
The relationship between CEI and ESG performance has significant economic implications. It not only reflects the performance of enterprises in addressing environmental challenges and fulfilling social responsibilities, but also has a profound impact on the sustainable development of the economy. Therefore, enterprises and governments should attach great importance to CEI and ESG performance, and take active measures to promote economic development towards a greener and more sustainable direction.
This study delves deeper into how CPU and DE could moderate the connection between ESG performance and CEI, as illustrated in Table 7.
By combining Table 6, it is apparent that both moderation models provide equivalent regression outcomes. More precisely, the research findings are additionally confirmed by the inverse relationship between ESG and CEI (β = −0.316, p < 0.01; β = −0.229, p < 0.01). The moderating model extends the baseline model by incorporating interaction terms between ESG and CPU in the first column, and between ESG and DE in the column (2) of Table 7. Thereunder, they respectively study whether CPU and DE affect the relationship between ESG performance and CEI, along with the direction of their impact.
The research results presented in Table 7 reveal two significant interaction terms (ESG*CPU: β = 0.071, p < 0.01; ESG*DE: β = 0.690, p < 0.01), in sharp contrast to the coefficient direction of ESG performance. The finding suggests that both the CPU and DE are involved in mitigating the influence of ESG performance on CEI, with empirical findings supporting hypotheses H2 and H3. To effectively illustrate the regulatory impact, this study draws two graphs depicting the regulatory effect. As shown in Figure 4, the reduction in CPU leads to an elevation in the slope of ESG and CEI, indicating that CPU hinders the inverse correlation between ESG and CEI. CPU, as an external pressure, drives high carbon emission industries to undergo structural adjustment and transformation and upgrading. Enterprises that cannot effectively respond to policy changes and reduce carbon emissions may gradually be eliminated by the market, while emerging industries and enterprises with lower carbon emissions and good ESG performance will gain more development opportunities, thereby promoting the entire industrial structure to develop towards low-carbon, environmentally friendly, and sustainable directions. Thus, it is inferred that hypothesis H2 receives additional confirmation. Similarly, Figure 4 reflects that reducing DE compared to cities with a higher DE development index will increase the slope of both ESG and CEI. The regulatory effect of DE indicates that high carbon emission industries are recommended to be transformed and upgraded through digital technology, such as using big data analysis to optimize production processes and utilizing artificial intelligence to achieve precise energy management. This results in lower energy usage and reduced carbon emissions, promoting a shift in industrial restructuring towards a more efficient and low-carbon direction, ultimately enhancing overall ESG performance. Thus providing further support for hypothesis H3.
The subsequent robustness test employs two techniques. Taking into account the potential time delay effect of ESG on CEI, the initial robustness study includes integrating CEI data with a lag of one period. Table 8 examines the direct impact of ESG performance on CEI and the moderating effects of CPU and DE. Firstly, the column (1) tests the robustness of the effect of ESG on L. CEI. The results suggest a strong negative relationship between ESG performance and levels of L. CEI (β = −0.618, p < 0.01), providing support for hypothesis H1. Furthermore, the second column of Table 8 illustrates the modifiable functionality of CPU. A statistically significant coefficient of interaction between ESG and CPU (β = 0.069, p < 0.05) is clearly visible. This discovery, contrasted with ESG performance (β = −0.311, p < 0.01), implies that the CPU mitigates the effect of ESG on CEI by acting as a negative regulator in the link between ESG and L. CEI. Similarly,the presence of interaction between ESG and DE is also indicated by a significant coefficient (β = 0.773, p < 0.01) in column (3). And ESG performance and L. CEI remain negative correlations. This implies that the existence of DE mitigates the influence of ESG performance on CEI. The results align closely with the findings presented in Tables 6, 7, indicating that assumptions H2 and H3 have successfully passed the test for robustness.
The adjustment of energy structure (ES) aims to decrease the need for and usage of fossil energy resources, diminish the proportion of coal-generated power, and actively promote the growth of new energy and renewable energy sources. Optimizing the energy structure is a crucial element in guaranteeing energy security and attaining carbon peak neutrality. Accordingly, the second approach to enhance resilience in this research is the incorporation of ES as a control variable, as depicted in Table 9. The regression results of both the baseline model and the moderating model coincide with the original regression. Following the aforementioned investigation, the conclusions of the current study remained steadfast, therefore showcasing the enhanced reliability of the study’s findings.
China has a vast territory, spanning the eastern, central and western regions. Regional heterogeneity in the impact of ESG performance on CEI stem from variations in resource endowment, maturity of market mechanism, and environmental policies across different regions. The research categorizes the 30 provinces within study into three sampling combinations, following the criteria set by the National Bureau of Statistics, which correspond to the three economic zones of Chinese eastern, central and western regions. The ESG performance and CEI by region are displayed in Figures 5A, B.
The regression results in the baseline model in Table 10 reveal how regional ESG performance affects CEI. The data clearly demonstrates that ESG have a substantial inhibitory impact on CEI in all three regions. Furthermore, the significance level of this effect is statistically significant at the 1% level. The suppressive impact was most pronounced in the eastern region (β = −0.272, p < 0.01). This may be traced to the eastern region’s dominant economic position, high market activity and substantial number of listed firms, which exacerbate the marginal impact of ESG performance on regional low-carbon activities.
Table 11 indicates notable geographic variations in how regional CPU and DE influenced the relationship between ESG performance and CEI. Specifically, regarding ESG’s interaction with the CPU, the central region is not significant. Nevertheless, the eastern and western regions had a substantial association at the 1% and 5% significance levels, respectively. CPU are utilized in both eastern region and western region to effectively mitigate the influence of ESG performance on CEI. The variations in the significance of the results perceived among regions can be ascribed to the diverse approaches adopted by climate policies in each region, highlighting distinct effectiveness of these regulations in various places.
Added to that, the relevance of ESG and DE interaction terms additionally reveals heterogeneity throughout various geographic areas. The eastern and central regions exhibited a moderation effect of DE at significance levels of 1% and 5%, respectively. However, the effect of DE regulation in western region is not considerable. This disparity may arise due to the abundance of natural resources and the prevalence of energy-focused sectors in the western region, along with the relatively underdeveloped digital economy and slower overall rate of development compared to the eastern and central regions.
This study provides important insights into the direct relationship between ESG performance and CEI, with a particular insight on the moderating effects of CPU and DE.
This study affirms that ESG performance strongly hinders CEI. This discovery is in line with the increasing amount of literature that highlights the importance of accountability in encouraging sustainable behaviours and lessening environmental damage. Companies that prioritize ESG factors tend to adopt more efficient technologies and practices, thereby lowering their carbon footprint. The study confirms that ESG performance has a significant inhibitory effect on CEI, aligning with the Sustainable Development Theory, which posits that sustainable practices can be a source of core competitive advantage (Ziolo et al., 2019). Firms focusing on ESG are more likely to adopt cleaner technologies and practices that minimize their environmental footprint. The approach not only reduces emissions but also enhances resilience against regulatory risks, lining up with global sustainability goals like the SDGs.
Consequentially, research indicates that provinces with strong ESG performance are better positioned to attract investment, as investors increasingly seek to support environmentally responsible performance (Ong et al., 2019). The investment trend creates a positive feedback loop, where ESG-focused companies can access capital more easily, further enabling them to invest in green technologies and sustainable practices (Kong et al., 2024). The alignment of ESG practices with environmental stewardship reflects a broader shift in the corporate world toward integrating sustainability into core operations, driven by both regulatory pressures and market demand. These findings imply that firms with higher ESG scores typically have lower carbon intensities, reinforcing the importance of integrating ESG into SDG strategies for both environmental and economic benefits (Persakis, 2023).
Moreover, the moderating effect of CPU weakens the impact of ESG on CEI, indicating that inconsistent or unpredictable climate policies can undermine the effectiveness of ESG initiatives. Uncertainty in climate policy creates a challenging environment for regions, which may hesitate to invest in long-term sustainability measures due to doubts about future regulations or support (Ma et al., 2023b). The uncertainty often drives short-term decision-making, prioritizing immediate economic benefits over environmental concerns, thereby exacerbating carbon emission intensity. When climate policy is uncertain, managers and decision makers may adopt a “wait-and-see” approach, delaying investments in green technologies and practices that would otherwise reduce their carbon footprint (Dubash, 2020). The behaviour is particularly prevalent in industries with high exposure to regulatory changes, where the costs of compliance are substantial and long-term investments are risky without clear policy direction (Sarfraz et al., 2022). Therefore, the potential benefits of ESG strategies in mitigating environmental impact are not fully realized, and CEI may remain high or even increase. In essence, the effectiveness of ESG initiatives in reducing CEI is significantly influenced by the stability and clarity of climate policies. Without consistent and predictable regulations, the full potential of ESG practices in driving sustainable outcomes is compromised, highlighting the need for more coherent and supportive policy frameworks to achieve meaningful reductions in CEI.
The study also reveals that the development of DE weakens the relationship between ESG performance and CEI. While DE is associated with innovation and efficiency, it also has the potential to increase energy consumption and carbon emissions, particularly in regions where fossil fuels dominate the energy mix. The expansion of digital infrastructure can lead to increased electricity demand, which, if not met by renewable sources, exacerbates carbon emissions. Moreover, the rapid pace of digitalization often surpasses the implementation of green technologies, creating a disparity between digital growth and environmental sustainability (Li and Wang, 2022). This gap is further widened by the fact that digital innovations frequently prioritize economic gains over environmental considerations, especially in markets where regulatory frameworks are weak or non-existent. As a result, while DE drives economic growth and efficiency, it may inadvertently contribute to environmental degradation if not accompanied by strong ESG commitments and supportive policies that ensure sustainable energy use (Li Y. et al., 2021; Chen S. et al., 2023). To mitigate these effects, it is crucial to integrate sustainability into DE by promoting energy-efficient technologies and encouraging the use of renewable energy sources in digital infrastructure development (Dong et al., 2022). Furthermore, policymakers need to ensure that digitalization efforts are aligned with environmental goals, to reduce the potential negative impact on CEI.
Based on the regional heterogeneity results, ESG performance shows a significant negative impact on CEI. The findings suggest that province with higher ESG scores is generally more effective at reducing their carbon footprint, regardless of regional differences. The interaction term between ESG and CPU is significant in the eastern and western region, indicating that CPU weakens the positive impact of ESG on CEI. In regions where CPU is high, the effectiveness of ESG initiatives is diminished, likely due to the unpredictable regulatory environment, which deters long-term sustainability investments. The lack of sensitivity to climate policy in the western region due to its rich natural resources and less industrial impact. The eastern region’s advanced economy and higher levels of industrialization mean that businesses here are more exposed to regulatory risks, causing them to be more cautious about investing in ESG practices. This caution can result in a reduced impact of ESG efforts on carbon emission reduction, as firms may prioritize immediate economic stability over environmental considerations.
DE also demonstrates a significant moderating effect, particularly in the eastern and central regions. The interaction terms for ESG and DE in these regions display that while the DE could foster innovation (Lyu et al., 2023), it may also lead to increased CEI if not accompanied by a shift towards renewable energy sources. The eastern region, in particular, shows a significant positive interaction between ESG and DE, suggesting that digital advancements might temporarily exacerbate carbon emissions if not managed sustainably (Chen H. et al., 2023). The central region shows a strong positive interaction between DE and carbon emissions, indicating that digitalization might have a more detrimental impact on CEI here compared to other regions. This could be due to a less developed renewable energy infrastructure or higher dependence on traditional energy sources (Zhong et al., 2022).
In conclusion, while ESG performance plays a crucial role in reducing CEI, its effectiveness is moderated by external factors such as CPU and DE. These findings highlight the need for coherent and stable climate policies that can provide the necessary support for ESG initiatives. Furthermore, as the digital economy continues to grow, there is a need for policies that ensure this growth is aligned with sustainability goals. In addition, the results underline the importance of tailoring ESG performance and sustainability strategies to regional contexts, considering the varying impacts of CPU and DE on CEI. Policymakers should aim to reduce regulatory uncertainty and ensure that the growth of DE are still prevalent. Integrating ESG principles into DE and ensuring that digital advancements contribute to rather than detract from sustainability efforts is vital for accomplishing SDG 11.
In summary, the study confirms that ESG performance has a significant inhibitory effect on CEI. This finding aligns with the growing body of literature that emphasizes the role of firm responsibility in promoting sustainable practices and reducing environmental harm. It makes a significant theoretical contribution by reinforcing the Sustainable Development Theory, suggesting that ESG performance acts as a valuable sustainable resource that enhances a regions long-term competitive advantage through sustainability practices. By confirming the inhibitory effect of ESG on CEI, the study provides empirical evidence supporting the idea that managers and decision makers with strong ESG performance are better equipped to manage environmental risks and match global sustainability goals. The finding contributes to the broader discourse on corporate responsibility and environmental stewardship by highlighting the importance of integrating ESG factors into SDG strategies. Practically, the study offers actionable insights for policymakers and business leaders. It emphasizes the need for clear and consistent climate policies to enhance the effectiveness of ESG initiatives in reducing CEI.
The findings related to the interaction between ESG performance and CPU, particularly in the eastern region and western region, have significant implications for achieving the SDG 11, which focuses on sustainable cities and communities. As urban areas in the eastern region are often more industrialized and economically developed, the reduced effectiveness of ESG initiatives due to policy uncertainty poses a challenge to creating sustainable, resilient communities. Ensuring consistent and supportive climate policies is crucial for fostering urban environments that fit SDG 11, promoting sustainability and reducing environmental impacts in these regions.
In addition, this study highlights the significant moderating effect of CPU on the relationship between ESG performance and CEI. The findings suggest that inconsistent or unpredictable climate policies can undermine the effectiveness of ESG initiatives, leading firms to prioritize short-term economic gains over long-term environmental sustainability. This behaviour increases CEI, posing challenges for achieving sustainable development goals. Practically, the study underscores the need for stable and predictable climate policies to maximize the effectiveness of ESG initiatives in reducing CEI. Policymakers should focus on creating a stable regulatory environment that encourages long-term sustainability investments. For businesses, the findings highlight the importance of resilience and adaptability in ESG strategies, particularly in contexts of policy uncertainty. The study enriches the literature by revealing how external factors, like climate policy uncertainty, can disrupt the positive impacts of ESG efforts. This finding broadens the understanding of the conditions under which ESG initiatives are most effective, adding nuance to the relationship between corporate responsibility and environmental outcomes.
This study also finds that DE can weaken the relationship between ESG performance and CEI. Although digitalization drives innovation and efficiency, it can also increase energy consumption, especially in regions dependent on fossil fuels. The rapid growth of digital infrastructure may lead to higher electricity demand and outpace the adoption of green technologies, creating a disparity between digital expansion and environmental sustainability. The study expands the existing literature by examining the complex interplay between DE development and ESG effectiveness in reducing CEI. It introduces a critical perspective on how technological advancement, while beneficial in many ways, can also exacerbate environmental challenges if not managed alongside sustainable practices. The findings highlight the importance of integrating renewable energy sources into digital infrastructure planning to mitigate the potential negative environmental impacts. Policymakers and businesses should consider the environmental trade-offs of digital expansion and ensure that digital growth is aligned with sustainability goals.
Furthermore, the study emphasizes the significance of aligning DE growth with sustainable practices to prevent the unintended environmental consequences of rapid digitalization. These insights are critical for designing strategies that balance economic growth with environmental sustainability, thereby contributing to the achievement of SDGs, particularly SDG 11. The study is limited by its exclusive focus on mainland China, which restricts the generalizability of the findings to other regions with different regulatory environments, economic structures, and levels of digital economy development. The unique socio-economic and policy context in China may result in outcomes that differ from those in other countries, especially where climate policies and digital infrastructure are at different stages of development. Therefore, future studies should consider cross-country comparisons to better understand the global applicability of the findings. Additionally, further research could explore the role of digitalization in ESG strategies across diverse sectors, as well as the impact of emerging technologies such as AI and blockchain on both ESG practices and environmental outcomes. Future research could also investigate how ESG initiatives interact with local-level policy frameworks in different economic contexts and analyze the broader implications of digitalization on corporate sustainability efforts.
The raw data supporting the conclusion of this article will be made available by the authors, without undue reservation.
SL: Writing–original draft, Formal Analysis. XA: Conceptualization, Writing–review and editing. MZ: Methodology, Software, Writing–review and editing. MP: Investigation, Supervision, Writing–review and editing.
The author(s) declare that financial support was received for the research, authorship, and/or publication of this article. This work was supported by: (1) 2022 Anhui Province Social Science Innovation and Development Research Project: Mechanism and Path Research on Inclusive Finance Assisting Rural Revitalization in Anhui Province (2022CX199). (2) General Projects of the 2022 Excellent Youth Talent Support Program in Universities (gxyq2022131). (3) Provincial Quality Engineering Project in 2019—First-class Undergraduate Professional Construction Point—Financial Management (2019ylzy03).
The authors would like to express their gratitude to all those who helped them during the writing of this manuscript. The authors owe their sincere gratitude to friends and colleagues who gave them much enlightening advice and encouragement.
The authors declare that the research was conducted in the absence of any commercial or financial relationships that could be construed as a potential conflict of interest.
The author(s) declare that no Generative AI was used in the creation of this manuscript.
All claims expressed in this article are solely those of the authors and do not necessarily represent those of their affiliated organizations, or those of the publisher, the editors and the reviewers. Any product that may be evaluated in this article, or claim that may be made by its manufacturer, is not guaranteed or endorsed by the publisher.
The Supplementary Material for this article can be found online at: https://www.frontiersin.org/articles/10.3389/fenvs.2024.1526681/full#supplementary-material.
1The author calculated it based on Bloomberg ESG scores (https://www.bloombergchina.com/solution/sustainable-finance/) and the China Statistical Yearbook (https://www.mee.gov.cn/hjzl/sthjzk/sthjtjnb/).
Adams, S., Adedoyin, F., Olaniran, E., and Bekun, F. V. (2020). Energy consumption, economic policy uncertainty and carbon emissions; causality evidence from resource rich economies. Econ. Analysis Policy 68, 179–190. doi:10.1016/j.eap.2020.09.012
Afjal, M., Kathiravan, C., Dana, L. P., and Nagarajan, C. D. (2023). The dynamic impact of financial technology and energy consumption on environmental sustainability. Sustainability. 15 (12), 9327. doi:10.3390/su15129327
Akuraju, V., Pradhan, P., Haase, D., Kropp, J. P., and Rybski, D. (2020). Relating SDG11 indicators and urban scaling – an exploratory study. Sustain. Cities Soc. 52, 101853. doi:10.1016/j.scs.2019.101853
Avramov, D., Cheng, S., Lioui, A., and Tarelli, A. (2022). Sustainable investing with ESG rating uncertainty. J. Financ. Econ. 145 (2), 642–664. doi:10.1016/j.jfineco.2021.09.009
Azam, M., Rehman, Z. U., and Ibrahim, Y. (2022). Causal nexus in industrialization, urbanization, trade openness, and carbon emissions: empirical evidence from OPEC economies. Environ. Dev. Sustain. 24 (12), 13990–14010. doi:10.1007/s10668-021-02019-2
Barrett, S. E., Ochapa, M. O., and Warren, T. (2023). Digital technology in community engagement: impacts and implications. J. Health Care Poor Underserved 34 (1), 425–430. doi:10.1353/hpu.2023.0027
Berry, A., McCabe, C. S., Muir, S., and Walsh, N. (2018). Digital behaviour change interventions to facilitate physical activity in osteoarthritis: a systematic review. Physical Therapy Reviews. 23 (3), 197–206. doi:10.1080/10833196.2018.1470747
Chen, G., Han, J., and Yuan, H. (2022a). Urban digital economy development, enterprise innovation, and ESG performance in China. Front. Environ. Sci. 10. doi:10.3389/fenvs.2022.955055
Chen, H., Hou, N., Zhao, W., Hou, N., and Liu, W. (2023a). The digital economy, industrial structure upgrading, and carbon emission intensity — empirical evidence from China’s provinces. Energy Strategy Rev. 50, 101218. doi:10.1016/j.esr.2023.101218
Chen, H.-M., Kuo, T.-C., and Chen, J.-L. (2022b). Impacts on the ESG and financial performances of companies in the manufacturing industry based on the climate change related risks. J. Clean. Prod. 380 (1), 134951. doi:10.1016/j.jclepro.2022.134951
Chen, S., Song, Y., and Gao, P. (2023b). Environmental, social, and governance (ESG) performance and financial outcomes: analyzing the impact of ESG on financial performance. J. Environ. Manag. 345, 118829. doi:10.1016/j.jenvman.2023.118829
Cheng, S., Liu, W., and Lu, K. (2018). Economic growth effect and optimal carbon emissions under China’s carbon emissions reduction policy: a time substitution DEA approach. Sustainability 10 (5), 1543. doi:10.3390/su10051543
Chen, Y. (2020). Improving market performance in the digital economy. China Economic Review, 62, 101482. doi:10.1016/j.chieco.2020.101482
Cheng, Y., Zeng, B., and Lin, W. (2024). Heterogenous effects of inclusive digital economy and resource distribution mismatch on corporate ESG performance in China. Resour. Policy 92, 104973. doi:10.1016/j.resourpol.2024.104973
Christensen, H. B., Hail, L., and Leuz, C. (2021). Mandatory CSR and sustainability reporting: economic analysis and literature review. Rev. Account. Stud. 26 (3), 1176–1248. doi:10.1007/s11142-021-09609-5
Conen, R., and Hartmann, S. (2019). The hidden risks of ESG conformity - benefiting from the ESG life cycle. SSRN Electron. J. doi:10.2139/ssrn.3426204
Di Vaio, A., Hasan, S., Palladino, R., and Hassan, R. (2022). The transition towards circular economy and waste within accounting and accountability models: a systematic literature review and conceptual framework. Environ. Dev. Sustain. 25 (1), 734–810. doi:10.1007/s10668-021-02078-5
Dong, F., Hu, M., Gao, Y., Liu, Y., Zhu, J., and Pan, Y. (2022). How does digital economy affect carbon emissions? Evidence from global 60 countries. Sci. Total Environ. 852, 158401. doi:10.1016/j.scitotenv.2022.158401
Dopp, K. A. (2016). Global warming, carbon emissions, and atmospheric carbon. SSRN Electron. J. doi:10.2139/ssrn.2780689
Dubash, N. K. (2020). Climate laws help reduce emissions. Nat. Clim. Change 10 (8), 709–710. doi:10.1038/s41558-020-0853-6
Edmans, A. (2023). Applying economics—not gut feel—to ESG. Financial Analysts J. 79 (4), 16–29. doi:10.1080/0015198x.2023.2242758
Gu, R., Li, C., Yang, Y., and Zhang, J. (2023). The impact of industrial digital transformation on green development efficiency considering the threshold effect of regional collaborative innovation: evidence from the Beijing-Tianjin-Hebei urban agglomeration in China. J. Clean. Prod. 420, 138345. doi:10.1016/j.jclepro.2023.138345
Guo, D., Li, L., Qiao, L., and Qi, F. (2023). Digital economy and consumption upgrading: scale effect or structure effect? Econ. Change Restruct. 56 (6), 4713–4744. doi:10.1007/s10644-023-09571-z
He, Z., Dong, T., Qian, W., and Xu, W. (2024). Dynamic interactions among trade policy uncertainty, climate policy uncertainty, and crude oil prices. Int. Rev. Econ. & Finance 95, 103479. doi:10.1016/j.iref.2024.103479
James, N., and Menzies, M. (2022). Global and regional changes in carbon dioxide emissions: 1970–2019 Phys. A: Stat. Mech. Appl. 608, 128302. doi:10.1016/j.physa.2022.128302
Johri, A., Asif, M., Tarkar, P., Khan, W., Rahisha, , and Wasiq, M. (2024). Digital financial inclusion in micro enterprises: understanding the determinants and impact on ease of doing business from World Bank survey. Humanit. Soc. Sci. Commun. 11 (1), 1–10. doi:10.1057/s41599-024-02856-2
Keeley, A. R., Chapman, A. J., Yoshida, K., Xie, J., Imbulana, J., Takeda, S., et al. (2022). ESG metrics and social equity: investigating commensurability. Front. Sustain. 3. doi:10.3389/frsus.2022.920955
Kishor, K., Bansal, S. K., and Kumar, R. (2024). The role of Fintech in promoting financial inclusion to achieve sustainable development: an integrated bibliometric analysis and systematic literature review. J. Knowl. Econ. doi:10.1007/s13132-024-02168-5
Kong, X., Li, Z., and Lei, X. (2024). Research on the impact of ESG performance on carbon emissions from the perspective of green credit. Sci. Rep. 14 (1), 10478. doi:10.1038/s41598-024-61353-3
Kwilinski, A., Lyulyov, O., and Pimonenko, T. (2023). Unlocking sustainable value through digital transformation: an examination of ESG performance. Information 14 (8), 444. doi:10.3390/info14080444
Lee, C., Yuan, Z., Lee, C., and Chang, Y. (2022). The impact of renewable energy technology innovation on energy poverty: Does climate risk matter? Energy Economics, 116, 106427. doi:10.1016/j.eneco.2022.106427
Lee, K., and Cho, J. (2023). Measuring Chinese climate uncertainty. Int. Rev. Econ. & Finance 88, 891–901. doi:10.1016/j.iref.2023.07.004
Li, L., Saat, M. M., and Jiayi, W. (2024). The Effect of ESG Disclosure on Firm Value: An Empirical Evidence from Chinese Listed Companies. International Journal of Academic Research in Business and Social Sciences 14 (1). doi:10.6007/ijarbss/v14-i1/20524
Li, J., and Xu, X. (2024). Can ESG rating reduce corporate carbon emissions? – An empirical study from Chinese listed companies. J. Clean. Prod. 434, 140226. doi:10.1016/j.jclepro.2023.140226
Li, T. T., Wang, K., Sueyoshi, T., and Wang, D. D. (2021a). ESG: research progress and future prospects. Sustainability 13 (21), 11663. doi:10.3390/su132111663
Li, Y., Yang, X., Ran, Q., Wu, H., Irfan, M., and Ahmad, M. (2021b). Energy structure, digital economy, and carbon emissions: evidence from China. Environ. Sci. Pollut. Res. 28 (45), 64606–64629. doi:10.1007/s11356-021-15304-4
Li, Z., and Wang, J. (2022). The dynamic impact of digital economy on carbon emission reduction: evidence city-level empirical data in China. J. Clean. Prod. 351, 131570. doi:10.1016/j.jclepro.2022.131570
Liang, C., Umar, M., Ma, F., and Huynh, T. L. D. (2022). Climate policy uncertainty and world renewable energy index volatility forecasting. Technol. Forecast. Soc. Change 182, 121810. doi:10.1016/j.techfore.2022.121810
Luo, L., and Tang, Q. (2022). The real effects of ESG reporting and GRI standards on carbon mitigation: international evidence. Bus. Strategy Environ. 32 (6), 2985–3000. doi:10.1002/bse.3281
Liu, Y., Zhang, X., and Shen, Y. (2024). Technology-driven carbon reduction: analyzing the impact of digital technology on China’s carbon emission and its mechanism Technol. Forecast. Soc. Change 200, 123124. doi:10.1016/j.techfore.2023.123124
Lyu, K., Yang, S., Zheng, K., and Zhang, Y. (2023). How does the digital economy affect carbon emission efficiency? Evidence from energy consumption and industrial value chain. Energies 16 (2), 761. doi:10.3390/en16020761
Ma, Y., Ji, Q., Liu, Z., Ma, D., Zhai, P., Guo, K., et al. (2023a). China’s provincial CPU index. Figshare. doi:10.6084/m9.figshare.24071256.v1
Ma, Y., Liu, Z., Ma, D., Zhai, P., Guo, K., Zhang, D., et al. (2023b). A news-based climate policy uncertainty index for China. Sci. Data 10 (1), 881. doi:10.1038/s41597-023-02817-5
Matos, P. (2020). ESG and responsible institutional investing around the world: a critical review. SSRN Electron. J. doi:10.2139/ssrn.3668998
Ong, T. S., Lee, A. S., Teh, B. H., and Magsi, H. B. (2019). Environmental innovation, environmental performance and financial performance: evidence from Malaysian environmental proactive firms. Sustainability 11 (12), 3494. doi:10.3390/su11123494
Persakis, A. (2023). The impact of climate policy uncertainty on ESG performance, carbon emission intensity and firm performance: evidence from Fortune 1000 firms. Environ. Dev. Sustain. 26, 24031–24081. doi:10.1007/s10668-023-03634-x
Qian, Y., and Liu, Y. (2024). Improve carbon emission efficiency: what role does the ESG initiatives play? J. Environ. Manag. 367, 122016. doi:10.1016/j.jenvman.2024.122016
Ren, X., Li, J., He, F., and Lucey, B. (2023). Impact of climate policy uncertainty on traditional energy and green markets: evidence from time-varying granger tests. Renew. Sustain. Energy Rev. 173, 113058. doi:10.1016/j.rser.2022.113058
Ren, X., Xiao, Y., Xiao, S., Jin, Y., and Farhad, T.-H. (2024). The effect of climate vulnerability on global carbon emissions: evidence from a spatial convergence perspective. Resour. Policy 90, 104817. doi:10.1016/j.resourpol.2024.104817
Ren, X., Zhang, X., Yan, C., and Gozgor, G. (2022). Climate policy uncertainty and firm-level total factor productivity: evidence from China. Energy Econ. 113, 106209. doi:10.1016/j.eneco.2022.106209
Sarfraz, M., Mohsin, M., and Naseem, S. (2022). A blessing in disguise: new insights on the effect of COVID-19 on the carbon emission, climate change, and sustainable environment. Environ. Sci. Pollut. Res. 29 (20), 29651–29662. doi:10.1007/s11356-021-17507-1
Shao, Y. (2018). Does FDI affect carbon intensity? New evidence from dynamic panel analysis. Int. J. Clim. Change Strategies Manag. 10 (1), 27–42. doi:10.1108/ijccsm-03-2017-0062
Shu, H., and Tan, W. (2023). Does carbon control policy risk affect corporate ESG performance? Econ. Model. 120, 106148. doi:10.1016/j.econmod.2022.106148
Su, J., and Xue, L. (2023). ESG performance, demographic trend, and labour investment efficiency in China. Appl. Econ. Lett. 31, 2207–2213. doi:10.1080/13504851.2023.2212956
Sun, X., Tao, J., and Kuang, X. (2023). A study of industrial structure, government attention, and coupling coordination of digital-green economy in China. J. Environ. Plan. 1–28. doi:10.1080/09640568.2023.2276681
Tarmuji, I., Maelah, R., and Tarmuji, N. H. (2016). The impact of environmental, social and governance practices (ESG) on economic performance: evidence from ESG score. Int. J. Trade, Econ. Finance 7 (3), 67–74. doi:10.18178/ijtef.2016.7.3.501
Vannoni, V., and Ciotti, E. (2020). Esg or not esg? A benchmarking analysis. Int. J. Bus. Manag. 15 (8), 152. doi:10.5539/ijbm.v15n8p152
Wang, G., Liao, M., and Jiang, J. (2020). Research on agricultural carbon emissions and regional carbon emissions reduction strategies in China. Sustainability 12 (7), 2627. doi:10.3390/su12072627
Wang, J., Ma, M., Dong, T., and Zhang, Z. (2023). Do ESG ratings promote corporate green innovation? A quasi-natural experiment based on SynTao green Finance’s ESG ratings. Int. Rev. Financial Analysis 87, 102623. doi:10.1016/j.irfa.2023.102623
Wang, L., and Shao, J. (2023). Digital economy, entrepreneurship and energy efficiency. Energy 269, 126801. doi:10.1016/j.energy.2023.126801
Wang, Y., Xiang, D., Yang, Z., and Ma, S. (2019). Unraveling customer sustainable consumption behaviors in sharing economy: a socio-economic approach based on social exchange theory. J. Clean. Prod. 208, 869–879. doi:10.1016/j.jclepro.2018.10.139
Xie, Y. (2024). The interactive impact of green finance, ESG performance, and carbon neutrality. J. Clean. Prod. 456, 142269. doi:10.1016/j.jclepro.2024.142269
Xiong, C., Yang, D., Xia, F., and Huo, J. (2016). Changes in agricultural carbon emissions and factors that influence agricultural carbon emissions based on different stages in Xinjiang, China. Sci. Rep. 6 (1), 36912. doi:10.1038/srep36912
Yi, M., Liu, Y., Sheng, M. S., and Wen, L. (2022). Effects of digital economy on carbon emission reduction: new evidence from China. Energy Policy 171, 113271. doi:10.1016/j.enpol.2022.113271
Zakari, A., Khan, I., Tan, D., Alvarado, R., and Dagar, V. (2022). Energy efficiency and sustainable development goals (SDGs). Energy 239, 122365. doi:10.1016/j.energy.2021.122365
Zhong, K., Fu, H., and Li, T. (2022). Can the digital economy facilitate carbon emissions decoupling? An empirical study based on provincial data in China. Int. J. Environ. Res. Public Health 19 (11), 6800. doi:10.3390/ijerph19116800
Zhu, L., Yang, M., Li, W., Liao, H., and Huang, H. (2021). The spatial–temporal changes of the coupling relationship among agricultural labor force, agricultural economy, and farmland in chongqing. Sustainability 13 (16), 8780. doi:10.3390/su13168780
Keywords: sustainable development goals, ESG performance, carbon emission intensity, climate policy uncertainty, digital economy
Citation: Li S, Ao X, Zhang M and Pu M (2025) ESG performance and carbon emission intensity: examining the role of climate policy uncertainty and the digital economy in China’s dual-carbon era. Front. Environ. Sci. 12:1526681. doi: 10.3389/fenvs.2024.1526681
Received: 15 November 2024; Accepted: 31 December 2024;
Published: 24 January 2025.
Edited by:
Xiaohang Ren, Central South University, ChinaReviewed by:
Najaf Iqbal, Anhui University of Finance and Economics, ChinaCopyright © 2025 Li, Ao, Zhang and Pu. This is an open-access article distributed under the terms of the Creative Commons Attribution License (CC BY). The use, distribution or reproduction in other forums is permitted, provided the original author(s) and the copyright owner(s) are credited and that the original publication in this journal is cited, in accordance with accepted academic practice. No use, distribution or reproduction is permitted which does not comply with these terms.
*Correspondence: Mingyue Pu, MTE4MzM0Nzg3NUBxcS5jb20=
Disclaimer: All claims expressed in this article are solely those of the authors and do not necessarily represent those of their affiliated organizations, or those of the publisher, the editors and the reviewers. Any product that may be evaluated in this article or claim that may be made by its manufacturer is not guaranteed or endorsed by the publisher.
Research integrity at Frontiers
Learn more about the work of our research integrity team to safeguard the quality of each article we publish.