- 1Business School, University of Jinan, Jinan, China
- 2Shandong Collaborative Innovation Center for Capital Market Innovation and Development, Jinan, China
Introduction: Green finance plays a pivotal role in advancing China’s ecological civilization construction and achieving sustainable economic and social development.
Methods: Using panel data from 30 provinces in China from 2009 to 2021, this study applies the entropy weighting method and the super-efficiency slack-based measure (Super-SBM) model to assess the levels of green finance and eco-efficiency in each province. Employing spatial statistical methods such as the spatial standard deviation ellipse (SSDE) and the spatial Markov chain, the study reveals the spatiotemporal evolution characteristics of green finance and eco-efficiency. Additionally, the spatial Durbin model is used to analyze the direct effects, spatial spillover effects, and spatial heterogeneity features of green finance on regional eco-efficiency.
Results: The results show that (1) From 2009 to 2021, green finance and regional eco-efficiency levels exhibited an overall upward trend, though with notable spatiotemporal differences. (2) The direct and spatial spillover effects of green finance on regional eco-efficiency followed a notable inverted U–shaped relationship. However, in most provinces, green finance levels had not yet reached the turning point during the study period, and the impact of green finance on regional eco-efficiency remained largely positive. (3) The effects of green finance on regional eco-efficiency showed considerable structural differences due to regional heterogeneity, with the strongest effects observed in the Eastern regions.
Discussion: This study provides policymakers with strategies to enhance regional eco-efficiency through the development of green finance, offering valuable insights for promoting ecological civilization construction and achieving sustainable development.
1 Introduction
With global society facing the pressures of resource demand and environmental degradation caused by economic growth, the pursuit of sustainable development has rapidly become a shared principle among countries at different stages of development (Shao et al., 2024). This shift reflects a growing concern for the future, recognizing the necessity to take action to safeguard the interests of our planet and future generations. From governments to businesses and individuals to international organizations, all are contributing to achievement of sustainable development. Since initiating economic reforms and opening-up policies, China has sustained prolonged periods of high-speed economic growth. However, the negative externalities on the environment and society have become increasingly evident (Jiang et al., 2023; Yan et al., 2021). The 2024 Global Performance Indicator report (Block et al., 2024) indicated that China’s EPI score dropped from 43 to 35.5 between 2014 and 2024, resulting in a ranking of 154th out of 180 participating countries and regions. According to the 2024 Statistical Review of World Energy (Energy Institute, 2024), China’s total carbon emissions in 2023 reached 11.22 billion tonnes, accounting for 31.9% of global emissions. To effectively address the resource and environmental constraints stemming from an extensive growth model, China has set the strategic goal of building an ecological civilization. Moreover, it has emphasized the need to improve green and low-carbon development policies as well as strengthen financial support for sustainable development.
In this context, the selection of eco-efficiency indicators to measure the level of regional ecological civilization development in China, and the examination of the impact of green finance on eco-efficiency, are critical for understanding how China can effectively and fully leverage the development of green finance to support its construction of ecological civilization. This study also provides clear empirical evidence and valuable insights for emerging economies to promote regional sustainable development through financial elements. Due to inherent factors, such as policy support and resource endowment, the foundational conditions for developing green finance systems and advancing ecological civilization have varied across different regions in China for a long time (Geng et al., 2023; Liang et al., 2021). This has resulted in substantial disparities in the development levels of green finance and eco-efficiency among these regions (Lv et al., 2021). Thus, accurate measurement and demonstration of the spatial and temporal evolution characteristics of green finance development and regional eco-efficiency are crucial for the systematic promotion of China’s ecological civilization. As a series of major regional strategies—such as the coordinated development of the Beijing–Tianjin–Hebei Region, the construction of the Guangdong–Hong Kong–Macao Greater Bay Area, and the ecological conservation and high-quality development of the Yellow River Basin—continue to progress steadily, China is accelerating efforts to promote integration and collaborative development among regions. In addition, considering the virtual nature of financial services and the high mobility of financial elements, it is essential to fully consider the spatial spillover effects of regional green finance (Li and Wang, 2019). This will help explore effective pathways and policy measures to strengthen the role of green finance in promoting regional eco-efficiency. However, the academic community has paid insufficient attention to the temporal and spatial evolution characteristics of green finance and regional eco-efficiency, as well as the spatial spillover effects of green finance on regional eco-efficiency.
Green finance also represents an important policy innovation that integrates market-based environmental regulation with the resource allocation functions of the financial system. Existing research focuses on the economic and environmental effects of green finance development. On the one hand, regarding economic effects, most studies agree that green finance can significantly promote green economic development (CİTİL, 2024; Hu et al., 2023; Ouyang et al., 2023; Zhang and Zhao, 2024). However, some researchers hold alternative viewpoints. For example, Shi and Shi (2022) and Li (2023) found that green finance has a nonlinear effect on green economic development, exhibiting threshold effects. These findings indicate that this study should not oversimplify the relationship between green finance and regional eco-efficiency as linear in empirical analyses. On the other hand, with regards to environmental effects, the majority of studies have explored the impacts of green finance on pollution reduction (Zhang et al., 2024), carbon reduction (Zhao et al., 2024), clean energy technology innovation (Liu F. et al., 2024), as well as energy transition and upgrading (Chen et al., 2025), analyzing both meso-level industry and micro-level enterprise perspectives. However, few studies have integrated finance, economy, environment, and resources into a unified macro-level framework. There is also a notable lack of in-depth discussion regarding the impacts of green finance on regional eco-efficiency and spatial effects. This drawback limits a comprehensive understanding of how green finance promotes sustainable development and constrains policymakers in their efforts to facilitate coordinated regional development and ecological civilization construction. Therefore, this study aimed to construct a general framework for investigating the impact of green finance on regional eco-efficiency from a spatial perspective. The goal is to achieve an overall improvement and differentiated governance of China’s regional eco-efficiency driven by green finance, thereby accelerating ecological civilization construction and achieving sustainable development.
Based on the above logical analysis, the study first scientifically measures the levels of green finance and regional eco-efficiency across 30 provinces in China from 2009 to 2021. Second, it employs spatial statistical methods to demonstrate the spatiotemporal evolution characteristics of both. Third, it uses spatial econometric models to empirically explore the direct effects, spatial spillover effects, and spatial heterogeneity of green finance on regional eco-efficiency. The main contributions of this study are as follows: Firstly, it employs spatial standard deviation ellipse (SSDE) and spatial Markov chain methods to examine the spatiotemporal evolution characteristics of green finance and regional eco-efficiency. This addresses the existing research gap regarding directional distribution features, migration paths of hotspot areas, internal liquidity, and stability conditions of both, thereby enriching the evaluation of these two concepts. Secondly, research on the association between green finance and regional eco-efficiency is limited in the academic field, lacking investigations into potential nonlinear relationships between the two and consideration of the spatial spillover effects of green finance. The study serves as a valuable supplement to the existing literature. Thirdly, through systematic theoretical deduction, the study elucidates the mechanisms by which green finance influences regional eco-efficiency, thereby providing a perspective and inspiration for future academic research as well as offering decision-making insights for tailoring regulatory policies to specific local conditions in different regions.
The remainder of the paper is organized as follows. Section 2 reviews relevant literature. Section 3 constructs empirical methods. Section 4 presents results. Section 5 provides some discussion. Section 6 covers conclusions and implications. The specific research framework is illustrated in Figure 1.
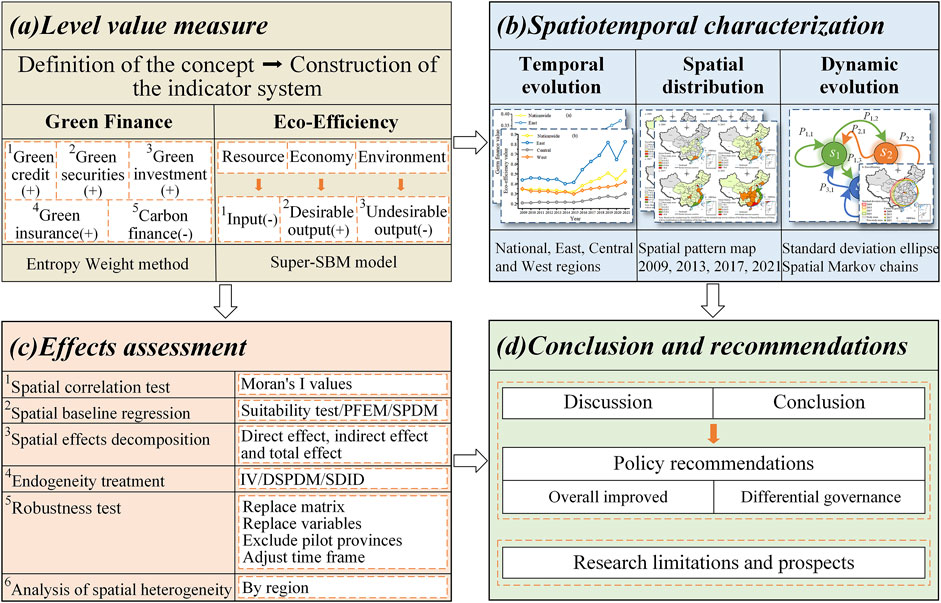
Figure 1. The research framework. (A) Level value measure. (B) Spatiotemporal characterization. (C) Effects assessment. (D) Conclusion and recommendations.
2 Literature review
2.1 Research on regional eco-efficiency
Currently, research on regional eco-efficiency mainly concentrates on three key aspects: measurement methods, evaluation of typical regions, and analysis of influencing factors. Firstly, regarding measurement methods, studies have gradually transitioned from single-factor evaluations to multi-factor assessments. Traditional single-factor measures often calculate eco-efficiency by comparing economic output to environmental load. Among economic output indicators, GDP is the most commonly used (Robaina-Alves et al., 2015; Yang and Yang, 2019; Zhang et al., 2008), with other studies utilizing indicators such as green GDP (Huang et al., 2018; Wang et al., 2015) or industrial GDP (Seppäläa et al., 2005; Ren et al., 2017). Environmental load is typically assessed through indicators like energy consumption, land use, wastewater, waste gas, and solid waste emissions (Yu et al., 2013). However, since different types of environmental impacts cannot be directly aggregated like economic indicators, it is necessary to assign appropriate weights to each environmental impact before integration. Common weighting methods include factor loading (Chen, 2008), preference-based methods (Huppes and Ishikawa, 2005), equal-weight methods used in the Human Development Index (Liu, 2010), and principal component analysis (Jollands et al., 2004). Although the single ratio approach is simple and intuitive, it does not provide decision-makers with the flexibility to choose optimal ratios and may be prone to subjective weight assignments (Kuosmanen, 2005; Quariguasi Frota Neto et al., 2009). Since eco-efficiency results from the combined impacts of energy consumption, economic development, and pollution emissions, it is inherently multi-dimensional. As a result, comprehensive evaluation methods, such as both parametric and non-parametric approaches, are increasingly applied. Among parametric methods, Stochastic Frontier Analysis (SFA) is widely used to measure efficiency with multiple inputs and a single output (Deng and Gibson, 2019; Song and Chen, 2019). Non-parametric methods, such as Data Envelopment Analysis (DEA) and its variations, are also commonly employed (Sun and Zhou, 2023; Tan and Wang, 2021). Notably, the DEA method, by utilizing linear programming techniques, does not require pre-defined input-output relationships, thereby helping to avoid systematic errors and ensuring minimal algorithmic inaccuracies (Liu, Q. et al., 2020). Furthermore, the DEA method is advantageous over SFA when dealing with multiple inputs and outputs across similar decision-making units (DMUs) (Sun et al., 2023), making it a prevalent tool for assessing regional eco-efficiency. Additionally, DEA models have been continually refined. For instance, the Charnes–Cooper–Rhodes (CCR) model (Charnes et al., 1978), the Banker–Charnes–Cooper (BCC) model (Banker et al., 1984), the SBM model (Tone, 2001), and the Super-SBM model (Andersen and Petersen, 1993; Tone, 2002) have been developed to tackle various efficiency measurement challenges. The Super-SBM model, which incorporates both desirable and undesirable outputs, overcomes the limitations of the original CCR and BCC models, which failed to consider environmental pollutants. This improvement ensures that the evaluation results are more aligned with real-world conditions (Zeng et al., 2023). Furthermore, the Super-SBM model allows for a finer distinction and ranking of DMUs on the efficiency frontier by permitting efficiency scores greater than 1. In the context of China, regional eco-efficiency is influenced by numerous factors, leading to significant disparities across cities. The Super-SBM model has been effectively employed to capture subtle differences in resource utilization, environmental impact, and economic development among cities, providing more refined and reliable eco-efficiency assessments. Consequently, the Super-SBM model has become widely utilized in measuring eco-efficiency in Chinese regions.
Secondly, regarding empirical research on regional eco-efficiency evaluation, academic studies have gradually shifted from static assessments based on a single time point or single region to spatiotemporal dynamic evaluations. Due to data limitations and other constraints in earlier studies, scholars were often restricted to using data from a single year or a single province to conduct static characterizations and differential analyses of regional eco-efficiency, which failed to capture the evolution mechanisms and spatial distribution characteristics of regional eco-efficiency. For example, Yin et al. (2011) used cross-sectional data from 2009 and applied three DEA models to assess the eco-efficiency of environmental model cities in China. Wang et al. (2015) comprehensively evaluated the regional eco-efficiency of Jiangsu Province from 1993 to 2012 using the DEA model. Subsequently, researchers began integrating both spatial and temporal dimensions to conduct dynamic studies on the changing trends of eco-efficiency, which have been widely applied at the county, provincial, watershed, economic belt, and urban agglomeration levels, thus providing more effective guidance for regional coordinated and sustainable development. For instance, Wang C. et al. (2023) used an SBM model with undesirable outputs to measure the eco-efficiency levels of provinces in the Yellow River Basin of China from 2005 to 2020, analyzing their spatial variation trends and internal differences. Liu et al. (2020) investigated the periodic characteristics of eco-efficiency in 57 cities along the Yangtze River Economic Belt between 2008-2012 and 2013-2016. Ren et al. (2019) used traditional DEA models and SBM models with undesirable outputs to evaluate and compare the eco-efficiency of four major urban agglomerations in eastern China in 2005, 2011, and 2014. In terms of evaluation methods, research has gradually shifted from quantitative statistical methods to spatial statistical methods, with the increasing incorporation of geographic information system (GIS) technology. Earlier studies predominantly relied on quantitative statistical models such as the σ-convergence coefficient (Yu et al., 2020), the Malmquist index (Luo and Wang, 2023), two-dimensional kernel density estimation (Zhao and Li, 2023), and traditional Markov chains. These traditional quantitative models typically assumed that regional spatial entities were independent and that there was no spatial correlation between them. While these methods focus on examining the temporal trends and spatial distribution patterns of regional eco-efficiency, they fail to comprehensively capture its spatiotemporal evolution. As a result, with the development and application of GIS technology, methods such as exploratory spatial data analysis (ESDA), ArcGIS spatial pattern maps, spatial standard deviation ellipses (SSDE), and spatial Markov chains have become increasingly popular in studying the spatiotemporal evolution of regional eco-efficiency. Among these, many scholars have applied ESDA methods to explore the spatial relationships of regional eco-efficiency (Cui and Wang, 2023). One of the approaches involves global spatial autocorrelation testing, which calculates the global Moran’s I index; another involves local spatial autocorrelation testing, using the local form of the Moran’s I index, which can be visualized through local indicators of spatial association (LISA) clustering maps. Zou et al. (2024) used the global Moran’s I index and kernel density estimation to reveal the spatiotemporal characteristics of eco-efficiency across 284 cities in China from 2009 to 2021. Li X. et al. (2022) used multi-source remote sensing data to extract input-output data and measured the eco-efficiency levels of counties in Shandong Province from 2000 to 2015, analyzing their spatiotemporal characteristics using kernel density estimation and LISA clustering maps. On the other hand, the diffusion (drip) and polarization (echo) effects of eco-efficiency between regions can either narrow or enlarge the regional differences in eco-efficiency (Han and Deng, 2020), which makes it difficult to capture the complex spatial dynamics of regional eco-efficiency accurately using ESDA methods. To address this, methods such as SSDE and spatial Markov chains have been gradually applied to analyze the spatiotemporal evolution of regional eco-efficiency. The SSDE method can characterize the directional features of the spatial distribution of eco-efficiency and track the migration paths of the centroids (Chen et al., 2023). The spatial Markov chain method can reveal the internal mobility and stability of regional eco-efficiency and forecast future trends in its evolution (Wang Y. et al., 2023). This study combines the advantages of the SSDE in describing the spatial distribution and heterogeneity of data with the strengths of the spatial Markov chain in analyzing spatial data evolution and transition patterns. This approach effectively quantifies the multidimensional dynamic evolution characteristics of eco-efficiency, improving the accuracy and depth of spatiotemporal analysis of regional eco-efficiency. It also contributes to promoting the coordinated development of regional eco-efficiency, providing a valuable complement and enhancement to existing research.
Thirdly, regarding the research on the influencing factors of regional eco-efficiency, scholars have increasingly shifted their focus from evaluating numerical results to analyzing the factors that influence changes in eco-efficiency patterns. The main factors influencing regional eco-efficiency include environmental regulation, industrial structure, urbanization rate, technological innovation, trade openness, and environmental awareness. Firstly, environmental regulation presents contrasting views. The “cost theory” suggests that such regulation suppresses corporate economic output and hinders the improvement of eco-efficiency. Conversely, the “innovation compensation theory” argues that well-designed environmental policies can stimulate firms’ technological innovation, with the resulting revenue offsetting or exceeding the costs of regulation and innovation, thereby promoting economic growth (Liu et al., 2020; Xu, 2021; Zhang and Li, 2024). Moreover, upgrading the industrial structure improves resource allocation by shifting production factors such as labor and capital from low-value-added, labor-intensive industries to high-value-added, technology- and knowledge-intensive sectors. This shift promotes the growth of high-tech and green industries (Han et al., 2021; Niu S. et al., 2024; Zhang Q. et al., 2023). In addition, urbanization influences regional eco-efficiency through mechanisms such as technological spillovers, acting as a platform for industrial upgrades, and generating scale effects from population concentration (Qin et al., 2021; Xiao et al., 2023; Yao et al., 2021). Furthermore, green innovation helps achieve clean production at the source, reducing resource consumption intensity while maintaining high output. It also minimizes or eliminates pollutants during production or end-of-pipe treatment processes, thus decreasing undesirable outputs (Niu Z. et al., 2024). Another influential factor is trade openness, which has dual effects on eco-efficiency. On one hand, foreign direct investment (FDI) introduces new technologies and management practices to the host country, promoting technological advancement and industrial upgrading that benefit the environment. On the other hand, the involvement in international division of labor can lead to developed nations relocating polluting industries to developing countries, creating a “pollution haven” effect (Gong, 2023). Finally, higher education levels are generally associated with stronger environmental awareness. Educated populations are more likely to practice green consumption and lifestyles and actively participate in environmental policy-making and implementation, thus enhancing regional eco-efficiency (Tong et al., 2021).
In summary, scholars have progressively shifted the focus of research on regional eco-efficiency measurement methods from single-factor evaluations to multi-factor assessments, and from subjective weighting to objective weighting. This shift has contributed to the gradual maturation and refinement of these measurement methods, providing crucial technical support for the quantitative assessment of regional development quality and sustainability. Simultaneously, the academic community has transitioned from static evaluations of eco-efficiency to spatial dynamic assessments covering long time series, thereby uncovering regional disparities in eco-efficiency and their evolving patterns. To further investigate the causes behind these disparities, some scholars have started to focus on the factors influencing regional eco-efficiency, offering a robust theoretical foundation and valuable empirical insights for effectively improving regional eco-efficiency.
2.2 Research on the impact of green finance on regional eco-efficiency
In response to climate change and the global financial crisis, financial geographers have begun to reconsider the role of financial elements in the relationships among the economy, society, and nature. The role of green finance in reducing pollution, enhancing energy efficiency, carbon reduction, promoting green innovation, and fostering green economic growth has attracted significant attention from academia. Although these concepts are not synonymous with eco-efficiency, they all fall under the umbrella of sustainable development, providing valuable insights, methods, and theoretical frameworks for further research. Specifically, multiple empirical studies have demonstrated the positive impact of green finance on environmental efficiency and innovation capabilities. For instance, Chin et al. (2024), Li C. et al. (2022), Numan et al. (2023), and Zhang Z. et al. (2023) found that green finance can effectively reduce environmental pollution and enhance regional environmental efficiency in regions such as the Belt and Road Initiative countries, Mexico, other emerging economies, and China. Similarly, Sun and Chen (2022), Zhang H. et al. (2023), and Wang Z. et al. (2023) conducted empirical research in China and the E-7 economies, revealing that green finance improves regional energy consumption structures and enhances energy efficiency. Furthermore, studies by Al Mamun et al. (2022), Wan et al. (2022), Li Q. et al. (2022), and Umar and Safi (2023) examining 46 countries, 100 developed and emerging economies, G10 countries, and Organisation for Economic Co-operation and Development (OECD) economies, respectively, found that green finance significantly reduces regional carbon emissions. Additionally, Pan et al. (2024) discovered that green finance can significantly stimulate improvements in green technological innovation capabilities by accelerating the flow of research and development talent and capital. Studies by Yu et al. (2021) and Irfan et al. (2022) also showed that green finance effectively alleviates financing constraints and significantly promotes green innovation in China. Moreover, research by Zhang et al. (2021), Huang (2022), and Xu et al. (2023a) indicates that green finance fosters regional green economic growth by inhibiting investments in energy-intensive and high-emission enterprises, optimizing industrial structures, and reducing pollutant emissions.
Certainly, a few scholars have specifically focused on examining the impact of green finance on eco-efficiency. However, there is limited research on the impact of green finance on regional eco-efficiency, and significant discrepancies exist among the conclusions drawn by different scholars. Wang R. et al. (2022) utilized the DEA model to compute the eco-efficiency of Chinese regions and employed the Tobit model for empirical analysis. Their study suggested a nonlinear U–shaped relationship between green finance and regional eco-efficiency in China, indicating that at lower levels of green finance development, improvements in eco-efficiency were not observed. However, once green finance surpassed a certain threshold, it further was found to enhance eco-efficiency. This conclusion was empirically validated by Zhang, W. et al. (2023). On the other hand, divergent findings have been reported by other scholars. Liu et al. (2019) and Huang et al. (2014) employed the Super-SBM model to assess the eco-efficiency of Chinese provinces. Subsequent econometric analyses revealed that the overall impact of green finance development on regional eco-efficiency in China was found no significant statistical relationship. Furthermore, insufficient attention has been paid to the spatial spillover effects of green finance on eco-efficiency. Zhu et al. (2022) identified an inverted U–shaped relationship between green finance and eco-efficiency in resource-based cities in the Yellow River Basin, with surrounding cities showing a U–shaped pattern in spatial spillover effects. In contrast, Ye et al. (2022) reported that the spatial spillover effects of green finance on eco-efficiency were showed no significant spillover in China and the Central region but showed positive spillover effects in the East and negative impacts in the West.
The above research demonstrates that green finance can promote regional eco-efficiency in certain contexts, while in others, it may exert a suppressive effect. However, scholars have not yet explored or explained the potential reasons behind this phenomenon. Furthermore, as financial factors become increasingly mobile, neighboring regions exhibit strong associations and diffusion effects. However, insufficient attention has been paid to the spatial spillover effects of green finance, which may lead to biases in assessing its true impact, ultimately affecting the effectiveness and precision of policy implementation. Therefore, based on the differing conclusions in existing literature regarding the relationship between green finance and regional eco-efficiency, this study proposes the following hypothesis: the impact of green finance on regional eco-efficiency exhibits a nonlinear relationship, which may manifest differently in direct effects and spatial spillover effects. Specifically, the impact of green finance may follow one of the following patterns: both direct effects and spatial spillover effects could either follow a U–shaped or an inverted U–shaped relationship, or one could follow a U–shape while the other follows an inverted U–shape (or vice versa). To test this, the study constructs a spatial econometric model to empirically examine the specific effects of green finance on regional eco-efficiency, and, through mechanism analysis, clearly delineates the underlying mechanisms. This approach aims to fill existing research gaps, contribute to theoretical development, and provide valuable insights for policy formulation.
3 Methods, variable selection, and data sources
3.1 Methods
3.1.1 The Super-SBM model incorporating undesirable outputs
In the literature review, a detailed discussion of regional ecological efficiency measurement methods is provided, focusing on both single-factor and multi-factor evaluation approaches. Among multi-factor methods, the Super-SBM model has gradually become the dominant approach. The DEA model, originally proposed by operations researchers Charnes and Cooper (Charnes et al., 1978), is widely used in assessing efficiency in multi-input, multi-output contexts. However, traditional DEA models have limitations, especially in the evaluation of regional ecological efficiency. These models typically focus on desirable outputs, such as GDP and industrial output, while neglecting undesirable outputs, such as pollution emissions and other negative externalities. This limitation prevents a comprehensive assessment of the environmental burdens associated with economic development, thereby affecting the accuracy and reliability of the evaluations. To address these limitations, Tone (2001) introduced the SBM model, a non-radial efficiency measurement method. In contrast to traditional DEA models, the SBM model simultaneously considers both desirable and undesirable outputs, thereby overcoming DEA’s inability to address environmental pollution and negative externalities. Another key advantage of the SBM model is its ability to effectively handle input and output slacks, ensuring more accurate efficiency assessments that reflect the input-output gaps. Building on the SBM model introduced by Tone (2001) and Tone (2002) extended this framework by incorporating the concept of super-efficiency, drawing on the work of Andersen and Petersen (1993). The innovation of the super-efficiency SBM model lies in its ability to differentiate and rank decision-making units (DMUs) that have already achieved optimal efficiency scores (i.e., efficiency = 1). Traditional SBM models cannot distinguish between these efficient units. The Super-SBM model expands the analysis beyond the original efficiency frontier, enabling a more detailed comparison of highly efficient units based on both desirable and undesirable outputs. This capability makes the Super-SBM model highly significant in ecological efficiency evaluations, providing policymakers with more granular and scientifically grounded insights. It plays a crucial role in promoting green economic transformation, optimizing resource allocation, and achieving sustainable development goals. Therefore, this study employs the Super-SBM model to measure regional eco-efficiency. Consistent with prevailing practices in the academic community, the study posits that each DMU operates under a common production frontier to ensure horizontal comparability among regions. The specific model expressions are as follows:
In Equations 1, 2, ρ denotes regional eco-efficiency, and xmk, yrk, and bhk represent the mth input, rth desirable output, and hth undesirable output of the kth DMU, respectively. M, R, and H denote the number of input, desirable output, and undesirable output indicators, respectively. Sx-, Sy+, and Sb− are their respective relaxation variables. Where, m = 1, 2, … M; r = 1, 2, … R; h = 1, 2, … H; n = 1, 2, … N (n≠k).
3.1.2 Spatial standard deviation ellipse
SSDE is derived from standard deviation analysis in spatial statistics and is used to describe the distribution range, concentration, and directional patterns of spatial data points (Lefever, 1926). Initially applied to study the spatial distribution of geographical phenomena, this method gained prominence with the advancement of geographic information science, particularly with the large-scale accumulation of spatial data and the growing demand for analytical tools. Traditional spatial analysis methods, such as point density analysis and spatial autocorrelation, face limitations when handling data with directional or clustering patterns (Anselin, 1995). These limitations led to the development of the SSDE method, which has become an effective tool for analyzing spatial data distribution. The SSDE method quantitatively captures the directional characteristics of regional economic attributes and their spatial distribution, utilizing key parameters of the spatial statistical ellipse such as area, center, and azimuthal angle (Duman et al., 2023). The advantages of the SSDE method are threefold. First, it is simple and intuitive. By representing spatial distribution with a single ellipse, it effectively conveys the concentration and range of data, especially in cases of concentrated spatial patterns. Compared to other methods, SSDE communicates more spatial information while maintaining clarity. Second, SSDE effectively reveals the directional characteristics of the data, which is particularly useful for analyzing phenomena like urban expansion or financial clustering. Third, SSDE handles data heterogeneity well, adapting to various scales and densities. It avoids the distortion issues that often arise when dealing with heterogeneous data in other methods (Liu Z. et al., 2024; Wang N. et al., 2022). Given these advantages, this study employs SSDE to depict and explore the center of gravity migration path and spatial distribution pattern of green finance and eco-efficiency during the sample period. The expression is:
In Equations 3–5, n denotes the number of provinces, and wi represents the green finance or eco-efficiency index corresponding to province i. (
3.1.3 Spatial Markov chain
In regional development studies, spatial interactions and spillover effects are considered key factors for understanding the relationships between regions and their dynamic changes. Traditional Markov chain models typically assume that state transitions depend solely on a region’s historical state, overlooking the complexity of spatial dependencies and interregional interactions. This assumption presents significant limitations when examining economic activities and resource flows between regions, as it fails to account for the external influences arising from spatial dependencies (Wang Y. et al., 2023). As regional development research has progressed, scholars have increasingly recognized the limitations of traditional Markov chain models in handling spatially structured data, particularly in capturing spatial spillover effects. Therefore, the spatial Markov chain introduces the concept of “spatial lag” based on the traditional Markov chain, addressing the limitations of the conventional Markov chain that neglects spatial spillover effects (Xin et al., 2023). This approach effectively reveals the spatial interaction between a region and its neighboring regions during changes in a specific attribute (Wang Y. et al., 2023). The spatial Markov chain decomposes the k × k traditional Markov chain transition probability matrix into k conditional transition probability matrices, each of size k × k, based on the k types of spatial lag for region a in the initial year. For the kth conditional transition probability matrix, Mkij denotes the probability that a region transitions from state type i at time t to state type j at time t+1, given that the spatial lag type is k. The spatial lag type of a region is classified based on the spatial lag value of its attributes, which is the spatially weighted average of the attribute values of adjacent regions (Lv et al., 2021). The specific formula is as follows:
In Equation 6, Xi denotes an attribute value in the region, Wij denotes the spatial lag weight using the adjacency criterion: if area i and area j are adjacent, the value is 1; otherwise, it is 0.
3.1.4 Spatial Durbin model
In examining the impact of green finance on regional eco-efficiency, accounting for spatial spillover effects is essential. Traditional econometric models tend to focus on the analysis of individual regions, overlooking the interregional interactions and spatial dependencies. However, the First Law of Geography asserts that regions are inherently interconnected, and as financial factors increasingly flow across spatial boundaries, the correlations and diffusion effects between neighboring regions become more significant. This means that changes in green finance within one region can affect not only the eco-efficiency of that region but also spill over into adjacent regions, influencing their eco-efficiency outcomes. Thus, incorporating spatial spillover effects provides a more comprehensive understanding of the relationship between green finance and regional eco-efficiency. Therefore, this study uses the spatial panel Durbin model (SPDM) to examine the spatial spillover effect of green finance on eco-efficiency. Considering the potential nonlinear relationship between the two, we also introduce the quadratic term of green finance. The model is determined as follows:
In Equation 7, Eeit denotes the eco-efficiency index of province i in year t. ρ denotes the spatial lag coefficient. Wij represents the weight matrix. Gfit and Gfit2 represent the green finance index and its squared term for province i in year t, respectively. Zit denotes a series of control variables. β1 and β2 denote the elasticity coefficients of the core explanatory variables and their squared terms, respectively. γ1 and γ2 denote the coefficients for spatial autoregression of the core explanatory variables and their squared terms, respectively. μi denotes the regional effect, and δi denotes the time effect. εi represents a random disturbance term that follows an independent distribution.
Considering that the spatial spillover effect of green finance on eco-efficiency can be influenced by variations in provincial economic development levels and geographical distances, this study uses the economic geography nested matrix (W1) to address the spatial econometric model. Moreover, it incorporates the inverse square geographical distance matrix (W2) and the geographical adjacency matrix (W3) to examine the robustness of the findings. The specific formulas for the three spatial weight matrices used in our analysis are detailed in Supplementary Material.
3.2 Variable selection
3.2.1 Explained variable: eco-efficiency index measurement
This study refers to the existing literature (Sun and Zhou, 2023; Wang R. et al., 2022), selects relevant indicators from the perspective of input and output, and develops a multidimensional evaluation index system for regional eco-efficiency (Table 1). The study has conducted data preprocessing on the relevant input and output indicators to eliminate issues, such as outliers, missing values, and differences in statistical standards, thereby ensuring the accuracy of the results. The total investment in fixed assets is based on the methodology of Shan (2008), taking 2000 as the base period and employing the perpetual inventory method with a depreciation rate of 10.96%. The total energy consumption is calculated by converting the energy used in production and daily life, such as coal, coke, and oil, into standard coal and summing the results. Ultimately, by consolidating the data from the aforementioned indicators and using the Dearun Tools v 3.1.0 software, this study employs the Super-SBM model with nonradial and variable returns to scale to assess the eco-efficiency levels of 30 Chinese provinces from 2009 to 2021.
3.2.2 Core explanatory variable: green finance index measurement
The measurement methods for green finance mainly fall into two categories. The first category, considering the accessibility and representativeness of green finance data, relies solely on green credit as an effective measurement indicator for green finance (Wan S. et al., 2023). The second category involves establishing a green finance indicator system and employing various weighting methods to examine the evaluation subjects comprehensively (Han et al., 2023; Liu et al., 2023). Considering the systemic and multidimensional nature of green finance, the second category of methods is more widely applied. In addition, the methods for calculating the weights of the indicator system mainly include the entropy weight method, principal component analysis, analytic hierarchy process, and combined weighting method. Among these, the entropy weight method determines the weight of each indicator based on the dispersion of sample data, effectively avoiding subjective bias and ensuring objectivity in weight allocation, thereby making it particularly suitable for the measurement of variables within complex indicator systems (Wan Y. et al., 2023; Xu et al., 2023b). The construction of the indicator system has a significant impact the accurate measurement of green finance development levels. Therefore, the study is based on the core concept of green finance outlined in the “Guiding Opinions on Building a Green Financial System” issued by the People’s Bank of China and seven other commissions. It draws upon a large body of existing literature (Guo et al., 2023; He et al., 2019; Lin et al., 2024; Su et al., 2024; Yang et al., 2021; Zhang, 2023; Zhang H. et al., 2022) to select a relatively unified and comprehensive indicator system. The scientific validity and rationality of this indicator system have been reviewed by experts to ensure its accuracy in measuring green finance development levels. Specifically, this study selects nine secondary indicators from five dimensions: green credit, green securities, green investment, green insurance, and carbon finance, to build a green financial evaluation index system (Table 2). The entropy weight method is utilized to measure the level of green finance in each province. The specific explanation for the selection of green finance indicators is provided in Supplementary Material.
3.2.3 Control variables
Considering the influence of other key factors on regional eco-efficiency, the following indicators were selected as control variables based on existing literature:
(1) Industrial structure (Is): represented by the proportion of the tertiary industry’s output value to regional gross domestic product (GDP)
(2) Urbanization rate (Ur): measured by the proportion of the urban population to the total population of the region
(3) Environmental regulation (Er): reflected by the frequency of words related to “environment” in the local government’s work report, expressed as a proportion of the total word frequency in the report
(4) Technological innovation (Rd): expressed by the proportion of research and development expenditure to regional GDP
(5) Trade openness (To): assessed by the proportion of actual foreign direct investment utilization to regional GDP
(6) Environmental awareness (El): measured by the proportion of individuals with a bachelor’s degree or higher among the population aged 6 and above in each province
3.3 Data sources
In July 2007, the issuance of the “Opinions on Implementing Environmental Protection Policies and Regulations to Prevent Credit Risks” officially marked the beginning of green finance practices in China. Therefore, considering the lag associated with policy implementation, the study sets the starting point at 2009. Spatial econometric models require the use of balanced panel data. If any key variable is missing for a province in a given year, the entire sample for that province becomes unusable for all years. Furthermore, there must be no missing data for any variable in each year; otherwise, subsequent research cannot proceed. To ensure scientific rigor and data availability, and to obtain as many provincial samples as possible while ensuring that the data are relatively recent, the study sets the endpoint at 2021. This results in a balanced panel dataset covering 30 provinces in China from 2009 to 2021, excluding Tibet, Hong Kong, Macao, and Taiwan. The sample data are sourced from various publications spanning the years 2008–2022, including the Energy, Environmental, Population and Employment, Industrial, and Economic Statistical Yearbooks, as well as Statistical Yearbooks from individual provinces (municipalities, autonomous regions) and the Wind database. The primary variables used in our analysis, along with their descriptive statistics and multicollinearity tests, are detailed in Supplementary Material.
4 Results and analysis
4.1 Spatiotemporal characteristics of green finance and eco-efficiency
4.1.1 Analysis of temporal evolution characteristics
4.1.1.1 Evolutionary trend of green finance over time
Overall, the level of green finance in China showed a sustained strengthening trend from 2009 to 2021, increasing from 0.121 to 0.251, with an average annual growth rate of 6.269%. These results indicate that the development of green finance progressed rapidly and that there remains significant potential for further increase in the total amount. Currently, it is still in the stage of exploration and catching up. The trends in green finance levels have converged across the three major regions. However, the disparity has increased yearly, with the Eastern region1 having the highest level, followed by the Central region2, and the Western region3 demonstrating the lowest level (Figure 2). The main reasons stem from the fact that following China’s reform and opening-up, the Eastern region spearheaded development, achieving higher economic growth. It possesses more abundant capital investment and a more sophisticated market mechanism, giving it an initial advantage in green finance levels. Also, the Eastern region demonstrates a higher level of industrial transformation and upgrading, better optimization of the energy-consumption structure, and substantial demand for green finance. Consequently, the Eastern region exhibits a dual advantage of high development levels and rapid development speed.
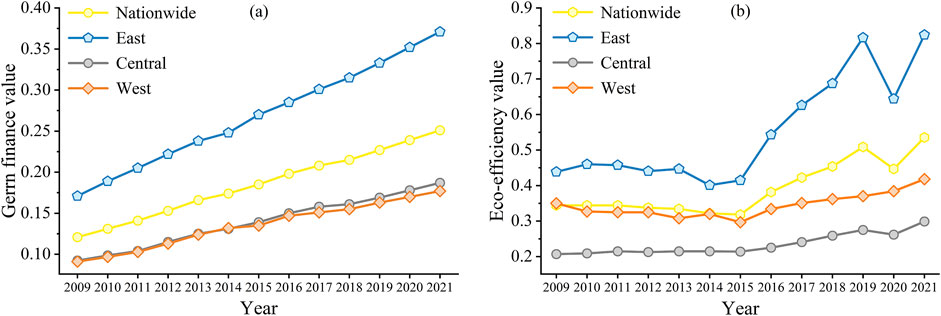
Figure 2. Trends in the levels of green finance (A) and eco-efficiency (B) for China as a whole and its three regions from 2009 to 2021.
4.1.1.2 Evolutionary trend of eco-efficiency over time
From an overall perspective, the eco-efficiency level in China exhibited a fluctuating upward trend from 2009 to 2021, increasing from 0.344 to 0.535, with an average annual growth rate of 3.749%. During the observation period, China’s eco-efficiency level consistently displayed a gradient distribution in the order of East > West > Center, showcasing a prominent leading effect in the Eastern region (Figure 2). The rationale behind these findings lies in the continuous benefits the Eastern region received from national policy support and the dividends of reform and opening-up. As a result, the region developed an advanced and robust industrial system, enabling it to achieve higher economic growth with relatively lower energy consumption. Additionally, its strategic positioning as a forerunner in development contributed to a significant lead in eco-efficiency. The Eastern region enjoyed superior endowments as an energy and raw materials industrial base compared to the Central region. However, challenges such as inadequate human capital and an imperfect industrial structure resulted in a weak capacity for conversion to economic efficiency. The Western region, rich in clean energy sources like wind and solar power, experienced the large-scale migration of high energy–consuming enterprises, leading to an increase in pollutant emissions. Moreover, many provinces in the West lacked development in the deep processing of energy products, hindering the realization of industrial structure upgrades.
4.1.2 Analysis of spatial distribution characteristics
4.1.2.1 Spatial distribution characteristics of green finance
Green finance steadily improved from 2009 to 2021, exhibiting significant spatial heterogeneity, with a clearly distribution pattern in the Eastern region compared to the Central and Western regions. Provinces with a high level of green finance gradually became concentrated, and a spatial agglomeration trend emerged, showing a distribution, primarily along the north–south axis. Overall, the spatial evolution of green finance was characterized by a decreasing gradient from east to west and from south to north (Figure 3). Specifically, in the Eastern region, Beijing served as the center of financial policy in China, while Shanghai was the center of China’s financial market. Both cities were at the forefront of China’s financial reform and opening-up, with relatively well-developed financial market systems and significant policy effects and market performance. Zhejiang and Guangdong were part of China’s green finance reform and innovation pilot zones, where they gained extensive practical experience and held a leading position in green financial infrastructure, market development, and product innovation. Tianjin, Shandong, and Jiangsu leveraged their industrial economic advantages to provide sufficient capital and funding for the development of green finance. Furthermore, the economic structures of these three regions continuously transformed and upgraded, particularly in energy conservation, emission reduction, environmental protection, and renewable energy, which further increased the demand for green financial products and services. In contrast, the Central and Western regions experienced relatively lagging levels of green finance development due to weaker economic and financial development and insufficient policy implementation.
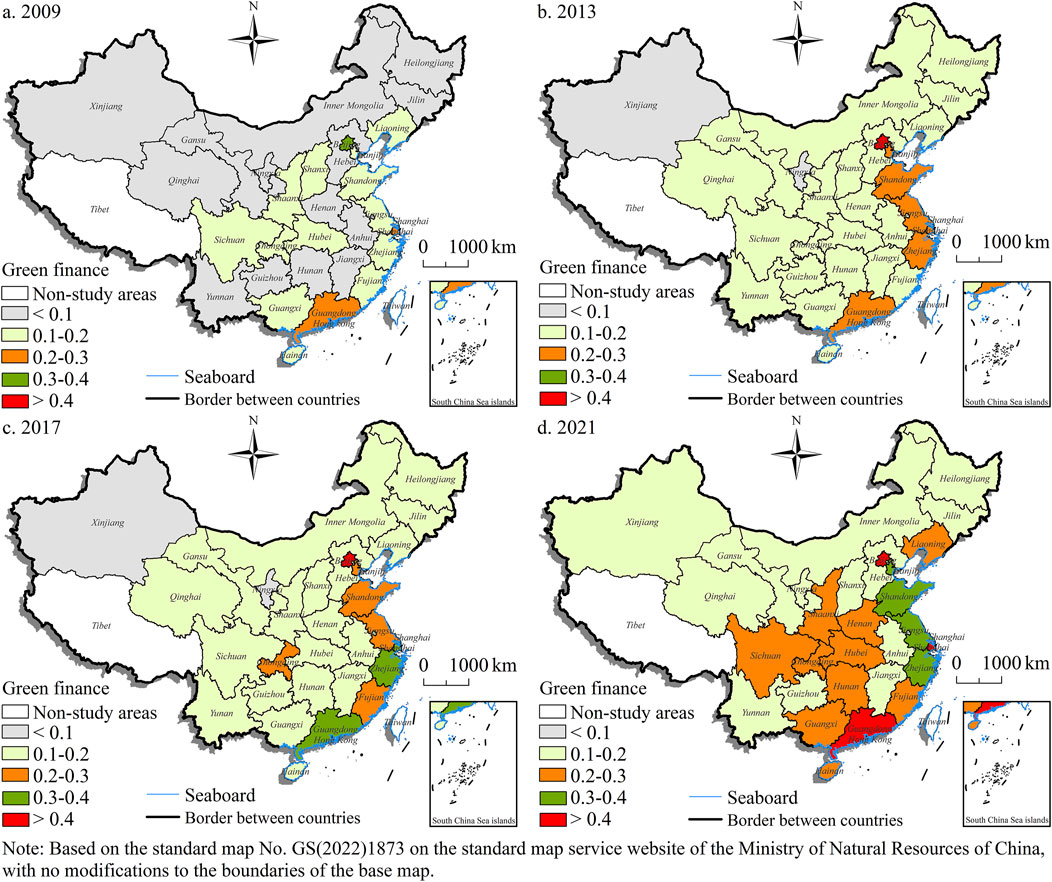
Figure 3. Spatial distribution patterns of Chinese provincial green finance. (A) 2009. (B) 2013. (C) 2017. (D) 2021.
4.1.2.2 Spatial distribution characteristics of eco-efficiency
The eco-efficiency levels experienced continuous enhancement from 2009 to 2021. Initially from the regions of Beijing, Tianjin, Hainan, Qinghai, and Ningxia as relatively high baseline points, this improvement diffused toward the Eastern and Western regions. Eventually, it manifested a spatial distribution pattern characterized by a “med-range depression,” with the highest eco-efficiency in the Eastern region, followed by the Western region, and the lowest in the Central region (Figure 4). As China’s economic structure upgraded, pollution- and resource-intensive industries gradually shifted to the Central and Western regions. In contrast, modern service and high-tech industries characterized by high efficiency and low energy consumption, such as financial services, education, healthcare, and renewable energy, emerged as the economic backbone of the Eastern region. This shift led to an increasing concentration of provinces with high eco-efficiency values in the Eastern region. During the observation period, Qinghai, Ningxia, and Hainan consistently maintained high levels of eco-efficiency. As crucial ecological barriers in China, Qinghai and Ningxia took on the roles of pioneering zones for ecological protection and high-quality development. They actively established ecological civilization demonstration zones and promoted clean energy industries and green organic agriculture, significantly contributing to the development of a green economy. Hainan’s exceptional eco-efficiency was largely attributed to its robust natural ecological foundation, eco-priority policies, and a low-carbon, green industrial structure encompassing tourism, tropical agriculture, and modern services. In contrast, Heilongjiang and Xinjiang faced substantial challenges as they historically relied on heavy industry and resource-based economic models. Although their eco-efficiency improved, they remained at the lower end of the national rankings.
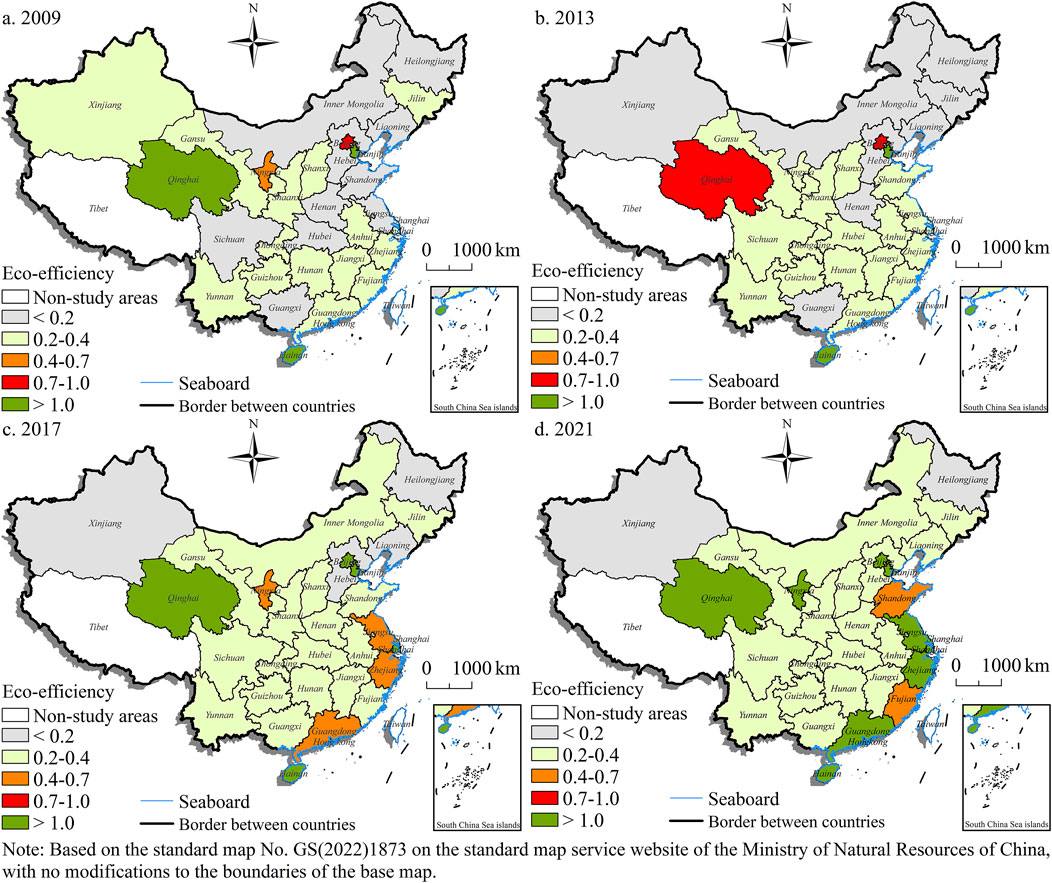
Figure 4. Spatial distribution patterns of Chinese provincial eco-efficiency. (A) 2009. (B) 2013. (C) 2017. (D) 2021.
4.1.3 Analysis of SSDE
4.1.3.1 The SSDE of green finance
From 2009 to 2021, the centroid of green finance shifted in the southeast direction. The overall migration distance was relatively small, approximately 23.945 km. The area of the SSDE for green finance fluctuated, ultimately decreasing by 28,000 km2. This indicated that green finance exhibited a tendency to cluster in its spatial distribution. The azimuth of the green finance ellipse consistently decreased, dropping from 58.060° to 55.919°. This change suggests that relative to the southern provinces in the Eastern region, the northern provinces in the Eastern region exerted an enhanced pulling effect on green finance. The main reason for this was the significant advantages that the Beijing region possessed in financial markets, policy integration, infrastructure, and open cooperation, which exerted a notable clustering effect on the regional development of green finance (Figure 5).
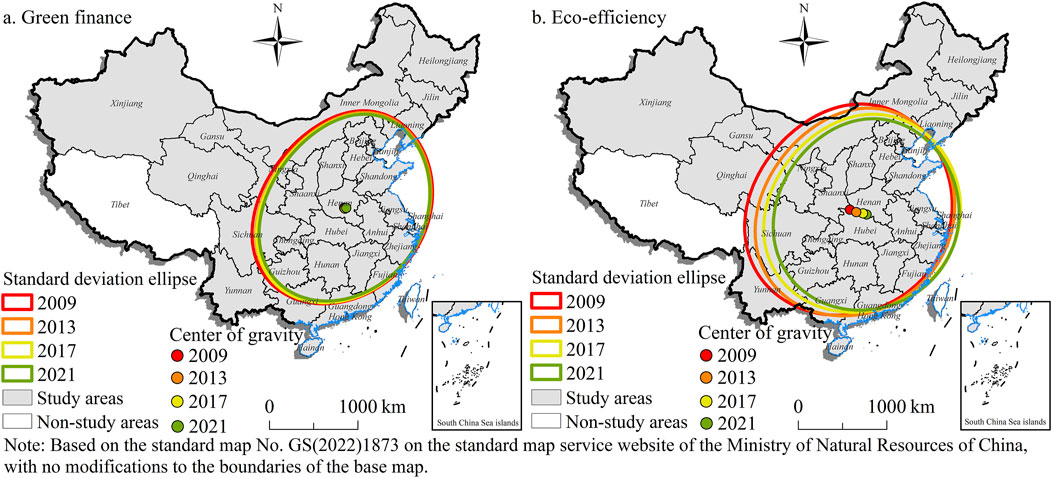
Figure 5. Spatial standard deviation ellipse and centroid migration trajectory of Chinese provincial green finance (A) and eco-efficiency (B) in 2009, 2013, 2017, and 2021.
4.1.3.2 The SSDE of eco-efficiency
From 2009 to 2021, the centroid of eco-efficiency similarly shifted southeastward, passing through Hubei into Henan. It moved with an overall displacement of approximately 196.735 km. Compared with the migration range of green finance, the shift in eco-efficiency was more pronounced. The area of the eco-efficiency ellipse decreased by 73,890 km2, indicating that the clustering effect of eco-efficiency became more pronounced. The azimuth of the eco-efficiency ellipse exhibited a fluctuating trend with an increasing tendency. This change can be mainly divided into two phases: from 2009 to 2013, the azimuth decreased from 71.182° to 66.350°, and then continued to increase to 75.037° by 2021. This suggested that relative to the northern provinces in the Eastern region, the provinces in the southern part had a more substantial pulling effect on eco-efficiency, which was opposite to the direction of influence observed in green finance. The main reasons were that the urban clusters of the Yangtze River Delta and Pearl River Delta vigorously developed the tertiary and high-tech innovation industries, acquiring practical experience and enhancement strategies in ecological civilization construction. This made the two urban clusters stand out in terms of demonstration and leadership effects (Figure 5).
4.1.4 Analysis of spatial Markov chains
This study further employed spatial Markov chains to visually illustrate the dynamic evolution trends and the spatial effects accumulated over time of green finance and eco-efficiency in different provinces. It aimed to explore the internal mobility and stability of green finance and eco-efficiency nationwide, as presented in Table 3. The results of the traditional Markov chain are detailed in Supplementary Material. This study adhered to a method of having roughly equal provinces for each type. The green finance and eco-efficiency levels were categorized into four types—low, relatively low, relatively high, and high—based on quartiles (0.25/0.5/0.75), corresponding to k = 1, 2, 3, and 4. If the types in the initial year were the same as those in the next period, it indicated a “steady” regional transition type. If the regional type improved in the next period, it indicated an “upward transition”; otherwise, it was a “downward transition.”
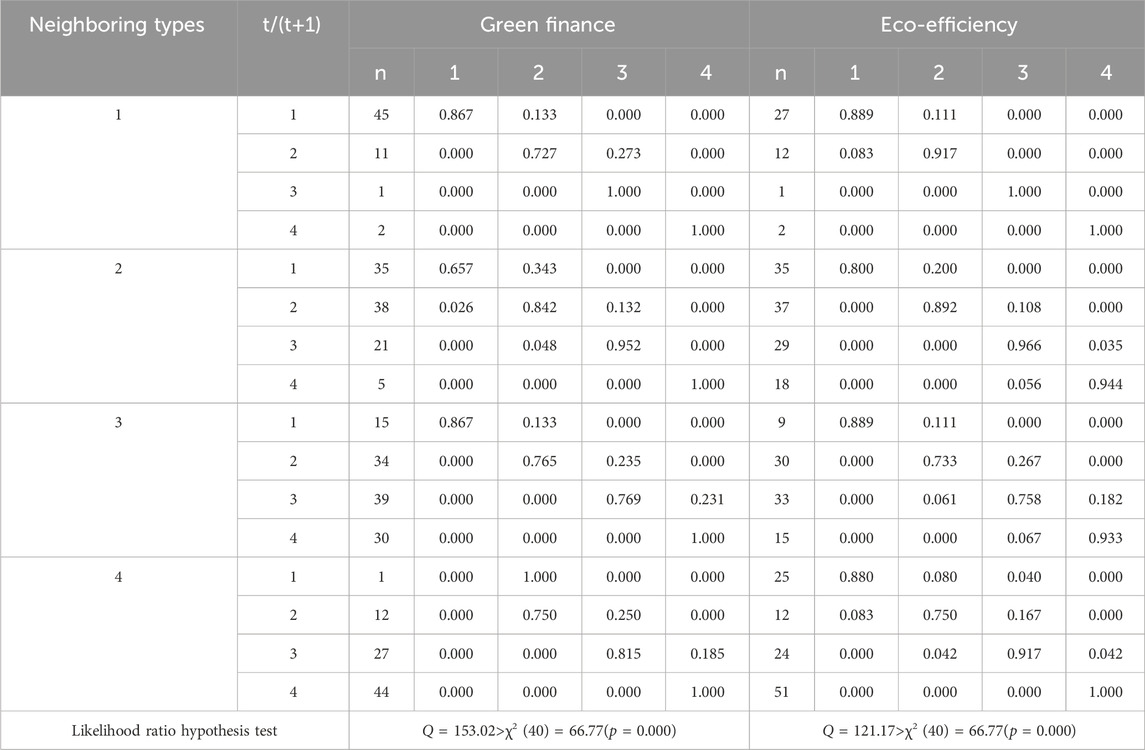
Table 3. Spatial Markov transition probability matrices of green finance and eco-efficiency types from 2009 to 2021.
First, considering spatial lag conditions, there were significant changes in the type transition probabilities for both green finance and eco-efficiency. In addition, based on the likelihood ratio hypothesis test, green finance showed Q = 153.02 > χ2 (40) = 66.77 and eco-efficiency showed Q = 121.17 > χ2 (40) = 66.77, both passing the 1% significance test. This revealed a notable spatial correlation between the provincial types of green finance and eco-efficiency and their neighboring types. Second, green finance and eco-efficiency exhibited spatial spillover effects, where high-performing provinces had a significant positive spillover effect on their neighboring areas. Third, the highest-level types exhibited the most stability. Throughout the period, there was nearly a 100% probability that both green finance and eco-efficiency at the high level would remain unchanged, demonstrating a prominent “club convergence” feature. On the one hand, green finance and eco-efficiency exhibited significant spatial correlation and spillover effects among provinces in China. High-performing provinces influenced lower-performing ones through different mechanisms, such as technological innovation, policy coordination, resource flow, and industrial chain integration, particularly evident in regions including the Beijing–Tianjin–Hebei area, Yangtze River Delta, and Pearl River Delta, where integrated development is actively pursued. On the other hand, the regional development of green finance and eco-efficiency displayed a “club convergence” characteristic, reflecting the imbalance and inadequacy of green development among provinces. The main reason for this lies in the sustained agglomeration effects generated by high-performing provinces, which leveraged their long-term advantages in economic development, policy support, and external exchanges. Conversely, lower-performing provinces struggled to fundamentally break through existing low-efficiency pathways due to a lack of sufficient resources and talent.
4.2 The spatial econometric analysis of green finance on eco-efficiency
4.2.1 Spatial correlation test
This study investigated the spatial correlation between green finance and eco-efficiency before identifying causality. As shown in Table 4, univariate and bivariate Moran’s I values of green finance and eco-efficiency from 2009 to 2021, considering the economic geographical nesting matrix (W1), inverse square geographical distance matrix (W2), and geographical adjacency matrix (W3), were all positive and passed the significance test. First, the univariate Moran’s I values for green finance and eco-efficiency show a strong spatial clustering pattern, suggesting that regions with similar levels of green finance and eco-efficiency are geographically proximate. Over time, this spatial clustering exhibits an upward trend with some fluctuations, ultimately stabilizing, indicating that the regional pattern is persistent and reinforcing. Second, the bivariate Moran’s I values reveal a significant positive spatial dependence between green finance and eco-efficiency, meaning that regions with higher (or lower) levels of green finance generally also have higher (or lower) levels of eco-efficiency, and vice versa. Notably, this spatial dependence initially strengthens before gradually weakening over time. These results provide solid empirical evidence for the spatial effects between green finance and eco-efficiency, highlighting the dynamic evolution of their relationship. Therefore, introducing spatial econometric methods is crucial for further exploring the complex relationship between these two variables, as it allows for a more comprehensive understanding of spatial interactions and spillover effects.
4.2.2 Spatial baseline regression
Based on the LM, LR, Wald, and Hausman test results, this study ultimately employed the SPDM with both time and space fixed effects for spatial econometric analysis, as presented in Table 5. The results of the spatial panel model suitability test are detailed in Supplementary Material. Specifically, the economic geographic nesting matrix (W1) was utilized as the baseline for regression results, whereas the inverse square geographic distance matrix (W2) and geographic adjacency matrix (W3) were used for robustness checks. In addition, for comparison purposes, this study reported the estimation results of the panel fixed effects model (PFEM).
First, in column (1), the quadratic coefficient of green finance was negative and passed the 1% significance test, indicating an inverted U–shaped relationship between green finance and eco-efficiency. Moreover, the “U-test” passed the 1% significance test, suggesting that the turning point of the inverted U–shaped curve was within the range of effective values for green finance, further confirming the nonlinear relationship between green finance and eco-efficiency. Second, considering spatial effects, columns (2) to (4) showed that the quadratic coefficients of green finance and its spatial spillover term all passed the significance test. The relationship between green finance and eco-efficiency still exhibited a significant inverted U–shaped curve. Third, compared with the PFEM, the quadratic coefficients under the SPDM were larger, suggesting that neglecting spatial effects, an essential factor, might have affected the unbiasedness, consistency, and effectiveness of the estimation results. Fourth, the spatial autoregressive coefficient (Spatial rho) of eco-efficiency was significantly positive at the 1% level, indicating that in the spatial dimension, the positive spatial correlation feature of eco-efficiency was significant. There existed a strategic interaction of eco-efficiency “top competition” among provinces.
4.2.3 Spatial effects decomposition
Based on the model estimation (Table 6) using the economic geographic nesting matrix (W1), the direct effect indicated that the coefficient of the quadratic term of green finance was −3.104 and passed the test at a 1% significance level. This suggests that green finance has an inverted U–shaped impact on eco-efficiency. The indirect effect, characterized as the spatial spillover impact, indicated that the coefficient of the quadratic term for green finance was −7.158, passing the 1% significance level. This implies that the spillover influence of green finance on eco-efficiency also exhibits a distinctive inverted U–shaped pattern.
To understand these findings, it's important to look at the development of green finance in China. Initially, green finance injected capital into the green industry through policy support and tax incentives, which helped improve regional eco-efficiency. Since the introduction of China’s “Green Credit Guidelines” in 2012, the country began to establish and enhance its green finance system across multiple dimensions. First, the development of green finance was integrated into national strategies, including the overall plan for ecological civilization reform and the “13th Five-Year Plan.” Second, a systematic approach was employed to advance green finance in various areas, such as credit, bonds, and insurance, constructing a multitiered system of green financial products and markets. Third, distinctive green finance reform and innovation pilot zones were established to explore replicable and scalable practices in institutional mechanisms. Consequently, innovations in green financial products and services continuously emerged, and the scale of the green finance market expanded, effectively directing funds toward the green low-carbon sector and promoting resource efficiency while improving environmental quality. However, as green finance evolved in China, the majority of the green financial product market faced issues, such as irregular development, small scale, and inactive trading, which led to a lack of enthusiasm on the issuance side and insufficient attractiveness on the investment side. For example, in the green bond market, China’s market has experienced fragmented regulation, which led to a lack of uniformity in the issuance standards of green bonds. In addition, significant discrepancies exist between the scope of supported green projects and international standards, thereby creating substantial barriers for overseas investord Market Report 2020’ s, planning to enter the Chinese green bond market. According to the “China Green Bononly 23.8 billion US dollars of the 44.07 billion US dollars” in green bonds issued in China met the definitions of the China and Climate Bonds Initiative, which is just over half of the total issued. Labeled green bonds currently represent less than 1% of the overall bond market in China. In the green credit market, which is the earliest and largest sector in China’s green finance system, there is a lack of stringent information disclosure and multiple external reviews regarding the tracking of postloan fund usage and actual capital flows. This has led to “greenwashing” and “cleanwashing.” Furthermore, support for traditional green projects has become saturated, while emerging green industries and high-carbon sectors still face unmet credit demands. These challenges not only significantly limit the effectiveness of green finance but also lead to issues, such as cost constraints, innovation bubbles, and overinvestment. This, in turn, leads to resource waste, misallocation, and diminishing returns on investment, ultimately hindering improvements in eco-efficiency.
Second, the demonstrative and resource diffusion effects from the early stages of green finance positively influenced the eco-efficiency of neighboring provinces. Provinces across China actively explored governance models and production systems aligned with green finance development. By building on the establishment of green finance reform and innovation pilot zones, they created a set of replicable and adaptable practical experiences and institutional achievements that hold significant demonstrative value. According to the “Report on Local Green Finance Development in China 2022,” by December 2021, provinces across China had held a total of 142 academic or market-related events focused on green finance and established 27 nationally recognized green project databases with local characteristics. Additionally, provinces facilitated the flow of resources such as technology, talent, and information to neighboring areas through initiatives such as green project cooperation, green technology promotion, and green supply chains. For example, Shanghai played a leading role with the national green development fund to promote green development along the Yangtze River Economic Belt; Guangdong established a unified green finance market for the Guangdong–Hong Kong–Macau Greater Bay Area, systematically advancing the two-way opening of the green finance market; Hubei led demonstrations in green technology innovation in the Central region; and Guizhou promoted the construction of a green finance center in the Western region. However, as higher levels of green finance became concentrated within certain provinces, a notable spatial clustering trend emerged, leading to a “siphon effect” on neighboring regions. According to the “Green Finance Development Report under the Dual Carbon Goals 2021,” in 2020, the Eastern region accounted for 68.68% of the cumulative issuance of green bonds, with Guangdong contributing 15.54%. In contrast, the Central and Western regions combined accounted for only 31.32%. Similarly, in the same year, the Eastern region held 87.44% of the green credit market, with Beijing accounting for 46.48% and ranking first nationwide, while the Central and Western regions together made up just 12.56%. This indicates that provinces in the Eastern region, with their higher levels of green finance, attracted a significant share of funding for green credit and green bonds, showcasing a strong financial concentration trend. Furthermore, the green industries in these high-performing provinces benefited from abundant financial resources, while traditional, energy-intensive industries were phased out or relocated to neighboring provinces due to stricter environmental regulations and industrial upgrading. This process led to clear spatial redistribution of industries, where more competitive sectors outcompeted less efficient ones, ultimately hindering eco-efficiency improvements in adjacent provinces. Currently, there is a discernible trend of highly polluting industries spreading from east to west and from coastal to inland regions, with the output value of such industries steadily increasing in Central and Western provinces such as Xinjiang, Sichuan, Shanxi, Hubei, and Anhui. As a result, as green finance development reached a turning point, its direct and spillover effects on eco-efficiency shifted from positive to negative.
4.3 Mechanism analysis
In response to the inverted U–shaped relationship between green finance and regional eco-efficiency in direct and spatial spillover effects, we delve further into the specific mechanisms underlying this phenomenon (Figure 6).
(1) Mechanisms of Direct Effects. First, green finance optimizes the supply-demand dynamics and developmental scale of the green finance market through a multifaceted system of eco-efficient financial products. This continual optimization directs capital in an orderly manner toward green industries. Second, green finance effectively restrains the expansive growth of sectors marked by high energy consumption, elevated pollution levels, and overcapacity sectors. This initiative fosters the research and adoption of clean, low-carbon energy, reducing energy consumption per output unit. Third, the mandatory information disclosure in the green security market and financial institutions’ engagement in green debt financing collectively prompt investors and financial entities to exert a supervisory influence on enterprises. In summary, on the fronts of resource input, economic output, and environmental impact, green finance aims to enhance eco-efficiency through capital allocation, technological enhancement, structural optimization, and business supervision.
However, the “compliance cost theory” argues that the governance costs for businesses arising from environmental regulations increase with regulatory stringency. Green finance, functioning as an environmental regulatory tool, also raises business operational costs. This can exacerbate “greenwashing” and create a “green innovation bubble,” thereby contributing to cost constraints on enterprises’ green development efforts. Furthermore, in some regions, the construction of green financial products and market systems is incomplete, making it challenging to effectively implement and manage large-scale green financial projects. In addition, there is an issue of insufficient innovation in green technologies. The combination of these challenges results in a certain degree of overinvestment in the green finance market, leading to resource waste and imbalance. Ultimately, this hinders the improvement of regional eco-efficiency.
(2) Mechanisms of Spatial Spillover Effects. Green finance exerts a radiating impact on surrounding regions through the efficient flow of production factors. This is exemplified by benefits from green innovation, scale economies from industry clustering, and enhanced information-sharing through green financial platforms. Concurrently, the demonstrative influence of local green finance policies may incentivize neighboring regions to emulate, thereby narrowing or maintaining the eco-efficiency gap with the local region.
Nevertheless, when the level of green finance reaches a threshold, further increases may impede ecological efficiency enhancement in other provinces. On the one hand, areas with higher levels of green finance are better equipped to meet market demands for green products, attracting resource-efficient and environmentally friendly industries from neighboring provinces. On the other hand, green finance restricts the credit rates and loan sizes for polluting enterprises, increasing financing difficulties and costs and leading to the transfer of polluting enterprises to provinces with lower levels of green finance. Consequently, the migration of green industries and the inflow of polluting industries significantly exacerbates pollution output in neighboring areas, hindering ecological efficiency enhancement in such regions.
4.4 Robustness test
Herein, three endogeneity treatment methods and three robustness tests were sequentially implemented to ensure the reliability of the research conclusions, as presented in Supplementary Material.
(1) Instrumental variable (IV). Drawing inspiration from the study by Chen et al. (2024), this research employed the lagged one-period green finance indicator as an instrumental variable.
(2) Dynamic Spatial Panel Durbin Model (DSPDM). This study incorporated lagged terms of green finance and spatial lag terms into the model to construct the DSPDM, addressing endogeneity and estimation bias caused by omitted variables.
(3) Spatial Difference-in-Differences Model. This study considered the green finance reform and innovation pilot zones as a quasi-natural experiment. Policy groups (Post) and treatment groups (Treat) were constructed to analyze the changes in eco-efficiency levels between pilot and nonpilot provinces before and after policy implementation.
(4) The spatial weight matrix was replaced by an inverse geographical distance weight matrix to reflect spatial correlation among provinces.
(5) Ecological civilization pilot provinces and green finance pilot provinces were excluded from the analysis. These pilot provinces may have exhibited differences in support policies, and their exclusion aimed to eliminate potential influences from outliers.
(6) The estimation sample was adjusted. Since 2012, the Chinese economy has entered the “new normal,” reflecting balanced development in the economic environment. Considering the impact of the public health incident in 2019, the sample period was shortened to 2012–2019 to align with the balanced development of the economic environment and to conduct the baseline regression again. The results of several endogeneity treatment and robustness tests show that the regression results remain valid.
4.5 Analysis of spatial heterogeneity
Based on the previous analysis of the spatiotemporal evolution characteristics of green finance and eco-efficiency in China, it was evident that the levels of green finance and eco-efficiency exhibited significant spatial heterogeneity. Therefore, this study further investigated the differential relationship between green finance and eco-efficiency in various regions, as shown in Table 7. The results indicated that only in the Eastern region did green finance exhibit an inverted U–shaped pattern in both its direct effect and spatial spillover effect on eco-efficiency. The coefficient of the quadratic term for green finance was negative and passed the significance test at the 1% level. In contrast, in the Central and Western regions, the impact of green finance on eco-efficiency did not pass the significance test. When applying a full-sample empirical test, it was similarly found that during the study period, most provinces in the Central and Western regions had not yet surpassed the inverted U–shaped turning point for green finance development. These findings indicate that the positive impact of green finance on regional eco-efficiency still has significant potential for improvement and growth in the Central and Western regions.
Several factors contributed to this situation: First, institutional friction. The lack of fiscal resources, talent reserves, and technical support in local governments in the Central and Western regions limited the effectiveness of green finance policies. This slower progress hindered the development of market-driven green finance mechanisms and the establishment of efficient financial capital operations. In provinces like Xinjiang, Gansu, and Jiangxi, where green finance reform and innovation pilot zones were established, the development of green finance remained at a low level, further reflecting the persistent barriers between policy formulation and market practice.
Second, industrial path dependence. The Central and Western regions were dominated by resource-based industries, with traditional high-pollution and high energy–consumption industries playing a crucial role in their economic development. As key sources of revenue and growth, these industries placed significant pressure on local governments during their transition to green finance. Moreover, the large development gap between the Eastern region and the Central and Western regions followed theories of comparative advantage, resource endowment, and industrial gradient transfer. As a result, energy-intensive industries from the more developed Eastern region gradually relocated to the less-developed Central and Western regions, and the higher levels of green finance development in the East accelerated this process. Therefore, green finance faced strong constraints from path dependence in driving industrial upgrades in the Central and Western regions. Although there was substantial demand for green finance, achieving a full green transition in the short term through financial support was challenging.
Third, an underdeveloped capital market. The development of green finance depended on diversified financing tools and a mature capital market. However, China’s capital markets exhibited distinct regional segmentation. The Central and Western regions had fewer financial institutions and shallower capital markets, making it difficult to replicate the financial agglomeration effects observed in the Eastern region. Consequently, these regions were unable to effectively connect local green projects with capital markets, limiting the financing scale of green projects and stifling the expansion of the green finance market.
5 Discussion
The study reveals that both green finance and eco-efficiency levels showed an upward trend, with green finance experiencing a faster average annual growth rate. However, the overall level of green finance remained relatively low. Among the three major regions, the Eastern region consistently demonstrated a dual advantage in both the high level and rapid growth of green finance and eco-efficiency, with a notable leadership effect. In terms of spatial distribution, the evolution of green finance followed a gradient decrease from east to west and from south to north. Eco-efficiency exhibited a spatial distribution pattern of an “intermediate depression,” being highest in the Eastern region, followed by the Western area, and lowest in the Central region. These findings align with the majority of existing research conclusions (Gong, 2023; Liu et al., 2023; Shang et al., 2022; Zhang H. et al., 2022; Zhang Y. et al., 2022). However, most of these studies only utilize line graphs or ArcGIS spatial pattern maps, lacking dynamic representations of spatial distribution.
This study combines the advantages of SSDE, which describes spatial data distribution and heterogeneity, with the benefits of spatial Markov chains, which reveal spatial data evolution and transition patterns. This approach effectively quantifies the multidimensional dynamic evolution of green finance and eco-efficiency. The study finds that the concentration centers for green finance and eco-efficiency have shifted toward the southeast. Compared to the southern provinces in the Eastern region, the northern provinces in the Eastern region have shown an increasing agglomeration effect on the development of green finance, while the opposite is true for eco-efficiency. Additionally, green finance and eco-efficiency demonstrate relatively stable short-term dynamics, making hierarchical leaps difficult to achieve. A degree of polarization and club convergence is also observed. Furthermore, when geographic factors are considered, green finance and eco-efficiency exhibit significant spatial spillover effects.
Through regression analysis, this study finds that the direct impact of green finance on regional eco-efficiency follows an inverted U–shaped pattern, which differs somewhat from the U-shaped pattern identified by other scholars (Wang R. et al., 2022; Zhang W. et al., 2023). This discrepancy may arise from differences in eco-efficiency measurement and regression methodologies. To ensure the reliability of our conclusions, two additional steps were taken. First, robustness tests were conducted using methods such as replacing spatial weight matrices, substituting control variables, excluding policy pilot provinces, and adjusting estimation samples. Second, further analysis revealed that most provincial green finance levels remain on the left side of the turning point in the inverted U–shaped curve. The direct impact of green finance on eco-efficiency is primarily positive, a finding consistent with other scholars’ perspectives (Sun and Chen, 2022; Zhang, H. et al., 2023; Zhang, Z. et al., 2023).
Compared to existing research, this study makes a significant contribution by uncovering an inverted U–shaped pattern in the spatial spillover effects of green finance on eco-efficiency in China. Moreover, most provincial green finance levels have not yet reached the turning point. This is due to the positive effects of local green finance development on eco-efficiency in neighboring areas, such as knowledge spillovers, economies of scale, information sharing, and exemplary followership, which outweigh the negative effects like resource siphoning and the creation of pollution havens. These findings suggest that supporting the construction of ecological civilization in China through the development of green finance is a beneficial strategy. The spatial heterogeneity analysis reveals a significant inverted U–shaped pattern in the impact of green finance on eco-efficiency in the Eastern region, while this relationship lacks significance in the Central and Western areas. A comparison with other studies shows that the conclusions for the Eastern region align more closely with statistical significance tests. In contrast, the Central and Western regions often fail to demonstrate statistical significance or exhibit negative effects (Sun and Chen, 2022; Wang and Wang, 2022; Ye et al., 2022). The conclusion suggests that green finance has played a positive role in improving eco-efficiency in the Eastern region, while there is significant room for improvement in the Central and Western areas.
In summary, this study follows the financial geography research paradigm, focusing on the impact and spatial effects of green finance on regional eco-efficiency. It provides valuable supplements and enhancements to previous research. The findings offer practical insights for addressing real-world issues in China and provide useful references for academia to explore how financial elements can promote regional sustainable development.
6 Conclusion and implications
6.1 Conclusion
Based on the analysis of green finance and eco-efficiency across 30 provincial regions in China from 2009 to 2021, this study reveals the spatiotemporal evolution characteristics of both. It specifically examines the direct effects, spatial spillover effects, and spatial heterogeneity of green finance on eco-efficiency. The results indicate the following: (1) both green finance and eco-efficiency exhibit a fluctuating upward trend, with noticeable spatial clustering and regional disparities. (2) Green finance has a significant direct and spatial spillover effect on eco-efficiency, following an inverted U–shaped pattern, a conclusion that remains robust after endogeneity and robustness tests. (3) Spatial heterogeneity analysis reveals that the inverted U–shaped pattern of green finance’s impact on eco-efficiency is significant only in the Eastern region, while it fails to pass the significance test in the Central and Western regions.
6.2 Suggestions
(1) Based on local development conditions, it is crucial to design tailored development paths for green finance while carefully balancing the goal of maximizing eco-efficiency. In the Eastern region, the marginal benefits of green finance in enhancing regional eco-efficiency are gradually diminishing. Therefore, the focus should shift from expanding scale to improving quality. Specifically, efforts should be made to drive innovation in green financial products and promote efficient resource allocation to accelerate the effective supply of green finance. Strategically, the emphasis should be on building a modern industrial system, fostering technological innovation, and creating new growth drivers to ensure the high-quality circular development of finance, technology, and industry. In terms of development paths, the region should explore innovative pilots such as “green finance + advanced manufacturing” and “green finance + carbon sinks” to build a diversified green financial product system and improve access to financing for green projects.Alternatively, in the Central and Western regions, the role of green finance in promoting regional eco-efficiency needs to be further strengthened. These regions should prioritize the development of green financial markets and accelerate the elimination or transformation of high-pollution, high energy–consuming industries. One key approach involves optimizing green finance policies based on each region’s industrial structure, stage of economic development, and market maturity. This includes refining the implementation requirements for various mechanisms and providing strong institutional support for green finance development. Policy tools like tax incentives, R&D subsidies, and loan guarantees can encourage financial institutions and enterprises to invest more in green projects, fostering the growth of the green finance market. Additionally, financial institutions should offer tailored green credit products, providing preferential loan rates or flexible repayment options for high-pollution, high energy–consuming industries committed to transformation. Innovation is crucial for industrial upgrading, and local governments should support this by promoting partnerships between universities, research institutes, and financial institutions to form green technology innovation consortia, driving significant advancements in these industries.
(2) Comprehensive efforts should be made to promote a strategy for coordinated regional development, fully leveraging the positive spatial spillover effects of green finance on regional eco-efficiency. First, building on the practical experience of green finance reform and innovation pilot zones, it is crucial to gradually expand these pilot programs in a location-specific and steady manner. This approach not only helps to continuously improve green finance levels but also fosters the development of eco-efficiency in the Central and Western regions through a point-to-area strategy. Second, establishing regional cooperation and mutual assistance mechanisms is vital. By harnessing the radiating effects of the Eastern region, initiatives like targeted collaboration, project assistance, industry transfer, and joint park development can accelerate the flow of resources such as capital, technology, talent, and information from the Eastern to the Central and Western regions. Finally, it is important to focus on developing local, high-end, and quality industries. Before and after accepting industry transfers, increasing environmental standards and pollution supervision is essential to prevent the “siphoning effect” of high-value provinces on lower-value ones and the “pollution haven effect” that passes environmental burdens to neighboring areas.
(3) Strengthening risk management and prevention capabilities in green finance is essential for promoting the sustainable development of the green finance market. On one hand, government departments should accelerate the improvement of green finance product standards, clarifying product definitions and supported areas to ensure consistency in the definitions, assessment methods, and certification standards of green projects across different regions and markets. This will reduce policy uncertainty and effectively mitigate policy and market risks. On the other hand, financial institutions should leverage financial technologies, such as big data and blockchain, to establish platforms that align green finance with industry needs, reducing information asymmetry in investment and financing. Additionally, a dynamic monitoring mechanism should be established to regularly evaluate the effectiveness of green finance implementation and promptly adjust support levels. This combined approach of preassessment and postregulation can effectively identify and eliminate projects that do not meet the criteria for green, clean, or low-carbon transitions, enhancing resource allocation efficiency and reducing the risks of “greenwashing” and “cleanwashing.”
6.3 Limitations and prospects
Although this study has provided valuable insights, it has limitations for further research:
(1) This study employs an indicator system to measure green finance and regional eco-efficiency, reflecting the systematic and multidimensional nature of both. However, using a dual indicator system may introduce multicollinearity issues, potentially leading to bias in the empirical analysis. To address this concern, the study references authoritative literature in the field, consults with experts, and implements measures to ensure the scientific validity of the indicator system. Additionally, we employ a series of methods, including variance inflation factors, endogeneity handling, and robustness tests, to guarantee the accuracy and reliability of the empirical results.
(2) Influenced by limitations in data accessibility, this study is conducted at the provincial level. While provincial panel data provide a robust macrolevel understanding of the research theme, it falls short in capturing disparities among prefecture-level cities within the provinces. In subsequent investigations, contingent upon data availability, representative regions should be selected, and analyses should be refined at a smaller scale, such as the municipal or county level.
Data availability statement
The original contributions presented in the study are included in the article/Supplementary Material, further inquiries can be directed to the corresponding author.
Author contributions
YZ: Data curation, Funding acquisition, Investigation, Writing–original draft, Writing–review and editing. JZ: Methodology, Software, Visualization, Writing–original draft, Writing–review and editing. YL: Conceptualization, Writing–review and editing.
Funding
The author(s) declare that financial support was received for the research, authorship, and/or publication of this article. This study is co-funded by the Natural Science Foundation Youth Program of Shandong Province (No: ZR2020QD006) and the Higher Education Institution Youth Entrepreneurship Team Plan of Shandong Province (No: 2023RW091).
Conflict of interest
The authors declare that the research was conducted in the absence of any commercial or financial relationships that could be construed as a potential conflict of interest.
Generative AI statement
The author(s) declare that no Generative AI was used in the creation of this manuscript.
Publisher’s note
All claims expressed in this article are solely those of the authors and do not necessarily represent those of their affiliated organizations, or those of the publisher, the editors and the reviewers. Any product that may be evaluated in this article, or claim that may be made by its manufacturer, is not guaranteed or endorsed by the publisher.
Supplementary material
The Supplementary Material for this article can be found online at: https://www.frontiersin.org/articles/10.3389/fenvs.2024.1506734/full#supplementary-material
Footnotes
1Beijing, Fujian, Guangdong, Hainan, Hebei, Jiangsu, Liaoning, Shandong, Shanghai, Tianjin, Zhejiang.
2Chongqing, Anhui, Heilongjiang, Henan, Hubei, Hunan, Jiangxi, Jilin, Shanxi.
3Gansu, Guangxi, Guizhou, Inner Mongolia, Ningxia, Qinghai, Shaanxi, Sichuan, Xinjiang, Yunnan.
References
Al Mamun, M., Boubaker, S., and Nguyen, D. K. (2022). Green finance and decarbonization: evidence from around the world. Finance Res. Lett. 46, 102807. doi:10.1016/j.frl.2022.102807
Andersen, P., and Petersen, N. C. (1993). A procedure for ranking efficient units in data envelopment analysis. Manage. Sci. 39 (10), 1261–1264. doi:10.1287/mnsc.39.10.1261
Anselin, L. (1995). Local indicators of spatial association—LISA. Geogr. Anal. 27 (2), 93–115. doi:10.1111/j.1538-4632.1995.tb00338.x
Banker, R. D., Charnes, A., and Cooper, W. W. (1984). Some models for estimating technical and scale inefficiencies in data envelopment analysis. Manage. Sci. 30 (9), 1078–1092. doi:10.1287/mnsc.30.9.1078
Block, S., Emerson, J. W., Esty, D. C., de Sherbinin, A., Wendling, Z. A., et al. (2024). 2024 environmental performance index. New Haven, CT, USA: Yale Center for Environmental Law and Policy. Available at: https://epi.yale.edu/measure/2024/EPI.
Charnes, A., Cooper, W. W., and Rhodes, E. (1978). Measuring the efficiency of decision making units. Eur. J. Oper. Res. 2 (6), 429–444. doi:10.1016/0377-2217(78)90138-8
Chen, A. (2008). Evaluation of regional eco-efficiency and an empirical analysis of influencing factors in China: a case study of provincial data from 2000 to 2006. Chin. J. Manag. Sci. 16 (S1), 566–570. doi:10.16381/j.cnki.issn1003-207x.2008.s1.023
Chen, F., Zeng, X., and Guo, X. (2024). Green finance, climate change, and green innovation: evidence from China. Finance Res. Lett. 63, 105283. doi:10.1016/j.frl.2024.105283
Chen, R., Zhang, Q., and Wang, J. (2025). Impact assessment of green finance reform on low-carbon energy transition: evidence from China's pilot zones. Environ. Impact Assess. Rev. 110, 107654. doi:10.1016/j.eiar.2024.107654
Chen, S., Tan, Z., Wang, J., Zhang, L., He, X., and Mu, S. (2023). Spatial and temporal evolution of synergizing the reduction of pollution and carbon emissions and examination on comprehensive pilot effects–evidence from the national eco-industrial demonstration parks in China. Environ. Impact Assess. Rev. 101, 107147. doi:10.1016/j.eiar.2023.107147
Chin, M.-Y., Ong, S.-L., Ooi, D.B.-Y., and Puah, C.-H. (2024). The impact of green finance on environmental degradation in BRI region. Environ. Dev. Sustain. 26 (1), 303–318. doi:10.1007/s10668-022-02709-5
Ci̇ti̇l, M. (2024). Is green finance a prerequisite for green growth of G-20 economies. Innov. Green Dev. 3 (4), 100170. doi:10.1016/j.igd.2024.100170
Cui, S., and Wang, Z. (2023). The impact and transmission mechanisms of financial agglomeration on eco-efficiency: evidence from the organization for economic co-operation and development economies. J. Clean. Prod. 392, 136219. doi:10.1016/j.jclepro.2023.136219
Deng, X., and Gibson, J. (2019). Improving eco-efficiency for the sustainable agricultural production: a case study in Shandong, China. Technol. Forecast. Soc. Change 144, 394–400. doi:10.1016/j.techfore.2018.01.027
Duman, Z., Mao, X., Cai, B., Zhang, Q., Chen, Y., Gao, Y., et al. (2023). Exploring the spatiotemporal pattern evolution of carbon emissions and air pollution in Chinese cities. J. Environ. Manage. 345, 118870. doi:10.1016/j.jenvman.2023.118870
Energy Institute (2024). Statistical review of world energy 2024. Available at: https://www.energyinst.org/statistical-review.
Geng, Q., Wang, Y., and Wang, X. (2023). The impact of natural resource endowment and green finance on green economic efficiency in the context of COP26. Resour. Policy. 80, 103246. doi:10.1016/j.resourpol.2022.103246
Gong, W. (2023). A study on the effects of natural resource abundance and foreign direct investment on regional eco-efficiency in China under the target of COP26. Resour. Policy. 82, 103529. doi:10.1016/j.resourpol.2023.103529
Guo, Q.-t., Dong, Y., Feng, B., and Zhang, H. (2023). Can green finance development promote total-factor energy efficiency? Empirical evidence from China based on a spatial Durbin model. Energy Policy 177, 113523. doi:10.1016/j.enpol.2023.113523
Han, X., Wang, Y., Yu, W., and Xia, X. (2023). Coupling and coordination between green finance and agricultural green development: evidence from China. Finance Res. Lett. 58, 104221. doi:10.1016/j.frl.2023.104221
Han, Y., and Deng, M. (2020). Spatio-temporal evolution of eco-efficiency and influencing factors of central plains urban agglomeration. Acta Ecol. Sin. 40 (14), 4774–4784. doi:10.16381/j.cnki.issn1003-207x.2008.s1.023
Han, Y., Zhang, F., Huang, L., Peng, K., and Wang, X. (2021). Does industrial upgrading promote eco-efficiency? ─A panel space estimation based on Chinese evidence. Energy Policy 154, 112286. doi:10.1016/j.enpol.2021.112286
He, L., Liu, R., Zhong, Z., Wang, D., and Xia, Y. (2019). Can green financial development promote renewable energy investment efficiency? A consideration of bank credit. Renew. Energy 143, 974–984. doi:10.1016/j.renene.2019.05.059
Hu, M., Sima, Z., Chen, S., and Huang, M. (2023). Does green finance promote low-carbon economic transition? J. Clean. Prod. 427, 139231. doi:10.1016/j.jclepro.2023.139231
Huang, H., Hu, Q., and Qiao, X. (2018). Changes of eco-efficiency under green GDP and ecological footprints: a dynamic analysis in Jiangxi Province. Shengtai Xuebao/Acta Ecol. Sin. 38, 5473–5484. doi:10.5846/stxb201708151464
Huang, J., Lv, H., and Wang, L. (2014). Mechanism of financial development influencing regional green development: based on eco-efficiency and spatial econometrics. Geogr. Res. 33 (03), 532–545. doi:10.11821/dlyj201403012
Huang, S.-Z. (2022). Do green financing and industrial structure matter for green economic recovery? Fresh empirical insights from Vietnam. Econ. Analysis Policy 75, 61–73. doi:10.1016/j.eap.2022.04.010
Huppes, G., and Ishikawa, M. (2005). A framework for quantified eco-efficiency analysis. J. Ind. Ecol. 9 (4), 25–41. doi:10.1162/108819805775247882
Irfan, M., Razzaq, A., Sharif, A., and Yang, X. (2022). Influence mechanism between green finance and green innovation: exploring regional policy intervention effects in China. Technol. Forecast. Soc. Change 182, 121882. doi:10.1016/j.techfore.2022.121882
Jiang, T., Cao, C., Lei, L., Hou, J., Yu, Y., and Jahanger, A. (2023). Temporal and spatial patterns, efficiency losses and impact factors of energy mismatch in China under environmental constraints. Energy 282, 128875. doi:10.1016/j.energy.2023.128875
Jollands, N., Lermit, J., and Patterson, M. (2004). Aggregate eco-efficiency indices for New Zealand—a principal components analysis. J. Environ. Manage. 73 (4), 293–305. doi:10.1016/j.jenvman.2004.07.002
Kuosmanen, T. (2005). Measurement and analysis of eco-efficiency: an economist's perspective. J. Ind. Ecol. 9 (4), 15–18. doi:10.1162/108819805775248025
Lefever, D. W. (1926). Measuring geographic concentration by means of the standard deviational ellipse. Am. J. Sociol. 32 (1), 88–94. doi:10.1086/214027
Li, C. (2023). The impact of green finance on high-quality economic development. J. Zhongnan Univ. Econ. Law (2), 65–77. doi:10.19639/j.cnki.issn1003-5230.2023.0014
Li, C., Sampene, A. K., Agyeman, F. O., Brenya, R., and Wiredu, J. (2022). The role of green finance and energy innovation in neutralizing environmental pollution: empirical evidence from the MINT economies. J. Environ. Manage. 317, 115500. doi:10.1016/j.jenvman.2022.115500
Li, Q., Sharif, A., Razzaq, A., and Yu, Y. (2022). Do climate technology, financialization, and sustainable finance impede environmental challenges? Evidence from G10 economies. Technol. Forecast. Soc. Change 185, 122095. doi:10.1016/j.techfore.2022.122095
Li, W., and Wang, X. (2019). Towards a mega-regional economy: a financial geography perspective to understanding Shanghai’s role in the Yangtze River Delta integration. Geoforum 101, 1–9. doi:10.1016/j.geoforum.2019.02.016
Li, X., Liu, Z., Lin, Y., Jiang, Z., and Cai, L. (2022). Eco-efficiency measurement and spatial-temporal characteristics analysis of county-scale in Shandong Province. World Reg. Stud. 31 (1), 120–129. doi:10.3969/j.issn.1004-9479.2022.01.2020165
Liang, L., Chen, M., Luo, X., and Xian, Y. (2021). Changes pattern in the population and economic gravity centers since the Reform and Opening up in China: the widening gaps between the South and North. J. Clean. Prod. 310, 127379. doi:10.1016/j.jclepro.2021.127379
Lin, J., Zhang, L., and Dong, Z. (2024). Exploring the effect of green finance on green development of China’s energy-intensive industry—a spatial econometric analysis. Resour. Environ. Sustain. 16, 100159. doi:10.1016/j.resenv.2024.100159
Liu, F., Xia, Z., and Lee, C.-C. (2024). Does green credit benefit the clean energy technological innovation and how? The policy catering behavior of enterprises. J. Clean. Prod. 444, 141256. doi:10.1016/j.jclepro.2024.141256
Liu, R., Wang, D., Zhang, L., and Zhang, L. (2019). Can green financial development promote regional ecological efficiency? A case study of China. Nat. Hazard. 95 (1), 325–341. doi:10.1007/s11069-018-3502-x
Liu, Y. (2010). Study on the relationship between land use degree and regional ecological efficiency in Jiangsu Province. China Land Sci. 24 (04), 19–24. doi:10.13708/j.cnki.cn11-2640.2010.04.004
Liu, Y., Zhao, C., Dong, K., Wang, K., and Sun, L. (2023). How does green finance achieve urban carbon unlocking? Evidence from China. Urban Clim. 52, 101742. doi:10.1016/j.uclim.2023.101742
Liu, Y., Zhu, J., Li, E. Y., Meng, Z., and Song, Y. (2020). Environmental regulation, green technological innovation, and eco-efficiency: the case of Yangtze river economic belt in China. Technol. Forecast. Soc. Change 155, 119993. doi:10.1016/j.techfore.2020.119993
Liu Z, Z., Han, L., and Liu, M. (2024). High-resolution carbon emission mapping and spatial-temporal analysis based on multi-source geographic data: a case study in Xi'an City, China. Environ. Pollut. 361, 124879. doi:10.1016/j.envpol.2024.124879
Luo, G., and Wang, X. (2023). Spatial association network structure of eco-efficiency and its influencing factors: evidence from the Beijing-Tianjin-Hebei region in China. Ecol. Modell. 475, 110218. doi:10.1016/j.ecolmodel.2022.110218
Lv, C., Bian, B., Lee, C.-C., and He, Z. (2021). Regional gap and the trend of green finance development in China. Energy Econ. 102, 105476. doi:10.1016/j.eneco.2021.105476
Niu, S., Zhang, K., Zhang, J., and Feng, Y. (2024). How does industrial upgrading affect urban ecological efficiency? New evidence from China. Emerg. Mark. Finance Trade 60 (5), 899–920. doi:10.1080/1540496X.2023.2260544
Niu, Z., Yan, C., and Tan, F. (2024). Green innovation and eco-efficiency: interaction between society and environment of sustainable development demonstration belt in China. Environ. Technol. Innov. 34, 103620. doi:10.1016/j.eti.2024.103620
Numan, U., Ma, B., Sadiq, M., Bedru, H. D., and Jiang, C. (2023). The role of green finance in mitigating environmental degradation: empirical evidence and policy implications from complex economies. J. Clean. Prod. 400, 136693. doi:10.1016/j.jclepro.2023.136693
Ouyang, H., Guan, C., and Yu, B. (2023). Green finance, natural resources, and economic growth: theory analysis and empirical research. Resour. Policy. 83, 103604. doi:10.1016/j.resourpol.2023.103604
Pan, M., Xie, Q., and Cui, R. (2024). Research on the impact of green finance on green technology innovation from the perspective of resource allocation. Econ. issues (4), 52–59. doi:10.16011/j.cnki.jjwt.2024.04.006
Qin, M., Sun, M., and Li, J. (2021). Impact of environmental regulation policy on ecological efficiency in four major urban agglomerations in eastern China. Ecol. Indic. 130, 108002. doi:10.1016/j.ecolind.2021.108002
Quariguasi Frota Neto, J., Walther, G., Bloemhof, J., van Nunen, J. A. E. E., and Spengler, T. (2009). A methodology for assessing eco-efficiency in logistics networks. Eur. J. Oper. Res. 193 (3), 670–682. doi:10.1016/j.ejor.2007.06.056
Ren, Y., Fang, C., and Lin, X. (2017). Evaluation of eco-efficiency of four major urban agglomerations in eastern coastal area of China. Acta Geogr. Sin. 72 (11), 2047–2063. doi:10.11821/dlxb201711010
Ren, Y., Fang, C., Lin, X., Sun, S., Li, G., and Fan, B. (2019). Evaluation of the eco-efficiency of four major urban agglomerations in coastal eastern China. J. Geogr. Sci. 29 (8), 1315–1330. doi:10.1007/s11442-019-1661-7
Robaina-Alves, M., Moutinho, V., and Macedo, P. (2015). A new frontier approach to model the eco-efficiency in European countries. J. Clean. Prod. 103, 562–573. doi:10.1016/j.jclepro.2015.01.038
Seppäläa, J., Melanen, M., Mäenpää, I., Koskela, S., Tenhunen, J., and Hiltunen, M.-R. (2005). How can the eco-efficiency of a region be measured and monitored? J. Ind. Ecol. 9 (4), 117–130. doi:10.1162/108819805775247972
Shan, H. (2008). Reestimating the capital stock of China: 1952-2006. J. Quantitative and Tech. Econ. 25 (10), 17–31. doi:10.13653/j.cnki.jqte.2008.10.003
Shang, H., Jiang, L., Pan, X., and Pan, X. (2022). Green technology innovation spillover effect and urban eco-efficiency convergence: evidence from Chinese cities. Energy Econ. 114, 106307. doi:10.1016/j.eneco.2022.106307
Shao, H., Peng, Q., Zhou, F., and Wider, W. (2024). Environmental regulation, industrial transformation, and green economy development. Front. Environ. Sci. 12, 1442072. doi:10.3389/fenvs.2024.1442072
Shi, D., and Shi, X. (2022). Green finance and high-quality economic development:mechanism, characteristics and empirical study. Stat. Res. 39 (1), 31–48. doi:10.19343/j.cnki.11-1302/c.2022.01.003
Song, J., and Chen, X. (2019). Eco-efficiency of grain production in China based on water footprints: a stochastic frontier approach. J. Clean. Prod. 236, 117685. doi:10.1016/j.jclepro.2019.117685
Su, X., Qiao, R., and Xu, S. (2024). Impact of green finance on carbon emissions and spatial spillover effects: empirical evidence from China. J. Clean. Prod. 457, 142362. doi:10.1016/j.jclepro.2024.142362
Sun, C., Fu, L., Jiang, B., Wang, Y., and Zhu, Z. (2023). Distribution dynamics and regional differences of eco-efficiency development in China. Acta Ecol. Sin. 43 (4), 1366–1379. doi:10.5846/stxb202108022107
Sun, H., and Chen, F. (2022). The impact of green finance on China's regional energy consumption structure based on system GMM. Resour. Policy. 76, 102588. doi:10.1016/j.resourpol.2022.102588
Sun, J., and Zhou, T. (2023). Urban shrinkage and eco-efficiency: the mediating effects of industry, innovation and land-use. Environ. Impact Assess. Rev. 98, 106921. doi:10.1016/j.eiar.2022.106921
Tan, J., and Wang, R. (2021). Research on evaluation and influencing factors of regional ecological efficiency from the perspective of carbon neutrality. J. Environ. Manage. 294, 113030. doi:10.1016/j.jenvman.2021.113030
Tone, K. (2001). A slacks-based measure of efficiency in data envelopment analysis. Eur. J. Oper. Res. 130 (3), 498–509. doi:10.1016/S0377-2217(99)00407-5
Tone, K. (2002). A slacks-based measure of super-efficiency in data envelopment analysis. Eur. J. Oper. Res. 143 (1), 32–41. doi:10.1016/S0377-2217(01)00324-1
Tong, Y., Zhou, H., and Jiang, L. (2021). Exploring the transition effects of foreign direct investment on the eco-efficiency of Chinese cities: based on multi-source data and panel smooth transition regression models. Ecol. Indic. 121, 107073. doi:10.1016/j.ecolind.2020.107073
Umar, M., and Safi, A. (2023). Do green finance and innovation matter for environmental protection? A case of OECD economies. Energy Econ. 119, 106560. doi:10.1016/j.eneco.2023.106560
Wan, Q., Qian, J., Baghirli, A., and Aghayev, A. (2022). Green finance and carbon reduction: implications for green recovery. Econ. Analysis Policy 76, 901–913. doi:10.1016/j.eap.2022.09.022
Wan, S., Lee, Y. H., and Sarma, V. J. (2023). Is Fintech good for green finance? Empirical evidence from listed banks in China. Econ. Analysis Policy 80, 1273–1291. doi:10.1016/j.eap.2023.10.019
Wang, C., Gong, W., Zhao, M., Zhou, Y., and Zhao, Y. (2023). Spatio-temporal evolution characteristics of eco-efficiency in the Yellow River Basin of China based on the super-efficient SBM model. Environ. Sci. Pollut. Res. Int. 30 (28), 72236–72247. doi:10.1007/s11356-023-27363-w
Wang, N., Fu, X., and Wang, S. (2022). Spatial-temporal variation and coupling analysis of residential energy consumption and economic growth in China. Appl. Energy. 309, 118504. doi:10.1016/j.apenergy.2021.118504
Wang, R., Zhao, X., and Zhang, L. (2022). Research on the impact of green finance and abundance of natural resources on China's regional eco-efficiency. Resour. Policy. 76, 102579. doi:10.1016/j.resourpol.2022.102579
Wang, Y., Chen, F., Wei, F., Yang, M., Gu, X., Sun, Q., et al. (2023). Spatial and temporal characteristics and evolutionary prediction of urban health development efficiency in China: based on super-efficiency SBM model and spatial Markov chain model. Ecol. Indic. 147, 109985. doi:10.1016/j.ecolind.2023.109985
Wang, Y., Sun, M., Wang, R., and Lou, F. (2015). Promoting regional sustainability by eco-province construction in China: a critical assessment. Ecol. Indic. 51, 127–138. doi:10.1016/j.ecolind.2014.07.003
Wang, Z., and Wang, X. (2022). Research on the impact of green finance on energy efficiency in different regions of China based on the DEA-Tobit model. Resour. Policy. 77, 102695. doi:10.1016/j.resourpol.2022.102695
Wang, Z., Yao-Ping Peng, M., Anser, M. K., and Chen, Z. (2023). Research on the impact of green finance and renewable energy on energy efficiency: the case study E−7 economies. Renew. Energy 205, 166–173. doi:10.1016/j.renene.2022.12.077
Wan Y., Y., Sheng, N., Wei, X., and Su, H. (2023). Study on the spatial spillover effect and path mechanism of green finance development on China's energy structure transformation. J. Clean. Prod. 415, 137820. doi:10.1016/j.jclepro.2023.137820
Xiao, S., Liang, Q., and Li, J. (2023). An analysis of the spatial spillover and heterogeneity of urbanization to urban eco-efficiency. J. Dalian Univ. Technol. Soc. Sci. 44 (1), 50–62. doi:10.19525/j.issn1008-407x.2023.01.006
Xin, X., Lyu, L., and Zhao, Y. (2023). Dynamic evolution and trend prediction of multi-scale green innovation in China. Geogr. Sustain. 4 (3), 222–231. doi:10.1016/j.geosus.2023.05.001
Xu, C. (2021). Research on industrial structure adjustment and its ecological effects under environmental regulation. Peking: Economic Science Press.
Xu, J., She, S., Gao, P., and Sun, Y. (2023a). Role of green finance in resource efficiency and green economic growth. Resour. Policy. 81, 103349. doi:10.1016/j.resourpol.2023.103349
Xu, J., Wang, J., Li, R., and Gu, M. (2023b). Is green finance fostering high-quality energy development in China? A spatial spillover perspective. Energy Strategy Rev. 50, 101201. doi:10.1016/j.esr.2023.101201
Yan, L., Zhang, X., Pan, H., Wu, J., Lin, L., Zhang, Y., et al. (2021). Progress of Chinese ecological civilization construction and obstacles during 2003–2020: implications from one set of emergy-based indicator system. Ecol. Indic. 130, 108112. doi:10.1016/j.ecolind.2021.108112
Yang, L., and Yang, Y. (2019). Evaluation of eco-efficiency in China from 1978 to 2016: based on a modified ecological footprint model. Sci. Total Environ. 662, 581–590. doi:10.1016/j.scitotenv.2019.01.225
Yang, Y., Su, X., and Yao, S. (2021). Nexus between green finance, fintech, and high-quality economic development: empirical evidence from China. Resour. Policy. 74, 102445. doi:10.1016/j.resourpol.2021.102445
Yao, J., Xu, P., and Huang, Z. (2021). Impact of urbanization on ecological efficiency in China: an empirical analysis based on provincial panel data. Ecol. Indic. 129, 107827. doi:10.1016/j.ecolind.2021.107827
Ye, T., Xiang, X., Ge, X., and Yang, K. (2022). Research on green finance and green development based eco-efficiency and spatial econometric analysis. Sustainability 14 (5), 2825. doi:10.3390/su14052825
Yin, K., Wang, R., Yao, L., and Liang, J. (2011). The eco-efficiency evaluation of the model city for environmental protection in China. Acta Ecol. Sin. 31 (19), 5588–5598. Available at: https://www.ecologica.cn/stxb/article/abstract/stxb201106200860.
Yu, C.-H., Wu, X., Zhang, D., Chen, S., and Zhao, J. (2021). Demand for green finance: resolving financing constraints on green innovation in China. Energy Policy 153, 112255. doi:10.1016/j.enpol.2021.112255
Yu, W., Chen, T., Yu, S., and Wang, H. (2020). Study on spatial-temporal distribution and dynamic evolution of eco-efficiency in China's coastal provinces. J. Coast. Res. 106 (sp1), 454–458. doi:10.2112/SI106-102.1
Yu, Y., Chen, D., Zhu, B., and Hu, S. (2013). Eco-efficiency trends in China, 1978–2010: decoupling environmental pressure from economic growth. Ecol. Indic. 24, 177–184. doi:10.1016/j.ecolind.2012.06.007
Zeng, X., Ma, Y., Ren, J., and He, B. (2023). Assessing the network characteristics and structural effects of eco-efficiency: a case study in the urban agglomerations in the middle reaches of Yangtze River, China. Ecol. Indic. 150, 110169. doi:10.1016/j.ecolind.2023.110169
Zhang, B., Bi, J., Fan, Z., Yuan, Z., and Ge, J. (2008). Eco-efficiency analysis of industrial system in China: a data envelopment analysis approach. Ecol. Econ. 68 (1), 306–316. doi:10.1016/j.ecolecon.2008.03.009
Zhang, G. (2023). The heterogeneous role of green finance on industrial structure upgrading - based on spatial spillover perspective. Finance Res. Lett. 58, 104596. doi:10.1016/j.frl.2023.104596
Zhang, H., Duan, Y., Yang, J., Han, Z., and Wang, H. (2023). Can green finance improve China's haze pollution reduction? The role of energy efficiency. Environ. Dev. 45, 100833. doi:10.1016/j.envdev.2023.100833
Zhang, H., Geng, C., and Wei, J. (2022). Coordinated development between green finance and environmental performance in China: the spatial-temporal difference and driving factors. J. Clean. Prod. 346, 131150. doi:10.1016/j.jclepro.2022.131150
Zhang, Q., and Li, J. (2024). Spatial and temporal changes and influencing factors of urban ecological efficiency in the Yellow River Basin. Front. Environ. Sci. 12. doi:10.3389/fenvs.2024.1435331
Zhang, Q., Tang, X., Xiao, Y., Xiang, X., and Huang, H. (2023). Coordination of industrial structure and eco-efficiency in ecologically fragile areas: a case study of the Loess Plateau, China. J. Environ. Manage. 331, 117237. doi:10.1016/j.jenvman.2023.117237
Zhang, S., Wu, Z., Wang, Y., and Hao, Y. (2021). Fostering green development with green finance: an empirical study on the environmental effect of green credit policy in China. J. Environ. Manage. 296, 113159. doi:10.1016/j.jenvman.2021.113159
Zhang, T., and Zhao, F. (2024). A study on the relationships among green finance, environmental pollution and economic development. Energy Strategy Rev. 51, 101290. doi:10.1016/j.esr.2023.101290
Zhang, W., He, X., and Liu, X. (2023). Does green finance improve the industrial eco-efficiency in China? Environ. Sci. Pollut. Res. 30 (6), 14484–14496. doi:10.1007/s11356-022-23147-w
Zhang, Y., Bawuerjiang, R., Lu, M., Li, Y., and Wang, K. (2024). Green finance and environmental pollution: evidence from China. Econ. Analysis Policy 84, 98–110. doi:10.1016/j.eap.2024.08.022
Zhang, Y., Wang, L., Tang, Z., Zhang, K., and Wang, T. (2022). Spatial effects of urban expansion on air pollution and eco-efficiency: evidence from multisource remote sensing and statistical data in China. J. Clean. Prod. 367, 132973. doi:10.1016/j.jclepro.2022.132973
Zhang, Z., Fu, H., Xie, S., Cifuentes-Faura, J., and Urinov, B. (2023). Role of green finance and regional environmental efficiency in China. Renew. Energy 214, 407–415. doi:10.1016/j.renene.2023.05.076
Zhao, X., Benkraiem, R., Abedin, M. Z., and Zhou, S. (2024). The charm of green finance: can green finance reduce corporate carbon emissions? Energy Econ. 134, 107574. doi:10.1016/j.eneco.2024.107574
Zhao, Y., and Li, R. (2023). Research on eco-efficiency measurement, spatiotemporal analysis and prosperity warning based on the three-stage chain network SBM and MS-DDFM. Heliyon 9 (2), e13079. doi:10.1016/j.heliyon.2023.e13079
Zhu, M., Wang, K., and Tang, H. (2022). Study on the spatial spillover effect of green financial development on eco-efficiency:A case study of resource-based cities in the Yellow River Basin. J. Financial Dev. Res. 484 (04), 55–62. doi:10.19647/j.cnki.37-1462/f.2022.04.009
Keywords: green finance, eco-efficiency, inverted U-shaped, spatial evolution characteristics, spatial spillover effects
Citation: Zhang Y, Zhang J and Liu Y (2024) Does green finance promote the improvement of regional eco-efficiency? Direct and spillover effects. Front. Environ. Sci. 12:1506734. doi: 10.3389/fenvs.2024.1506734
Received: 06 October 2024; Accepted: 25 November 2024;
Published: 06 December 2024.
Edited by:
Mobeen Ur Rehman, Keele University, United KingdomCopyright © 2024 Zhang, Zhang and Liu. This is an open-access article distributed under the terms of the Creative Commons Attribution License (CC BY). The use, distribution or reproduction in other forums is permitted, provided the original author(s) and the copyright owner(s) are credited and that the original publication in this journal is cited, in accordance with accepted academic practice. No use, distribution or reproduction is permitted which does not comply with these terms.
*Correspondence: Jisong Zhang, emhhbmdqaXNvbmdobnh5QDE2My5jb20=