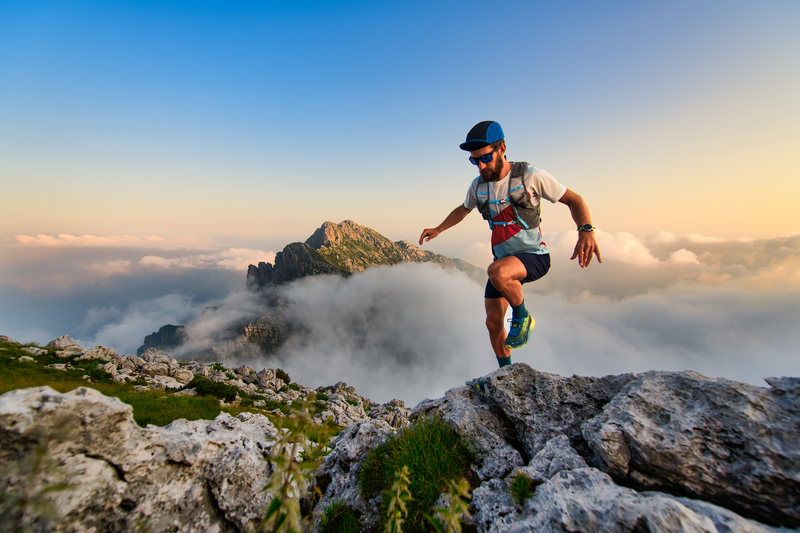
94% of researchers rate our articles as excellent or good
Learn more about the work of our research integrity team to safeguard the quality of each article we publish.
Find out more
ORIGINAL RESEARCH article
Front. Environ. Sci. , 26 November 2024
Sec. Environmental Economics and Management
Volume 12 - 2024 | https://doi.org/10.3389/fenvs.2024.1463833
With the widespread promotion of the concept of green development, China’s green credit policy system has been established, developed, and gradually improved during the past decade. Against the background of the country’s vigorous development of green finance, this finance has had an increasingly important influence on agricultural green total factor productivity (GTFP). In this study, we took 30 provinces (autonomous regions or municipalities directly under central government control) in China as research samples (Hong Kong, Macao, Taiwan, and Tibet were not included due to a lack of data). The time period from 2010 to 2020 was selected as the research period, given that 2010 was the year when the development stage of China’s green finance was first initiated. Through in-depth analysis of the spatial correlations of agricultural GTFP in China and the influences of green finance on agricultural GFTP, we constructed a research framework with multiple dimensions, including green credit, green bonds, green insurance, green investment, and carbon finance. We then systematically studied the influences of green finance on agricultural GTFP. Our results showed that: (1) The development levels of green finance and agricultural GTFP in China were high, but there were not able differences among provinces, with higher agricultural GTFP in northern China and lower agricultural GTFP in central China; (2) green finance had the greatest promoting effect in western China, a weaker promoting effect in central China, and the weakest promoting effect in eastern China; and (3) green finance can indirectly promote improvements in agricultural GTFP by promoting the upgrading of industrial structure, driving technological progress, and optimizing energy consumption structure. Our work not only provides valuable reference data and suggestions for the green and sustainable development of China’s agriculture but also academic support for the development of China’s agricultural economy.
Following the adoption of the UN’s Sustainable Development Goals and the Paris Agreement in 2015, the international development of green finance was included as part of the core agenda at the G20 Summit for the first time in 2016. This accelerated the pace of green development in both the public and private sectors of multiple economies. Since 2020, green finance has gradually become part of an international consensus. Under the dual boost of the 15th Conference of the Parties (COP15) to the Convention on Biological Diversity in 2021 and the 26th Conference of the Parties (COP26) to the United Nations Framework Convention on Climate Change, green finance has been accepted as an important tool to address climate change and protect biodiversity and has achieved penetration into global capital markets. In 2021, the volume of global green financing reached a record of $540.6 billion, indicating that today’s green finance has moved to center stage in global capital markets.
In recent years, with the increasing awareness of environmental and social responsibilities, the concept of green development has been widely promoted in China, especially in the field of agriculture. The promotion of agricultural green total factor productivity (GTFP) has become an inevitable requirement for China’s sustainable development (Shi, 2022). The report of the 19th National Congress of the Communist Party of China clearly stated that “high-quality development is the primary task of comprehensively building a modern socialist country”. As the world’s largest agricultural producer and consumer, China’s agricultural production has become an important part of the global food security system, and it is imperative to promote a transformation in China’s agricultural economy from large quantity to high quality (Hong, 2017; Du and Lingxiao, 2023). The top-level design of China’s green finance has now essentially finished, and related business is developing rapidly, promoting economic and social transformation and upgrading. Since 2012, the Chinese government has incorporated green development into its national strategy and promoted the rapid development of green finance. In 2020, the President of China, Xi Jinping, announced the “3,060” dual-carbon goals at the United Nations General Assembly, which showed the direction for promoting the development of green finance in China.
According to the White paper on China’s Green Development in the New Era (2023), China’s fiscal expenditure on energy conservation and environmental protection increased from 236.07 billion yuan in 2010 to 692.82 billion yuan in 2019, with a year-on-year growth rate of 193.48% and an average annual growth rate of 21.49%. By the end of 2020, the outstanding green credit of major Chinese banks had exceeded 10 trillion yuan, and they had issued 229.754 billion yuan of green bonds. China has become the world’s largest issuer of green bonds. In 2022, green financial products will perform well, and green credit and green bonds will make remarkable progress. By December 2022, the balance of green credit in China has reached 22.03 trillion yuan, with a year-on-year growth rate of 38.5%, 5.5 percentage points higher than that in 2021, with a relatively balanced and diversified structure. In 2022, the issuance of labeled green bonds in China and abroad was us $155 billion (RMB 1 trillion yuan), up 35% year on year. Among them, the issuance of green bonds meeting the CBI definition was US $85.4 billion (RMB 575.2 billion yuan), ranking first in the world. At the same time, most of China’s green bonds will mature by 2025, and the issuance scale still has the potential for growth. Affected by the volatility of the capital market, the number and scale of environmental-themed and ESG-themed funds have declined, but the number and scale of funds in new energy and other industries have increased. The implementation plan for carbon peak reaching compiled in 2021 was released in 2022, and the local or regional peak reaching implementation plan was not disclosed. By the end of 2022, a total of 18 provinces, municipalities and autonomous regions had announced plans, including those in energy, industry, urban and rural construction, agriculture and rural areas, as well as non-ferrous metals and building materials industries. The dual-carbon policy system has been further improved, bringing about a boom in investment in carbon neutrality. The first batch of carbon neutral ETF issuance reached 16.4 billion yuan; ABS market is closer to the concept of “carbon neutral” and the field concentration increases; China issued carbon neutral bonds totaling 470.56 billion yuan. 3, accounting for 33.2% of the total issuance and scale of green bonds. In the futures market, 16 futures varieties, including carbon emission right, electricity and other major strategic varieties related to the basic fields of national economy and energy price reform, indicating that carbon financial futures trading is about to begin.
Green finance plays an important role in promoting and guiding the green development of rural agriculture. According to the overall requirements of rural revitalization, financial institutions can provide diversified financial services, such as green funds, bonds, and credits; these can then be used to meet green, environmentally-friendly, and sustainable financial needs (Chen, 2021). Green finance can promote the green development of agriculture and simultaneously has a strong spillover effect. This means that agricultural GTFP in a given region will be affected by the development of green finance in local and neighboring regions (Xiao and Hu, 2023). Green finance is a driving force for the construction of rural ecological civilization and an important mechanism that affects rural ecological civilization. Consequently, increasing agricultural GTFP can improve the level of rural ecological civilization (Zhu and Zhang, 2023). Green finance can also improve the degree of food security by improving rural human capital and accelerating the agglomeration of agricultural industries (Jiangiang et al., 2024). In regions with a greater degree of marketization and more human capital, green finance can have a greater influence on rural revitalization (Tingting and Meiju, 2023). From the perspectives of increasing supply-side structural reform in the financial field, providing “agriculture, rural areas, and farmers” with a high-quality service, and achieving its own sustainable development, it is an inevitable choice to use green finance to support rural revitalization (Song, 2023). Green finance is a financial investment concept with environmental protection and pollution control as its core ideas, and it aims to achieve coordination and cooperation between financial and industrial policies (Gao, 2019). Green finance is a financial tool that follows market rules and plays an important role in improving the efficiency of agricultural production and protecting the ecological environment (Ji, 2023). By promoting the rational allocation of agricultural resources, green finance can enhance agricultural green productivity, thus promoting the upgrading of agricultural industry, improving agricultural productivity, optimizing the utilization efficiency of agricultural production resources, strengthening scientific and technological innovation in agriculture, and increasing farmers’ income (Qin, 2023; Zhao, 2023). In general, green finance has a positive influence on the level of integration of rural tertiary industry, and green investment and green insurance can effectively enhance the level of integration of green securities, carbon finance, and green credit. Green finance can increase consumer spending by rural residents, while fiscal expenditure, per capita GDP, and urbanization rate can also have a positive influence on consumer spending by rural residents (Yan and Wu, 2024; Fang, 2023).
Therefore, the study of this paper is to investigate the background of China’s green credit policy system in a short period of time, green finance to the green agricultural total factor productivity (GTFP) influence how associated, on China’s vast land area, regional environment difference between regional influence, on the influence of the green sustainable development of Chinese agricultural effect.
Compared with other relevant studies, the research contributions and innovations of this paper are mainly reflected in the following aspects: at the theoretical level, it enrich and improve the theoretical system of agricultural socialized service, helps to discuss the connotation, extension, practice path and development law of agricultural socialized service in the exploratory period of green finance promotion, and forms a theory of agricultural modernization and sustainable development with Chinese characteristics. At the practical level, consider agricultural socialization services to promote the modernization of green agriculture, improve agricultural production efficiency and farmers’ income, and enhance competitiveness. By improving the agricultural socialization service system, we can solve agricultural problems, strengthen agricultural economy, promote rural development, achieve sustainable agricultural development, and provide academic support for the development of China’s agricultural economy.
The research structure of this paper is as follows: first, with the help of GIS technology and spatial analysis model, combined with data envelope analysis method (DEA), at the same time, the study of time and space from the two dimensions of time and space. Secondly, with the help of the spatial Dubin model, there are relatively few studies on the impact of green finance on agricultural total factor productivity, and it is still in the preliminary exploration stage. This study in China 30 provinces (autonomous regions and municipalities directly under the Central Government) as the research object (Tibet, Hong Kong, Macao, Taiwan, due to the lack of data, not included in the target area of the study), using GIS technology and EBM-GML model, global space autocorrelation analysis, cold hotspot analysis, spatial Dubin model quantitative analysis, measurement model and index system, the level of Chinese agricultural green total factor productivity comprehensive measurement analysis, and further carry out green finance on agricultural green total factor productivity analysis.
Against the background of the dual-carbon goals, it is necessary to replace traditional total factor productivity (TFP) with agricultural GTFP, to promote the construction of a more ecological civilization. Agricultural GTFP is a key indicator that can be used to measure agricultural economic growth and environmental sustainability. The methods of measurement include the non-production frontier method and the production frontier method, of which the non-production frontier method has been more extensively studied. Conradie et al. (2021) extended the study of agricultural TFP in the Western Cape Province of South Africa to 11 Northern Cape regions. Hamid and Wang (2022) believed that it is crucial to assess agricultural TFP and that the factors influencing agricultural growth and environmental performance could be determined by combining environmental factors with production efficiency. Shiwen and Hu (2019),using the Solow residual method, found that the contribution rate of agricultural scientific and technological progress in Jiangxi Province, China, was closely related to capital input and that scientific and technological development and capital input were the key factors in promoting agricultural economic development. Huang (2021) believed that the growth of the agricultural green economy could mainly be attributed to technological progress and that it was also necessary to pay attention to the protection of natural resources and the environment. Wang and Feng (2013) studied the agricultural production input from 1995 to 2008 in Liaocheng City, China. They found that the government played a major role, whereas the market had a weak influence; the functions of various agricultural factors were well coordinated, and the agricultural production value increased steadily. Based on their findings, the authors suggested that more support should be given to improve agricultural GTFP. Peng-fei et al. (2018) measured the agricultural GTFP of various provinces in China according to the Luenberger index. They found that agricultural GTFP was successively lower in the eastern, central, and western regions and was successively lower in the main grain producing areas, main sales areas, and balance areas. Zhao and Dang (2023) used a model research method to study the agricultural GTFP of 77 counties and districts in Anhui Province, China, over the past 10 years; they concluded that different counties and districts had differentdegrees of demand and dependence on different factors. Bo and Bao (2023) measured the agricultural GTFP of various provinces in the Yellow River Basin using a slacks-based measure (SBM)-graph machine learning (GML) index model; they found that there were considerable differences in the agricultural production structure among different provinces. Zhang and Sun (2023) studied the influence of agricultural global value chains on total factor productivity in 58 countries. They found that agricultural global value chains had an improvement effect on TFP in high-and medium-to-high-income countries, but no obvious effect on TFP in low-to-medium-income countries. Zhang et al. (2022) analyzed the influences of seven variables on agricultural GTFP and determined which of these variables had direct and indirect influences. Chen et al. (2020) believed that problems in agricultural development could be identified by studying temporal and spatial changes in agricultural total factor productivity.
Agricultural TFP represents agricultural production efficiency. Improving agricultural production efficiency is of great importance for agricultural development. The higher the agricultural TFP, the less dependent the agriculture is on resource factors, the more technologically advanced the agriculture, and the greater the degree of agricultural sustainability. Hence, academics and governments alike have focused on the improvement of agricultural TFP. Jianiun et al. (2023) found that digital rural construction could promote scale management of agriculture and promote the improvement of agricultural GTFP. Xie et al. (2023a) suggested that inclusive rural finance could help farmers obtain increased financial resources, promote the development of agricultural industrialization, and thus considerably improve agricultural GTFP. Li et al. (2022) found that environmental regulations could enhance the influence of farmland water conservancy facilities on the TFP of grain production. Using a spatial panel model, Yang and Tong (2023) conducted an empirical study of the influence of agricultural trade on agricultural GTFP in 31 provinces, autonomous regions, and municipalities in China from 2004 to 2020. Theyfound that agricultural trade had distinct “U-shaped” rules that influenced the technical efficiency of agriculture. Sheng et al. (2016) used the growth accounting method to assess the TFP of the Australian agricultural industry between 1949 and 2012, and improved the measured results of agricultural productivity by considering the heterogeneity of output and input quality. Using the Solow residual method, Moghaddasi and Pour (2016) found that consumption of energy by agriculture in Iran between1974 and 2012 was negatively correlated with the growth of TFP, i.e., energy consumption increased while TFP decreased. Based on survey data from pastoral areas of Inner Mongolia, Zhang and Zhang (2022) found that the improvement in TFP of livestock husbandry in pastoral areas as a result of the grassland ecological compensation policy had a positive effect on the harmonious development of livestock production and grassland ecology in pastoral areas. Anubhab et al. (2021) analyzed the evolution of the influence of climate change on agricultural TFP in various regions of India and estimated that agricultural TFP in all states considered in their study would decline by 2050. Lajos et al. (2020) studied the influence of various types of subsidies on different components of agricultural TFP in Slovenia. Ghebremichael and Potter-Witter (2009) studied the influence of tax incentives on the long-term dynamics of TFP growth and capital formation in Canada’s sawmill industry and found that increased capital formation could considerably increase the total input when driven by tax incentives. Olomola and Osinubi (2018) found that human capital was always the main driving force of national agricultural TFP in both the short- and long-term.
In summary, previous studies related to agricultural TFP have made some achievements, but some shortcomings remain. First, there have been few studies on the spatio-temporal comprehensive analysis for the evolution laws and characteristics of agricultural GTFP from both temporal and spatial dimensions that have involved GIS technology and spatial analysis models using the data envelopment analysis (DEA) method. Second, there have been relatively few studies that have used the spatial Durbin model to analyze the influence of green finance on agricultural TFP, with such research still in the preliminary exploration stages. In this study, we took30 provinces (autonomous regions and municipalities directly under the control of the central government) in China as the research objects. We constructed a measure model and index system using GIS technology and various quantitative analysis methods, including explainable boosting machine-graph machine learning (EBM-GML) model, global spatial autocorrelation analysis, cold-and hot-spot analysis, and the spatial Durbin model. (Tibet, Hong Kong, Macao, and Taiwan were not included in the targeted regions of this study due to a lack of data.) We then conducted a comprehensive spatio-temporal measure analysis of the level of agricultural GTFP in China; we also analyzed the influence of green finance on agricultural GTFP. At a theoretical level, our study enriches and improves the theory of agricultural socialized service, and is helpful to explore the connotation, extension, practice paths, and development laws of agricultural socialized service, which facilitates the formation of a theory for agricultural modernization and sustainable development with Chinese characteristics. At the practical level, agricultural socialized services promote agricultural modernization, improves agricultural production efficiency, increases farmers’ income, and enhances agricultural competitiveness. By improving the agricultural socialized service system, we can solve agricultural problems, strengthen the agricultural economy, promote rural development, and achieve sustainable agricultural development. Our work thus provides academic support for the development of China’s agricultural economy.
The main content of this paper is to study the impact of green finance on agricultural green total factor productivity. This paper discusses the impact mechanism of green finance on agricultural green total factor productivity from four aspects, and proposes the research hypothesis through the analysis of the impact mechanism.
(1) Through the collation and analysis of existing literature, it is found that green finance has positive effect to agricultural green total factor productivity, Shi and Shi (2022) points out that the improvement of green financial development level can promote the improvement of green total factor productivity: Xie and Shihua (2023) research found that green finance through various green financial tools for reasonable allocation of resources can effectively improve green total factor productivity. Green finance is to promote the development of agricultural green through the rational application of green finance has a supervision and management role. After farmers obtain financial services, the use of funds must be used for agricultural green production and related aspects, which ensures that farmers invest funds into green production and guarantee the productivity of green finance.
Hypothesis 1. green finance has a significant positive impact on green total factor productivity in agriculture.
(2) Through the collation and analysis of existing literature, it is found that green finance promotes agricultural green total factor productivity regional heterogeneity, Xie et al. found that green finance can effectively improve green total factor productivity, and regional heterogeneity in the central and western regions; Liu and Chun (2021a) proposed that the development level of green finance on agricultural green total factor production has significant heterogeneity, which shows that the eastern region is greater than the central region and the western region, and the western region has the least influence. Although there is regional heterogeneity in the effect of green finance, green finance still promotes the agricultural green total factor productivity in eastern, central and western China, due to the different levels of economic development among different regions. However, with the continuous advancement of the new round of central region rise strategy and the western development strategy, the differences between the eastern, central and western regions will gradually narrow and tend to be consistent.
Hypothesis 2. the influence of green finance on agricultural green total factor productivity is heterogeneous.
(3) Green finance has an impact on agricultural green total factor productivity by promoting technological progress. Because green finance will guide the flow of capital from energy-intensive and polluting industries to enterprises with low energy consumption and advanced technology, First of all, green credit can broaden the channels for related enterprises to obtain funds, So that environmental protection enterprises have sufficient funds to research and develop new technologies; Secondly, green production enterprises can give priority to issuing green bonds, Enable enterprises to conduct direct financing, On the one hand, it can not only change the financing environment of green and environmental protection enterprises, It can also improve the development confidence of green and environmental protection enterprises themselves, On the other hand, it can improve the infrastructure construction of enterprises, To promote its better technological innovation: the government will also actively participate in the green finance activities, Provide risk compensation and guarantee subsidies for green technology innovation enterprises, It will also improve the enthusiasm of enterprises to make green technology innovation. Finally, relevant departments have strict approval systems and supervision and management for all kinds of green financial instruments, which further ensures that green finance encourages enterprises to improve green technology by providing financial support for environmental protection enterprises, so as to have a positive impact on the green total factor productivity of agriculture. Cui and Peng (2023) believed that green finance promotes the improvement of green total factor productivity through green R & D investment; Liu (2021); Liu and Chun (2021b) concluded that improving the development level of green finance can promote green technology innovation.
(4) Through the collation and analysis of existing literature, it is found that green finance for agricultural green whole orderly productivity has significant space spillover effect, the green financial region green agricultural total factor productivity promoting effect at the same time, also to its adjacent areas of agricultural green total factor productivity. Now the economic development between more and more close, the economic development of the region will also promote the economic development of adjacent areas, with the higher the green financial level of the region will drive the development of green financial level of adjacent areas, and the use of a green technology innovation, will take the region as the core to the surrounding radiation, drive the surrounding areas of the use of the green innovation technology. Zhang and Kui (2021) found that green finance has a significant promoting effect and spatial spillover effect on total factor productivity: Yin et al. (2021) pointed out in the study that there is a “U” -shaped spatial spillover effect between the development level of green finance on green total factor productivity. It can be seen that the promotion of green total factor productivity in this region will be indirectly affected by adjacent areas.
Hypothesis 3. the influence of green finance on agricultural green total factor productivity has a spatial spillover effect.
Taking into account existing research results (Zhu and Zhang, 2023; Han and Yang, 2023; Hong et al., 2023; Manyao et al., 2023; Shuai et al., 2022; Yang and Zuo, 2022; Luo and Wu, 2024; Longteng and Fan, 2024-02; Wang and Lei, 2023; Wu et al., 2023), in this study we comprehensively considered the United Nations Sustainable Development Goals and the characteristics of China’s agricultural industry. Wes elected input indexes from five aspects: Agricultural resources, labor, energy, innovation, and government support. The expected output indexes were selected from the perspective of useable outputs, and the non-expected output indexes were selected from the perspective of the main pollutants arising from agricultural production. We then constructed an index system to assess China’s agricultural TFP (Table 1).
Based on existing research results (Zhang and Jiang, 2006; Gang, 1995; Zhang, 2006; Zhu, 2009; Xia, 1999; Luyuan, 2024; Lu et al., 2024; Tong, 2024; Gu and Hao, 2024) and the characteristics of China’s agricultural development, we established an index framework to quantify green finance from multiple perspectives, including green credit, green education, green insurance, green investment, and carbon finance; we then constructed an index system for green finance, as shown in Table 2. The development of green finance can guide the flow of funds to environmental protection industries and effectively inhibit the transfer of industries that are highly polluting and have high energy consumption and excess capacity to rural areas, thus maintaining the fair development of urban and rural ecology. The green and low-carbon development of agriculture is a complex systematic project, which requires not only scientific, technological, and institutional innovation but also the promotion of talented individuals. Education is an important way to cultivate talent, with its importance lying in its ability to enhance individuals’ learning and ability to innovate while also shaping their sense of value and behavior patterns, thus promoting the green and low-carbon development of agriculture. Moreover, through the innovation of green insurance products and green claims management, insurance products and services can contribute to the sustainable development goals that relate to agriculture. The core of the policy of establishing green finance reform and innovation pilot zones is to improve innovations in green agriculture and the level of agricultural mechanization, thus improving agricultural production efficiency. Against the background of the dual-carbon goals, green development has become the main theme of rural economic development. Green finance can promote energy conservation and emission reductions by leveraging green financial tools. Combining green finance with agricultural and rural development can create a strong empowering effect for rural revitalization.
Considering that 2010 was the start of the initial development stage of China’s green finance initiative, we took the time period from 2010 to 2020 as the research period. We selected the indexes of 30 provinces in China (including autonomous regions and municipalities directly under the control of the central government) as the research samples to analyze the spatial correlation of agricultural GTFP in China, as well as the influences of green finance on the agricultural GTFP. (Hong Kong, Macao, Taiwan, and Tibet were not included in the targeted regions of this study due to a lack of data.) The research data were collected from the China Statistical Yearbook, China Rural Statistical Yearbook, China Environmental Statistical Yearbook, provincial statistical yearbooks and statistical bulletins, national economy and social development statistical bulletins, environmental status bulletins, and other literature covering the research period. For any data missing from the sample, linear interpolation was used to complete the data.
Data envelopment analysis (DEA) is a widely used method because it is suitable for studying multi-input and multi-output problems and does not need to set the production function form. During the early stages of its development, this method did not consider unexpected outputs, leading to calculation results that may have been biased. The improved SBM model is a non-radial model and cannot deal with radial problems. The EBM model can overcome this shortcoming. Considering that the global GML index has the characteristics of transitive, cyclic accumulation, comparable and reflecting long-term changes. Therefore, GML index is selected to measure agricultural green total factor productivity, and GML index is used to compare the changes of agricultural green total factor productivity. The EBM-GML model was selected to measure agricultural green total factor productivity and growth rate (Zhou and Nie, 2022). The specific model is expressed as shown in Equations 1, 2:
where X, YG, and YB represent m inputs, n expected outputs, and one non-expected output, respectively; H is the number of decision units;
By combining the above with the technical efficiency parameter, ρ, the GML index can be constructed as shown in Equation 3:
where
The spatial panel model covers the spatial effect and the time effect, which makes the given spatial regression model more realistic. Therefore, we adopted the spatial Durbin model (SDM) to analyze the influencing factors of the level of agricultural green development in China. The specific formula is as shown in Equation 4:
where IGDI it represents the agricultural GTFP of province i at period t; α is a constant term; ρ represents the spatial regression coefficient; β is the regression coefficient of the explanatory variable; θ is the regression coefficient of the spatial lag term of the explanatory variable; W is the spatial weight matrix (if there is a common boundary between two regions, W = 1; otherwise, W = 0. Due to the special geographical position of Hainan province, the common boundary between Hainan and Guangdong Provinces is adjusted to ensure the scientificity and preciseness of the research.); ui represents the space fixed-effect; λt represents the time fixed-effect (if ui and λt are correlated with the explanatory variables, it is a fixed-effects model; if not, it is a random-effects model.); and μit represents the error term of spatial autocorrelation.
According to our calculation results (Table 3), the average value of China’s agricultural GTFP from 2010 to 2020 was 0.86, which is much lower than the production frontier value, indicating that the agricultural GTFP level of Chinese agriculture is not high and that there is still much room for improvement. The average values of agricultural GTFP for Beijing, Tianjin, Shanghai, Heilongjiang, Jiangsu, Zhejiang, Fujian, Guangdong, Hainan, Yunnan, and Ningxia were all greater than 0.9, ranking among the top 11 of China’s provincial administrative regions. Moreover, their efficiency values were all greater than the national average value, also indicating that their agricultural GTFP level was higher than other provinces. Among these 11 provincial administrative regions, Beijing, Tianjin, Shanghai, Jiangsu, Zhejiang, Guangdong, and Hainan are economically developed regions in eastern China. These regions attach great importance to green finance policies and have made rapid technological progress and full use of scientific and technological innovation. Fujian, Heilongjiang, and Ningxia rigorously promote the development of green finance, which not only conforms to the mainstream policies of green development but also accelerates economic transformation and development, impelling their agricultural industry to move toward modernization. For Gansu and Shanxi Provinces, their comprehensive efficiency values were both lower than 0.6, ranking the lowest in China. Moreover, their average efficiency values were much lower than the national average. These results indicate that the comprehensive efficiency of the agricultural green economy in these two provinces is poor. Gansu Province is in the economically underdeveloped region of northwestern China, and its poor level of economic development has greatly affected its agricultural GTFP development. Shanxi Province is in the central economic region, and its unique resource-based industrial structure and economic structure bring difficulties and problems for the development of agricultural GTFP. To sum up, there are clear inter-provincial differences in China’s agricultural GTFP. The provinces with higher agricultural GTFP are mostly distributed in the eastern and northern regions, while the provinces with lower agricultural GTFP are mostly distributed in the central and western regions.
To more directly reflect the spatial distribution characteristics of China’s agricultural GTFP at the provincial level, we selected data relating to China’s agricultural GTFP in 2010, 2015, and 2020, taking 5 years as the time node. We then created visual representations using ArcGIS10.4 software (Figure 1). The 30 provinces in China were categorized into five types: low productivity areas, low-to-medium productivity areas, medium productivity areas, medium-to-high productivity areas, and high productivity areas.
Table 4 provides the agricultural GML values and related decomposition indexes of 30 provinces in China between2010 and 2020. As can be seen, the average annual growth value of agricultural GTFP in China from 2010 to 2020 was 1.03, with an average annual increase of 3.79%. The average annual growth value was greater than 1, indicating that the technical efficiency of agriculture showed an improving trend. The maximum value (1.1913) occurred in 2010–2011, and the minimum value (0.8969) occurred in 2011–2012. During the study period, there were 4 years in which the agricultural GML value did not exceed 1: 2011-2012, 2012-2013, 2016-2017, and 2017-2018. These results indicate a relatively high level of agricultural GTFP in China. From the perspective of the change trend of agricultural GML, the agricultural GTFPbetween2010 and 2014 and between2014 and 2020 showed a similar wave-like trend of first decreasing and then increasing, with a maximum value of 1.1508 in 2014–2015.
Table 4. Growth rate of China’s agricultural GTFP and its decomposition efficiency between 2010 and 2020.
The agricultural GML index of China was decomposed into a technical efficiency change (EC) index and a technical progress change (TC) index. The EC index represents the ratio between the actual output value and the optimal value in a given period. It measures the level of technical efficiency and reflects the degree of effective allocation of agricultural production resources by agricultural technologies. The TC index represents the ratio between the actual output value and the optimal value by using the same input factors in different periods. This measures the level of technological progress and reflects the degree of application of new agricultural technologies in agricultural production activities. Therefore, from the perspective of China’s EC and TC indexes, the average value of China’s EC index was 1.0059, which is greater than 1, and the average value of the TC index was 1.0313, exceeding the EC index.
Figure 2 shows more clearly the change trends in China’s GML, EC, and TC indexes. On the whole, the TC and GML indexes showed approximately consistent change and fluctuation rules, indicating that the TC index is the main influencing factor of the GML index. From the perspective of different time periods, from 2010 to 2014, the GML, EC, and TC indexes fluctuated considerably around 1.0000, showing overall the characteristics of steep declines followed by steep rises. The growth rate of agricultural GTFP and its decomposition efficiency fluctuated in a similar manner, indicating that the EC and TC indexes acted jointly on the development of agricultural GTFP between2010 and 2014. From 2014 to 2017, the change trends of the GML and TC indexes always maintained a downward trend, where as the EC index showed an upward trend. As this was contrary to that of the GML index, it indicated that a decrease in the growth rate of technological progress can improve the growth rate of technical efficiency. From 2017 to 2020, the change rates of the TC and EC indexes were both high, indicating sufficient technological input during this time period, which could effectively improve agricultural GTFP. To summarize, from 2010 to 2020, technical efficiency showed an overall trend of fluctuating decline. The growth impetus for agricultural GTFP mainly came from technological progress, whereas the contribution of technical efficiency improvement was relatively small. This means that although the level of agricultural technology progress in China had been further improved between 2010 and 2020, the level of technical efficiency was not satisfactory. Hence, technological progress should be combined with the enhancement of technological application ability and improvements in the level of technology management. Then, the effective improvement of agricultural GTFP could be achieved.
Figure 2. Growth rate of China’s agricultural GTFP from 2010 to 2020 and its decomposition broken line graphs.
According to the distribution of natural resources and the level of economic and social development of each provincial administrative region in China, China is roughly divided into three economic zones in the east, central and west with reference to the research practice of the academic circle, thus forming three major research areas. The eastern region includes Beijing, Tianjin, Hebei, Liaoning, Shanghai, Jiangsu, Zhejiang, Fujian, Shandong, Guangdong and Hainan; the central region means Shanxi, Jilin, Heilongjiang, Anhui, Jiangxi, Henan, Hubei and Hunan; and the western region is Inner Mongolia, Guangxi, Chongqing, Sichuan, Chuzhou, Yunnan, Shaanxi, Gansu, Qinghai, Ningxia and Xinjiang, as shown in Table 5.
According to Table 6, the average GML index in the eastern region between2010 and 2020 was 1.0063, while this value between 2011 and 2013 and between2016 and 2020 was less than 1, indicating that the agricultural GTFP in the eastern region showed a trend of fluctuating decline. The average value of the EC index across the 11-year period was 0.9972. The EC index was greater than 1 in 2010–2011, 2012-2014, and 2014-2016, whereas the EC index in the other years showed a declining trend. The EC index in the eastern region can thus be considered to have been in a retrogressive state from 2010 to 2020. The TC index, however, was always greater than 1 except for in the years 2011–2013 and 2016-2018, confirming that this value improved in most years from 2010 to 2020. In the central region, the GML index was always greater than 1 except for in the years 2011–2013 and 2015-2017. The maximum value of the GML index occurred in 2010–2011, when it was 1.2285 (22.85%) higher than during the previous year. The minimum value of the GML index occurred in 2011–2012, which was 0.8319 (16.81%) lower than that in the previous year. The EC index was less than 1 in the years 2011–2012, 2015-2016, 2017-2018, and 2019-2020, and greater than 1 in all other years. Hence, in most years, the EC index in the central region had a promoting effect on agricultural GTFP. The TC index was greater than 1 in all years except for in the years 2011–2013 and 2015-2017, with the highest value of 1.2061 occurring in the year 2010–2011, which played a crucial role in promoting the development of agricultural GTFP. In the western region, the GML index was less than 1 in the years 2011–2012, 2013-2014, and 2015-2017, indicating the retrogression of agricultural GTFP in these years compared to that in the corresponding previous years. In the remaining years, the GML index was greater than 1, indicating an improvement in agricultural GTFP in these years compared to that in the corresponding previous years. The growth rate of the GML index reached its highest value of 29.78% in 2010–2011, while the greatest decrease in this index, of 9.26%, occurred in 2011–2012, which were similar values to those seen in the central region. The EC index in the western region was less than 1 in the years 2011–2012, 2014-2015, 2017-2018, and 2019-2020, indicating that the EC index had an inhibitory effect on the GML index in these years. In the remaining years, the EC index showed an increasing trend. The TC index was less than 1 in the years 2011–2012, 2013-2014, and 2016-2019, with the most rapid decrease compared with that in the previous year (of 11.55%) occurring in the year 2013–2014. In all other years, the TC index showed an increasing trend.
Table 6. Growth rate of agricultural GTFP in the eastern, central, and western regions of China and the corresponding average values of decomposition efficiency.
As can be seen from Figure 3, the GML index in the eastern region was in a continuously fluctuating state between 2010 and 2020. The period from 2010 to 2014 was in a sharply fluctuating stage, while the period from 2014 to 2020 was in a steady stage. The minimum value of the GML index (0.9335) occurred in 2011–2012, while the maximum value (1.0866) occurred in 2014–2015. From the perspective of growth impetus, the TC and the GML indexes showed similar fluctuation trends, indicating that agricultural GTFP in the eastern region was driven by progress in agricultural technologies. The change trend of the EC index could also be divided, into a period with large fluctuations from 2010 to 2014 and a period with smaller fluctuations from 2014 to 2020, but the EC index showed an opposite fluctuating trend to that of the GML index. The GML index in the central region fluctuated greatly between 2010 and 2020, with a maximum value of 1.2245 occurring in the year 2014–2015 and two low values, of 0.8319 in 2011–2012 and 0.9525 in 2015–2016. During the sampling period, the TC and EC indexes both changed sharply, but showed opposite fluctuating trends, and the EC index fluctuated less than the TC index. In the western region, the change trend of the GML index between 2010 and 2020 was similar to that seen in the central region, with a maximum value of 1.1859 occurring in the year 2014–2015 and three low values, of 0.9074 in 2011–2012, 0.9119 in 2013–2014, and 0.9493 in 2016–2017. However, the GML index in the western region fluctuated less than that in the central region. As the GML index is the product of the TC index and the EC index, in the three regions, the increase in the TC index was accompanied by a decrease in the EC index in almost all years from 2010 to 2020. This may be due to an unreasonable allocation of the input of agricultural technology and agricultural efficiency in the process of agricultural development. Moreover, this may also reflect that agricultural technological progress plays an important role in promoting the growth of agricultural GTFP.
Figure 3. Growth rate of agricultural GTFP and the decomposition point plots in the three regions of China from 2010 to 2020.
From 2010 to 2020, the average annual growth rate of agricultural GTFP in western China was the highest, followed by central China and eastern China. The annual average growth rate of agricultural GTFP in the western and central regions was higher than the national average, while that in the eastern region was lower than the national average. The eastern region has a high level of economic development and rapid renewal of agricultural technologies, so the room for development in the eastern region was smaller than that in the central and western regions, resulting in insufficient technology applications and inability to effectively transform technological progress into agricultural GTFP. Hence, the growth rate of agricultural GTFP was lower in the eastern region. The development concept in the central region was advanced, and the technical efficiency was higher. Although the technological progress lags behind that in the eastern region, the existing technologies can be transformed into productivity increase, resulting in a faster growth rate of agricultural GTFP. The level of technological progress in the western region was the highest among the three regions. Although level of economic development in the western region was low, the room for development was large and technological progress could be effectively transformed into technical efficiency, thus considerably improving agricultural GTFP.
The influence of green finance on agricultural green total factor productivity shows heterogeneity in the eastern, central and western parts of China. Analysis of the possible reasons: First, the economic development in eastern, central and western China is significantly unbalanced. Due to the early economic start in the eastern region, its economic level is ahead of the central and western regions. Economic advantages enable the eastern coastal developed economic zone to better promote green finance, make full use of its rich financial resources advantages, and effectively promote the construction of green industry and agricultural technology innovation in local rural areas. In contrast, the rural economic foundation of the central and western regions is relatively weak and lacks of necessary technical support. Secondly, the economic foundation of the western region is weak, and the development of green finance can quickly improve the efficiency of financial services for benefiting farmers, and promote agricultural modernization, technological improvement and innovation. In addition, green finance to promote agriculture the main benefit object is farmers, small micro enterprises and other relatively poor vulnerable groups, and the western provinces far more than the eastern and central provinces, means that green finance in the western region has a wider application space and more benefit groups, combined with the western region has fertile soil, diverse climate conditions of natural resources, as well as the national policy support for the western region, provides the foundation of a good for agricultural green development.
Chang and Chen found that while promoting high-quality economic development in the region, financial development has a spatial spillover effect on the neighboring areas. In the process of pursuing the maximization of capital interests, financial development promotes the cross-regional flow of capital, talents and commodities, which must have spatial effect. Whether green finance also has spatial effect is a question worth discussing (Chang and Chen, 2020).
There are two types of effect models that can be used for panel data analysis. According to the statistical values obtained from the Hausman test and Lagrange multiplier (LM) test, adual fixed-effects model was chosen for this study. According to the estimated results, Wald test and LR test refused to simplify the spatial Dubin model, through the 1% significance test, R2 value is 0.957, and space overflow term is 0.5821, through 1% significance test, shows that the model fitting effect is good, and agricultural green total factor productivity has significant space spillover effect, green financial level similar agglomeration phenomenon. That is, areas with high green finance level and areas with high green finance level, areas with low green finance level and areas with low green finance level gather. Therefore, we chose the dual fixed-effects spatial Durbin model for our calculation, with agricultural GTFP as the explained variable and various indexes of green finance as the explanatory variables (Table 7).
As can be seen from Table 7, the direct influence coefficient and spatial lag coefficient of green bonds (X2) were both negative at the significance level of 1%. Agricultural enterprises can issue green bonds to raise money, and investors decide whether to buy the bonds based on their benefits and risks. The issuance of green bonds can effectively expand financing channels for agriculture and attract more funds to flow into the sector. Moreover, the issuance of green bonds can also improve the transparency and responsibility of agricultural enterprises, promote their sustainable development, and improve the level of agricultural green development. However, due to the stricter regulation on the use of funds raised by green bonds and the requirement for more frequent information disclosure, green financial bonds can negatively affect agricultural GTFP in neighboring regions. The spatial lag coefficient of green insurance (X3) was negative at the significance level of 1%. The direct influence coefficient of green insurance was negative but not significant. The development of green agriculture is faced with risks such as climate change and natural disasters, and green insurance can provide corresponding risk protection for agricultural enterprises and reduce their business risks. Moreover, green insurance can also guide funds to green agriculture and promote its sustainable development through insurance companies that provide insurance services for agricultural development. The direct influence coefficient of green investment (X4) was positive at the significance level of 5%, whereas the spatial lag coefficient was negative but not significant. Green finance, as a financial mode oriented to environmental protection and sustainable development, can provide financial support and an innovative financing mode for the green development of agriculture. Through the guidance and support of green finance, the transformation and upgrading of agricultural ecological development can be promoted, and the efficient use of resources and the protection of the ecological environment can be achieved, providing strong support for the sustainable development of agriculture. The development of green agriculture needs continuous scientific and technological support and technological innovation to improve agricultural production efficiency and resource utilization efficiency. The establishment of special funds can provide financial support for scientific and technological research and development and technological demonstration in agricultural field, promoting the transformation and application of relevant scientific and technological achievements, and thus improving the level of green agricultural development. However, due to regional restrictions on agricultural development, local environmental regulations may bring higher production costs and risks to rural green development and reduce the demand for green finance, thus weakening the promoting effect of green investment on rural green development. As a result, the spatial lag term of green investment was not significant.
In the effect decomposition of the spatial Dubin model, due to the spatial spillover effect, the coefficient of each factor cannot explain the influence on the green total factor productivity of agriculture alone. The partial differential decomposition is conducted, and the influence of each explanatory variable on the green total factor productivity is divided into direct effect and indirect effect (Xie, 2024; Zhao et al., 2024; Ma et al., 2024; Wu et al., 2024; Hu et al., 2024; Zhao et al., 2023; Wang et al., 2023). Both reflect the influence of the changes of the local and adjacent regions on the green total factor productivity (Table 8).
In terms of direct effect, the elasticity coefficient of green credit (X1) is negative but not significant. Green financial credit institutions green development concept and consciousness is not strong, specializing in the development of green financial institutions outlets, green credit business and professionals, and due to the rural agricultural development integration project new pledge to set up, green rights and interests value identified, public welfare green project difficult to ground is widespread, lead to green credit support rural industrial integration is not enough. The elasticity coefficient of green bonds (X2) and green insurance (X3) is negative, which has passed the significance test of 1% and 5%, respectively. Green finance compared with the traditional financial support, more focused on green, low carbon contribute to environmental protection and maintenance of ecological balance, is conducive to enhance the availability of funds needed for green agricultural development, targeted at the same time strong financial support can improve the financing efficiency of demand subject, is conducive to quickly promote the green transformation of traditional agriculture, realize agricultural green development. Due to China region across dimension range, there are huge differences in regional agricultural production structure, and the current support for agricultural production funds mostly provided by banking institutions, insurance, securities and funds and other financial products for the participation of green agriculture is low, financial institutions demonstration guide role is not strong, cannot become a strong support of the green financial system. Compared to manufacturing, service industry, green insurance for green agriculture development of product types is less, and can perfect implementation and efficient application of green insurance category, green insurance in support green agriculture development has not yet formed a complete system, which greatly interfere with the green financial support for green agriculture, restricting the development of green agriculture conditions increase. This shows that every 1% increase in green bonds and green insurance in the region will increase the green total factor productivity in regional agriculture by-0.2098% and-0.6255% respectively. The elasticity coefficient of green investment (X4) is positive and has passed the 10% significance test. The largest direct effect of green investment is 1.565, indicating that green investment is the most important factor affecting the level of green development of Chinese agriculture in the indicators of green finance, and will play a significant role in China’s agricultural total factor productivity. That is, every 1% increase in green investment in the region will increase the green total factor productivity of agriculture by 1.565%. The elasticity coefficient of carbon finance (X5) is positive but not significant. Carbon finance is mainly measured by carbon intensity indicators. In addition, carbon finance started late in China, so it has s impact on the green agricultural development is not significant.
From the perspective of indirect effects, the elasticity coefficients of green bonds (X2) and green insurance (X3) were both negative at the significance level of 1%; the elasticity coefficients of green credit (X1) and green investment (X4) were both negative but not significant; and the elasticity coefficients of carbon finance (X5) were positive but not significant. Among these, the issue of green bonds can strengthen the guidance and support for green finance and achieve a win-win situation between agricultural ecological development and economic benefits, thus providing strong support for sustainable agricultural development. However, the application of green bonds in agricultural development still faces some challenges, such as information asymmetry, risk assessment and other problems, local governments, financial institutions and agricultural enterprises need to work together to strengthen cooperation, promote the development and application of green finance, and achieve green agricultural development. Green insurance can provide economic compensation for farmers in the region and help them resume production, but the complexity and uncertainty of the agricultural ecosystem make it difficult to design and price green insurance. Insurers need to accurately assess the risks and potential losses of crops to determine reasonable insurance rates. The above reasons lead to the negative indirect effect of green bonds and green insurance on agricultural green total factor productivity in neighboring areas. In general, green bonds (X2) and green insurance (X3) both have negative direct and indirect effects on green total factor productivity. It shows that the green development of agriculture in this region is mainly affected by local green finance, perhaps that the transportation, information and other connectivity in neighboring regions is more advantageous than that in the region. The innovation of green financial system products in a region is more likely to be imitated and overflowed by neighboring regions, thus promoting the green development of agriculture in neighboring regions.
This paper can supplement and improve the research system of agricultural geography and regional financial research theoretically and empirically, enrich the theoretical content of human economic geography and sustainable development, and has certain theoretical significance. It can also provide a scientific basis for China to further improve the level of green agricultural development and narrow regional differences, and provide theoretical support and decision-making basis for the further promotion of green finance and the green and sustainable development of China’s agriculture, which has important practical significance. Green finance promotes the increase of agricultural GTFP and is of great importance for the realization of green and sustainable development in China. Here, we have systematically analyzed the direct and indirect effects of green finance on agricultural GTFP and drawn the following conclusions:
(1) Although China has made some achievements in green finance and agricultural GTFP development, there are significant differences between specific provinces, especially in northern China, where green total factor productivity is significantly higher than that in central and western China. From the perspective of spatial spillover effect, both EBM and SDM models can conclude that the development level of green finance can significantly improve the development level of agricultural GTFP in the region and neighboring regions, which is similar to the research of Xie et al. (2023); Shi-qing and Ling-cheng (2024). Green finance has obvious spatial spillover effect. Moreover, the promotion effect of green finance on agricultural GTFP of neighboring cities is greater than that of local cities. At the same time, due to the imbalance of economic development between regions, environmental governance policies are not uniform and other reasons, this difference will continue to exist in the future for some time. Therefore, we need to pay attention to and study this inter-provincial difference, and actively find solutions to further improve the integration of green finance and agricultural GTFP in China, and promote the sustainable development of China’s agricultural economy.
(2) From the analysis of regional heterogeneity, the agricultural green total factor productivity in China shows obvious imbalance among different regions, and its influence of green finance also varies from region to region. The influence of green finance development level on agricultural green total factor productivity is significantly greater in the eastern region than in the central and western regions. The effect was positive in the western region and negative in the central region, but neither significant. Due to the high level of economic development in the eastern provinces, more financial resources are available to support the green development of local agriculture and agricultural technology innovation, which plays a better role than the other two regions. However, the rural economy in the central and western regions is relatively weak and lacks technical support, so its role will be weakened.
(3) The green financial system can upgrade the industrial structure and promote innovation, drive the continuous progress of technology, so as to guide and encourage enterprises to optimize the energy consumption structure, reduce energy consumption and pollution, and improve the overall level of agricultural GTFP. Compared with the eastern region, the western region has the largest role in promoting green finance, which is inseparable from the economic development and ecological barrier needs of the western region. At the same time, the promotion role of green finance in the central region cannot be ignored. With the implementation of a series of ecological and environmental protection policies in the central region, the level of green finance in the central region is also constantly improving. Compared with the western and central regions, the eastern region has a higher level of economic development, but its role in promoting green finance is relatively weak. This is mainly due to the rapid process of industrialization and urbanization in the eastern region, which brings greater pressure on the environment, thus restricting the development of green finance to a certain extent. The influence of green finance on the eastern, central and western regions of China is significantly different. The influence of green finance on the total factor productivity of agricultural green is greater in the eastern region, followed by the central region and less in the western region. This is similar to the research results of Xiao and Miao (2023). Both direct effects and spatial spillover effects are significant in the eastern region, while only direct effects are significant in the central and western regions, and spatial spillover effects are not significant. Miao (2023) also believes that this result may be caused by the imbalance of economic development between regions (Miao, 2023), which verifies our research conclusion.
(4) From the perspective of dynamic analysis, China’s agricultural GTFP as a whole presents a wavy change trend, first declining, then rising, then declining and finally stabilizing. From decomposition index, in the process of our country’s agricultural green development, the promoting effect of technical progress on our agricultural green development is obvious, but the level of technical efficiency has no obvious effect on promoting the development of agricultural green development. This is similar to the findings of Hu (2022) and Huang (2020). They confirm that technological progress is the source of green total factor productivity growth in China’s agriculture.
(5) Green investment plays a significant role in promoting China’s green total factor productivity and is the most effective tool to promote the green development of regional agriculture. By guiding funds to sustainable agriculture projects, green investment directly contributes to the improvement of agricultural GTFP by promoting technological innovation, ecological agriculture development and resource utilization efficiency. It provides continuous financial support for long-term green agricultural projects and promotes the green upgrading of the whole industrial chain. However, other green financial instruments such as green credit, green bonds, green insurance and carbon finance may face some negative effects when applied and need to be further improved.
Based on the above conclusions, we would like to put forward the following policy recommendations:
(1) To build a better green finance system and strengthen the standardized management of the green financial market, the government should strengthen the supervision off financial institutions at the following three levels. First, the government should establish a comprehensive green financial supervision mechanism, clarify the responsibilities and obligations of financial institutions, and effectively supervise green financial products and services to ensure their legitimacy and safety. Second, the government should introduce the power of social organizations to widely supervise the green financial market, achieving the diversification and universality of regulatory forces. Finally, the government should enhance the public’s attention to green finance and strengthen social supervision, creating a good environment for the whole of society to participate in green finance. In addition, in terms of technological innovation, the government should guide financial institutions to improve their technical ability, strengthen information disclosure and data sharing, and establish a sound risk assessment and monitoring system, thus ensuring the steady development of the green financial market.
(2) To develop the green finance market according to local conditions, China should find an innovative path that can make the most of local advantages and integrate environmental protection regulatory requirements based on local resource endowments and development status. First, based on local resource advantages, local governments should take green energy as the entry point and implement renewable energy projects, such as wind energy, solar energy, geothermal energy, and other energy projects, thus creating rich investment opportunities and growth space for the development of green finance. In addition, the government should increase the financial support for sustainable agricultural industries and encourage the implementation of local ecological conservation initiatives. This can not only improve the production efficiency of the green economy but also improve the local ecological environment, promoting the deep integration of green finance and the real economy, thus achieving a win-win effect. Moreover, this also lays a solid foundation for achieving sustainable development and promoting the growth of the green economy.
(3) To further enhance the sustainable development capacity of green agriculture, the government should seek a deep understanding of the importance of education and scientific and technological innovation for the development of green agriculture. First, the government should increase investment in educational facilities and gradually improve the cultural literacy of workers, providing a larger reserve of talent for the development of green agriculture. Second, the government should increase investment in scientific and technological innovation, continue to develop and launch new green agricultural technologies and products, and seek to continuously improve the competitiveness and sustainability of green agriculture. Finally, the government should pay attention to policy support for the development of green agriculture and provide a more relaxed policy environment and more supportive measures for the development of green agriculture, thus guaranteeing the sustainable development of green agriculture in China.
(4) Considering the vast geographical area of China, different regions should adopt different ways to optimize green finance.
The eastern region, central and western regions can combine their own characteristics and characteristics, and use innovative financial services to fund green industry projects and promote the development of green finance.
The eastern region can strengthen the cooperation with innovation banks, such as Zhejiang Chouzhou Commercial Bank, to use its credit line and its ability to issue green financial bonds to expand the loan scale and trigger greater environmental benefits. Industrial Bank can also introduce the “Belt and Road” green finance project to promote the high level of “smart Made in China” to go abroad. The central region can cite Industrial Bank’s experience in Xinjiang to innovate financial services and help the development of the clean energy industry. At the same time, we will strengthen cooperation with financial institutions, focus on the construction of the ecological civilization pilot zone, support Hunan in speeding up the construction of a world-class port group, and smooth the “Belt and Road” maritime trade corridor. The western region can learn from the experience of Industrial Bank in Fujian, focus on the construction of a pilot zone for ecological civilization, support Xinjiang in speeding up the construction of a world-class port group, and smooth the “Belt and Road” maritime trade corridor (Xu et al., 2023; Chen and Pan, 2019; Luo et al., 2023; Liu et al., 2024). At the same time, we will strengthen cooperation with financial institutions, innovate financial services, and help develop the clean energy industry.
In general, the eastern and western regions can promote the development of green finance by strengthening cooperation with innovative banks, innovating financial services, focusing on the construction of ecological civilization pilot zones, and strengthening cooperation with financial institutions.
(5) Accelerate the pilot zone for green finance reform and innovation, give play to the regional driving role, and improve the imbalance in regional development. The system of the green finance reform and innovation pilot zone is a local practice of the national green finance policy, which is more targeted on the basis of commonality and closely integrated with local development. The promotion of “first and first trial” in the pilot zone is conducive to improving the differences between regions, and discovering the difficulties and problems encountered in the specific implementation process from the practical level, providing a basis for determining the goal, planning the key direction and coordinating the pace of China’s overall green finance development. It is necessary to continue to accelerate the application of green finance reform and innovation pilot zones in other provinces and cities, give full play to the aggregation and spillover benefits of financial factors, drive the development of green finance in surrounding areas, narrow regional development differences, and comprehensively improve the development level of green finance in China.
(6) Strengthen the construction of green finance development system, and give play to the guiding and driving role of policy and strategy support. According to the driving mechanism of green finance development in each region, targeted policies focusing on the standard system and coordination mechanism construction are formulated respectively, and special guidance documents are combined with local power sources to create green finance ecology. For example, green credit is prone to fund misallocation or short-term problems, and loose credit standards may lead to funds flowing to non-green projects. To this end, strict project evaluation criteria need to be established to ensure that credit flows to agricultural projects with green benefits, and long-term, low-interest credit is provided to prevent funding from becoming short-term. Green bonds may suffer from lack of transparency and lower than expected environmental benefits, so detailed issuance standards should be developed, information disclosure and third-party audits should be strengthened, bond funds should be dedicated to green agriculture projects, and environmental impact assessment mechanisms should be introduced to regularly assess their contribution to agricultural GTFP. Green insurance in the agricultural sector faces the challenge of inadequate environmental and market risk assessment, and may not be able to effectively help farmers cope with green risks such as climate change. Therefore, it is recommended to introduce agricultural insurance products against climate risks, combine green credit or green investment, provide integrated financial services, and include provisions in insurance policies to encourage green practices, such as premium concessions. Carbon financial instruments in the agricultural sector face the problems of opaque carbon credit and high market volatility. Therefore, it is necessary to promote the standardization of carbon credit verification mechanism, ensure that carbon emission reduction behaviors in the agricultural sector are accurately quantified, establish a stable carbon trading market, reduce the impact of fluctuations on agriculture, promote agricultural carbon sequestration projects, and encourage the increase of carbon sequestration through soil carbon sequestration, afforestation and other means. Help farmers earn carbon credit income.
There are still some shortcomings in this study: (1) the selection of key indicators needs to be strengthened, and green investment and green energy projects are important influencing factors, but due to the lack of data, it cannot support scientific research, and the selection of key indicators needs to be further strengthened in the future. (2) The research objects of this paper are mainly narrow sense agriculture represented by planting industry, while the forestry, animal husbandry and fishery in the broad sense are not included in the research scope of agricultural green development considering the complex contents, and need to be further improved and explored in the future. (3) Due to the lack of data in some years, this paper uses the linear interpolation method according to the actual situation. The lack of indicators and replacement will affect the overall measurement results to some extent, therefore, this part is still the focus of the research team to continue to deepen the research work in the future. (4) Since the data scope of this paper is panel data of 30 provinces in China, the conclusion of the study on the impact of green finance on agricultural green total factor productivity should be limited to China. Secondly, the construction of green finance index in this paper mainly covers four dimensions: green credit, green bond, green investment, green insurance and carbon finance. But the true meaning of green finance may not be limited to that.
The datasets presented in this study can be found in online repositories. The names of the repository/repositories and accession number(s) can be found in the article/supplementary material.
The manuscript presents research on animals that do not require ethical approval for their study.
LCu: Data curation, Investigation, Methodology, Validation, Writing–original draft. LCe: Conceptualization, Formal Analysis, Writing–review and editing. YG: Formal Analysis, Investigation, Visualization, Writing–original draft. HD: Methodology, Software, Validation, Writing–original draft. QW: Data curation, Formal Analysis, Methodology, Software, Writing–review and editing. YL: Data curation, Investigation, Software, Visualization, Writing–original draft.
The author(s) declare that no financial support was received for the research, authorship, and/or publication of this article.
The authors declare that the research was conducted in the absence of any commercial or financial relationships that could be construed as a potential conflict of interest.
All claims expressed in this article are solely those of the authors and do not necessarily represent those of their affiliated organizations, or those of the publisher, the editors and the reviewers. Any product that may be evaluated in this article, or claim that may be made by its manufacturer, is not guaranteed or endorsed by the publisher.
The Supplementary Material for this article can be found online at: https://www.frontiersin.org/articles/10.3389/fenvs.2024.1463833/full#supplementary-material
Anubhab, P., Kavi Kumar, K. S., and Lavanya, R. A. (2021). Distributional effects of climate change on agricultural total factor productivity in India. Asia-pacific Econ. Rev. 26 (2), 381–401. doi:10.1080/13547860.2021.1917094
Bo, L. I., and Bao, H. U. (2023). Empirical analysis agricultural green total factor productivity based SBM-GML index model in the on Yellow River basin. J. Zhejiang Agric. Sci. 64 (04), 1004–1008. doi:10.16178/j.issn.0528-9017.20220629
Chang, X., and Chen, L. (2020). Analysis of the spatial spillover effects of financial development and capital efficiency on high-quality economic development. Financial Econ. Res. 35 (04), 35–45.
Chen, C., and Pan, J. (2019). The effect of the health poverty alleviation project on financial risk protection for rural residents: evidence from Chishui City, China. Int. J. Equity Health 18 (1), 79. doi:10.1186/s12939-019-0982-6
Chen, M., Ma, L., Che, X., and Dou, H. (2020). Identification of transformation stages and evolution of agricultural development types based on total factor productivity analysis: a case study of Gansu province, China. Agriculture 10, 363. doi:10.3390/agriculture10080363
Chen, Y. (2021). Study on the impact of green Finance on the green Development of rural enterprises. Agric. Econ. (12), 109–111. doi:10.3969/j.issn.1001-6139.2021.12.046
Conradie, B., Genis, A., Greyling, J., and Piesse, J. (2021). District-level agricultural total factor productivity for the Karoo, South Africa: 1952–2002. Agrekon 60 (4), 128–144. doi:10.1080/03031853.2021.1908155
Cui, Y., and Peng, L. (2023). Green finance development, green R&D investment and enterprise total factor productivity improvement door industrial technology and economy 2023. 42 (1), 28–36. doi:10.3969/j.issn.1004-910X.2023.02.003
Du, Z., and Lingxiao, H. (2023). Achievements and explanations of high-quality agricultural development in China since the 18th national congress of the CPC. Chin. Rural. Econ. (01), 2–17. doi:10.20077/j.cnki.11-1262/f.2023.01.001
Fang, S. (2023). Research on the impact of green finance on the consumption of the rural residents in China[D]. Liaoning University. doi:10.27209/d.cnki.glniu.2023.001598
Gang, X. (1995). On green finance: a new theory of environmental protection and financial orientation. China Environ. Manag. (04), 13–16.
Gao, G. (2019). Research on the support of green finance for the development of rural industrial integration. Tianjin University of Finance and Economics.
Ghebremichael, A., and Potter-Witter, K. (2009). Effects of tax incentives on long-run capital formation and total factor productivity growth in the Canadian sawmilling industry. For. Policy & Econ. 11 (2), 85–94. doi:10.1016/j.forpol.2008.09.004
Gu, T., and Hao, Z. (2024). Green finance and financial sustainable development. Trade Fair Econ. (03), 85–88. doi:10.19995/j.cnki.CN10-1617/F7.2024.03.085
Hamid, S., and Wang, K. (2022). Environmental total factor productivity of agriculture in South Asia: a generalized decomposition of Luenberger-Hicks-Moorsteen productivity indicator. J. Clean. Prod. 351, 131483. doi:10.1016/j.jclepro.2022.131483
Han, H., and Yang, D. (2023). Spatial spillover effects of agricultural industrial agglomeration on the growth of agricultural green total factor productivity. J. Arid Land Resour. Environ. 37 (06), 29–37. doi:10.13448/j.cnki.jalre.2023.134
Hong, D. (2017). The change of China's agricultural development strategy based on the perspective of world economic integration. Mark. Mod. (16), 180–181. doi:10.14013/j.cnki.scxdh.2017.16.106
Hong, M. A., Yibing, G., and Hui, W. (2023). Fiscal expenditure structure and agricultural green total factor productivity: Re-examining theStrategy of expanding domestic demand and supply-side structural reform. J. Shandong Univ. Sci. Technol. Sci. 25 (02), 75–86. doi:10.16452/j.cnki.sdkjsk.2023.02.009
Hu, F., Zhang, S., Gao, J., Tang, Z., Chen, X., Qiu, L., et al. (2024). Digitalization empowerment for green economic growth: the impact of green complexity. Environ. Eng. Manag. J. 23 (3), 519–536. doi:10.30638/eemj.2024.040
Hu, Y. (2022). Research on the growth of China's agricultural GreenTotal factor productivity and its influencing factors. Yangtze University. doi:10.26981/d.cnki.gjhsc.2022.000179
Huang, L. (2021). Agricultural capital deepening, partial technological progress and green agricultural economic growth. Southwest University. doi:10.27684/d.cnki.gxndx.2021.000090
Huang, X. (2020). Study on the spatio-temporal characteristics and influencing factors of agricultural green total factor productivity in China. Chongqing Technology and Business University. doi:10.27713/d.cnki.gcqgs.2020.000195
Ji, Y. (2023). A study of the impacts of green finance on Chinese agricultural carbon emissions. Shandong University. doi:10.27272/d.cnki.gshdu.2023.005479
Jiangiang, L. I., Changsong, W., and Yuan, Z. (2024). Enhancing food security through green finance: a study from the perspectives of rural human capital and agglomeration of agricultural industry. J. Univ. Jinan Social Sci. Ed. 34 (01), 52–68. doi:10.20004/j.cnki.ujn.2024.01.007
Jianiun, D. U., Youde, Z., Bomin, L. U., and Dong, R. (2023). Impact of digital village construction on agricultural green total factor productivity and its mechanisms. China Popul. Resour. Environ. 33 (02), 165–175. doi:10.12062/cpre.20221013
Lajos, B., Fert, I., and Bojnec, T. (2020). The effect of investment, LFA and agriculture environmental subsidies on the components of total factor productivity: the case of slovenian farms. J. Agric. Econ. doi:10.1111/1477-9552.12374
Li, Z. Q., Ye, W. J., Mei, D., and Zheng, C. W. (2022). Impacts of agricultural infrastructure on ecology total factor productivity of grain from the perspective of environmental regulation. Chin. J. Eco-Agriculture 30 (11), 1862–1876. doi:10.12357/cjea.20220214
Liu, B., Li, M., Ji, Z., Li, H., and Luo, J. (2024). Intelligent productivity transformation: corporate market demand forecasting with the aid of an AI virtual assistant. J. Organ. End User Comput. (JOEUC) 36 (1), 1–27. doi:10.4018/JOEUC.336284
Liu, H., and Chun, H. (2021a). Mechanism and inspection of green finance to promote high-quality development of urban economy — empirical evidence from 272 prefecture-level cities in China. Invest. Res. 40 (07), 37–52.
Liu, H., and Chun, H. (2021b). An empirical study on high-quality economic development based on intermediary effect model. New Finance (10), 21–27.
Liu, H. (2021). Study on the effect of inclusive finance on rural poverty reduction. Finance Econ. (05), 84–91. doi:10.19622/j.cnki.cn36-1005/f.2021.05.010
Longteng, X. A., and Fan, F. A. N. (2024). The impact of agricultural producer services on agricultural green total factor productivity. J. Zhejiang Agric. Sci., 1–14. doi:10.16178/j.issn.0528-9017.20230615
Lu, Z., Lihua, Q., Qi, F., Yin, C., and Jin, X. (2024). New opportunities for green transformation of “Carbon”-Green finance outlook of 2024. Finance Econ. (02), 18–30. doi:10.19622/j.cnki.cn36-1005/f.2024.02.002
Luo, H., and Wu, J. (2024). Study on the dynamic evolution of agricultural green total factor productivity in the Yangtze River Economic Belt: results based on SBM-GML index. Agric. Technol. 44 (02), 167–170. doi:10.19754/j.nyyjs.20240130038
Luo, J., Zhuo, W., and Xu, B. (2023). A deep neural network-based assistive decision method for financial risk prediction in carbon trading market. J. Circuits, Syst. Comput. 33 (8), 2450153. doi:10.1142/S0218126624501536
Luyuan, X. (2024). The influence mechanism of green finance and financing constraints on the transformation and upgrading of circulation industry. J. Commer. Econ. (03), 15–19. doi:10.3969/j.issn.1002-5863.2024.03.003
Ma, Q., Zhang, Y., Hu, F., and Zhou, H. (2024). Can the energy conservation and emission reduction demonstration city policy enhance urban domestic waste control? Evidence from 283 cities in China. Cities 154, 105323. doi:10.1016/j.cities.2024.105323
Manyao, J. A. N. G., Lin, X., Fan, M., and Zhang, R. (2023). Influence of income gap between urban and rural areas on agricultural green total factor productivity. J. Yunnan Agric. Univ. Sci. 17 (02), 38–43.
Miao, X. (2023). Study on the influence of green finance on agricultural green total factor productivity and its spatial spillover effect[D]. Shenyang Agricultural University. doi:10.27327/d.cnki.gshnu.2023.000679
Moghaddasi, R., and Pour, A. A. (2016). Energy consumption and total factor productivity growth in Iranian agriculture. Energy Rep. 2 (C), 218–220. doi:10.1016/j.egyr.2016.08.004
Olomola, P. A., and Osinubi, T. T. (2018). Determinants of total factor productivity in Mexico, Indonesia, Nigeria, and Turkey (1980–2014). Emerg. Econ. Stud. 4, 192–217. doi:10.1177/2394901518795072
Peng-fei, G. E., Song-ji, W., and Xiu-lu, H. (2018). Measurement for China's agricultural green TFP. China Popul. Resour. Environ. 28 (05), 66–74. doi:10.12062/cpre.20171010
Qin, S. (2023). Analysis on the impact of green finance on agricultural green productivity[D]. Hebei University. doi:10.27103/d.cnki.ghebu.2023.000293
Sheng, Y., Jackson, T., Zhao, S., and Zhang, D. (2016). Measuring output, input and total factor productivity in Australian agriculture: an industry-level analysis. Rev. Income Wealth 63. doi:10.1111/roiw.12250
Shi, D., and Shi, X. (2022). Green finance and high-quality economic development: mechanism, characteristics and empirical research. Stat. Res. 39 (01), 31–48. doi:10.19343/j.cnki.11-1302/c.2022.01.003
Shi, G. Z. (2022). Adhere to the concept of green development and promote the high-quality development of green agriculture in Songjiang District. Shanghai rural. Econ. (11), 28–30. doi:10.3969/j.issn.1671-6485.2022.11.010
Shi-qing, G. E., and Ling-cheng, K. (2024). Analysis of the spatial spillover effect of green finance on agricultural green total factor productivity in the yangtze river economic belt[J/OL]. J. Anhui Agric. Sci. 1-6–10-22].
Shiwen, L., and Hu, K. (2019). Estimation and prediction of contribution rate of agricultural science and technology progress in Jiangxi Province. Rural Econ. Science-Technology 30 (19), 159–163. doi:10.3969/j.issn.1007-7103.2019.19.068
Shuai, L. U., Hang-yu, Z., and Wen-jing, C. (2022). Spatial pattern and dynamic evolution of agricultural green total factor productivity in the Yellow RiverBasin. J. Ecol. Rural Environ. 38 (12), 1557–1566. doi:10.19741/j.issn.1673-4831.2021.0619
Song, X. (2023). Study on the influence of green finance on rural revitalization and development. Hubei Normal University. doi:10.27796/d.cnki.ghbsf.2023.000289
Tingting, X. E., and Meiju, F. (2023). Research on the path of empowering rural revitalization with green finance-on the mediating effect of agricultural ecological efficiency. Price Theory & Pract. (06), 174–178+212. doi:10.19851/j.cnki.CN11-1010/F.2023.06.401
Tong, W. (2024). Green finance and economic development path in China. Shanghai Enterprise, 45–47. doi:10.3969/j.issn.1004-7808.2024.02.014
Wang, X., and Feng, Z. (2013). Estimating and appraisal on the agricultural production factors in Liaocheng by C-D function. Hubei Agric. Sci. 52 (03), 734–737. doi:10.14088/j.cnki.issn0439-8114.2013.03.025
Wang, Z., Teng, Y., Wu, S., and Chen, H. (2023). Does green finance expand China's green development space? Evidence from the ecological environment improvement perspective. Systems 11 (7), 369. doi:10.3390/systems11070369
Wang, X., and Lei, Y. (2023). Evaluation of Xinjiang agricultural green total factor productivity based on SBM model. Mod. Agric. Sci. Technol. (22), 171–175. doi:10.3969/j.issn.1007-5739.2023.22.042
Wu, B., Chen, F., Li, L., Xu, L., Liu, Z., and Wu, Y. (2024). Institutional investor ESG activism and exploratory green innovation: unpacking the heterogeneous responses of family firms across intergenerational contexts. Br. Account. Rev., 101324. doi:10.1016/j.bar.2024.101324
Wu, J., Zhang, Y., and Zhang, X. (2023). Influence and mechanism of action of food production on green total factor productivity: from the perspective of spatial economics. J. Agro-Forestry Econ. Manag. 22 (04), 424–434. doi:10.16195/j.cnki.cn36-1328/f.2023.04.45
Xiao, X., and Hu, M. (2023). Research on the impact of green finance on the green development of agriculture and its spatial spillover effects and threshold effects. J. Regional Financial Res. (11), 49–61.
Xiao, X., and Miao, M. (2023). Research on the impact of green finance on the green development of agriculture and its spatial spillover effects and threshold effects. J. Regional Financial Res. (11), 49–61.
Xie, D., Hu, S., and Bao, Y. (2023b). Can green finance improve China’s urban green total factor productivity: based on data from 285 cities in China. J. China Univ. Geosci. Soc. Sci. 23, 122–137. doi:10.16493/j.cnki.42-1627/c.20230613.002
Xie, D., and Shihua, H. (2023). Green finance, maturity mismatch and green total factor productivity [J/OL]. Soft Sci. 1-16. doi:10.13956/j.ss.1001-8409.2023.06.08
Xie, H. (2024). Green finance helps low-carbon industries. Shenzhen Business Daily. doi:10.28774/n.cnki.nszsb.2024.000452
Xie, H., Wu, X., and Yang, L. (2023a). Impact of rural inclusive finance on green total factor productivity in agriculture: from the perspective of spatial spillover effect. J. Chin. Agric. Mech. 44 (04), 239–247. doi:10.13733/j.jcam.issn.2095-5553.2023.04.033
Xu, B., Tu, J., Kai, W., and Chen, X. (2023). Regional diversity and evolution of agricultural labor productivity in China. J. Chin. Hum. Resour. Manag. 14 (1), 90–105. doi:10.47297/wspchrmWSP2040-800504.20231401
Yan, P., and Wu, Y. (2024). The impact of green finance on the integration of the three industries in rural areas: an empirica research based on fixed effect model. Bus. & Econ. (02), 169–173. doi:10.19905/j.cnki.syjj1982.2024.02.041
Yang, J., and Zuo, T. (2022). Evaluation and spatiotemporal evolution analysis of agricultural green total factor productivity irYangtze river economic belt. Enterp. Econ. 41 (11), 152–160. doi:10.13529/j.cnki.enterprise.economy.2022.11.015
Yang, X., and Tong, J. (2023). Spatial spillover effects of agricultural product trade on agricultural green totalfactor productivity_-based on the regulatory role of agricultural industrialagglomeration[j/ol]. Chin. J. Agric. Resour. Regional Plan. 1-15. doi:10.7621/cjarrp.1005-9121.20231102
Yin, Z., Sun, X., and Xing, M. (2021). Study on the influence of green finance development on green total factor productivity. Statistics Decision-making 37 (03), 139–144. doi:10.13546/j.cnki.tjyjc.2021.03.030
Zhang, D., and Sun, Z. (2023). The impact of agricultural global value chain participation on agricultural total factor productivity. Agriculture 13, 2151. doi:10.3390/agriculture13112151
Zhang, J. (2006). Green insurance based on sustainable economic development. Zhejiang Finance (03), 45–47.
Zhang, L., and Jiang, X. W. (2006). Green Finance and sustainable development of rural economy in China. J. Inn. Mong. Agric. Univ. Sci. Ed. 41 (01), 232–235. doi:10.16853/j.issn.1009-4458.2006.01.089
Zhang, M., and Kui, Z. (2021). Research on the relationship between green finance and enterprise total factor productivity based on space spillover effect [J] technical economy, 40, 64–72. doi:10.3969/j.issn.1002-980X.2021.05.007
Zhang, X., and Zhang, X. (2022). Total factor productivity of herdsmen animal husbandry in pastoral areas: regional differences and driving factors. Sustainability2022 14, 15347. doi:10.3390/su142215347
Zhang, Y., Wei, J., Wang, Y., and Tsai, S.-B. (2022). An empirical study on the growth of agricultural green total factor productivity in the huanghuai river economic zone by big data computing. Math. Problems Eng., 2022. doi:10.1155/2022/1775027
Zhao, S., Zhang, L., An, H., Peng, L., Zhou, H., and Hu, F. (2023). Has China's low-carbon strategy pushed forward the digital transformation of manufacturing enterprises? Evidence from the low-carbon city pilot policy. Environ. Impact Assess. Rev. 102, 107184. doi:10.1016/j.eiar.2023.107184
Zhao, S., Zhang, L., Peng, L., Zhou, H., and Hu, F. (2024). Enterprise pollution reduction through digital transformation? Evidence from Chinese manufacturing enterprises. Technol. Soc. 77, 102520. doi:10.1016/j.techsoc.2024.102520
Zhao, Y., and Dang, G. (2023). Spatial and temporal differences and influencing factors of agricultural green total factor productivity in Anhui Province based on SBM-Tobit model. Agric. Technol. 43 (03), 128–133. doi:10.19754/j.nyyjs.20230215029
Zhao, C. (2023). Research on the impact of green finance on China's agricultural industrial structure. Shandong University of Technology. doi:10.27276/d.cnki.gsdgc.2023.000505
Zhou, Y., and Nie, Y. (2022). Dynamic measurement of green total factor productivity and regional characteristic decomposition. China statistics decision-making 38 (20), 37–42. doi:10.13546/j.cnki.tjyjc.2022.20.007
Zhu, Y. (2009). Developing commercial banks' green credit: reflections on supporting green economy Model. Econ. Forum (20), 57–58. doi:10.3969/j.issn.1003-3580.2009.20.020
Keywords: agricultural green total factor productivity (GTFP), green finance, China, EBM-GML model, spatial Durbin model
Citation: Chu L, Cheng L, Gao Y, Deng H, Wang Q and Luo Y (2024) Influence of green finance on agricultural green total factor productivity: a case study in China. Front. Environ. Sci. 12:1463833. doi: 10.3389/fenvs.2024.1463833
Received: 12 July 2024; Accepted: 31 October 2024;
Published: 26 November 2024.
Edited by:
Mobeen Ur Rehman, Keele University, United KingdomReviewed by:
Ilyas Ahmad, University of Education Lahore, PakistanCopyright © 2024 Chu, Cheng, Gao, Deng, Wang and Luo. This is an open-access article distributed under the terms of the Creative Commons Attribution License (CC BY). The use, distribution or reproduction in other forums is permitted, provided the original author(s) and the copyright owner(s) are credited and that the original publication in this journal is cited, in accordance with accepted academic practice. No use, distribution or reproduction is permitted which does not comply with these terms.
*Correspondence: Liang Cheng, Y29sZ2F0ZTc3QDE2My5jb20=
Disclaimer: All claims expressed in this article are solely those of the authors and do not necessarily represent those of their affiliated organizations, or those of the publisher, the editors and the reviewers. Any product that may be evaluated in this article or claim that may be made by its manufacturer is not guaranteed or endorsed by the publisher.
Research integrity at Frontiers
Learn more about the work of our research integrity team to safeguard the quality of each article we publish.