- Faculty of Humanities and Social Sciences, Macao Polytechnic University, Macau, China
Introduction: China is the largest emitter of carbon dioxide (CO2) in the world, with its power industry being the primary source of these emissions. The high emission levels are primarily attributed to the extensive consumption of fossil resources during thermal power generation. Developing clean energy power generation to replace thermal power generation is one of the main strategies to mitigate the high level of carbon emissions in the power industry and thus promoting its low-carbon transition.
Method: To explore the relationship between China’s development level of clean electricity and carbon emissions from thermal power, this paper conducts an empirical study using provincial data from 2008 to 2021, while employing fixed-effects models, mediation effect tests, and heterogeneity tests.
Results and discussion: The results indicate that an increase in the level of clean electricity development reduces CO2 emissions from thermal power, and this effect exhibits a trend of diminishing from the eastern economic zone to the western economic zone in China. In the eastern regions with higher levels of urbanization, the development of clean electricity has a more significant effect on mitigating CO2 emissions from thermal power, whereas upgrading industrial structure in central and western regions contributes more in decreasing CO2 emissions from thermal power. Research and development (R&D) investment plays a significant mediating role and thus helps to improve the level of clean electricity development and reduce carbon emissions. Finally, the policy implications are proposed to intensify R&D investment, promote crossregional cooperation in sharing of clean energy, implement differentiated clean energy development policies, and establish a sustainable monitoring and evaluation system.
1 Introduction
The development and utilization of energy are crucial factors driving economic growth (Wang N. et al., 2022). However, along with rapid economic growth, the sharp increase in energy demand and the worsening environmental pollution have become major challenges constraining the high-quality development of China’s economy and society. Against this backdrop, the Chinese government has formulated the “dual carbon” goals, namely, “China aims to achieve a peak in carbon dioxide emissions by 2030 and strives to achieve carbon neutrality by 2060.” To realize this ambitious target, the government has taken robust measures across multiple industries. For instance, in the power sector, China is actively promoting clean energy generation, thereby reducing the use of traditional fossil fuels such as coal to lower carbon emissions. Concurrently, the government is also promoting energy-saving and emission-reduction technologies in industries, transportation, construction, and other sectors, enhancing energy efficiency and accelerating green and low-carbon development. Furthermore, China has tightened carbon emission regulations and established a carbon emission trading market, using market mechanisms to incentivize enterprises to reduce their carbon footprint (Fang et al., 2023). Among these measures, the development of clean energy and the low-carbon transformation of the power industry are crucial for resolving the conflict between China’s tightening carbon emission constraints and the rising energy demand for economic and social development, thereby advancing low-carbon energy supply and the “dual carbon” goals (Zhao et al., 2021).
The power industry is the largest emitter of CO2 globally (IEA, 2018). China is the largest emitter of CO2 in the world, with the power industry being the primary contributor to carbon emissions (Du et al., 2018; Luo et al., 2022). As early as 2010, CO2 emissions from the power industry in China already accounted for 44% of the national total (Fei et al., 2014). Due to China’s energy endowment characteristics of being “rich in coal, poor in oil, and lacking in gas,” the power industry has long been highly dependent on coal resources. Coal accounts for over 65% of China’s primary energy consumption, with the power industry consuming 50% of the total coal consumption, primarily for coal-fired power generation (Yang and Lin, 2016). This coal-dominated power generation structure, coupled with the rapid growth of power demand caused by the rapid socio-economic development of China, is the main reason why the power industry has become the largest source of carbon emissions (Chen et al., 2017). In 2018, China’s power industry emitted 4.6 billion tons of CO2 from coal-fired power generation, exceeding the combined emissions from fossil fuel combustion in the EU and Japan and accounting for 13.85% of global CO2 emissions (IEA, 2020). The carbon emissions in China’s power industry primarily stem from coal-fired power generation (Wang et al., 2017; IEA, 2022a; Yang and Wang, 2023), with coal-fired electricity contributing to 97% of the carbon emissions from thermal power generation (Zhang and Tong, 2021). Given the high dependence on coal, the push for clean energy is seen as essential for reducing the carbon footprint of the power industry (Zhao et al., 2021; Wu and Niu, 2022).
In recent years, while China’s thermal power installed capacity has gradually increased, its proportion in the total installed capacity has been declining, as shown in Table 1. This change is primarily attributed to the Chinese government’s vigorous development and promotion of clean energy, which has led to a rapid increase in clean energy power generation installed capacity (Chai et al., 2023). The gradual replacement of thermal power generation by renewable energy generation is considered an important pathway to achieve decarbonization in the power industry (Chiu and Chang, 2009; Liu et al., 2022). According to the report by the International Energy Agency (IEA), the replacement of thermal power generation with renewable energy makes the greatest contribution to reducing carbon emissions across all policy scenarios for emission reduction. It is predicted that by 2030, nearly half of the emission reduction gap between the established policy goals and committed targets set by various countries will be achieved through the rapid deployment of renewable energy (IEA, 2022b). Given the dominant position of thermal power generation in China’s power industry and its significant contribution to CO2 emissions, exploring the impact of clean energy power generation on carbon emissions from thermal power is of great significance for formulating energy policies and promoting the low-carbon transformation of the power industry.
2 Literature review
The development of clean energy not only provides a new pathway for addressing energy shortage issues but also serves as a crucial measure to reduce dependence on fossil fuels, lower CO2 emissions, and mitigate climate change. Many researchers have begun to focus on this field, exploring whether it contributes to reducing CO2 emissions. Existing research conclusions can be primarily categorized into two viewpoints.
The first viewpoint suggests that the development of clean energy contributes to reducing CO2 emissions. In a study on global CO2 emissions in 2022, it was found that the robust expansion of renewable energy sources constrained the growth of carbon emissions from fossil fuel consumption in the power industry, with the increase in solar and wind power generation preventing approximately 465 million tons of CO2 emissions in the power industry (IEA, 2023). A study on centralized photovoltaic stations in China’s clean energy sector shows that photovoltaic technology features a short carbon payback period, achieves significant carbon emissions reduction, and offers economic benefits, thereby aiding in climate change mitigation (Deng et al., 2023). A study analyzing China’s energy transition highlights energy substitution, with renewable energy replacing coal-fired power, as key to carbon neutrality, concluding that renewable energy will become the main power supply in the later stages of the transition (Wang Y D. et al., 2022). An analysis of the factors influencing CO2 emissions in thirty-six (OECD) countries from 1985 to 2018 has shown that electricity consumption and economic growth contribute to an increase in CO2 emissions, while increasing renewable energy consumption aids in reducing CO2 emissions (Cao et al., 2021). Another analysis, based on time-series data from China spanning 1985 to 2015, reveals that increasing clean energy consumption plays a crucial role in CO2 emission reduction, with a 1% rise in the proportion of clean energy consumption leading to a reduction of 0.129% in CO2 emissions (Ling, 2017). A study examining the top six nuclear power-generating countries worldwide has revealed that the development of nuclear energy, as a clean energy source, contributes to mitigating CO2 emissions (Baek and Pride, 2014). A study using data from China spanning 1994 to 2008 analyzed the impact of energy consumption structure, energy intensity, industrial structure, and total output value on carbon emissions, suggesting that continuous development and application of clean energy can optimize the energy consumption structure and aid in controlling carbon emissions (Lu et al., 2013). Another viewpoint suggests that the development of clean energy does not significantly reduce CO2 emissions. The Granger causality test results for the period from 1960 to 2007 in the United States reveal that renewable energy consumption does not exhibit a causal relationship with CO2 emissions (Menyah and Wolde-Rufael, 2010). Another study on renewable energy consumption and carbon emissions in the United States suggests that an increase in nuclear energy consumption does not effectively reduce CO2 emissions (Jaforullah and King, 2015). A study examining the relationship between clean energy and CO2 emissions across 24 countries in the Middle East and North Africa region reveals that the effect of clean energy on reducing CO2 emissions is not significant (Kahia et al., 2016). Another study, examining actual data from 1979 to 2016 and forecast data from 2017 to 2030 for 30 provinces in China, indicates that from a purely linear perspective, the development of clean energy has not significantly reduced CO2 emissions (Xu et al., 2019).
In summary, existing literature and research conclusions have provided some reference and insights for this paper. The existence of different situations regarding related conclusions may be attributed to the following reasons: Firstly, some studies often tend to analyze data at the national level, but this approach may obscure the significant differences in economic development and energy consumption patterns across various regions. In fact, these disparities also impact the development of clean energy in different areas. Therefore, utilizing provincial data allows for a more precise revelation of these regional differences, providing a more scientific basis for research. Secondly, differences in research methods and the time span of the data used can also play a role. The development of clean energy is a phased process, and its impacts vary at different stages of development. Thirdly, when exploring the emission reduction effects of clean energy, some literature focuses on the carbon emissions of the entire industry as the research object. The carbon emissions of the entire industry are comprised of both the energy sector and non-energy sectors, and the carbon emissions from non-energy sectors also account for a certain proportion. The carbon emissions from non-energy sectors can act as a confounding factor, interfering with the accurate assessment of clean energy’s actual emission reduction effects and potentially leading to an underestimation of its reduction achievements in the early stages of clean energy development. To avoid the aforementioned issues, this paper conducts a study on carbon emissions solely from the perspective of the electricity industry, based on provincial data from China. Furthermore, it analyzes the heterogeneous characteristics existing across different regions, categorized according to China’s three major economic zones: eastern, central, and western regions.
3 Theoretical background and hypothesis
3.1 Impact of clean electricity development on carbon emissions
Clean electricity refers to the electrical energy that is converted from clean energy sources using specific power generation equipment. It primarily encompasses wind power, hydropower, solar power, and nuclear power. This type of electrical energy does not produce greenhouse gas emissions during its production process, and it possesses notable environmental advantages compared to traditional coal-fired power generation.
The impact of clean electricity development on carbon emissions from thermal power generation mainly consists of two aspects. On one hand, clean electricity development has a direct impact on carbon emissions from thermal power. Increasing the share of clean electricity in the power supply will directly replace some of the thermal power, thereby reducing fossil fuel consumption and corresponding carbon emissions from thermal power (Luo and Zhang, 2024; Song et al., 2023). On the other hand, clean electricity development has an indirect impact on carbon emissions from thermal power. The development of clean electricity is often accompanied by a series of preferential policies, such as subsidies for green electricity, green certificate transactions, green credit, and green bonds. These policies not only promote the rapid development of clean electricity but also reduce its production costs, enhance its competitiveness in the market, and guide more investors and consumers towards choosing clean electricity, ultimately reducing the market demand for thermal power (Xu and Xu, 2023; Xu et al., 2023; Shah et al., 2024). Meanwhile, to compete with clean electricity and maintain market competitiveness, thermal power companies are compelled to invest heavily in technology, enhancing power generation efficiency and reducing carbon emissions. This includes developing Carbon Capture and Storage (CCS) technology, which captures and stores carbon dioxide during the thermal power generation process. In addition, compared to thermal power, clean electricity generation equipment offers more economical deployment costs. This has led some high-energy-consuming enterprises to deploy “self-generation and self-consumption” distributed rooftop photovoltaic systems, along with corresponding large-scale energy storage equipment. During peak electricity usage periods, these enterprises release the clean electricity accumulated in the storage equipment to meet their own demands. This “microgrid” system, autonomously constructed and managed by the enterprises, reduces their reliance and load on external thermal power generation, thereby decreasing carbon emissions from thermal power.Hypothesis H1:. Clean electricity development may have an inhibitory effect on carbon emissions from thermal power.
3.2 The mediating mechanism of R&D investment
Research and Development (R&D) investment, as a crucial driver of technological innovation, plays a pivotal role in the development of the clean energy sector (Goers et al., 2021; Khan et al., 2024). Research indicates that higher R&D expenditure contributes to reducing CO2 emissions (Ma et al., 2021). When clean energy technologies receive support from R&D investment, their potential for technological breakthroughs and innovation is significantly enhanced, which can subsequently improve the efficiency of clean energy generation and reduce costs. Taking wind power generation technology as an example, the technological advancements fueled by substantial R&D investments have led to a continuous increase in the average capacity per wind turbine and a marked improvement in energy conversion efficiency. During the period from 2011 to 2021, the average capacity of new onshore wind turbines in China increased from 1.5 MW (MW) to 3.1 MW, whereas the average capacity of new offshore wind turbines rose from 2.7 MW to 5.6 MW, as shown in Figure 1. In addition, there has been a significant decrease in the costs of solar and wind power generation. Data indicates that over the past decade, the global costs of generating solar, onshore wind, and offshore wind power have declined by 83%, 42%, and 34% respectively, with China experiencing the most substantial reduction in these costs (International Renewable Energy Agency, 2023). This trend is likely to further enhance the competitive advantage of clean electricity in the power market, thereby increasing its substitution effect for fossil fuel-based power generation and accelerating the pace of low-carbon transformation in the power industry. The continuous increase in R&D investment has effectively driven the continuous emergence of technological innovations. This trend is likely to further enhance the competitive advantage of clean electricity in the power market, thereby increasing its substitution effect for fossil fuel-based power generation and accelerating the pace of low-carbon transformation in the power industry.Hypothesis H2:. R&D investment may play a mediating role between clean electricity development and carbon emissions from thermal power.
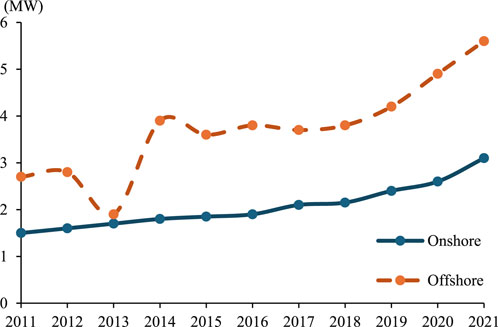
Figure 1. Average Capacity of New Wind Turbine Installations in China (from 2011 to 2021). Note: Data are from the China Wind Energy Association (CWEA).
3.3 Regional heterogeneity
The impact of clean electricity development on CO2 emissions from thermal power may exhibit significant regional heterogeneity due to variations in natural and geographical conditions, economic development levels, and energy structures. Firstly, due to variations in natural geographical conditions such as terrain and climate, the distribution of clean energy resources is uneven, leading to significant differences in the costs of their development and utilization across different regions (Liu et al., 2018; Tang et al., 2019). In regions with favorable natural geographical conditions and abundant clean energy resources, the development of clean electricity is more economical, resulting in a significant portion of the region’s energy supply coming from clean energy rather than relying on traditional fossil fuels (Mao et al., 2021). In such cases, further increasing the level of clean electricity development does not have a significant inhibitory effect on CO2 emissions from the thermal power. Secondly, different regions possess varying strengths and weaknesses in terms of factor resources such as capital, technology, and talent, which influence the research, development, and application of clean energy technologies. In regions with high levels of economic development and strong technological innovation capabilities, clean energy technologies are more likely to be developed and applied, thereby more effectively facilitating CO2 emission reduction in thermal power generation (He et al., 2023). In addition, differences in energy structures across regions, stemming from varying degrees of dependence on fossil fuels, also influence the progress and effectiveness of clean energy substituting for fossil fuels (Wang et al., 2024). Thus, due to regional variations in natural geographical conditions, economic development levels, and energy structures, there exist differences in the distribution of clean energy resources, technological research and development, application conditions, as well as the potential for energy substitution. These disparities may lead to regional heterogeneity in the impact of clean electricity development on carbon emission reduction from thermal power.Hypothesis H3:. There may be regional heterogeneity in the carbon emission reduction effects of clean electricity development on thermal power.
Figure 2 illustrates the research framework, with the hypotheses displayed in the figure.
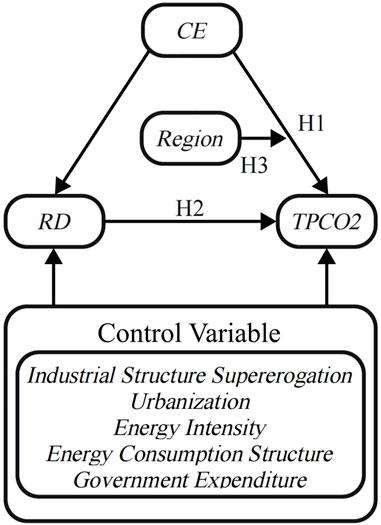
Figure 2. Research framework. Note: CE is the level of clean electricity development. TPCO2 is thermal power CO2 emissions. RD is research and development investment. Region refers to China’s three major economic zones.
4 Model construction and data
4.1 Variable selection
4.1.1 The explained variable
The explained variable is thermal power Carbon dioxide emissions (TPCO2), and take it logarithm (lnTPCO2). The specific calculation entails multiplying the annual thermal power generation output of each province by the national fossil fuel-based power CO2 emission factor. The national fossil fuel-based power CO2 emission factor refers to the amount of CO2 emitted per unit of fossil fuel-based power generation output, with an emission coefficient of 0.8426 kgCO2/kWh. The relevant data are sourced from the Ministry of Ecology and Environment of China and the National Bureau of Statistics.
4.1.2 The main explanatory variable
The main explanatory variable is the level of clean electricity (CE) development. The level of clean electricity development is measured by the ratio of the annual total clean energy generation to the regional gross domestic product (GDP) in each province. The clean energy generation is calculated based on the data of hydroelectric, nuclear, wind, and solar energy generation in each province, which are obtained from the provincial statistical yearbooks and the China Energy Statistical Yearbook.
4.1.3 The mediating variable
The mediating variable is the research and development (R&D) investment, which is represented by the RD expenditure of industrial enterprises above a designated size, and take it logarithm (lnRD). It is generally believed that higher R&D investment indicates more resources being allocated to the exploration of new technologies, products, or services, which directly enhances the activity of technological innovation. This, in turn, plays a positive role in improving energy efficiency, optimizing energy consumption structures, and promoting the development of green and low-carbon technologies.
4.1.4 Control variables
There are numerous factors that influence carbon emissions. Based on relevant research, this paper selects five control variables for analysis, which are as follows: (1) Industrial structure supererogation (ISS): measured by the ratio of the value added of the tertiary industry to the value added of the secondary industry, which primarily gauges the upgrading trend of the industrial structure (Gan et al., 2011). (2) Urbanization (URB): measured by the ratio of the number of urban residents to the total resident population. (3) Energy intensity (EI): measured by the ratio of total energy consumption (in ten thousand tons of standard coal) to the regional GDP. (4) Energy consumption structure (ECS): measured by the ratio of total coal consumption to total energy consumption. (5) Government expenditure (GE): represented by local government’s general budget expenditure, and take it logarithm.
4.2 Model construction
To investigate the impact of developing clean electricity on carbon emissions from thermal power in the electricity industry, this paper constructs the following model for analysis. Model (1) serves as the benchmark regression. Where subscript i represents the province; t represents the year; C is the constant term; CV is a series of control variables;
To examine Hypothesis H1 regarding the relationship between clean electricity development and carbon emissions from thermal power, as well as to investigate the regional heterogeneity of Hypothesis H3, the following model (1), also served as Equation 1, is constructed:
To test the mediating effect of R&D investment in Hypothesis H2, the following mediation effect models (2) and (3) are constructed:
4.3 Data source
This paper uses panel data from 30 provinces in China (excluding Tibet and the Hong Kong, Macao, and Taiwan regions) from 2008 to 2021 as the research sample. The original data is sourced from annual publications such as the China Statistical Yearbook, China Energy Statistical Yearbook, and China Electric Power Statistical Yearbook. Some missing values were filled by consulting provincial statistical yearbooks, while others were imputed using the linear interpolation method in Stata software. The descriptive results for each variable are presented in Table 2.
5 Empirical results and discussion
5.1 Regression results
To avoid the issue of multicollinearity among variables and enhance the accuracy of regression analysis, this study employs Variance Inflation Factor (VIF) as a diagnostic tool to examine multicollinearity in the model. Based on the VIF test results, the VIF values of all explanatory variables are less than the critical value of 5, with an average VIF of 2.1. This indicates that there is no severe multicollinearity among the variables in the model. Furthermore, the Hausman Test was conducted to choose between the Random Effects (RE) and Fixed Effects (FE) models. The test yielded a P-value of 0, which is significantly lower than the commonly used significance level of 0.05. Consequently, in the analysis of this panel data, the FE model is deemed more appropriate. The regression results are presented in Table 3.
Regardless of whether fixed effects are introduced, the regression coefficient of CE in the model exhibits negative significance. The results in columns (1), (3), and (4) all show significance at the 1% confidence level, while the results in column (2) indicate significance at the 5% confidence level. From the following regression results, it is evident that the two-way fixed-effect regression incorporating both year and province in column (4) is more appropriate. The results in column (4) indicate that CE is significant at the 1% confidence level, with a coefficient of −1.877. This suggests that for every one-unit increase in CE, TPCO2 will decrease by 1.877 units. These findings support the idea that enhancing the development level of clean electricity contributes to suppressing CO2 emissions from thermal power, thereby verifying Hypothesis H1.
5.2 Robustness tests
This paper uses four methods to test the robustness: (1) Replace the explained variable: Replace the explained variable with the per capita CO2 emissions from thermal power, and take it logarithm (lnTPCO2P). (2) Reduce the sample size: Due to the economic and political uniqueness of municipalities directly under the central government, some of their data may exhibit significant disparities with other provinces. To enhance the comparability of the data, we exclude the data of the four municipalities directly under the central government in China from the sample. (3) Lagged Variable: Regress after lagging the explanatory variable by one order. (4) Apply winsorization to the data: To avoid the interference of outliers on the results, this paper applies bilateral 1% tail trimming to the explanatory variables. F. lnTPCO2 is the explained variable of the previous stage, and CE_ w is the explanatory variable with bilateral 1% tail trimming. Table 4 shows the robustness test results. The regression coefficients are all significantly negative, which proves the previous benchmark analysis is strongly robust.
5.3 The mediating mechanism test
Table 5 reports the results of Bootstrap test, which are used to determine the presence and size of the mediation effect. First, after adding control variables, we conducted the Sobel test and obtained a P-value of 0, which is significantly lower than the commonly used significance level of 0.05. This suggests the presence of a mediation effect. Second, we performed the Bootstrap test with 1,000 bootstrap samples. The results indicated an indirect effect of −2.265, accounting for 33.6% of the total effect. The 95% confidence interval for the indirect effect was [-3.034, −1.495], excluding zero. The direct effect was −4.469, with a confidence interval of [-5.454, −3.483], also excluding zero. These findings confirm the presence of a partial mediation effect.
In addition, the stepwise regression method is also used for testing. Perform fixed-effects regression based on the mediation effect Formulas 2, 3 set in Section 4.2 above, and the results are shown in Table 6. The results in column (2) indicate a positive correlation between CE and RD at a 5% confidence level. The coefficient of 1.254 suggests that for every one-unit increase in CE, RD will increase by 1.254 units. The results in column (3) show that after adding the mediating variable RD, there is a negative correlation between CE and TPCO2 at a 1% confidence level. The absolute value of the coefficient of CE in column (3) decreases compared to that in column (1), indicating that part of the impact is transmitted through the mediating variable of R&D investment, which aligns with the criteria for judging mediation effects. Therefore, H2 is verified, indicating that R&D investment plays a mediating role between clean electricity development and CO2 emissions from thermal power. By increasing R&D investment, the level of clean electricity development can be effectively enhanced, CO2 emissions from thermal power can be reduced, and the low-carbonization of electricity supply can be promoted.
5.4 Regional heterogeneity analysis
Following the traditional division of economic zones in China, this paper categorizes the data samples into three major regions: the eastern economic zone, the central economic zone, and the western economic zone, and then conducts heterogeneity analysis, as shown in Table 7. The regression results indicate that the regression coefficients of CE are −11.136 and −3.677 for the eastern and central regions, respectively, both of which pass the test at the 1% significance level. In the western region, the regression coefficient of CE is −1.674, passing the test at the 10% significance level. Overall, from the eastern to the western regions, both the significance and the absolute values of the regression coefficients of CE show a clear decreasing trend. This suggests that the effectiveness of clean electricity development in reducing CO2 emissions from thermal power gradually decreases from the eastern region to the western region, demonstrating significant regional heterogeneity. Thus, H3 is verified.
The development of clean electricity has the most significant effect on reducing CO2 emissions from thermal power in the eastern region. This is primarily because the eastern region, as the most economically developed and highly urbanized area in China, experiences significant electricity demand due to its vibrant economic activities and large population. However, the region’s energy resources are not abundant, especially the scarcity of fossil fuels, resulting in a high dependence on external inputs for its electricity supply. In terms of local electricity supply, although the eastern region possesses a certain capacity of hydropower and nuclear power, thermal power still dominates as its primary mode of electricity generation, with a dependence on imported coal for thermal power production. According to data from the National Energy Administration in 2021, Shandong, Jiangsu, Guangdong, Zhejiang, and Hebei provinces rank first (528.104 billion kWh), third (484.131 billion kWh), fourth (463.857 billion kWh), seventh (304.854 billion kWh), and ninth (269.874 billion kWh), respectively, in thermal power generation among all provinces in China. This situation highlights the coal-dominated energy consumption structure in the eastern region. Therefore, the enhanced clean electricity development in the eastern region creates a pronounced substitution effect for thermal power, reducing fossil fuel consumption and enhancing CO2 emission reduction effects. At the same time, benefiting from the strong economic and technological capabilities of the eastern region, it has invested heavily in the research and development of clean energy technologies and has the ability to support large-scale clean energy project development, further enhancing the development of clean electricity. Similar conclusions have also been reached in related studies: Regions with a high level of urbanization have good conditions for technological R&D and expansion, high energy efficiency and renewable energy utilization technology, making the effect of reducing carbon emissions more pronounced (Adebayo and Ullah, 2023; Zheng et al., 2023). In addition, the regression coefficient of ISS is significantly positive in the eastern region but negative in the central and western regions, indicating that industrial structure upgrading reduces CO2 emissions from thermal power in the central and western regions but has the opposite effect in the eastern region. This is primarily because, in the early stages of industrial structure upgrading, energy demand was indeed reduced and CO2 emissions were decreased (Mi et al., 2017; Zheng et al., 2019). However, in the eastern region with a high degree of industrial transformation, the rapid development of the tertiary industry, especially information technology (IT) and artificial intelligence (AI), the demand for computing power has soared, leading to a sharp increase in electricity consumption (Xie et al., 2024). The main electricity for these computing centers still comes from fossil fuels, which partly offsets the carbon reduction achieved by upgrading industrial structures in the eastern regions. Similar viewpoints have been reached in other studies: the development of China’s tertiary industry can lead to an increasing trend in carbon dioxide emissions, but increasing energy investment, technological innovation, research and development expenditures, and the use of renewable energy can help mitigate these emissions (Ma et al., 2021; Xie et al., 2024).
The development of clean electricity has a significant effect in reducing CO2 emissions from thermal power in the central region, but it is still lower compared to the eastern region. This is primarily due to the abundant coal resource reserves in the central region, which provide convenient conditions for the deployment of thermal power generation. At the same time, considering that the economy in the central region is in a stage of rapid development, ensuring stable electricity supply has become a top priority. Due to the instability in power generation of some clean energy sources, the central region has shown insufficient enthusiasm in investing and promoting clean energy technologies. Although the central region possesses a certain scale of hydropower resources, which are mainly concentrated in Hubei and Hunan provinces, the scale effect of energy demand, driven by rapid economic development and sharp growth in total energy consumption, may outweigh the structural effect brought by the development of these clean energy sources. Looking ahead, with the further enhancement of economic development in the central region, it is expected that more funds will be invested in the development of clean energy, indicating significant potential for the low-carbon transformation of the power industry in this region.
The development of clean electricity has significantly reduced CO2 emissions from thermal power in the western region, but the effect and its significance have noticeably declined. This is primarily because the western region is endowed with abundant natural resources, especially wind, solar, and hydropower resources, which provide unique conditions for the development of clean energy in this area. In the southwestern region, the three provinces of Sichuan, Guizhou, and Yunnan, influenced by the Hengduan Mountains, have significant terrain variations and abound with rich hydropower resources. According to data from the National Energy Administration in 2021, Sichuan Province ranks first in hydropower generation nationwide (372.446 billion kWh), followed by Yunnan Province in second place (302.816 billion kWh) and Guizhou Province in fourth place (73.446 billion kWh). These abundant hydropower resources not only meet the demands of local industrial production and residential life, but also significantly reduce the consumption of fossil fuels in electricity supply. Meanwhile, due to its latitude and terrain, the northwest region also possesses abundant reserves of solar and wind energy resources. Therefore, a significant portion of the power supply in the western region comes from clean energy, and annually, a large amount of clean electricity is transmitted to the eastern region through the “West-to-East Electricity Transmission” project. Compared to the eastern and central regions, the western region has a lower dependence on thermal power.
6 Conclusion
To explore the specific role of developing clean electricity in controlling CO2 emissions from thermal power and promoting the low-carbon transition of the power industry, this paper utilizes provincial panel data from China spanning from 2008 to 2021. It analyzes the impact of clean electricity development on thermal power carbon emissions using a fixed-effects model and verifies the mediating effect of R&D investment between clean electricity development and thermal power carbon emissions using a mediation effect model. Additionally, this paper explains the reasons for the regional heterogeneity in the effectiveness of clean electricity development in reducing CO2 emissions from thermal power, considering factors such as natural geographical environment, energy consumption structure, industrial structure upgrading, and economic development level. Our research findings indicate the following.
(1) On the whole, the improvement in the development level of clean electricity plays a significant role in suppressing CO2 emissions from thermal power. It is of great importance for optimizing the coal-dominated electricity consumption structure and promoting the low-carbon transformation of the power industry.
(2) From the perspective of mediation effect, increasing investment in research and development can better enhance the development level of clean electricity, thereby reducing CO2 emissions from thermal power.
(3) From the perspective of regional heterogeneity, the improvement in the development level of clean electricity can reduce CO2 emissions from thermal power, and this effect shows a downward trend from the eastern economic zone to the western economic zone in China. In the eastern regions with higher urban development levels, the development of clean electricity has a more pronounced effect on mitigating CO2 emissions from thermal power. The advancement of industrial structure in central and western regions contributes to decreasing CO2 emissions from thermal power.
Based on the above research conclusions, this paper proposes the following suggestions to promote the development of clean energy and the low-carbon transformation of the power industry:
Firstly, to intensify R&D investment. To meet the practical requirements of achieving dual carbon goals, the future focus of the electricity market will be on high-proportion clean energy, with thermal power transitioning to a peak-shaving power source (Kopiske, et al., 2017). Consequently, research and development efforts should prioritize photovoltaic, wind power, and energy storage technologies. By increasing investment in clean energy technologies and fostering innovation, clean energy generation efficiency can be enhanced, costs reduced, and competitiveness in the electricity market strengthened. In this process, the government can offer incentives like subsidies and tax reductions to R&D enterprises and entities, aiming to reduce their costs and investment risks in clean energy and low-carbon technology. Additionally, the government should enhance the intellectual property protection system to safeguard the legitimate rights of research outcomes, thereby encouraging more enterprises and entities to actively engage in the R&D of clean energy and low-carbon technologies.
Secondly, to promote cross-regional cooperation and sharing of clean energy. Given the regional heterogeneity in clean energy development, it is essential to strengthen interregional cooperation. By establishing cross-regional clean energy trading platforms and improving power transmission networks, optimal allocation and efficient utilization of clean energy can be achieved across the country. In particular, for the abundant renewable energy resources in the western regions, we should increase development and transmission efforts to complement the high electricity demand in the eastern regions. Specifically, it is essential to leverage the role of market mechanisms in resource allocation and accelerate the reform of electricity marketization. In this process, emphasis should be placed on the effective integration of medium and long-term markets with spot markets within the inter-provincial electricity market, thereby enhancing the capacity to absorb clean energy electricity (Kang et al., 2016; Fan et al., 2021). In terms of grid construction, ultra-high-voltage transmission networks should be reasonably planned based on the distribution of clean energy resources and electricity demand to reduce transmission losses (Yi et al., 2016; Liu et al., 2018). Furthermore, it is crucial to prioritize the intelligent transformation of the grid, establishing an intelligent grid dispatching system to achieve precise forecasting and dynamic adjustment of electricity supply and demand, thereby further reducing the risk of electricity transmission congestion (Majeed Butt et al., 2021; Mahmood et al., 2024). This approach not only promotes economic development in the west but also contributes to reducing thermal power emissions in the east, driving the optimization and upgrading of China’s energy structure towards sustainable development.
Thirdly, to implement differentiated clean energy development policies. The government should formulate differentiated development policies tailored to each region’s economic level, resource endowments, and industrial structure. For eastern regions with high levels of urbanization, support for clean energy power generation projects should be strengthened. Active efforts should be made to promote the large-scale development and utilization of potential renewable energy resources in these regions, particularly wind power generation in coastal areas and photovoltaic power generation in mudflat zones. Meanwhile, it is crucial to ensure that the construction of grid infrastructure and advancements in energy storage technologies align with the pace of local renewable energy development, in order to mitigate the instability of renewable energy generation and enhance the stability of clean energy power supply (Leonard et al., 2020; Wang N. et al., 2022). For central regions, during the continuous promotion of clean energy development and industrial structure optimization, a batch of outdated high-energy-consuming thermal power generation facilities should be phased out in an orderly manner, and research and development efforts on Carbon Capture and Storage (CCS) technologies should be intensified to achieve carbon reduction targets for existing thermal power plants.
Fourthly, to establish a continuous monitoring and evaluation mechanism. Regular evaluations should be conducted on the application effects of clean energy in the power sector and the progress it has made in promoting the low-carbon transformation of the power industry. At the same time, the potential impact of clean energy projects on the ecological environment cannot be ignored, so environmental impact monitoring should also be included in the evaluation system. By monitoring key indicators such as the growth of clean energy generation, the reduction of carbon emissions from thermal power, the optimization of energy structure, and the improvement of environmental indicators, a comprehensive assessment of clean energy’s contribution to the low-carbon transformation of the power sector and environmental protection can be made. This enables policymakers to make data-driven decisions, promptly adjust and refine clean energy development strategies, ensure the high-quality development of clean energy, and effectively promote the low-carbon transformation of the power industry.
Data availability statement
Publicly available datasets were analyzed in this study. This data can be found here: China Statistical Yearbook, China Energy Statistical Yearbook, and China Electric Power Statistical Yearbook.
Author contributions
YC: Conceptualization, Data curation, Formal Analysis, Investigation, Software, Validation, Visualization, Writing–original draft, Writing–review and editing. EL: Formal Analysis, Visualization, Funding acquisition, Project administration, Resources, Supervision, Writing–review and editing. C-LT: Funding acquisition, Investigation, Resources, Supervision, Validation, Writing–review and editing. HW: Visualization, Writing–review and editing, Conceptualization, Data curation, Investigation, Software, Validation, Writing–original draft. JZ: Funding acquisition, Resources, Supervision, Validation, Writing–review and editing, Conceptualization, Investigation, Project administration, Writing–original draft, Data curation, Formal Analysis.
Funding
The author(s) declare that no financial support was received for the research, authorship, and/or publication of this article.
Conflict of interest
The authors declare that the research was conducted in the absence of any commercial or financial relationships that could be construed as a potential conflict of interest.
Generative AI statement
The author(s) declare that Generative AI was used in the creation of this manuscript. To help edit the manuscript and the Generative AI is not listed as an author of the manuscript, the content edited using the Generative AI has been checked for factual accuracy and plagiarism.
Publisher’s note
All claims expressed in this article are solely those of the authors and do not necessarily represent those of their affiliated organizations, or those of the publisher, the editors and the reviewers. Any product that may be evaluated in this article, or claim that may be made by its manufacturer, is not guaranteed or endorsed by the publisher.
References
Adebayo, T. S., and Ullah, S. (2023). Towards a sustainable future: the role of energy efficiency, renewable energy, and urbanization in limiting CO2 emissions in Sweden. Sustain. Dev., 1–16. doi:10.1002/sd.2658
Baek, J., and Pride, D. (2014). On the income–nuclear energy–CO2 emissions nexus revisited. Energy Econ. 43, 6–10. doi:10.1016/j.eneco.2014.01.015
Cao, H. M., Khan, M. K., Rehman, A., Dagar, V., Oryani, B., and Arifa, T. (2021). Impact of globalization, institutional quality, economic growth, electricity and renewable energy consumption on Carbon Dioxide Emission in OECD countries. Environ. Sci. Pollut. Res. 29, 24191–24202. doi:10.1007/s11356-021-17076-3
Chai, S., Liu, Q. Y., and Yang, J. (2023). Renewable power generation policies in China: policy instrumentchoices and influencing factors from the central and local government perspectives. Renew. Sustain. Energy Rev. 174, 113126. doi:10.1016/j.rser.2022.113126
Chen, H., Kang, J. N., Liao, H., Tang, B. J., and Wei, Y. M. (2017). Costs and potentials of energy conservation in China's coal-fired power industry: a bottom-up approach considering price uncertainties. Energy Policy 104, 23–32. doi:10.1016/j.enpol.2017.01.022
Chiu, C. L., and Chang, T. H. (2009). What proportion of renewable energy supplies is needed to initially mitigate CO emissions in OECD member countries? Renew. Sustain. Energy Rev. 13 (6-7), 1669–1674. doi:10.1016/j.rser.2008.09.026
Deng, Y. H., Wu, J. J., Yang, Q., Chen, W. Z., Li, P. H., Huang, C. H., et al. (2023). Life cycle-based carbon emission reduction benefit assessment of centralized photovoltaic power plants in China. Sustainability 15 (23), 16323. doi:10.3390/su152316323
Du, L. L., Li, X. Z., Zhao, H. J., Ma, W. C., and Jiang, P. (2018). System dynamic modeling of urban carbon emissions based on the regional National Economy and Social Development Plan: a case study of Shanghai city. J. Clean. Prod. 172 (20), 1501–1513. doi:10.1016/j.jclepro.2017.10.128
Fan, Y. Q., Ding, T., Sun, Y. G., He, Y. K., Wang, C. X., Wang, Y. Q., et al. (2021). Review and cogitation for worldwide spot market development to promote renewable energy accommodation. Proc. CSEE 41 (05), 1729–1752. doi:10.13334/j.0258-8013.pcsee.201408
Fang, K., Zhang, Q. F., and Tang, Y. Q. (2023). Emission trajectories and mitigation schemes for China. London: Taylor & Francis. doi:10.4324/9781003433859
Fei, T., Wang, X., and Lv, Z. (2014). Introducing the emissions trading system to China’s electricity sector: challenges and opportunities. Energy Policy 75, 39–45. doi:10.1016/j.enpol.2014.08.010
Gan, C. H., Zhang, R. G., and Yu, D. F. (2011). An empirical study on the effects of industrial structureon economic growth and fluctuations in China. Econ. Res. J. (05), 4–16+31.
Goers, S., Rumohr, F., Fendt, S., Gosselin, L., Jannuzzi, G. M., Gomes, R. D. M., et al. (2021). The role of renewable energy in regional energy transitions: an aggregate qualitative analysis for the partner regions bavaria, Georgia, québec, são paulo, Shandong, upper Austria, and western cape. Sustainability 13 (1), 76. doi:10.3390/su13010076
He, X., Sun, S. Q., Leong, L. W., Cong, P. T., Abu-Rumman, A., and Halteh, K. (2023). Does clean energy and technological innovation matter for economic growth? An Asian countries perspective. Econ. Analysis Policy 78, 1195–1208. doi:10.1016/j.eap.2023.04.017
IEA (2018). CO2 Emissions from fuel combustion 2018. Paris: International Energy Agency. doi:10.1787/co2_fuel-2018-en (Accessed November 6, 2024).
IEA (2020). China’s emissions trading scheme. Paris: International Energy Agency. Available at: https://www.iea.org/reports/chinas-emissions-trading-scheme (Accessed on November 6, 2024).
IEA (2022a). Global energy review: CO2 Emissions in 2021. Paris: International Energy Agency. Available at: https://www.iea.org/reports/global-energy-review-co2-emissions-in-2021-2 (Accessed on November 6, 2024).
IEA (2022b). World energy outlook 2022. Paris: International Energy Agency. Available at: https://www.iea.org/reports/world-energy-outlook-2022 (Accessed on November 6, 2024).
IEA (2023). CO2 Emissions in 2022. Paris: International Energy Agency. Available at: https://www.iea.org/reports/co2-emissions-in-2022 (Accessed on November 6, 2024).
International Renewable Energy Agency (2023). Renewable power generation costs in 2022. Abu Dhabi: International Renewable Energy Agency. Available at: https://www.irena.org/Publications/2023/Aug/Renewable-power-generation-costs-in-2022 (Accessed November 6, 2024).
Jaforullah, M., and King, A. (2015). Does the use of renewable energy sources mitigate CO2 emissions? A reassessment of the US evidence. Energy Econ. 49, 711–717. doi:10.1016/j.eneco.2015.04.006
Kahia, M., Kadria, M., and Ben Aissa, M. (2016) “What impacts of renewable energy consumption on CO2 emissions and the economic and financial development? A panel data vector autoregressive (PVAR) approach,” in 2016 7th international renewable energy congress (IREC). Hammamet, Tunisia, 22-24 March 2016 (IEEE), doi:10.1109/IREC.2016.7478912
Kang, C. Q., Du, E. S., Zhang, N., Chen, Q. X., Huang, H., and Wu, S. Y. (2016). Renewable energy trading in electricity market: review and prospect. South. Power Syst. Technol. 10 (03), 16–23+2. doi:10.13648/j.cnki.issn1674-0629.2016.03.003
Khan, S., Yuan, H. P., Yahong, W., and Xu, Q. (2024). Pathways to carbon neutrality in G7 economies: the role of technology-innovation and R&D in reducing CO2 emissions. Gondwana Res. 128, 55–68. doi:10.1016/j.gr.2023.10.015
Kopiske, J., Spieker, S., and Tsatsaronis, G. (2017). Value of power plant flexibility in power systems with high shares of variable renewables: a scenario outlook for Germany 2035. Energy 137 (15), 823–833. doi:10.1016/j.energy.2017.04.138
Leonard, M. D., Michaelides, E. E., and Michaelides, D. N. (2020). Energy storage needs for the substitution of fossil fuel power plants with renewables. Renew. Energy 145, 951–962. doi:10.1016/j.renene.2019.06.066
Ling, M. S. (2017). The clean consumption, environment governance and the sustainable economic growth in China. J. Quantitative and Tech. Econ. 34 (12), 3–21. doi:10.13653/j.cnki.jqte.2017.12.001
Liu, H. L., Andresen, G. B., and Greiner, M. (2018). Cost-optimal design of a simplified highly renewable Chinese electricity network. Energy 147, 534–546. doi:10.1016/j.energy.2018.01.070
Liu, S. Y., Lin, Z. Z., Jiang, Y. C., Zhang, T., Yang, L., Tan, W. T., et al. (2022). Modelling and discussion on emission reduction transformation path of China's electric power industry under “double carbon” goal. Heliyon 8 (9), e10497. doi:10.1016/j.heliyon.2022.e10497
Lu, W. B., Qiu, T. T., and Du, L. (2013). A study on influence factors of carbon emissions under different economic growth stages in China. Econ. Res. J. (04), 106–118.
Luo, C. F., and Zhang, K. Y. (2024). RETRACTED: elasticity of substitution between clean energy and non-clean energy: evidence from the Chinese electricity industry. Clean. Energy Syst. 8, 100117. doi:10.1016/j.cles.2024.100117
Luo, S. H., Hu, W. H., Liu, W., Zhang, Z. Y., Bai, C. G., Hang, Q., et al. (2022). Study on the decarbonization in China's power sector under the background of carbon neutrality by 2060. Renew. Sustain. Energy Rev. 166, 112618. doi:10.1016/j.rser.2022.112618
Ma, Q., Murshed, M., and Khan, Z. (2021). The nexuses between energy investments, technological innovations, emission taxes, and carbon emissions in China. Energy Policy 155, 112345. doi:10.1016/j.enpol.2021.112345
Mahmood, M., Chowdhury, P., Yeassin, R., Hasan, M., Ahmad, T., and Chowdhury, N. U. R. (2024). Impacts of digitalization on smart grids, renewable energy, and demand response: an updated review of current applications. Energy Convers. Manag. X 24, 100790. doi:10.1016/j.ecmx.2024.100790
Majeed Butt, O., Zulqarnain, M., and Majeed Butt, T. (2021). Recent advancement in smart grid technology: future prospects in the electrical power network. Ain Shams Eng. J. 12 (01), 687–695. doi:10.1016/j.asej.2020.05.004
Mao, A. H., Li, F. X., Yang, S. Y., Huang, T., Hao, R. F., Li, S. H., et al. (2021). Clean energy power generation potential and value in Qinghai Province. Resour. Sci. 43 (01), 104–121. doi:10.18402/resci.2021.01.09
Menyah, K., and Wolde-Rufael, Y. (2010). CO2 emissions, nuclear energy, renewable energy and economic growth in the US. Energy Policy 38 (6), 2911–2915. doi:10.1016/j.enpol.2010.01.024
Mi, Z. F., Meng, J., Guan, D. B., Shan, Y. L., Liu, Z., Wang, Y. T., et al. (2017). Pattern changes in determinants of Chinese emissions. Environ. Res. Lett. 12 (07), 074003. doi:10.1088/1748-9326/aa69cf
Shah, S. S., Murodova, G., and Khan, A. (2024). Achieving zero emission targets: the influence of green bonds on clean energy investment and environmental quality. J. Environ. Manag. 364, 121485. doi:10.1016/j.jenvman.2024.121485
Song, H. Y., Hou, G. S., and Xu, S. (2023). CO2 emissions in China under electricity substitution: influencing factors and decoupling effects. Urban Clim. 47, 101365. doi:10.1016/j.uclim.2022.101365
Tang, B. J., Li, R., Yu, B. Y., and Wei, Y. M. (2019). Spatial and temporal uncertainty in the technological pathway towards a low-carbon power industry: a case study of China. J. Clean. Prod. 230, 720–733. doi:10.1016/j.jclepro.2019.05.151
Wang, N., Fu, X. D., and &Wang, S. B. (2022). Spatial-temporal variation and coupling analysis of residential energy consumption and economic growth in China. Appl. Energy 309, 118504. doi:10.1016/j.apenergy.2021.118504
Wang, Q., Jiang, X. T., and Li, R. R. (2017). Comparative decoupling analysis of energy-related carbon emission from electric output of electricity sector in Shandong Province, China. Energy 127, 78–88. doi:10.1016/j.energy.2017.03.111
Wang, Y. B., Pan, H. Y., Zhen, J. J., and Xu, B. Y. (2024). Exploring the substitution within clean energy: evidence from China's top 14 hydropower provinces. Clean. Energy Syst. 9, 100152. doi:10.1016/j.cles.2024.100152
Wang, Y. D., Mao, J. Q., Chen, F., and Wang, D. L. (2022). Uncovering the dynamics and uncertainties of substituting coal power with renewable energy resources. Renew. Energy 193, 669–686. doi:10.1016/j.renene.2022.04.164
Wen, Z. L., and Ye, B. J. (2014). Analyses of mediating effects: the development of methods and models. Adv. Psychol. Sci. 22 (05), 731–745. doi:10.3724/SP.J.1042.2014.00731
Wu, G., and Niu, D. (2022). A study of carbon peaking and carbon neutral pathways in China's power sector under a 1.5 °C temperature control target. Environ. Sci. Pollut. Res. 29, 85062–85080. doi:10.1007/s11356-022-21594-z
Xie, X. M., Han, Y. H., and Tan, H. (2024). Greening China’s digital economy: exploring the contribution of the east–west computing resources transmission project to CO2 reduction. Humanit. Soc. Sci. Commun. 11, 466. doi:10.1057/s41599-024-02963-0
Xu, B., Chen, Y. F., and Shen, X. B. (2019). Clean energy development, carbon dioxide emission reduction and regional economic growth. Econ. Res. J. (07), 188–202.
Xu, J. J., Wang, J. C., Li, R., and Gu, M. X. (2023). Is green finance fostering high-quality energy development in China? A spatial spillover perspective. Energy Strategy Rev. 50, 101201. doi:10.1016/j.esr.2023.101201
Xu, S. Q., and Xu, Q. (2023). Optimal pricing decision of tradable green certificate for renewable energy power based on carbon-electricity coupling. J. Clean. Prod. 410, 137111. doi:10.1016/j.jclepro.2023.137111
Yang, L. S., and Lin, B. Q. (2016). Carbon dioxide-emission in China’s power industry: evidence and policy implications. Renew. Sustain. Energy Rev. 60, 258–267. doi:10.1016/j.rser.2016.01.058
Yang, R., and Wang, W. S. (2023). Potential of China's national policies on reducing carbon emissions from coal-fired power plants in the period of the 14th Five-Year Plan. Heliyon 9 (9), e19868. doi:10.1016/j.heliyon.2023.e19868
Yi, B. W., Xu, J. H., and Fan, Y. (2016). Inter-regional power grid planning up to 2030 in China considering renewable energy development and regional pollutant control: a multi-region bottom-up optimization model. Appl. Energy 184 (15), 641–658. doi:10.1016/j.apenergy.2016.11.021
Zhang, Q., and Tong, D. (2021). “Global energy infrastructure emissions and their lock-in effect,” in Institute for carbon neutrality. Beijing: Tsinghua University. Available at: https://www.efchina.org/Reports-zh/report-lceg-20220303-zh (Accessed November 3, 2024).
Zhao, G. P., Yu, B. Y., An, R. Y., Wu, Y., and Zhao, Z. H. (2021). Energy system transformations and carbon emission mitigation for China to achieve global 2 °C climate target. J. Environ. Manag. 292 (15), 112721. doi:10.1016/j.jenvman.2021.112721
Zheng, J. L., Mi, Z. F., Coffman, D., Milcheva, S., Shan, Y. L., Guan, D. B., et al. (2019). Regional development and carbon emissions in China. Energy Econ. 81, 25–36. doi:10.1016/j.eneco.2019.03.003
Keywords: clean energy, CO2 emissions, R&D investment, low carbon development, thermal power
Citation: Cai Y, Loi EH, Tung C-L, Wang H and Zheng J (2025) Clean energy development and low-carbon transition in China’s power industry. Front. Environ. Sci. 12:1525047. doi: 10.3389/fenvs.2024.1525047
Received: 08 November 2024; Accepted: 31 December 2024;
Published: 15 January 2025.
Edited by:
Saige Wang, University of Science and Technology Beijing, ChinaReviewed by:
Binzizi Dong, City University of Hong Kong, Hong Kong SAR, ChinaYiting Guo, Renmin University of China, China
Copyright © 2025 Cai, Loi, Tung, Wang and Zheng. This is an open-access article distributed under the terms of the Creative Commons Attribution License (CC BY). The use, distribution or reproduction in other forums is permitted, provided the original author(s) and the copyright owner(s) are credited and that the original publication in this journal is cited, in accordance with accepted academic practice. No use, distribution or reproduction is permitted which does not comply with these terms.
*Correspondence: Hao Wang, d2hhb21vQG91dGxvb2suY29t; Jiansong Zheng, amFuc29uNjg2OEAxNjMuY29t