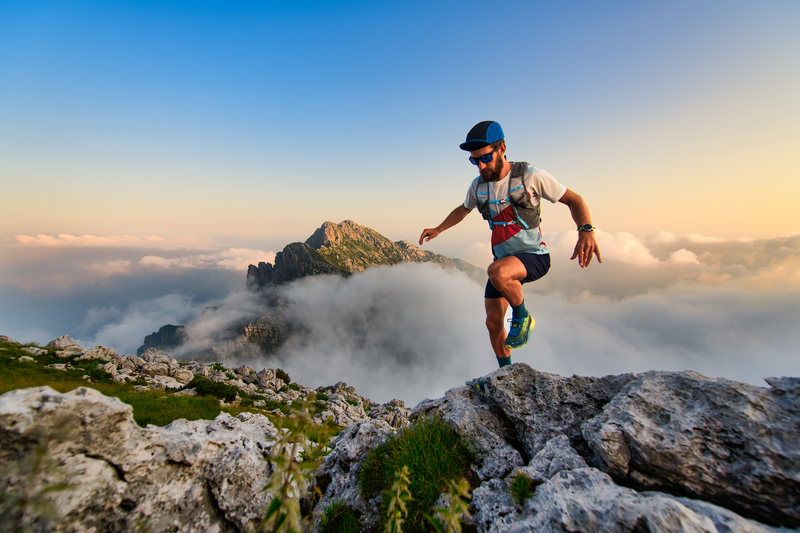
95% of researchers rate our articles as excellent or good
Learn more about the work of our research integrity team to safeguard the quality of each article we publish.
Find out more
ORIGINAL RESEARCH article
Front. Environ. Sci. , 07 January 2025
Sec. Environmental Economics and Management
Volume 12 - 2024 | https://doi.org/10.3389/fenvs.2024.1465619
This study explores the impacts of PEC on green technology innovation (GTI), which includes both independent green technology innovation (IGTI) and cooperative green technology innovation (CGTI). It also analyzes the threshold effects of green finance (GF) and information and communication technology (ICT) to unlock the growth potential of GTI. To achieve this, we employ a two-way fixed-effects model and a threshold-effects model, utilizing city panel data from the Yangtze River Delta region of China, covering the years 2011–2020. The results indicate that PEC has a positive effect on GTI, IGTI, and CGTI. However, its impact on CGTI is relatively weaker. These findings remain valid after several robustness tests. Heterogeneity analysis shows that PEC promotes IGTI in research organizations, yet it fails to do so in firms. PEC enhances CGTI within cities, but not between them. Additionally, PEC positively affects GTI only when GF and ICT surpass certain thresholds. The threshold effect of GF is limited to IGTI, whereas ICT’s threshold effect is more stringent in CGTI. Based on these conclusions, we provide insights on leveraging public concerns to promote the growth of green innovation and how governments can create a favorable external environment for this.
To mitigate the conflict between economic growth and environmental sustainability, countries globally are dedicated to green development. Especially for developing countries, economic development should not come at the expense of the environment (Meo et al., 2023). GTI merges “green” and “innovation” principles, targeting economic advantages of technological advances and ecological gains of clean production (Suki et al., 2022). Therefore, promoting GTI is vital for balancing economic growth and environmental protection. GTI can be divided into CGTI and IGTI. CGTI involves a collaborative innovation model that emphasizes resource complementarity, risk sharing, and mutual benefits (Li and Wang, 2022). Amid growing environmental sustainability pressures, this form of open green innovation is gaining more attention (Zor, 2023). IGTI is an independent innovation model that operates solely on the capabilities of the innovation subjects. IGTI offers unique competitive advantages and avoids the free-rider issues associated with CGTI (Nie et al., 2022). Therefore, both collaborative and independent innovation models are crucial for enhancing the green innovation capacity.
PEC reflects the public’s perception of environmental pollution and air quality (Ren and Ren, 2024) and serves as an informal type of environmental regulation (ER) (Wang L. et al., 2022). Environmental pollution negatively affects the health and longevity (Ebenstein et al., 2017; Shaari et al., 2021). Events such as extreme haze have significantly heightened public environmental concerns (Gu et al., 2021). Particularly with rapid economic growth and rising incomes, residents demand a higher quality of life and become more environmentally conscious. PEC pressure has become a key driver for innovation subjects to pursue green innovations. Therefore, under the pressure of PEC, exploring the growth space of GTI and release its growth potential hold significant practical value.
Environmental pollution in developing countries is more sensitive to changes in economic growth (Hassan et al., 2020). As the largest developing country, China is proposed by Fatima et al. (2021) to adopt green innovation to achieve a green transformation of its economy. China serves as a representative context for studying GTI to balance economic development and environmental sustainability. The Yangtze River Delta region, one of China’s economic centers, has residents with relatively high income, education, and cultural awareness. This results in greater environmental protection awareness and a stronger concern for environmental issues. In December 2020, China’s Ministry of Science and Technology released the Development Plan for the Construction of the Yangtze River Delta Science and Technology Innovation Community, highlighting the importance of collaboration to enhance independent innovation capabilities and promote open innovation. This indicates that the Yangtze River Delta has significant room for improvement in independent and open innovation. Therefore, using the region as a research sample can create a demonstration effect in China and provide value for other developing countries.
Existing studies emphasize PEC as an informal ER and examine the impact of heterogeneous ER on GTI. It is insufficient for a deeper exploration of GTI. In this study, we categorize GTI into IGTI and CGTI from the perspective of heterogeneous GTI to fully explore the growth potential of GTI under the pressure of PEC. There are several contributions: First, we reveal the heterogeneous effects of PEC on IGTI and CGTI. Second, we examine the heterogeneity of innovation subjects in IGTI and the heterogeneity of cooperation scope in CGTI to analyze the hindering factors faced by IGTI and CGTI under the pressure of PEC. Finally, we verify the threshold roles of GF and ICT, as well as the heterogeneity of these roles in IGTI and CGTI, aiming to explore effective ways to mitigate the impediments to GTI.
The structure of the remainder is as follows: Section 2 presents the literature review and research hypotheses. Section 3 introduces the data and methods. Section 4 analyzes the results. Section 5 discusses the results. Section 6 draws the conclusion and implications.
PEC is a form of ER. The theoretical relationship between ER and GTI has be thoroughly discussed, leading to three perspectives: 1) Traditional neoclassical economics posits that, market players will engage in short-term behavior to accommodate ER. This process increases operating costs, resulting in a crowding-out effect on GTI (Petroni et al., 2019; Ramanathan et al., 2010). 2) The Porter hypothesis suggests that, contrary to traditional neoclassical economics, an appropriate level of ER can offset additional costs by promoting green technological change in firms and improving productivity and product quality (Berrone et al., 2013; Porter and Linde, 1995). 3) Other scholars have synthesized these two perspectives and argued that the relationship between PEC and GTI is nonlinear (Ouyang et al., 2020).
In empirical research, the relationship between PEC as voluntary or informal ER and GTI varies depending on the research sample. Some studies focus on the firm level. Liu et al. (2023) conclude that, using large industrial firms in China as their sample, voluntary ER has a U-shaped effect on GTI. In contrast, Wang L. et al. (2022) found that voluntary ER inhibited GTI when using listed companies as their sample. This may be because listed companies have strong information disclosure and adopt short-term governance behaviors to evade ER from the public or media, which crowds out green R&D inputs and inhibits GTI. Additionally, Li and Wang (2021) found that PEC encouraged green innovation behaviors in firms within the automotive industry. This may be because road transportation is one of the major contributors to CO2 emissions, drawing public attention to GTI in the automotive industry. Other studies focus on the regional level. Liu et al. (2022) find that, based on a linear relationship perspective and data from 285 cities in China, voluntary ER inhibit urban GTI, possibly because public concern for the environment is generally low in Chinese cities. However, Jiao et al. (2024) and Xiong and Gao (2023) conclude that PEC promotes GTI in the Yangtze River Economic Belt. This is because the region has advanced economic development, higher per capita income, and residents who pay more attention to environmental protection.
Additionally, GF and ICT actively contribute to GTI. Capital seeks profit, and due to the unique characteristics of GTI, it is challenging for innovators to secure financial support (Li et al., 2023; Ren et al., 2020). Under the GF policy, firms are allowed to issue green bonds to raise funds for enhancing GTI (Wang T. et al., 2022). For highly polluting firms, green innovation can enhance legitimacy and attract additional funding (Zhang et al., 2022). Lee et al. (2022) found that ICT fosters technology innovation in the renewable energy sector by improving human capital and increasing information disclosure. Wang et al. (2023) suggested that governments prioritize green growth by increasing investment in ICT.
In summary, the existing studies thoroughly discusses the theoretical relationship between ER, including PEC, and GTI, while also presenting various empirical case studies. Additionally, the positive roles of GF and ICT in GTI are widely recognized. However, existing studies have overlooked that under PEC pressure, innovators can choose between IGTI and CGTI when facing cost constraints. Examining the effect of PEC on different types of GTI, particularly IGTI and CGTI, can help further identify the growth potential of GTI. Additionally, including GF and ICT in the research framework of PEC and GTI will help further unleash the positive impact of PEC on GTI.
GTI can only be effectively driven when PEC translates into active public participation. PEC typically leads to four forms of public participation. First, the public forms Environmental Non-Governmental Organizations. The organizations act as a third force that compensates for governmental and market failures, coordinate stakeholder interactions, and enhance public involvement in environmental governance (Wu et al., 2017). Second, the public directly negotiates with polluters or seeks judicial settlements. If negotiations fail, the public can seek help from the environment court, compelling polluters to increase environmental investments and GTI (Qi et al., 2023). Third, the public conveys environmental demands to higher government levels through open channels. A traditional method for public participation in environmental governance involves using open channels like letters and telegrams to seek help or apply pressure on the government, thereby expressing their environmental demands (Ren et al., 2018). Fourth, the public supports green products through market forces. Specifically, the public demonstrates a preference for green products by exercising stronger purchasing power in the commodity market and supporting environmentally friendly enterprises in the capital market (Ogiemwonyi et al., 2020; El Ouadghiri et al., 2021).
Once PEC evolves into public environmental participation, it influences GTI by affecting local governments and firms. Public environmental participation leads local governments to prioritize environmental governance. Recognizing the critical threat that environmental degradation poses to social and political stability, the Chinese government has prioritized environmental management (Liu et al., 2012). Meanwhile, many firms are actively engaging in green innovation behaviours to meet the public’s needs for the environment and thus gain public acceptance (Fu et al., 2022). Heavy polluters, in particular, mitigate the pressures of PEC by increasing their green investments (Gu et al., 2021). In conclusion, PEC effectively promotes GTI. Under PEC pressures, collaborating with other subjects in green technology projects is a beneficial way to share R&D costs, mitigate risks, access innovation resources and integrate varied knowledge (Van Beers and Zand, 2014; Hagedoorn et al., 2018). Meanwhile, some innovation subjects prefer to innovate independently in green technologies, as this avoids free-riding in the process of co-operation and maintains their unique competitive advantages (Wu et al., 2020; Nie et al., 2022).
Therefore, we propose three hypotheses:
H1a. PEC significantly promotes GTI.
H1b. PEC significantly promotes IGTI.
H1c. PEC significantly promotes CGTI.
Different innovation subjects face different degrees of R&D cost constraints due to R&D costs. The primary categories of innovation subjects include firms and research organizations, the latter comprising universities, government-affiliated research institutions, and research institutions affiliated with large firms. GTI in firms focuses on commercialization and end-product development, aiming to maximize profits but facing significant R&D cost constraints. Conversely, GTI in research organizations concentrates on basic R&D and upstream innovation, primarily conducting publicly-funded research projects (Von Raesfeld et al., 2012; Popp, 2017). Given that large firms seek a long-term competitive edge through green innovation (Huang et al., 2021), their affiliated research organizations can obtain specialized financial support. In summary, research organizations hold an advantage over firms in R&D cost investment when implementing IGTI.
The scope of innovation cooperation is the core factor influencing the cooperation cost. Although CGTI is effective in improving environmental performance and meeting the public’s environmental needs (Zhao et al., 2023), it faces cooperation cost constraints. Similarity between innovation subjects, termed “proximity,” can lower cooperation costs. Geographical proximity is the primary factor influencing cooperation (Zhou and Li, 2023). Inter-city cooperative innovation may incur higher communication and cooperation costs due to a lack of overall proximity among innovation subjects. In contrast, intra-city cooperative innovation benefits from geographical proximity and similarities in economic, cultural, and policy dimensions.
Therefore, we propose two hypotheses:
H2a. PEC is more likely to promote IGTI in research organizations than in firms.
H2b. PEC is more likely to promote CGTI within cities than between cities.
GF can convert the pressure and motivation from PEC into actionable GTI activities. To promote green development, China has implemented policies on GF (Ge et al., 2022; Afshan et al., 2023). First, the GF project selection mechanism directs capital towards green projects, ensuring long-term funding for GTI that meets its developmental needs and enhances innovation quality and quantity (Wang and Wang, 2021; Liu and Xiong, 2022). Second, GF establishes threshold criteria that restrict borrowing for heavily polluting firms, potentially forcing them to enhance their environmental practices and thus promote GTI (Zhang et al., 2022). Therefore, high levels of GF may contribute to the effect of PEC on GTI. Compared to IGTI, CGTI faces fewer constraints from R&D costs. Consequently, the impact of GF varies between IGTI and CGTI.
ICT applications create new opportunities for PEC and GTI. The Internet, a key component of ICT, not only enhances public concern in environmental matters, but also facilitates effective public participation in environmental governance and crucial monitoring of government and corporate behaviors (Ngai et al., 2020; Kang et al., 2021). Additionally, ICT enhances the efficiency of internal operations and external cooperation within innovation subjects. First, ICT drives the digitization of social activities (Zhang and Wang, 2019), boosts internal operational efficiency (Parida et al., 2016), and fosters GTI (Wei and Sun, 2021). Second, ICT reduces communication costs and removes time and space barriers for information resources, thus enhancing cross-regional integration efficiency (Bernard et al., 2019). It also creates a vital environment for cross-regional collaboration in GTI. Therefore, high levels of ICT may contribute to the effect of PEC on GTI. Compared to IGTI, CGTI faces more significant limitations due to cooperation costs. Consequently, ICT’s impact varies between IGTI and CGTI.
Therefore, we propose two hypotheses:
H3a. GF exhibits a threshold effect between PEC and GTI, varying between IGTI and CGTI.
H3b. ICT exhibits a threshold effect between PEC and GTI, varying between IGTI and CGTI.
This paper establishes a theoretical framework based on the above analysis in Figure 1.
This section outlines the data sources, quantifies indicators, and constructs regression models.
The Yangtze River Delta Region encompasses Shanghai, Jiangsu, Zhejiang, and Anhui provinces, including 41 cities. The selected data consists of panel data from 41 cities, spanning from 2011 to 2020. Data characterizing PEC is sourced from the Baidu platform (https://www.baidu.com/). Data on GTI, represented by patents, is obtained from the State Intellectual Property Office of China’s advanced search platform (https://www.cnipa.gov.cn/). And data for the other variables is derived from the China Urban Statistical Yearbook, Statistical Bulletin, and government annual financial reports. Missing data were addressed using interpolation.
Patents are commonly utilized as indicators of innovation in academic research (Lin and Ma, 2022; Wang, 2023). To ensure timeliness and authority, our process of screening and processing patents is as follows: First, select invention patents from three types of patents: utility model, design and invention. Second, choose invention patents that satisfy both timeliness and authority criteria. This study sets the patent application period from 1 January to 31 December of the respective year and the authorization period from 1 January of the application year to 31 May 2023. Third, identify green invention patents based on the WIPO’s 2010 green patent list. Fourth, match applicants and cities for green invention patents using the platforms Qichacha (https://www.qcc.com/) and Tianyancha (https://www.tianyancha.com/). Fifth, a city’s GTI, IGTI, and CGTI are measured using the following criteria: GTI is defined as the number of green invention patents held by the city; IGTI is the number of patents with a single applicant; and CGTI is the number of patents with two or more applicants.
Figure 2 illustrates the steps and details of the data processing.
Baidu is the most popular search engine in China, and its web search data can reflect public concern about specific events in a timely manner. Existing literature frequently uses Baidu’s keyword search index to measure PEC (Ren and Ren, 2024). In this study, Baidu’s search index for the keywords “haze” and “environmental pollution” was utilized.
The literature typically uses indicators like green credit, green securities, green insurance, and green investment to comprehensively measure GF in China, though primarily at the provincial level or higher (Ren et al., 2020; Wang and Wang, 2021; Ge et al., 2022). Given the available data and this study’s needs, we use the coupled coordination degree between green investment and financial development as a proxy for city-level GF. Specifically, we measure green investment using the ratio of energy-saving and environmental protection expenditures to total financial expenditures, and financial development by the ratio of loans to city deposits.
The literature commonly measures ICT based on Internet and telephone penetration rates (Lee et al., 2022). We select four indicators: the number of Internet broadband subscribers, mobile phone subscribers, the proportion of employees in the computer and software services sector, and per capita telecommunications service volume. We then use the entropy method to calculate a composite value.
This study identifies control variables across four dimensions: economic development, industrial structure, educational support, and financial environment. 1) Economic Development (ECO): Measured by the deflated gross regional product (in tens of billions of yuan). 2) Industrial Structure (STR): Defined by the ratio of value added in the tertiary industry to that in the secondary industry. 3) Educational Support (EDU): Represented by deflated government education expenditure (in tens of billions of yuan). 4) Financial Environment (FIN): Calculated as the ratio of year-end loan balances at financial institutions to regional GDP.
Table 1 shows the variables used.
To assess the effect of PEC on GTI, IGTI, and CGTI, we constructed a benchmark model based on related studies (Lee et al., 2022). This model included city and year dummy variables, which better address individual heterogeneity and time trends, while also reducing omitted variable bias and improving estimation accuracy. The benchmark model is presented in Equation 1.
where
To assess the threshold roles of GF and ICT, we constructed a threshold model based on related studies (Ge et al., 2024). The benchmark model helps us assess the average impact of PEC on GTI while overlooking potential structural issues. According to the threshold theory proposed by Hansen (1999), the threshold model can estimate the specific threshold value. The panel threshold model is presented in Equation 2.
where
Note that GTI, IGTI, and CGTI are count-type data. To fit both the benchmark and threshold models, this study standardizes all three variables. To manage the scale of the estimated coefficient for the core explanatory variable, this paper scales down PEC by a factor of 100.
Table 2 reflects significant disparities in GTI, IGTI, CGTI and PEC across the 41 cities. We employ two-dimensional scatter plots to clearly illustrate the relationships between GTI, IGTI, CGTI, and PEC, as shown in Figure 3. First, in all three plots, the majority of data points cluster in the lower left corner, suggesting significant potential for enhancing both PEC and green innovation capabilities. Second, a few points in the upper right corner indicate a strong likelihood that positive advancements in green innovation are driven by PEC. Lastly, the slope of the fitted line in each plot shows that GTI, IGTI, and CGTI each have a positive correlation with PEC.
This part empirically investigates the impact of PEC on GTI, IGTI, and CGTI. Initially, it assesses whether PEC influences GTI. Secondly, GTI is categorized into IGTI and CGTI, and the analysis determines if both categories are significantly impacted. In Table 3, columns (1) to (6) incorporate year and city fixed effects. Columns (4) to (6) also include control variables. This comprehensive control helps mitigate omitted variable bias, enhancing result accuracy.
The result in column (4) indicates that the coefficient of PEC is positive at the 5% significance level, signifying that PEC significantly enhances GTI. This occurs because, in the process of green innovation and development, the public can effectively supervise the market players to assume environmental responsibility. This prompts innovation subjects to engage in green innovation. Research hypothesis H1a is confirmed. The results in columns (5) and (6) indicate that the estimated coefficients of PEC are positive at the 5% and 10% significance levels, respectively, signifying that PEC also significantly increases IGTI and CGTI. This occurs because, under the pressure of PEC, some innovation subjects tend to pursue IGTI to enhance their competitiveness and establish a leading position in their fields. Other innovation subjects tend to pursue CGTI, which facilitates resource sharing and complementary advantages, while also sharing R&D costs and innovation risks. Research hypotheses H1b and H1c are confirmed. A comparison of the results in columns (5) and (6) reveals that the degree of influence and significance of PEC’s impact on IGTI and CGTI are inconsistent. This may result from the heterogeneity of the challenges faced by innovation subjects in the two different models of the innovation process. This will be further analyzed in the subsequent sections of this study.
The results for the control variables in Table 3 align with economic principles. Coefficients for ECO are significantly positive, suggesting that higher economic development promotes GTI, IGTI, and CGTI. Coefficients for STR positively affect GTI and IGTI but show no significant impact on CGTI, indicating a lack of positive influence on CGTI. Coefficients for EDU and FIN are significantly positive, demonstrating that stronger educational support and financial environment support GTI, IGTI, and CGTI. The regression results are generally consistent with economic logic, which justifies the model and variables.
To assess the robustness, we conducted several tests, including addressing endogeneity, altering the study duration, replacing the dependent and core independent variables, controlling the other ER.
To address endogeneity, we employed the lagged one-period PEC (L.PEC) as an instrumental variable, estimated using 2SLS. Results in Table 4, column (1), indicate that the coefficient of L.PEC are positive at the 1% level. Columns (2) to (4) confirm that PEC coefficients remain significantly positive, aligning with benchmark results. Additionally, the value of the Anderson LM statistic is 155.056, which rejects the original hypothesis of non-identifiable at a 1% significance level; the value of the Cragg-Donald Wald F statistic is 228.30, which is much larger than 16.38 (Stock-Yogo weak ID test critical values), rejecting the original hypothesis of a weak instrumental variable. That is, the choice of this instrumental variable is reasonable.
Given that patents may take one to 3 years to be authorized from the application date, some of the patents filed in 2020 may be authorized after 31 May 2023. Consequently, we excluded data from 2020 and conducted regressions using data from 2011 to 2019. Results in columns (5) to (7) of Table 4 support the conclusions drawn from the benchmark regression.
For patents with multiple applicants, we counted cooperations using a two-by-two crossover method. Measure CGTI (CGTI1) by the number of cooperative applications a city participates in; IGTI (IGTI1) by the number the city applies independently; and GTI (GTI1) by adding cooperative and independent applications. We conducted regressions with GTI1, IGTI1, and CGTI1 serving as proxy variables for the original dependent variables. The coefficients of PEC in columns (1) to (3) of Table 5 are significantly positive, consistent with the benchmark results.
Existing literature uses public environmental awareness as a proxy variable for PEC (Pargal and Wheeler, 1996; Zhou et al., 2019). We select five indicators based on data availability: the average wage of employed workers, per capita gross regional product, population density, the proportion of tertiary sector employees, and the percentage of college students. These indicators are integrated using the entropy method to measure PEA comprehensively. We performed regression analysis using city-level Public Environmental Awareness (PEA) as a proxy for PEC. The results from columns (4) to (6) in Table 5 support the conclusions drawn from the benchmark regression.
PEC is a form of public-led ER. Additionally, government-led ER (GER) also impacts GTI. This may influence the empirical findings of the benchmark regression.We measure GER by the frequency of environment-related words in the government’s annual work report. According to the results in columns (7) to (9) of Table 5, the benchmark results still hold when we control for GER. Furthermore, we find that GER inhibits GTI, consistent with existing literature (Jiao et al., 2024).
There is a known heterogeneity in the impact of PEC on IGTI and CGTI. This has been analyzed in the theoretical section, which may be attributed to the different constraints on the two. Specifically, while IGTI can achieve unique competitive advantages, it incurs significant R&D costs; in contrast, CGTI can share costs but must overcome cooperation barriers. To verify this, we analyze the heterogeneity of innovation subjects for IGTI and the heterogeneity of cooperation scope for CGTI within the context of PEC.
We categorize innovation subjects as research organizations and firms. The results in Table 6 indicate that the estimated coefficient for PEC in column (1) are insignificant, while it in column (2) is positive at the 1% significance level. This means PEC effectively contributes to IGTI in research organizations but not in firms. This is because research organizations typically have specialized financial support, whereas firms face more severe R&D cost constraints. These constraints prevent firms from converting the pressure of PEC into actual autonomous green innovation. Research hypothesis H2a is supported.
We categorize the scope of cooperation as intra-city and inter-city cooperation. The results in Table 6 indicate that the estimated coefficient for PEC in column (3) are significantly positive at the 5% level, while it in column (4) is not significant. This suggests that PEC effectively promotes CGTI within cities but not between cities. This is because innovation subjects within cities share similar institutional, historical, and cultural backgrounds, facilitating cooperation. In contrast, cooperation between cities lacks these advantages and is hindered by spatial and temporal constraints, which increase cooperation costs and impede CGTI. Research hypothesis H2b is supported.
R&D costs and cooperation costs are known constraints for IGTI and CGTI, respectively. The theoretical section analyzes how GF and ICT may alleviate the constraints on GTI and fully unleash its growth potential under PEC. To verify this, we conduct a panel threshold regression using GF and ICT as threshold variables and analyze the heterogeneity of their effects on IGTI and CGTI.
According to row (1) in Table 7, GF passed the single threshold test for the impact on PEC and GTI, with a threshold value of 0.303. Results from column (1) of Table 8 indicate that PEC’s coefficient is significantly positive at the 1% level only when GF exceeds 0.303. This suggests that only when GF reaches a certain threshold can PEC effectively facilitate GTI. This may be because GTI has a long cycle, high risk, and significant investment requirements, and the pressure of PEC is insufficient to promote GTI when GF levels are below the threshold. Only when GF levels reach the threshold can funds flow sufficiently to green R&D projects to facilitate green innovation activities.
Similarly, PEC is effective in promoting IGTI only when GF reaches a certain threshold based on the results in row (2) of Table 7 and column (2) of Table 8. But row (3) of Table 7 reveals that GF failed the single threshold test for the impact on PEC and CGTI. This confirms the previous analysis that the cooperative innovation model enables sharing of R&D costs and innovation risks, meaning GF does not have a significant threshold effect. In contrast, the independent innovation model faces greater constraints, so surpassing the GF threshold is crucial for providing financial security for IGTI. Research hypothesis H3a is confirmed.
Row (4) of Table 7 indicates that ICT passed the single threshold test for the impact on PEC and GTI, with a threshold value of 0.539. Results from column (4) of Table 8 reveal that PEC’s coefficient is significantly positive at the 5% level only when ICT exceeds 0.539. Two reasons explain this: first, advances in ICT have facilitated the expansion and application of the Internet, providing an important platform for the public to learn about environmental events and express concerns. Second, ICT promotes the digital transformation of innovation subjects, facilitating GTI activities.
Row (5) of Table 7 shows that ICT passed the single threshold test for the impact on PEC and IGTI, with a threshold value of 0.619. Column (5) of Table 8 shows that the PEC coefficient is 0.068 at the 10% significance level when ICT is below 0.619, and 0.174 at the 1% level when above 0.619. The marginal effect of PEC on IGTI and its significance increase with the enhancement of ICT. Row (6) of Table 7 indicates that ICT passed the single threshold test for the impact on PEC and CGTI, with a threshold value of 0.431. Column (6) of Table 8 reveals that PEC’s coefficient is significantly positive at the 5% level only when ICT exceeds 0.431. The threshold role of ICT is more stringent for CGTI than for IGTI. This is because cooperative innovation subjects may differ in institutional, historical, and cultural backgrounds, along with facing obstacles from spatial and temporal distances, leading to higher communication and cooperation costs. Since ICT plays a crucial role in enhancing communication efficiency, facilitating information sharing, and reducing time and spatial distances, cooperative green innovation activities impose stricter requirements on ICT. Research hypothesis H3b is confirmed.
(1) This study confirms that PEC can promote GTI, aligning with previous research (Li and Wang, 2021; Lin et al., 2024). In both China and other developing countries, governments play a crucial role in resource allocation and regulatory policies for environmental governance. Notably, as the concept of sustainable development becomes more ingrained in public consciousness, the public plays an increasingly vital role in achieving green development goals. Public participation can largely mitigate the issues of “market failure” and “government failure.” Additionally, we categorize GTI into IGTI and CGTI, finding that PEC significantly promotes both IGTI and CGTI, though the impacts exhibit heterogeneity. This aspect has been overlooked in existing studies. Clarifying the heterogeneity between the two in the context of PEC can further explore opportunities for improving GTI.
(2) IGTI can enhance unique competitiveness and achieve initial monopoly profits. Simultaneously, CGTI not only shares costs and risks but also acquires external heterogeneous knowledge, enhancing innovation quality. Therefore, both IGTI and CGTI are advantageous options for innovation subjects under PEC. However, analysis of the heterogeneity regression results reveals that IGTI under PEC is constrained by high R&D costs, while CGTI faces barriers to cooperation across city administrative boundaries. Overall, R&D and cooperation costs restrict innovation actors from converting the pressure of PEC into effective green innovation actions. Addressing how to alleviate R&D and cooperation cost constraints is the next question for discussion.
(3) Consistent with previous research (Lee et al., 2022; Wang T. et al., 2022), high levels of GF and ICT are beneficial for GTI. This aligns with China’s current policy orientation. Specifically, China actively promotes pilot policies for GF and “Broadband China”, aiming to enhance both GF and ICT levels to promote innovation and achieve green development. Additionally, this study finds that the threshold effect of GF exists only between PEC and IGTI, while the threshold effect of ICT is more stringent between PEC and CGTI. This indicates that GF can alleviate R&D cost constraints in IGTI, while ICT can reduce cooperation barriers in CGTI.
Exploring the growth potential of GTI under the pressure of PEC is highly valuable. PEC, as an informal ER, is a key driver of GTI. This study analyzes city panel data from 2011 to 2021 in China’s Yangtze River Delta region, categorizing GTI into IGTI and CGTI, and investigates the heterogeneous impacts of PEC on these types to unlock GTI’s growth potential.
The main findings of this study are: 1) PEC promotes GTI, with a greater effect on IGTI than on CGTI, indicating heterogeneity between the two. 2) Due to R&D cost constraints, PEC promotes IGTI in research organizations but fails to do so in firms. Due to cooperation cost constraints, PEC promotes CGTI within cities but not between them. 3) GF and ICT play a threshold role between PEC and GTI. The threshold effect of GF applies only to IGTI, suggesting that GF provides financial security for R&D investments in IGTI. The threshold effect of ICT is stricter in CGTI, indicating that widespread ICT application effectively reduces barriers to cooperation in collaborative innovation.
Based on these findings, this paper presents the following recommendations:
(1) Promote effective interaction between the public and innovation subjects. The public should actively translate their environmental concerns into practical participation in governance to positively impact GTI. Specific forms of participation include establishing Environmental Non-Governmental Organizations, negotiating with authorities, utilizing the judiciary, voicing demands to the government, and showing preference for green products in the marketplace. In the face of strong and ongoing PEC, innovation subjects should engage in green innovation activities, disclose information, and seek public recognition to enhance their environmental awareness and competitive advantage.
(2) Create a supportive external environment for PEC and GTI. While pursuing green financial policies, the government should enhance the targeting of GF. Given that IGTI faces significant R&D cost constraints, the green financial project selection mechanism should offer greater financial security for developing an independent R&D system. Additionally, the government should invest in enhancing and applying ICT. The government should leverage ICT to create an innovation platform that unites various green innovation subjects, promotes information exchange, shares technical ideas, and reduces barriers to cooperation and innovation.
This study has two areas where further improvement and expansion are needed. First, we outlined the theoretical mechanism through which PEC translates into public environmental participation, subsequently affecting GTI. However, empirical verification of this mechanism is lacking. Future studies can build upon this work. Second, since the Internet remains unfamiliar to some uneducated members of the public, the use of the Baidu index to measure PEC is limited. Future research should aim for a more comprehensive measurement.
The raw data supporting the conclusions of this article will be made available by the authors, without undue reservation.
SH: Conceptualization, Data curation, Methodology, Software, Writing–original draft. HD: Funding acquisition, Project administration, Writing–original draft. HJ: Formal Analysis, Software, Validation, Writing–review and editing. SQ: Data curation, Validation, Writing–review and editing.
The author(s) declare that financial support was received for the research, authorship, and/or publication of this article. This research was financially supported by National Social Science Fund of China (No. 22FJLB006).
The authors declare that the research was conducted in the absence of any commercial or financial relationships that could be construed as a potential conflict of interest.
All claims expressed in this article are solely those of the authors and do not necessarily represent those of their affiliated organizations, or those of the publisher, the editors and the reviewers. Any product that may be evaluated in this article, or claim that may be made by its manufacturer, is not guaranteed or endorsed by the publisher.
Afshan, S., Yaqoob, T., Meo, M. S., and Hamid, B. (2023). Can green finance, green technologies, and environmental policy stringency leverage sustainability in China: evidence from quantile-ARDL estimation. Environ. Sci. Pollut. Res. Int. 30 (22), 61726–61740. doi:10.1007/s11356-023-26346-1
Bernard, A. B., Moxnes, A., and Saito, Y. U. (2019). Production networks, geography, and firm performance. J. Polit. Econ. 127 (2), 639–688. doi:10.1086/700764
Berrone, P., Fosfuri, A., Gelabert, L., and Gomez-Mejia, L. R. (2013). Necessity as the mother of ‘green’ inventions: institutional pressures and environmental innovations. Strategic Manag. J. 34 (8), 891–909. doi:10.1002/smj.2041
Ebenstein, A., Fan, M., Greenstone, M., He, G., and Zhou, M. (2017). New evidence on the impact of sustained exposure to air pollution on life expectancy from China’s Huai River Policy. Proc. Natl. Acad. Sci. 114 (39), 10384–10389. doi:10.1073/pnas.1616784114
El Ouadghiri, I., Guesmi, K., Peillex, J., and Ziegler, A. (2021). Public attention to environmental issues and stock market returns. Ecol. Econ. 180, 106836. doi:10.1016/j.ecolecon.2020.106836
Fatima, T., Karim, M. Z. A., and Meo, M. S. (2021). Sectoral CO2 emissions in China: asymmetric and time-varying analysis. J. Environ. Plann. Manage. 64 (4), 581–610. doi:10.1080/09640568.2020.1776691
Fu, H., Zeng, S., and Sun, D. (2022). Top-down or bottom-up? How environmental state attention and civic participation coordinate with green innovation. Technol. Anal. Strateg. 36, 2187–2199. doi:10.1080/09537325.2022.2131515
Ge, L., Zhao, H., Yang, J., Yu, J., and He, T. (2022). Green finance, technological progress, and ecological performance—evidence from 30 Provinces in China. Environ. Sci. Pollut. Res. Int. 29 (44), 66295–66314. doi:10.1007/s11356-022-20501-w
Ge, L., Zheng, H., Wang, C., Ye, Y., and Xu, Z. (2024). The impact of foreign direct investment on green technology innovation: evidence from the threshold effect of absorptive capacity. J. Urban Technol. 31, 107–130. doi:10.1080/10630732.2024.2385116
Gu, Y., Ho, K. C., Yan, C., and Gozgor, G. (2021). Public environmental concern, CEO turnover, and green investment: evidence from a quasi-natural experiment in China. Energy Econ. 100, 105379. doi:10.1016/j.eneco.2021.105379
Hagedoorn, J., Lokshin, B., and Zobel, A. K. (2018). Partner type diversity in alliance portfolios: multiple dimensions, boundary conditions and firm innovation performance. J. Manag. Stud. 55 (5), 809–836. doi:10.1111/joms.12326
Hansen, B. E. (1999). Threshold effects in non-dynamic panels: estimation, testing, and inference. J. Econom. 93 (2), 345–368. doi:10.1016/S0304-4076(99)00025-1
Hassan, M. S., Meo, M. S., Abd Karim, M. Z., and Arshed, N. (2020). Prospects of environmental Kuznets curve and green growth in developed and developing economies. Stud. Appl. Econ. 38 (3). doi:10.25115/eea.v38i3.3367
Huang, J., Xiang, S., Wang, Y., and Chen, X. (2021). Energy-saving R&D and carbon intensity in China. Energy Econ. 98, 105240. doi:10.1016/j.eneco.2021.105240
Jiao, H., Deng, H., and Hu, S. (2024). Driving green technology innovation: the impacts of heterogeneous environmental regulation and digital financial inclusion. Front. Environ. Sci. 12, 1392419. doi:10.3389/fenvs.2024.1392419
Kang, A., Ren, L., Hua, C., Dong, M., Fang, Z., and Zhu, M. (2021). Stakeholders’ views towards plastic restriction policy in China: based on text mining of media text. Waste. Manage. 136, 36–46. doi:10.1016/j.wasman.2021.09.038
Lee, C. C., He, Z. W., and Xiao, F. (2022). How does information and communication technology affect renewable energy technology innovation? International evidence. Renew. Energ. 200, 546–557. doi:10.1016/j.renene.2022.10.015
Li, C., and Wang, S. (2022). Digital optimization, green R&D collaboration, and green technological innovation in manufacturing enterprises. Sustainability 14 (19), 12106. doi:10.3390/su141912106
Li, X., Xu, Q., and Wang, H. (2023). Environmental effects of the establishment of pilot free trade zones: evidence from Chinese resource-based enterprises. Environ. Sci. Pollut. Res. Int. 30 (8), 21384–21403. doi:10.1007/s11356-022-23722-1
Li, Y., and Wang, Z. (2021). Will public environmental concerns foster green innovation in China’s automotive industry? An empirical study based on multi-sourced data streams. Front. Energy Res. 9, 623638. doi:10.3389/fenrg.2021.623638
Lin, B., Li, B., and Zhang, D. (2024). ESG implementation, public environmental concern, and corporate green technology innovation: analysis based on the background of China’s. Environ. Dev. Sustain., 1–38. doi:10.1007/s10668-024-04670-x
Lin, B., and Ma, R. (2022). Green technology innovations, urban innovation environment and CO2 emission reduction in China: fresh evidence from a partially linear functional-coefficient panel model. Technol. Forecast. Soc. Change 176, 121434. doi:10.1016/j.techfore.2021.121434
Liu, B., Wang, J., Li, R. Y. M., Peng, L., and Mi, L. (2022). Achieving carbon neutrality–the role of heterogeneous environmental regulations on urban green innovation. Front. Ecol. Evol. 10, 923354. doi:10.3389/fevo.2022.923354
Liu, C., and Xiong, M. (2022). Green finance reform and corporate innovation: evidence from China. Finance Res. Lett. 48, 102993. doi:10.1016/j.frl.2022.102993
Liu, J., Zhao, M., Zhang, C., and Ren, F. (2023). Analysis of the influence of heterogeneous environmental regulation on green technology innovation. Sustainability 15 (4), 3649. doi:10.3390/su15043649
Liu, L., Zhang, B., and Bi, J. (2012). Reforming China’s multi-level environmental governance: lessons from the 11th Five-Year Plan. Environ. Sci. Policy 21, 106–111. doi:10.1016/j.envsci.2012.05.001
Meo, M. S., Nathaniel, S. P., Khan, M. M., Nisar, Q. A., and Fatima, T. (2023). Does temperature contribute to environment degradation? Pakistani experience based on nonlinear bounds testing approach. Glob. Bus. Rev. 24 (3), 535–549. doi:10.1177/0972150920916653
Ngai, C. S. B., Singh, R. G., and Lu, W. (2020). Exploring drivers for public engagement in social media communication with medical social influencers in China. Plos one 15 (10), e0240303. doi:10.1371/journal.pone.0240303
Nie, P. Y., Wen, H. X., and Wang, C. (2022). Cooperative green innovation. Environ. Sci. Pollut. Res. Int. 29 (20), 30150–30158. doi:10.1007/s11356-021-18389-z
Ogiemwonyi, O., Harun, A. B., Alam, M. N., Karim, A. M., Tabash, M. I., Hossain, M. I., et al. (2020). Green product as a means of expressing green behaviour: a cross-cultural empirical evidence from Malaysia and Nigeria. Environ. Technol. Innov. 20, 101055. doi:10.1016/j.eti.2020.101055
Ouyang, X., Li, Q., and Du, K. (2020). How does environmental regulation promote technological innovations in the industrial sector? Evidence from Chinese provincial panel data. Energy Policy 139, 111310. doi:10.1016/j.enpol.2020.111310
Pargal, S., and Wheeler, D. (1996). Informal regulation of industrial pollution in developing countries: evidence from Indonesia. J. Polit. Econ. 104 (6), 1314–1327. doi:10.1086/262061
Parida, V., Oghazi, P., and Cedergren, S. (2016). A study of how ICT capabilities can influence dynamic capabilities. J. Enterp. Inf. Manag. 29 (2), 179–201. doi:10.1108/JEIM-07-2012-0039
Petroni, G., Bigliardi, B., and Galati, F. (2019). Rethinking the Porter hypothesis: the underappreciated importance of value appropriation and pollution intensity. Rev. Policy Res. 36 (1), 121–140. doi:10.1111/ropr.12317
Popp, D. (2017). From science to technology: the value of knowledge from different energy research institutions. Res. Policy 46 (9), 1580–1594. doi:10.1016/j.respol.2017.07.011
Porter, M. E., and Linde, C. V. D. (1995). Toward a new conception of the environment-competitiveness relationship. J. Econ. Perspect. 9 (4), 97–118. doi:10.1257/jep.9.4.97
Qi, X., Wu, Z., Xu, J., and Shan, B. (2023). Environmental justice and green innovation: a quasi-natural experiment based on the establishment of environmental courts in China. Ecol. Econ. 205, 107700. doi:10.1016/j.ecolecon.2022.107700
Ramanathan, R., Black, A., Nath, P., and Muyldermans, L. (2010). Impact of environmental regulations on innovation and performance in the UK industrial sector. Manag. Decis. 48 (10), 1493–1513. doi:10.1108/00251741011090298
Ren, S., Li, X., Yuan, B., Li, D., and Chen, X. (2018). The effects of three types of environmental regulation on eco-efficiency: a cross-region analysis in China. J. Clean. Prod. 173, 245–255. doi:10.1016/j.jclepro.2016.08.113
Ren, X., and Ren, Y. (2024). Public environmental concern and corporate ESG performance. Finance Res. Lett. 61, 104991. doi:10.1016/j.frl.2024.104991
Ren, X., Shao, Q., and Zhong, R. (2020). Nexus between green finance, non-fossil energy use, and carbon intensity: empirical evidence from China based on a vector error correction model. J. Clean. Prod. 277, 122844. doi:10.1016/j.jclepro.2020.122844
Shaari, M. S., Abidin, N. Z., Ridzuan, A. R., and Meo, M. S. (2021). The impacts of rural population growth, energy use and economic growth on CO2 emissions. Int. J. Energy Econ. Policy 11 (5), 553–561. doi:10.32479/ijeep.11566
Suki, N. M., Afshan, S., Sharif, A., Kasim, M. A., and Hanafi, S. R. M. (2022). How does green technology innovation affect green growth in ASEAN-6 countries? Evidence from advance panel estimations. Gondwana Res. 111, 165–173. doi:10.1016/j.gr.2022.06.019
Van Beers, C., and Zand, F. (2014). R&D cooperation, partner diversity, and innovation performance: an empirical analysis. J. Prod. Innov. Manage. 31 (2), 292–312. doi:10.1111/jpim.12096
Von Raesfeld, A., Geurts, P., Jansen, M., Boshuizen, J., and Luttge, R. (2012). Influence of partner diversity on collaborative public R&D project outcomes: a study of application and commercialization of nanotechnologies in The Netherlands. Technovation 32 (3-4), 227–233. doi:10.1016/j.technovation.2011.12.001
Wang, F. (2023). The intermediary and threshold effect of green innovation in the impact of environmental regulation on economic Growth: evidence from China. Ecol. Indic. 153, 110371. doi:10.1016/j.ecolind.2023.110371
Wang, L., Long, Y., and Li, C. (2022a). Research on the impact mechanism of heterogeneous environmental regulation on enterprise green technology innovation. J. Environ. Manage. 322, 116127. doi:10.1016/j.jenvman.2022.116127
Wang, T., Liu, X., and Wang, H. (2022b). Green bonds, financing constraints, and green innovation. J. Clean. Prod. 381, 135134. doi:10.1016/j.jclepro.2022.135134
Wang, X., and Wang, Q. (2021). Research on the impact of green finance on the upgrading of China’s regional industrial structure from the perspective of sustainable development. Resour. Policy 74, 102436. doi:10.1016/j.resourpol.2021.102436
Wang, Z., Huang, Y., Ankrah, V., and Dai, J. (2023). Greening the knowledge-based economies: harnessing natural resources and innovation in information and communication technologies for green growth. Resour. Policy 86, 104181. doi:10.1016/j.resourpol.2023.104181
Wei, Z., and Sun, L. (2021). How to leverage manufacturing digitalization for green process innovation: an information processing perspective. Ind. Manag. Data Syst. 121 (5), 1026–1044. doi:10.1108/IMDS-08-2020-0459
Wu, J., Chang, I. S., Yilihamu, Q., and Zhou, Y. (2017). Study on the practice of public participation in environmental impact assessment by environmental non-governmental organizations in China. Renew. Sust. Energ. Rev. 74, 186–200. doi:10.1016/j.rser.2017.01.178
Wu, Y., Gu, F., Ji, Y., Guo, J., and Fan, Y. (2020). Technological capability, eco-innovation performance, and cooperative R&D strategy in new energy vehicle industry: evidence from listed companies in China. J. Clean. Prod. 261, 121157. doi:10.1016/j.jclepro.2020.121157
Xiong, R., and Gao, D. (2023). Exploring the impact of heterogeneous environmental regulations on green innovation efficiency: evidence from China’s Yangtze River Economic belt. Systems 11 (10), 516. doi:10.3390/systems11100516
Zhang, C., and Wang, X. (2019). The influence of ICT-driven innovation: a comparative study on national innovation efficiency between developed and emerging countries. Behav. Inf. Technol. 38 (9), 876–886. doi:10.1080/0144929X.2019.1584645
Zhang, Y., Li, X., and Xing, C. (2022). How does China’s green credit policy affect the green innovation of high polluting enterprises? The perspective of radical and incremental innovations. J. Clean. Prod. 336, 130387. doi:10.1016/j.jclepro.2022.130387
Zhao, Y., Zhao, Z., Qian, Z., Zheng, L., Fan, S., and Zuo, S. (2023). Is cooperative green innovation better for carbon reduction? Evidence from China. J. Clean. Prod. 394, 136400. doi:10.1016/j.jclepro.2023.136400
Zhou, Q., Zhang, X., Shao, Q., and Wang, X. (2019). The non-linear effect of environmental regulation on haze pollution: empirical evidence for 277 Chinese cities during 2002–2010. J. Environ. Manage. 248, 109274. doi:10.1016/j.jenvman.2019.109274
Zhou, W., and Li, H. (2023). A study on the multidimensional driving mechanism of cross-regional scientific collaboration network in China. Technol. Anal. Strateg. Manag., 1–15. doi:10.1080/09537325.2023.2220824
Keywords: public environmental concern, independent green technology innovation, cooperative green technology innovation, green finance, information and communication technology
Citation: Hu S, Deng H, Jiao H and Qin S (2025) Impact of public environmental concern on green technology innovation: a case study of the Yangtze River Delta in China. Front. Environ. Sci. 12:1465619. doi: 10.3389/fenvs.2024.1465619
Received: 16 July 2024; Accepted: 16 December 2024;
Published: 07 January 2025.
Edited by:
Martin Siegert, University of Exeter, United KingdomReviewed by:
Muhammad Saeed Meo, Sunway University, MalaysiaCopyright © 2025 Hu, Deng, Jiao and Qin. This is an open-access article distributed under the terms of the Creative Commons Attribution License (CC BY). The use, distribution or reproduction in other forums is permitted, provided the original author(s) and the copyright owner(s) are credited and that the original publication in this journal is cited, in accordance with accepted academic practice. No use, distribution or reproduction is permitted which does not comply with these terms.
*Correspondence: Hongbing Deng, ZGVuZzExNUBjdWcuZWR1LmNu
Disclaimer: All claims expressed in this article are solely those of the authors and do not necessarily represent those of their affiliated organizations, or those of the publisher, the editors and the reviewers. Any product that may be evaluated in this article or claim that may be made by its manufacturer is not guaranteed or endorsed by the publisher.
Research integrity at Frontiers
Learn more about the work of our research integrity team to safeguard the quality of each article we publish.