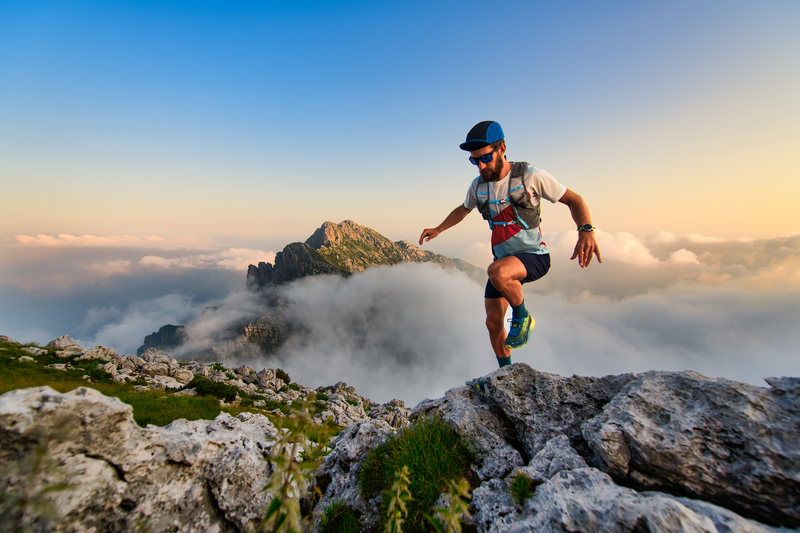
95% of researchers rate our articles as excellent or good
Learn more about the work of our research integrity team to safeguard the quality of each article we publish.
Find out more
ORIGINAL RESEARCH article
Front. Environ. Sci. , 24 October 2024
Sec. Land Use Dynamics
Volume 12 - 2024 | https://doi.org/10.3389/fenvs.2024.1486596
China is undergoing rapid urbanization, which brings about drastic land cover changes and thus has an important impact on land carbon stocks. Therefore, it is of great significance to study the driving factors of land cover changes in typical cities and simulate their carbon stocks in multiple scenarios, in order to promote the development of sustainable use of land resources and to achieve the goal of “dual-carbon.” In this study, based on the synergistic relationship between land cover and carbon stock (CS), a coupled modeling framework based on MOP-FLUS-InVEST (MFI) is proposed, which integrates the advantages of three models: targeted optimization of the land cover (LC) structure, patch-level simulation of the layout, and rapid probing of spatial and temporal evolutions of CS. In addition, based on the 30 m resolution surface cover data, we analyzed the land cover change characteristics of Shijiazhuang, a city undergoing rapid urbanization in China, from 2000 to 2020 using a dynamic attitude model. The results show that the rate of surface cover change in Shijiazhuang City is relatively fast, but the rate of surface cover change gradually slows down during the 20-year period. The LC change is mainly manifested in the mutual transfer of cropland, woodland and grassland. In the future, the area of cropland, water bodies and bare land decreases, the business-as-usual development (BAU) scenario has the most drastic increase in construction land, and the changes in woodland and grassland are weak, with an increase in economic benefits. In the Ecological Priority Development (EDP) scenario, woodland and grassland expand significantly while construction land growth stagnates, and ecological functions are restored. In the Ecologically and Economically Balanced Development (EEB) scenario, ecological land increases and the growth of built-up land slows down, realizing both economic and ecological benefits. The continuous shrinkage of water bodies is a pressing issue. The coupled model can provide scientific references for the simulation of spatial and temporal changes of LC and CS, the early warning of ecological risks, and the development of land cover planning.
With the growing demand for urban development, human transformation of land has been increasing, leading to significant changes in land-use structure. This rapid urbanization process not only drives the evolution of land cover and function, but also triggers multiple ecological responses. For example, during urbanization, natural ecosystems such as agricultural land, forests and wetlands are developed for urban use, leading to a decline in carbon storage capacity. Construction activities usually destroy soil structure and reduce soil organic carbon content, further affecting carbon storage capacity. Rapid urbanization may lead to the destruction of biological habitats, which reduces the stability and resilience of ecosystems to climate change, thereby affecting carbon storage. The sustainable development goals set out by the United Nations emphasize the importance of protecting ecosystems and promoting sustainable urban development. Countries are also considering how to balance urbanization and carbon storage when formulating urban development policies, such as the Paris Agreement, which encourages countries to take measures to reduce greenhouse gas emissions and promote carbon neutrality, to which urbanized areas need to actively contribute. Multi-temporal and spatial scale land cover simulation can help to deepen the understanding of the current land cover status and its dynamic changes, and thus provide strong support for scientific planning of land cover (Sun et al., 2016; Gashaw et al., 2018). Understanding the impacts of rapid urbanization on land carbon storage can help formulate effective adaptation and mitigation strategies to ensure the coordination of urban development and environmental protection. Meanwhile, realizing the vision of “carbon neutrality” (Guo et al., 2024) puts forward new requirements for the prediction of LC and carbon stock (CS) of terrestrial ecosystems.
With the rapid progress of urbanization in China, the urban population has been increasing, resulting in the intensification of human-land conflicts and the deterioration of the ecological environment (Zhu et al., 2023b; 2023a; 2024a; 2024b). Shijiazhuang City is located in Hebei Province, which is the capital city and economic center of Hebei Province. It is located in the Bohai Economic Circle and Beijing-Tianjin-Hebei integrated development area, and is situated on the Beijing-Guangzhou Railway line, with a dense railroad transportation network and rapid urban development, which is an important position among the cities in northern China (Cao et al., 2024; Song et al., 2024). Therefore, the study takes Shijiazhuang City as an example, and the study of multi-scenario land cover and carbon storage is of great significance for realizing ecological environmental protection and sustainable development and utilization of land resources.
In recent years, ecological process modeling based on land cover has been regarded as an effective tool for terrestrial simulation and environmental change. Currently, many scholars have carried out a large number of evolutionary and simulation studies on LC and ecological issues, and explored the optimal scenarios for land planning under their respective settings. Guo et al. conducted a multi-scenario evolutionary simulation of the Beijing-Tianjin-Hebei region using land cover data, and analyzed the simulation results of carbon storage in the Beijing-Tianjin-Hebei region under different scenarios (Guo et al., 2022). Ji et al. analyzed land cover and habitat quality changes in the Yellow River Basin based on different scenarios and multi-scale perspectives using land cover/land cover data (Ji et al., 2023). Lv et al. proposed a PLUS-ESI-Circuit coupling framework for multi-scenario ecological security evaluation in Ningxia Hui Autonomous Region, and analyzed the impacts of land cover changes on ecological security under different development goals (Lv et al., 2024). Ma et al. (2024) used the North China Plain as the study area and applied the Patch-Generating Land Use Simulation (PLUS) model and Integrated Valuation of Ecosystem Services and Tradeoffs (InVEST) model in an integrated manner to construct three land use policies to predict land use changes and measure carbon stock changes in 2030 (Ma et al., 2024). Zafar et al. (2024) used CA-Markov model to simulate future LULC scenarios and InVEST model to assess CS changes in Pakistan from 2001 to 2030 (Zafar et al., 2024; Zhang et al., 2024a evaluated the spatial and temporal changes of carbon stocks in mid-to high-latitude alpine watersheds in China under historical and future climate scenarios based on the “quantity-mechanism-scenario” framework, integrating the MCE-CA-Markov and InVEST models (Zhang M. et al., 2024). An et al. (2024) used the Factor-generating landuse simulation (FLUS) model to generate 1 km resolution LUCC datasets for China in 2030 and 2060, and analyzed the change of carbon density in Chinese terrestrial ecosystems and its relationship with land use changes from 1990 to 2060 based on the Chinese Terrestrial Ecosystem Carbon Density dataset (An et al., 2024). However, the above studies tend to be larger in scale, ignoring the study of spatial and temporal changes in carbon stocks generated by land cover changes at small and medium scales represented by cities, and paying less attention to the study of their future trends of multi-scenario changes.
In addition, in order to quickly carry out the calculation of different carbon pools for each land cover type and analyze the spatial and temporal characteristics and dynamics of carbon stocks, the InVEST model was developed, whose carbon stock calculation module only requires inputting Its carbon stock calculation module only needs to input the carbon density of various land types to realize the estimation and mapping of carbon stocks in terrestrial ecosystems (Spawn et al., 2020). Since the CS estimation of the InVEST model is based on LC spatial data, obtaining accurate historical and future simulation data of LC is the basis for reasonable CS spatio-temporal evolution analysis (Adelisardou et al., 2022). For future land cover spatial layout prediction, the land cover simulation model (FLUS) integrates the advantageous land expansion analysis strategy (LEAS) (VERBURG et al., 2002) and a metacellular automaton (MAC) based on the roulette wheel mechanism and the threshold decreasing The Cellular automata (CA) model CARS, which is improved by the algorithm, can simulate the fine-grained changes of each land cover type on the basis of mining the triggers of LC changes (Liang et al., 2021). In the determination of future land cover demand, some kind of development planning is generally designed by artificially changing the transfer probability of each land cover type on the basis of Markov model (Chang et al., 2021), but the realization of Multi Objective Optimization (MOP) model under different scenarios based on the reality of the study area is still less applied, especially for the ecological processes of land cover change at the urban level is even more lacking. Therefore, understanding the synergistic mechanism between LC and CS changes, dynamically adjusting land cover planning, and establishing a multi-scenario evolution model study of carbon stock at the urban level are the key tasks to optimize the future spatial resource allocation of the land and improve the ecosystem function.
In order to solve the above problems, this study takes Shijiazhuang as an example and proposes the MOP-FLUS-InVEST modeling framework, which is combined with the land cover changes in Shijiazhuang from 2000 to 2020, to analyze the future land cover and carbon stock in a multi-scenario simulation. The main innovations and contributions of this study are as follows: 1) The land cover data of Shijiazhuang, a typical rapidly urbanizing city, were dynamically analyzed for three periods in 2000, 2010, and 2020 to characterize the land cover changes in Shijiazhuang from 2000 to 2020. 2) A coupled MOP-FLUS-InVEST (MFI) modeling framework is proposed, and a multi-scenario simulation analysis of the historical evolution and future of land cover and carbon stock is carried out using Shijiazhuang as an example. 3) This study can be used to explore the coordinated sustainable development of ecology and economy to some extent.
As shown in Figure 1, Shijiazhuang (113°18ˊ∼115°30′E, 37°30ˊ∼38°40ˊW) is located in North China, the capital city of Hebei Province, and the political, economic and cultural center of the province, situated in the south-central part of Hebei Province, with an advantageous geographic location and convenient transportation (Li et al., 2024; Zhang J. et al., 2024). Shijiazhuang has a total area of 14,530 km2, including 8 districts, 11 counties and 3 county-level cities. Shijiazhuang has a complex geomorphology, with its western part distributed in the Taihang Mountains and its eastern part in the North China Alluvial Plain, with its topography high in the west and low in the east and high in the north and low in the south. Shijiazhuang has a temperate monsoon climate with four distinct seasons, total annual precipitation of 401.1–752.0 mm, and abundant sunshine (Ni et al., 2024; Zhao et al., 2024).
The vector data of Shijiazhuang administrative area and precipitation data used in this study were downloaded from the Resource and Environmental Science Data Registration and Publication System (http://www.resdc.cn/doi), and the temperature data (Kašpar et al., 2021) were obtained from NOAA Physical Science Laboratory (www.psl.noaa.gov). The data used for land cover evolution analysis are: 2000–2020 land cover data, natural drivers, socio-economic drivers, accessibility factors, statistical data, policy planning documents, etc. The data used for carbon stock evolution analysis are: 2000–2020 land cover data, carbon density data.
The land cover data were selected from the GlobeLand30 dataset (http://www.globallandcover.com), and seven land cover types were divided within the study area (Hu et al., 2020); the policy planning documents were obtained from the website of the Ministry of Natural Resources (http://www.mnr.gov.cn/), which was used to develop the objective function of the MOP model. Carbon density data included aboveground and belowground biogenic carbon density and soil surface organic carbon density (Lu et al., 2018). Above- and below-ground biogenic carbon densities were obtained from the time-consistent and harmonized global map of above- and below-ground biomass carbon densities produced by Spawn et al. (2020) (https://doi.org/10.3334/ORNLDAAC/1763).
As shown in Figure 2, combined with the current situation of the study area, the research objectives are proposed, the land cover dynamic attitude model analysis is carried out and the content of the coupled MOP-FLUS-InVEST model for the study is determined.
The land cover structure quantity optimization module MOP, the spatial layout prediction module FLUS and the carbon stock analysis module InVEST. The role of the MOP model is to determine the land cover demand under different development scenarios through the historical evolution of land cover in the study area and the future land cover planning, and to provide data inputs for the layout simulation. The FLUS model firstly uses the LEAS land cover expansion strategy, and analyzes the relationship between the expansion and potential driving factors of each land cover type through the random forest analysis. The FLUS model first uses the LEAS land expansion strategy to analyze the relationship between the expansion of each land cover type and the potential driving factors through random forests, and spatially speculates the expansion probability of each land cover type, and then determines the future spatial layout of LC by combining the future land cover demand and CARS, a metacellular automaton model that improves based on the seeding mechanism of multiple types of stochastic patches. The InVEST model is used to determine the spatial distribution of the carbon stock in the past and the future, and performs the analysis on the evolution of the carbon stock. The MOP- FLUS-InVEST coupled model is used to explore the driving mechanism of LC change, determine the development target according to local conditions and conduct multi-scenario evolution analysis of LC and CS, which will ultimately be used in territorial spatial planning, and provide a reference for the formulation of related policies.
The land cover momentum attitude model, which is able to analyze the magnitude of surface cover changes from a quantitative perspective (Jin et al., 2023).
In this study, a single land cover momentum attitude is used to reflect the change in the number of a particular surface cover type in Shijiazhuang City within a certain time frame. As shown in Equation 1.
where
In this study, the comprehensive land cover motivation attitude is used to express the comprehensive quantitative changes of all surface cover types in Shijiazhuang City. As shown in Equation 2.
where
Currently, the contradiction between China’s economic development and ecological environment is becoming more and more prominent. With the goal of prioritizing and balancing the development of the two, the MOP model designs three different scenarios by properly defining the objective optimization function and constraints, namely, BAU, EDP, and EEB, have been designed to simulate the future state of land cover. The three scenarios represent different input parameter Settings and optimization objectives, and the parameters and data requirements for each scenario reflect different emphasis on land use, ecological services, and economic benefits.
BAU: The BAU scenario refers to the creation of a baseline state of land cover change based on the trend of land cover change from 2000 to 2020 without the interference of other factors. The 2000–2020 land cover transfer matrix is extracted and the Markov model is used to forecast land cover demand;
EDP: The EDP scenario is an ecological development prioritization scenario, and the goal of this scenario is to protect the ecosystem and restore ecosystem functions. The objective is to maximize ESV. As shown in Equation 3.
where
EEB: The EEB is the ecological and economic balance scenario, which aims to coordinate the improvement of economic efficiency and ecological function by adjusting land cover planning.
To improve the reliability of land cover layout simulation. FLUS model needs to set parameters such as driving factors, land use transfer rules, and land use type neighborhood weights according to the specific situation of the study area. The FLUS model was used to extract the parts of various types of land use expansion between the two phases of land use change, and the random forest algorithm was used to excavate the factors of various types of land use expansion and driving force one by one, and the domain weights were set. In addition, five natural drivers: elevation, slope, average temperature, average precipitation, and soil type; two socio-economic drivers: GDP and population distribution; and five accessibility factors: distance to government sites, water bodies, highways, railroads, and urban arterials were selected in the study area (Figures 3A–L).
The InVEST model has the advantage of simple model inputs - land use distribution and carbon density. The carbon stock estimation of the InVEST model (Fang Z. et al., 2022) contains four carbon pools, namely, above-ground vegetation, below-ground vegetation, soil surface and apomictic, and the apomictic part was not considered in this study because it is difficult to access. As shown in Equation 4.
Where
a. Total area constraint: the area of each land use type is greater than zero, and the sum of the areas is equal to the total area of the study area.
b. Population constraint: Ensure that the population of construction land is limited within the range of the predicted total population to ensure the population carrying capacity. Based on the demographic data of Shijiazhuang, Hebei Province from 2000 to 2020 and GM (1,1) model, the population density and population size in 2030 were predicted.
c. Constraints on cultivated land area: Food security is the basis for ensuring national and regional stability. The grain yield of cultivated land should meet the local demand: As shown in Equation 5.
In this context, f1 represents per capita food consumption (kg), f2 is the regional food self-sufficiency rate, f3 is the yield of food crops per unit area (kg/km2), f4 is the proportion of food crop planting, and f5 is the cropping index. Hebei Province is an important grain base in North China; therefore, f2 = 100%. Based on yearbook data and the GM (1, 1) model, f3 and f4 are calculated. The constraints are set with the current permanent farmland area as the lower limit and the area in 2020 as the upper limit.
d. Forest area constraint: The Chinese government has proposed an ecological protection strategy framework through the ‘General Plan for Major Projects in the Protection and Restoration of Important National Ecosystems (2021–2035), which aims to build the “Two Screens and Three Belts” strategy. The study area is advancing the construction of the Northern Sand Prevention Belt system. In the next 10 years, the growth rate of forest area should exceed that of the BAU scenario.
e. Grassland area constraint: Since 2000, the grassland area has remained relatively stable. In the study area, there has been frequent conversion among grassland, arable land, and forest land, while reserving some space for landscape diversity and urban development. Therefore, the 2020 grassland area (Area) is set as the baseline, with changes in grassland area controlled within 5%, which can be expressed as: As shown in Equation 6.
f. Construction land constraint: In the future, the growth of construction land will be strictly controlled, and its expansion rate will be slowed down. Therefore, the area of construction land should be less than that in the BAU scenario.
g. Water body and wetland area constraint: Water bodies and wetlands are important ecological lands. With the implementation of policies such as returning farmland to wetlands, it is expected that the decrease in water bodies and wetlands will slow down, while ecological land in nature reserves will be restored. Therefore, the results from the BAU scenario are set as the lower limit, with the average value from 2000 to 2020 as the upper limit.
h. Bare land area constraint: In the future, bare land will be converted into construction land and ecological land. Therefore, the areas from 2020 to 2000 are set as the upper and lower limits.
i. Important ecological function constraint: The functions of water conservation and soil conservation are emphasized in the new ecological protection strategy. Therefore, it is set that by 2030, the ‘hydrological regulation’ and ‘soil conservation’ functions, as well as the overall ESV in the study area, should be higher than the levels in 2020.
a. Transfer rules: The establishment of transfer rules determines whether the conversion of one land use type to another is restricted. According to the general of land use change, construction land is generally difficult to convert to other land use types and is only allowed to temporarily convert to bare land. Under the current restorative governance of water bodies and wetlands in the study area, their conversion to non-ecological land (arable land and construction land) should be strictly controlled. It is stipulated that the water bodies and wetlands in the ecological protection area for water conservation are not allowed to be converted.
b. Neighborhood weight: The weight reflects the influence of the current pixel on surrounding pixels, with a range of 0–1. The larger the value, the more likely other types within the neighborhood are to convert to the type of the current pixel. The land use weight is determined based on the proportion of the expansion area of each land use type from 2000 to 2020 in the total expansion area.
The speed of land cover change quantitatively describes the speed of regional land cover change, and the attitude of motivation can indicate the degree of stability of a certain type of land cover change, and the smaller the value of motivation, the more stable the area of land cover of that type is. Table 1, 2 show the single land cover momentum and comprehensive land cover momentum in Shijiazhuang City from 2000 to 2020.
From the data in Table 1, 2, it can be found that the attitude of each type of land cover motivation in Shijiazhuang City ranges from −6.26% to 2.57%. Among them, forest land and impervious surface are always positive, indicating that forest land and impervious surface have been showing a trend of gradual increase during the period of 2000–2020, in which the area of impervious surface increases more, and the urban area gradually increases; cultivated land and wasteland are always negative, indicating that cultivated land and wasteland have been showing a trend of gradual decrease during the period of 2000–2020. Shijiazhuang shrubs showed an increasing trend during 2000–2000, a decreasing trend during 2000–2010, and an increasing trend during 2010–2020, with an overall increase over the 20-year period and a gradually accelerating rate of change. Grassland showed an increasing trend between years, a decreasing trend between 2000 and 2010, and a continuing decrease between 2010 and 2020, with an overall decreasing trend over the 20-year period. Water shows an increasing trend during 2000–2010, continues to increase during 2010–2020, and overall shows an increase over the 20-year period, with the rate of change gradually accelerating.
During the 2000–2010 period, impervious surfaces had the largest single land cover trend of 2.01%, and wasteland had the smallest single land cover trend of −6.26%, with larger changes in wasteland and grassland, and smaller changes in cropland and water. In the period 2010–2020, the single land cover motivation for shrubs is the largest at 2.44%, the single land cover motivation for wasteland is the smallest at −5.93%, and the magnitude of change is larger for wasteland and shrubs and smaller for cropland and woodland. In the period 2000–2010, the single land cover motivation of wasteland was the largest at −6.26% and the single land cover motivation of cropland was the smallest at −0.28%. The magnitude of change was larger for wasteland and grassland and smaller for water and cropland.
Comprehensive land cover motivation, on the other hand, reflects the change relationship between all land types, and in the 2 time periods of 2000–2010 and 2010–2020, the comprehensive land cover motivation of 0.42% and 0.39%, respectively, are positive, indicating that on the whole, Shijiazhuang City’s surface cover changes at a faster rate, but gradually decelerates during the 20-year period, and the rate of surface cover change gradually slows down.
Currently, the contradiction between economic development and ecological environment is becoming more and more prominent in China. With the goal of prioritizing and balancing the development of the two, three different scenarios, namely, BAU, EDP, and EEB, have been designed to simulate the future land cover status.
The land cover layout of the study area is shown in Figures 4A–E. It can be seen that the historical evolution of land cover in the study area from 2000 to 2020 is mainly reflected in the transfer between arable land, forest land, and grassland, on the basis of which the explosive growth of construction land encroached on a large amount of arable land, which is mainly characterized by 1) the continuous decrease in the area of arable land and water bodies, which is converted to construction land, followed by the transfer to grassland 2) the continuous increase of forest land, construction land, and bare land, and the bare land area’s growth then comes mainly from the decrease of grassland; 3) the area of grassland and wetland increases and then decreases, mainly due to the transfer to water bodies and cultivated land. 4) There are obvious spatial differences in the spatial location of growth of different land types. Because topography and geomorphology, water and heat conditions, and economic level affect the suitability of spatial expansion of land cover types, the expansion of forest land and grassland is mainly located in the mountainous areas of the Taihang Mountains; and the expansion of construction land is mainly outward along the edges of the original construction land.
The results of the future multi-scenario simulation (Figures 5A–F) show that compared with the historical changes in land cover, the area of cropland and water bodies will continue to decrease in the BAU, EDP, and EEB scenarios, and the rate of decrease decreases in turn. The results of different scenarios have obvious goal-oriented effects. Under the BAU scenario, the policy focus of social construction in the study area will continue the trend from 2000 to 2020, which is still tilted toward economic development, and the land for construction in the plains will continue to grow, and the rest of the land cover types will decrease, with wetlands being the most important, and mainly converted into forested land and water bodies. Under the EDP scenario, the development of the study area is targeted at ecological service functions and protection of the ecological environment. The ESV has increased compared to 2020. Compared to 2020, the area of woodland and grassland increased, while the rest of the land types decreased. In terms of spatial location, the expansion of grassland and woodland mainly occurs in the western and northern mountainous areas. Under the EEB scenario, the development objective of the study area is to balance the value of ecological services with socio-economic benefits. Comparing the land cover results of the three scenarios, the degree of land cover change under the EEB scenario is basically between the BAU scenario and the EDP scenario, with an increase in the three types of ecological land cover: forest land, grassland and wetland, while the growth of construction land cover is mitigated, with cropland and bare land being the main source of growth for the remaining land cover types, and the water body still showing a decreasing trend. The divergence of the spatial location of the expansion of different land types is similar to the remaining two scenarios.
The results of historical land cover layout and and simulation were inputted into the InVEST model to obtain the CS evolution results from 2000 to 2020 (Figures 6A–F). Among the various land cover types, forest land, cropland and grassland contributed the most to the CS in the study area, so the spatial distribution of CS converged with the distribution of land cover types, which was mainly influenced by the topography, showing high in the northwest and low in the southeast.
As shown in Figures 5A–F, the carbon stock evolution in the study area under different scenarios shows obvious spatial differences. In the BAU scenario, carbon stock increases in very few areas and decreases in the rest of the area, which indicates that urbanization and human activities will continue to pressure the ecosystems without interventions, thus affecting the carbon storage capacity. In the EDP scenario, the carbon stock change is small, indicating that more ecological protection measures are taken in this scenario, which helps to stabilize the carbon stock and provides an important reference for urban planning, emphasizing the need for ecological protection and sustainable development. In the EEB scenario, there is a significant increase in carbon stock, indicating that certain ecological protection strategies are adopted while taking into account the economic development, realizing the benign interaction between ecology and economy, and that reasonable land management strategies can effectively increase carbon stock, bringing win-win effects. The carbon stock in Shijiazhuang in 2010 is 159.69 Tg, and in 2020 it is 158.75 Tg, 158.35 Tg in BAU scenario, 168.32 Tg in EDP scenario, and 165.55 Tg in EEB scenario. The change in carbon stocks from 2010 to 2020 is 0.94 Tg, 0.4 Tg in the BAU scenario, 9.57 Tg in the EDP scenario and 6.8 Tg in the EEB scenario. Further studies showed that forest land, cropland and grassland contributed the most to the carbon stock, so priority should be given to the protection of these land use types in urban planning to prevent their diversion to other uses. Combined with the spatial distribution characteristics of CS, urban planning should consider topographic factors, rationalize the layout of green spaces and ecological corridors, and enhance the carbon storage capacity of the region through the planning of contiguous green spaces. In addition, under the BAU scenario, policies to enhance ecological restoration, such as afforestation and wetland restoration, are proposed. At the same time, an ecological compensation mechanism can be set up to encourage farmers and developers to protect the ecological environment. Urban green infrastructure (e.g., rain gardens, urban forests, etc.) can be upgraded to enhance the carbon storage capacity of the city and improve the city’s microclimate.
In this study, the impacts of land cover changes on carbon stocks under different development scenarios and targets are explored, and the evolution of the spatial and temporal patterns of the two is simulated. The conclusions of the study are as follows:
(1) The results of the dynamic attitude analysis show that overall the rate of land cover change in Shijiazhuang City is relatively fast, but it gradually decelerates during the 20-year period, and the rate of land cover change gradually slows down. On the trend of land cover and carbon stock changes from 2000 to 2020, the transfer of each land cover type is dominated by the mutual conversion of cropland, forest land and grassland, in which a large amount of cropland becomes the source of new construction land, while at the same time water bodies and wetlands are encroached by construction land and cropland.
(2) The simulation results of land cover and carbon stock distribution in 2030 are similar to the historical situation. The main difference between the three simulation results is that the BAU scenario has a sharp increase in construction land and ecological land damage. The EDP scenario controls the growth of construction land, the expansion of woodland and grassland area, and wetland restoration. The EEB scenario weighs the ecological and economic benefits.
Therefore, the study concludes that the EEB scenario is more in line with the long-term development of Shijiazhuang under the background of green economy and the vision of “carbon neutrality.” Meanwhile, Shijiazhuang should consolidate the forest protection achievements and curb the degradation of aquatic ecosystems. Focusing on the rational use of land resources and the protection of the ecological environment, it should realize the goal of sustainable urban development through scientific planning and management (Fang et al., 2022b; 2022a). At the same time, it is necessary to strengthen the monitoring and assessment of changes in surface cover, and to adjust and improve relevant policies and measures in a timely manner to ensure the sustainable utilization of land resources and the continuous improvement of the ecological environment (Fang et al., 2024).
Future research will be devoted to exploring more efficient methods of land cover structure optimization, taking into account the dynamic changes of climate, human activities, vegetation and other influencing factors, considering the carbon stock differences within land cover types, and improving the accuracy of the model with the measured data of the sample plots.
The original contributions presented in the study are included in the article/supplementary material, further inquiries can be directed to the corresponding author.
QL: Conceptualization, Data curation, Funding acquisition, Investigation, Methodology, Software, Visualization, Writing–original draft, Writing–review and editing. LK: Conceptualization, Data curation, Funding acquisition, Investigation, Methodology, Software, Supervision, Visualization, Writing–original draft, Writing–review and editing.
The author(s) declare that no financial support was received for the research, authorship, and/or publication of this article.
The authors would like to thank the editors and the reviewers for their constructive comments and suggestions, which greatly helped to improve the quality of the manuscript.
Authors QL and LK were employed by China Water Resources Beifang Investigation, Design and Research Co., Ltd.
All claims expressed in this article are solely those of the authors and do not necessarily represent those of their affiliated organizations, or those of the publisher, the editors and the reviewers. Any product that may be evaluated in this article, or claim that may be made by its manufacturer, is not guaranteed or endorsed by the publisher.
Adelisardou, F., Zhao, W., Chow, R., Mederly, P., Minkina, T., and Schou, J. S. (2022). Spatiotemporal change detection of carbon storage and sequestration in an arid ecosystem by integrating Google Earth Engine and InVEST (the Jiroft plain, Iran). Int. J. Environ. Sci. Technol. 19, 5929–5944. doi:10.1007/s13762-021-03676-6
An, Y., Tan, X., Ren, H., Li, Y., and Zhou, Z. (2024). Historical changes and multi-scenario prediction of land use and terrestrial ecosystem carbon storage in China. Chin. Geogr. Sci. 34, 487–503. doi:10.1007/s11769-024-1424-y
Cao, L., Liu, X., Zhu, P., and Wang, L. (2024). The distribution and evolution of groundwater level depths and groundwater sustainability in the hexi corridor over the last five years. Sustainability 16, 2527. doi:10.3390/su16062527
Chang, X., Zhang, F., Cong, K., and Liu, X. (2021). Scenario simulation of land use and land cover change in mining area. Sci. Rep. 11, 12910–12912. doi:10.1038/s41598-021-92299-5
Fang, G., Chen, G., Yang, K., Yin, W., and Tian, L. (2024). How does green fiscal expenditure promote green total factor energy efficiency? — Evidence from Chinese 254 cities. Appl. Energy 353, 122098. doi:10.1016/j.apenergy.2023.122098
Fang, G., Gao, Z., Wang, L., and Tian, L. (2022a). How does green innovation drive urban carbon emission efficiency? —evidence from the Yangtze River Economic Belt. J. Clean. Prod. 375, 134196. doi:10.1016/j.jclepro.2022.134196
Fang, G., Yang, K., Tian, L., and Ma, Y. (2022b). Can environmental tax promote renewable energy consumption? — An empirical study from the typical countries along the Belt and Road. Energy 260, 125193. doi:10.1016/j.energy.2022.125193
Fang, Z., Ding, T., Chen, J., Xue, S., Zhou, Q., Wang, Y., et al. (2022c). Impacts of land use/land cover changes on ecosystem services in ecologically fragile regions. Sci. Total Environ. 831, 154967. doi:10.1016/j.scitotenv.2022.154967
Gashaw, T., Tulu, T., Argaw, M., and Worqlul, A. W. (2018). Modeling the hydrological impacts of land use/land cover changes in the Andassa watershed, Blue Nile Basin, Ethiopia. Sci. Total Environ. 619–620, 1394–1408. doi:10.1016/j.scitotenv.2017.11.191
Guo, W., Lv, L., Zhao, X., Cui, X., and Rienow, A. (2024). Multiscale coupled development and linkage response evaluation of China’s carbon neutrality and sustainable development capability–A quantitative analysis perspective. Renew. Sustain. Energy Rev. 200, 114569. doi:10.1016/j.rser.2024.114569
Guo, W., Teng, Y., Yan, Y., Zhao, C., Zhang, W., and Ji, X. (2022). Simulation of land use and carbon storage evolution in multi-scenario: a case study in beijing-tianjin-hebei urban agglomeration, China. Sustainability 14, 13436. doi:10.3390/su142013436
Hu, Q., Xiang, M., Chen, D., Zhou, J., Wu, W., and Song, Q. (2020). Global cropland intensification surpassed expansion between 2000 and 2010: a spatio-temporal analysis based on GlobeLand30. Sci. Total Environ. 746, 141035. doi:10.1016/j.scitotenv.2020.141035
Ji, X., Sun, Y., Guo, W., Zhao, C., and Li, K. (2023). Land use and habitat quality change in the Yellow River Basin: a perspective with different CMIP6-based scenarios and multiple scales. J. Environ. Manage. 345, 118729. doi:10.1016/j.jenvman.2023.118729
Jin, Y., Li, A., Bian, J., Nan, X., and Lei, G. (2023). Modeling the impact of investment and national planning policies on future land use development: a case study for Myanmar. ISPRS Int. J. Geo-Information 12, 22. doi:10.3390/ijgi12010022
Kašpar, V., Hederová, L., Macek, M., Müllerová, J., Prošek, J., Surový, P., et al. (2021). Temperature buffering in temperate forests: comparing microclimate models based on ground measurements with active and passive remote sensing. Remote Sens. Environ. 263, 112522. doi:10.1016/j.rse.2021.112522
Li, S., Zhou, Y., Yue, D., and Zhao, Y. (2024). Streamflow variation under climate conditions based on a soil and water assessment tool model: a case study of the bailong River Basin. Sustainability 16, 3901. doi:10.3390/su16103901
Liang, X., Guan, Q., Clarke, K. C., Liu, S., Wang, B., and Yao, Y. (2021). Understanding the drivers of sustainable land expansion using a patch-generating land use simulation (PLUS) model: a case study in Wuhan, China. Comput. Environ. Urban Syst. 85, 101569. doi:10.1016/j.compenvurbsys.2020.101569
Lu, X., Kuang, B., Li, J., Han, J., and Zhang, Z. (2018). Dynamic evolution of regional discrepancies in carbon emissions from agricultural land utilization: evidence from Chinese provincial data. Sustainability 10, 552–565. doi:10.3390/su10020552
Lv, L., Guo, W., Zhao, X., Li, J., Ji, X., and Chao, M. (2024). Integrated assessment and prediction of ecological security in typical ecologically fragile areas. Environ. Monit. Assess. 196, 286. doi:10.1007/s10661-024-12453-0
Ma, D., Huang, Q., Wang, Q., Lin, Z., and Xu, H. (2024). Simulation of LUCC scenarios and analysis of the driving force of carbon stock supply changes in the North China plain in the context of urbanization. Forests 15, 1414. doi:10.3390/f15081414
Ni, L., Li, J., and Namaiti, A. (2024). Classification and planning strategies of multidimensional resilience units for urban waterlogging: a case study of the old city district in Shijiazhuang, China. Sustainability 16, 2717. doi:10.3390/su16072717
Song, X., Zhai, S., and Zhou, N. (2024). The carbon emissions from public buildings in China: a systematic analysis of evolution and spillover effects. Sustainability 16, 6622. doi:10.3390/su16156622
Spawn, S. A., Sullivan, C. C., Lark, T. J., and Gibbs, H. K. (2020). Harmonized global maps of above and belowground biomass carbon density in the year 2010. Sci. Data 7, 112. doi:10.1038/s41597-020-0444-4
Sun, L., Wei, J., Duan, D. H., Guo, Y. M., Yang, D. X., Jia, C., et al. (2016). Impact of Land-Use and Land-Cover Change on urban air quality in representative cities of China. J. Atmos. Solar-Terrestrial Phys. 142, 43–54. doi:10.1016/j.jastp.2016.02.022
Verburg, P. H., Soepboer, W., Veldkamp, A., Limpiada, R., Espaldon, V., and Mastura, S. S. A. (2002). Modeling the spatial dynamics of regional land use: the CLUE-S model. Environ. Manage. 30, 391–405. doi:10.1007/s00267-002-2630-x
Zafar, Z., Zubair, M., Zha, Y., Mehmood, M. S., Rehman, A., Fahd, S., et al. (2024). Predictive modeling of regional carbon storage dynamics in response to land use/land cover changes: an InVEST-based analysis. Ecol. Inf. 82, 102701. doi:10.1016/j.ecoinf.2024.102701
Zhang, J., Zhang, W.-G., Zhang, X.-Y., Li, Y.-H., Feng, M.-M., Qi, P., et al. (2024a). Mechanisms for carbon stock driving and scenario modeling in typical mountainous watersheds of northeastern China. Environ. Monit. Assess. 196, 798. doi:10.1007/s10661-024-12947-x
Zhang, M., Chen, E., Zhang, C., Liu, C., and Li, J. (2024b). Multi-scenario simulation of land use change and ecosystem service value based on the markov–FLUS model in ezhou city, China. Sustainability 16, 6237. doi:10.3390/su16146237
Zhao, Z., Hu, Z., Han, X., Chen, L., and Li, Z. (2024). Evaluation of urban resilience and its influencing factors: a case study of the yichang–jingzhou–jingmen–enshi urban agglomeration in China. Sustainability 16, 7090. doi:10.3390/su16167090
Zhu, L., Guo, Z., Xing, H., and Sun, W. (2023a). A coupled temporal-spectral-spatial multidimensional information change detection framework method: a case of the 1990-2020 Tianjin, China. IEEE J. Sel. Top. Appl. Earth Obs. Remote Sens. 16, 5741–5758. doi:10.1109/JSTARS.2023.3288218
Zhu, L., Jiang, X., Zhao, L., Qu, H., and Sun, W. (2023b). A temporal-spectral value and shape change detection method integrating thematic index information and spectral band information. Environ. Sci. Pollut. Res. 30, 47408–47421. doi:10.1007/s11356-023-25685-3
Zhu, L., Shi, M., Fan, D., Tu, K., and Sun, W. (2023c). Analysis of changes in vegetation carbon storage and net primary productivity as influenced by land-cover change in inner Mongolia, China. Sustainability 15, 4735. doi:10.3390/su15064735
Zhu, L., Sun, W., Fan, D., Xing, H., and Liu, X. (2024a). Unsupervised spatial self-similarity difference-based change detection method for multi-source heterogeneous images. Pattern Recognit. 149, 110237. doi:10.1016/j.patcog.2023.110237
Zhu, L., Sun, W., Wu, J., and Fan, D. (2023d). Spatiotemporal distribution of carbon sink indicators — NPP and its driving analysis in ordos city, China. Appl. Sci. 13, 6457. doi:10.3390/app13116457
Zhu, L., Sun, W., Xing, H., Fan, D., and Wang, C. (2024b). A phenological knowledge transfer-based fine grained land cover change sample collection method: a case study of coastal wetlands. Int. J. Digit. EARTH 17. doi:10.1080/17538947.2024.2310090
Zhu, L., Xing, H., and Hou, D. (2022). Analysis of carbon emissions from land cover change during 2000 to 2020 in Shandong Province, China. Sci. Rep. 12, 8021–8033. doi:10.1038/s41598-022-12080-0
Keywords: MFI coupled model, scenario simulation, sustainable development, land cover change, carbon stock evolution
Citation: Li Q and Kong L (2024) Multi-scenario evolutionary simulation of land cover-based carbon stock during rapid urbanization: a case of shijiazhuang city. Front. Environ. Sci. 12:1486596. doi: 10.3389/fenvs.2024.1486596
Received: 30 August 2024; Accepted: 15 October 2024;
Published: 24 October 2024.
Edited by:
Merja H. Tölle, University of Kassel, GermanyReviewed by:
Wenbin Sun, China University of Mining and Technology, Beijing, ChinaCopyright © 2024 Li and Kong. This is an open-access article distributed under the terms of the Creative Commons Attribution License (CC BY). The use, distribution or reproduction in other forums is permitted, provided the original author(s) and the copyright owner(s) are credited and that the original publication in this journal is cited, in accordance with accepted academic practice. No use, distribution or reproduction is permitted which does not comply with these terms.
*Correspondence: Qiang Li, bGlfcTNAYmlkci5jb20uY24=
Disclaimer: All claims expressed in this article are solely those of the authors and do not necessarily represent those of their affiliated organizations, or those of the publisher, the editors and the reviewers. Any product that may be evaluated in this article or claim that may be made by its manufacturer is not guaranteed or endorsed by the publisher.
Research integrity at Frontiers
Learn more about the work of our research integrity team to safeguard the quality of each article we publish.