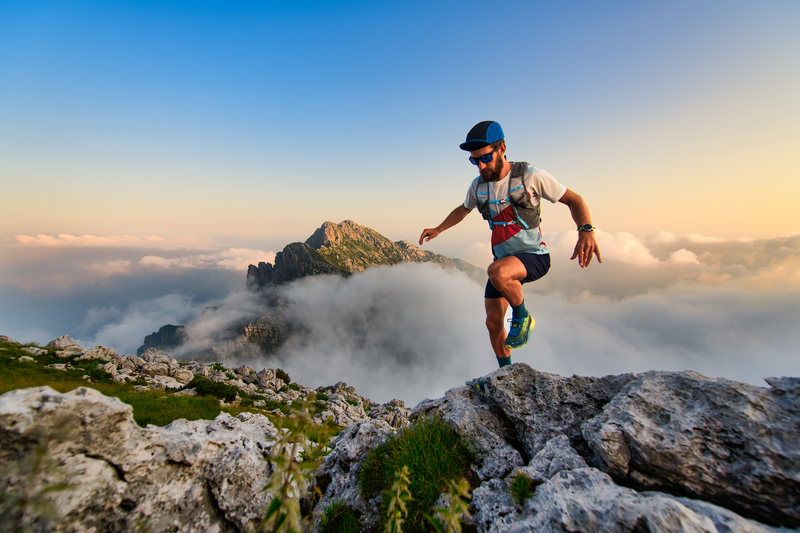
94% of researchers rate our articles as excellent or good
Learn more about the work of our research integrity team to safeguard the quality of each article we publish.
Find out more
ORIGINAL RESEARCH article
Front. Environ. Sci. , 05 November 2024
Sec. Freshwater Science
Volume 12 - 2024 | https://doi.org/10.3389/fenvs.2024.1451394
This article is part of the Research Topic Advances in Fluvial, Coastal, Wastewater and Hydro-Environment Systems, Volume II View all 4 articles
Wetland ecosystems provide numerous services to humanity and the biosphere at large. However, these services are constantly dwindling due to the massive wetland loss caused by urbanization. Urbanization-induced wetland loss is a global problem, with most developing countries experiencing it currently. Several studies on wetland loss have been conducted. However, there is a dearth of studies integrating GIS and other ecological models to estimate the periodic deterioration of wetlands in rural settings in underdeveloped nations. As a result, this study aims to determine wetland loss in a developing country’s countryside, using the Greater Amanzule Wetland in the Ellembelle District of Ghana as a case study. The study used the GIS and Remote Sensing dataset, the Normalization Difference Vegetation Index, and the InVEST-Habitat Quality model to analyze the spatial pattern of wetland loss and habitat quality variations caused by industrialization and community expansions between 2000 and 2020. Our results indicate that, from 2000 to 2015, community built-up and industrial land areas grew from 25.19 ha to 84.21 ha and 0–4.71 ha respectively, causing a wetland loss of −39.64 ha (−31.10%). Between 2015 and 2020, community built-up land grew to 78.65 ha while industrial land slightly reduced to 2.54 ha, leading to the wetland degradation of approximately −69.06 ha (−42.53%). Wetland habitat quality steadily declined, from 0.97 in 2000 to 0.93 in 2015 and 0.93 in 2020. The study findings led to policy recommendations to reduce wetland encroachment and enhance sustainability. These recommendations included conducting effective land-use plan inspections before issuing permits, coordinating effective management, and promoting the recovery of natural and artificial vegetation to sustain the wetland.
Wetland ecosystems provide adequate services to the biosphere and society in several places where they are found (Cao and Anthony, 2009). These wetland services include climate change regulation, water purification, biodiversity conservation, groundwater restoration, flood control, storm protection, wetland product supply, cultural values, and tourism and recreation centers (Cao and Anthony, 2009; Jafari, 2009; Mao et al., 2018). Specifically, mangrove trees considered one of the dominant species of the wetland ecosystem, are well known for their numerous ecosystem services, especially carbon sequestration and sinking for atmospheric carbon (Junk et al., 2013; Ramsar Convention Secretariat, 2013; Yevugah et al., 2017). The degradation and loss of wetland ecosystems globally over the past decade are alarming.
Wetland degradation is the loss of wetlands’ ecological health (Douglas, 2017; Finlayson et al., 2015), involving changes in structure and function caused by many ecological and environmental disasters, especially human activity (Cazenave and Robert, 2010; Cui et al., 2018; McCauley et al., 2013). It is estimated that 50% of the world’s salt marshes and 35% of mangroves have been damaged, with the proportion nearing 90% in some locations (Douglas, 2017; Gibson et al., 2015; Ramsar Convention Secretariat, 2013). For instance, the ever-increasing human population and the desire to fulfill unlimited human demands through urbanization and industrialization have resulted in the degradation and extinction of natural resources, contributing to the loss of ecosystem services as well as increased ecological consequences such as the loss and fragmentation of natural habitats, alterations in water quality, and decreased food supply (Grumbine, 2014; He C. et al., 2014; Kuchay and Bhat, 2014; Mao et al., 2018; Zhao et al., 2006). The continued expansion of urban centers has aggravated the ecological vulnerability of wetlands and caused obvious ecological consequences (He Q. et al., 2014; Mao et al., 2018; Wang et al., 2020). Deliberate efforts to address and solve the causes of wetland degradation are ultimately necessary to make sounder management decisions (Kuchay and Bhat, 2014; McCauley et al., 2013). Thus, it is critical for ecological management in rapidly changing and human-dominated environments to properly assess and monitor wetlands and their habitat quality changes, as well as determine the mechanisms underlying these changes. (Sun et al., 2019).
Researchers have heavily employed remote sensing methodologies in several studies assessing the causes of wetland degradation and its conservation. Most of these studies focused on urbanization-induced wetland loss (Cui et al., 2018; Mao et al., 2018) at the regional scales of economically developed countries such as Portland in the United States of America (Kentula et al., 2004), Lianyungang City in China (Li et al., 2014), and Balcovas’ Delta in Turkey (Bolca et al., 2007). However, there is a dearth of studies integrating GIS and other ecological models to estimate the periodic deterioration of wetlands in local or rural settings in underdeveloped nations.
Remote sensing (RS) enables the evaluation of LULC changes in regional and local landscapes as well as the estimation of socio-economic repercussions on the surrounding ecological resources, including wetlands (Shuangao et al., 2021). Compared to traditional techniques that rely on field surveys and single-sensor satellite images (Sun et al., 2019), available RS techniques and related indexes, including the Normalization Difference Vegetation Index (NDVI), accurately calculate spatial ecological changes and are convenient and reliable for monitoring LULC and ecological changes (Wu and Ke, 2016). Consequently, RS techniques and the NDVI have been extensively employed to evaluate changes in land use and land cover (LULC) and vegetation cover changes across various countries worldwide (Holland et al., 1995; Mao et al., 2018; Ochoa-Gaona et al., 2010; Tian et al., 2017; Zhai et al., 2020).
The NDVI differencing and classification method has emerged as the frequently used change detection method for past years, providing detailed and accurate information for monitoring and determining LULC changes (Ehsan and Kazem, 2013; Huang et al., 2021; Kaku et al., 2021). The most popular indexation for vegetation assessment today is NDVI, a remote sensing analytical product that simplifies complex multispectral imagery. The widespread usage of NDVI is due to its ability to be easily calculated with any multispectral sensor with a visible and near-IR band (Alex et al., 2017; Huang et al., 2021; Kaku et al., 2021; Zaitunah et al., 2018). The NDVI can detect changes in vegetation at regional and global scales using reflective bands of satellite data. NDVI-generated images are very convenient and practical for determining vegetation dynamics between multiple-year periods (Ehsan and Kazem, 2013; Drisya et al., 2018; Choubin et al., 2019; Huang et al., 2021). The NDVI image values can be deduced by subtracting two or more different times pixel by pixel to create the differentiated image (Diallo et al., 2010; Huang et al., 2021; Kaku et al., 2021). Lyon et al. (1998a) examined seven vegetation indices using Landsat MSS image data for land cover change analysis from three dates and concluded that the NDVI differencing technique was the most suitable for vegetation change detection (Huang et al., 2021; Lyon et al., 1998b; Lyon et al., 1998a).
Over the years, the NDVI and other numerous ecological assessment indexes such as the Soil Adjusted Vegetation Index (SAVI) (Faisal et al., 2019) and the Normalized Difference Built-up Index (NDBI) (Faisal et al., 2019) were primarily concerned with determining any disruption to the structure, process, function, stability, and sustainability caused by threat factors external to the ecosystem, as well as identifying the ecosystem’s hazards (Terrado et al., 2016). Recently, vegetation and ecological assessment efforts have shifted toward comprehending and embracing the distribution of threats to design and implement biodiversity conservation and management strategies. Techniques for mapping threats consist of mapping the prevalence of a specific threat and scoring methods for multiple threats, such as ecosystem vulnerability and sensitivity (Terrado et al., 2016; Uerbach et al., 2014). The Integrated Valuation of Environmental Services and Tradeoffs (InVEST) model uses threat mapping analysis to track changes in ecosystems and trends in habitat quality while accurately estimating how much damage is caused by threat variables. This makes it a useful tool, especially in places where biodiversity data is difficult to get (Sharp et al., 2020).
The InVEST-Habitat Quality (HQ) model is the most commonly used program for ecological service assessment and spatialization, with a complete procedure for biodiversity evaluation (Sharp et al., 2020; Sun et al., 2019; Xu et al., 2019). The habitat quality model can determine the impact of human activities on terrestrial ecosystems. This model assumes a relationship between a high level of biodiversity and good habitat quality (Sun et al., 2019; Zhai et al., 2020). Habitat quality refers to an ecosystem’s ability to offer the circumstances essential for the survival and reproduction of species at a given location (Zhai et al., 2020). The model assumes that biodiversity patterns are intrinsically spatial (Sun et al., 2019). Habitat quality can be estimated utilizing geospatial data, such as LULC maps, threat layers, habitat categories, and threat-specific habitat sensitivities (Sharp et al., 2020; Zhai et al., 2020). The model’s advantages include simple operation, data processing efficiency, quick parameter access, and the requirement of minimal parameters (Terrado et al., 2016; Wu et al., 2014). This has led to its extensive use in habitat quality evaluations across the globe (Sallustio et al., 2017; Sun et al., 2019), demonstrating its advantages for resource and environmental management, as well as land-use planning (Li et al., 2018; Wu et al., 2014; Xu et al., 2019).
Nevertheless, there is a scarcity of research using RS, the NDVI, and the HQ model, as most previous studies used them separately to evaluate the influence of anthropogenic activities on wetland ecosystems. Furthermore, previous studies on wetland loss, including Kuchay and Bhat (2014), McCauley et al. (2013), USAID GHANA (2017), Kentula et al. (2004), and Li et al. (2014), have failed to categorize wetlands into distinct categories, such as river, lake, and wetland vegetation species, to estimate the extent to which threat factors have impacted these categories.
Locally in Ghana, wetlands conservation and ecological restoration have received much attention due to the speedy deterioration of wetlands over the past years, especially coastal wetlands (Abdullah, 2018; Bernard et al., 2020; Coastal Resources Center, 2013; Kumi et al., 2015). As a developing country, Ghana’s cities and regions are rapidly urbanizing and industrializing, negatively impacting local ecologies (Adams et al., 2016; Addae and Oppelt, 2019; Ashiagbor et al., 2019; USAID GHANA, 2017). The Western Region of Ghana is expected to be one of Ghana’s and Africa’s greatest industrial zones in the coming years due to the abundance of natural resources in the area. (Ackah et al., 2014). Coastal districts of Ghana’s Western Region, especially the Ellembelle and Jomoro Districts, face several land-use pressures (Mpoano, 2018). Coastal wetlands and inland forests are damaged to provide space for commercial and economic activities like establishing industries, artisanal small-scale, and large-scale mining (Addae and Oppelt, 2019; Mensah et al., 2015; Owusu-Adjapong, 2018; Owusu-Nimo et al., 2018; USAID GHANA, 2017).
Despite the adverse impacts on wetlands caused by accelerated urbanization and industrial development in the region, community land use and industrialized-induced wetlands loss receive less attention. The status and extent of wetland degradation, as well as the habitat quality variations, are rarely examined. Therefore, based on updated datasets retrieved from satellite images, this study uses the Greater Amanzule Wetland (GAW) in the Ellembelle district of Ghana as a case study to primarily assess the impacts of industrial establishments and community land-use expansions on wetlands. The study specifically aims (a) to assess land-use changes from 2000 to 2020, (b) to examine the land cover density and habitat quality changes in the wetland area, and (c) to identify conservation and restoration measures implemented in the region following the nature and level of degradation. The findings offer a scientific foundation for the protection and comprehensive management to enhance the wetland’s sustainability in the study area and beyond.
The study was conducted on a portion of the Greater Amanzule Wetland (GAW) along the Amanzule River in the Ellembelle district of Ghana. The Greater Amanzule Wetland is an important natural wetland ecosystem located in the coastal zone of the district, with mangroves as the dominant species (Mpoano, 2018; Yevugah et al., 2017). The GAW functions as a home for various animal species, including crocodiles, crabs, snails, monkeys, and tortoises. It also functions as a crucial habitat for migratory birds to forage and repose. (Mpoano, 2018; USAID GHANA, 2017; Yevugah et al., 2017). The Ellembelle District constitutes the 14 Metropolitan, Municipal, and District Assemblies (MMDAs) in the Western Region of Ghana, and it is found at the southern part of the region between longitudes 2005′W 2035′W and latitude 4040′N and 5020′N. It has a total area of 995.8 km2, representing about 9.8% of the entire land surface of the Western Region (Ghana Statistical Service, 2014; Ghanadistricts.com, 2019). It lies within the semi-equatorial climatic zone of the West African sub-region and has moist semi-deciduous rainforest vegetation but changes to secondary forest southwards due to human activities like logging and farming (Ghana Statistical Service, 2014). The Ellembelle district is recognized as a resource-based district and has proven to be an industrial hub due to the massive extraction of gold and crude oil. Most of the industries are concentrated along the coast, where the wetland is the primary landscape of the area (Ghana Statistical Service, 2014; Mpoano, 2018; USAID GHANA, 2017). Industrial activities such as crude oil extraction and gold mining are vital factors contributing to the district’s economic development and detrimental to the regional eco-environment (Ackah et al., 2014; Ghana Statistical Service, 2014; Kaku, 2018; Kaku et al., 2021). Figure 1 shows the study area map obtained from the Ellembelle District map.
Distribution datasets were extracted by collecting three Landsat images of the study area (Landsat7, ETM+) for the year 2000 and (Landsat8, OLI) images for the 2015 and 2020 years (Table 1) from the archives of the United States Geological Survey (United State Geological Survey, 2021; http://earthexplorer.usgs.gov) to analyze the LULC changes in the area. These images have a spatial resolution of 30 m, covering the entire study area. The processing template parameter in the ArcGIS software was used to process these images. Additionally, existing literature concerning the study area and the study domain was accessed from governmental agencies to support the analysis.
This study extracted different land-use and wetland clusters through GIS techniques, a remote sensing dataset, the Normalized Difference Vegetation Index (NDVI), and the Invest-habitat quality model to create Land-use Land-cover (LULC) and habitat quality maps. Excel (Microsoft, Redmond, DC, United States of America), ArcGIS (Esri, Redlands, CA, United States of America), ENVI 5.3, InVEST 3.10.1, and OriginLab software (OriginLab, Northampton, United Kingdom) were used to analyze and process available data. Results were presented as summary statistics in tables, graphs, and maps.
Using the NDVI, we estimated vegetation values by analyzing and detecting wetland and other land cover changes in the study area. The statistical definition of the NDVI data layer is provided by (Ehsan and Kazem, 2013; Huang et al., 2021; Lyon et al., 1998b):
where NDVI is the normalized difference vegetation index. Red and NIR are spectral radiance (or reflectance) measurements recorded with sensors in red (visible) and NIR regions, respectively. Radiance (watts steradian−1 m−2 μm−1) measures energy flux recorded by a sensor. The values of radiance are often rescaled to digital numbers (DN) as 6-bit or 7-bit (MSS), 8-bit (TM, ETM+), or 12-bit (Landsat8) unsigned integers. Reflectance is a unitless measure of the ratio of radiation reflected by an object relative to the radiation incident upon it (Hilson and Nyame, 2006; Huang et al., 2021). The NDVI values should be greater than zero (0) and between −1 and +1, where rising positive values indicate healthy and green vegetation. Negative values denote sparse or non-vegetated surface features such as barren land or water, snow, or clouds. The presence of atmospheric effects causes the red and near-infrared wavelengths to scatter, affecting the NDVI calculation. The use of reflectance is significant in controlling the impact of scattered radiation in the atmosphere. The changes in NDVI values among different objects are due to their relative changes in spectral responses. Therefore, Equation 1 is modified as follows (Huang et al., 2021; Kulawardhana, 2011):
where NDVI is the normalized difference vegetation index. R is the ratio of NIR to Red and is commonly referred to as the ratio of vegetation index (Huang et al., 2021; Kaku et al., 2021). Equation 2 is explicit for defining NDVI behavior patterns due to different responses of NIR and Red to atmospheric effects (Huang et al., 2021; Mcveagh et al., 2012).
The study used Surface Reflectance Products, i.e., atmospherically corrected images (USGS archives), and ArcGIS software to confirm the removal of atmospheric scattering and absorption effects, followed by supervised classification to create land cover types. It was carried out based on confirmed results from the Google Earth map and existing topographical maps, which were used as reference data. Subsequently, spectral signatures were employed to classify all images’ pixels by creating a group of random training points (50 points for each class) (Shuangao et al., 2021). LULC varieties were represented in the training area. The maximum likelihood in the ArcGIS software was used to classify images to generate four (4) LULC types based on the training area. These LULC types were wetlands, community land, Industrial land, and Empty land (Table 2). Wetlands were further categorized into river, lake, and wetland vegetation by combining the NDVI, object brightness, and texture. Human knowledge about the study location was utilized to support and enhance the accuracy of the LULC classification processes. A definitive false-color image composite of the near-infrared, blue, violet, cyan, and green bands was used to depict the various land cover classes, and LULC maps were generated.
An investigation was done to quantify the differences in each land cover type (during the three-time intervals) to identify where the changes occurred using the change detection statistics tool in ArcGIS 10.3. In detecting areas with LULC changes, cross-tabular calculations were performed on the three (3) image values. The previous year’s image values were subtracted from the subsequent year’s LULC image values [Equation 3], resulting in the two (2) stages; 2015–2000 is stage 1, and 2020–2015 is stage 2. An overlay comprising LULC maps of 2000, 2015, and 2020 was developed through the ArcGIS software, and a transition matrix was done for the overlaid LULC maps. Based on the NDVI values from Equation 2, the vegetation density was determined by subtracting the values between the three study periods to quantify the changes and reclassifying them as low, medium, and high density by using the equal interval tool in ArcGIS. The NDVI density values range from −1 to 1, with −1 the lowest and 1 the highest. Dense vegetation is indicated by values close to 1. NDVI density values vary from −0.1 to 0.1 for low density, 0.1 to 0.2 for medium density, and 0.3 to 1 for high density (Alex et al., 2017). These density class definitions led to the categorization of NDVI density into three classes: low-density, medium-density, and high-density, which represent wetland areas and LULC types. Low-density areas have NDVI values from −0.37 to 0.03, medium-density areas have values from 0.03 to 0.23, and high-density areas have values from 0.23 to 0.47. A definitive color of the red, yellow, and green bands was used to depict the three density classes, and an overlay comprising NDVI density maps of 2000, 2015, and 2020 was generated.
Where LULCC is the Land-use/Land-cover changes, Sy is the subsequent year value, and Py is the previous year value.
The InVEST’s Habitat Quality module (InVEST-HQ, v3.4.2) (Sharp et al., 2020; Sun et al., 2019) was used to evaluate the GAW area’s habitat quality; this model established information on ecosystem types and anthropogenic disruption variables based on LULC. The model’s primary objective is to establish a link between habitat quality and threats, specifically to calculate the negative impact of threats on a habitat, determine the level of habitat degradation, and then compute habitat quality by integrating habitat suitability and degradation levels. The habitat quality index measures the habitat’s quality and the extent to which it has been fragmented due to threats (Terrado et al., 2016). The ecological structure and habitat quality are stable in places with high values. The anti-interference capability is limited in low-value places, and the ecological environment is easily harmed. The habitat quality scores ranged from 0 to 1. The poorer the habitat quality, the lower the value, and vice versa. The overall habitat quality values were categorized into three (3) classes based on the habitat quality class definition by (Sharp et al., 2020; Sun et al., 2019; Zhai et al., 2020) Equation 4. The following formula is used to determine habitat quality (Sharp et al., 2020; Sun et al., 2019; Zhai et al., 2020):
where
The study’s primary objective is to examine the impacts of land-use change; therefore, it did not consider the numerous anthropogenic factors, such as alien species invasion, that function as sources of habitat degradation. Based on the ecological features and the LULC maps generated for the study, community expansions, and industry were selected as the major threat factors to habitat. The model also assumes that there is no habitat in the bare area and is therefore considered a threat factor with a lower sensitivity. Each threat map was derived from land-use maps. The extent of influence, weight, the sensitivity of habitat types to threat factors, and other parameters like habitat suitability (Tables 3, 4) were chosen and assigned following the InVEST VERSION User’s references, previous studies (Sun et al., 2019; Zhai et al., 2020) and expert suggestions.
The spatial extent of community built-up and industrial land constantly increases, while wetland vegetation and bare land decrease throughout the study periods. As shown in Table 5, the spatial values of wetland vegetation reduced from 73.93% to 70.73% between 2000 and 2015 and further decreased to 62.20% between 2015 and 2020. Furthermore, the river’s spatial values decreased from 1.94% to 1.49% between 2000 and 2015, and then slightly expanded to 1.85% in 2020. Similarly, the lake experienced a reduction from 3.10% to 1.97% from 2000 to 2015, followed by an expansion to 2.06% in 2020. Between 2000 and 2015, Community built-up land expanded from 2.98% to 9.95% and massively increased to 19.27%in 2020. From 2000 to 2015, Industrial land rose from 0% to 0.56%and expanded to 0.86%between 2015 and 2020. Between 2000 and 2015, Bare land’s spatial values decreased from 18.05% to 15.30% and further decreased to 13.76% between 2015 and 2020. However, comparisons in the overall percentage changes in LULC categories between stages 1 and 2 show that community built-up land hugely expanded by 46.31%, and industrial land expanded by 3.70%; this directly affected other land cover categories in stage 1 by significantly reducing their spatial values. Wetland vegetation was reduced by −20.65%, river decreased by −2.97%, lake decreased by −7.48%, and bare land was reduced by −18.90%. Again, slight decreases in community built-up land and industrial land to 46.28% and 1.49% directly affected wetland categories and bare land in stage 2. Figures 2, 3 represent the percentage changes in all LULC categories, as well as the LULC classified maps of the study area for 2000, 2015, and 2020.
Table 5. Comparisons of areas and patterns of changes in LULC categories between 2000, 2015, and 2020.
Despite the availability of bare land to absorb the increasing community expansions and industrial activities, the rate of industrial and community-induced wetland loss was very severe. As presented in Table 6 and Supplementary Figure S2 in stage 1 (2000–2015), community built-up and industrial lands covering an area of 59.02 ha and 4.71 ha resulted in the loss of −39.64 ha of wetland, representing −31.10%, while −24.10 ha representing −18.91% of bare land was also captured. In stage 2 (2015–2020), community built-up land increased to 78.65 ha, and industrial lands slightly reduced to 2.54 ha, causing the disappearance of −69.06 ha of wetland and −12.13 ha of bare land, representing percentage losses of −42.53% and −7.47%, respectively. This shows that wetland loss was higher in stage 2 than in stage 1.
Table 6. Area (Ha) of wetland and bare land losses to community built-up and industrial lands within stage 1 (2015–2000) and stage 2 (2020–2015).
There were significant changes within all density classes during our study periods. Between 2000 and 2015, land cover in low-density and high-density classes reduced from 16.34% to 9.77% and 59.03%–52.96%, respectively, while the medium-density increased from 24.63% to 37.27%. Similarly, between 2015 and 2020, there was an increase in low- and medium-density areas, from 9.77% to 17.70% and 37.27% to 46.42, while high-density areas massively decreased from 52.96% to 35.88%. As presented in Table 7 and Figure 4, the medium-density category kept rising within all years, while the low-density and high-density categories changed from 1 year to another. In the NDVI density change analysis, these three categories indicate the changes in the area within the 20-year (2000, 2015, 2020) period. The low-density category in red indicates a negative change, the medium-density category in yellow indicates no change, and the high-density category in green shows a positive change. Figure 5 displays the NDVI density maps of the study area for the years 2000, 2015, and 2020.
Table 8 displays the results of the InVEST-HQ model’s simulation of habitat quality from 2000 to 2020, indicating a continuous decline in habitat type qualities in the GAW area due to the high intensity of land development and exploitation. Between 2000 and 2015, the habitat quality of wetland vegetation decreased from 0.99 to 0.91 and then further decreased from 0.91 to 0.86 between 2015 and 2020, as degradation levels increased from 0.02 to 0.04 and 0.07, respectively. Between 2000 and 2015, the river’s habitat quality decreased from 0.99 to 0.96 and marginally increased from 0.96 to 0.97 in 2020, decreasing the degradation level from 0.02 in 2000 to 0.01 in both 2015 and 2020. Similarly, from 2000 to 2015, the lake’s quality slightly decreased from 1.00 to 0.99, and then further to 0.98 from 2015 to 2020, with an increase in deterioration level from 0.01 to 0.02.
Except for the river, which had habitat quality variations, wetland vegetation and lake habitat qualities constantly decreased across the periods, with wetland vegetation experiencing a sharp decline. The overall habitat quality of the GAW area reduced from 1 to 0.97, to 0.93 in 2000, 2015, and 2020, respectively.
The spatial variance in habitat quality was obvious, as the spatial pattern of habitat quality decreased while the spatial pattern of threat factors continued to increase. As presented in Figure 6, the low-class areas in red have no (0) habitat quality values, indicating the presence of serious threats that caused severe habitat degradation. The habitat values in the medium-class areas ranged from 0.01–0.9, indicating fewer threats and minimal habitat loss. These areas are denoted with yellow color and are areas where rivers and lakes are located in the GAW.
The habitat quality values in the high-class areas ranged from 0.91 to 1, indicating good habitat quality due to the absence of threats in such areas. These areas are denoted by a green color and are widely dominated by wetland vegetation. Between 2000 and 2015, the spatial values of community built-up areas increased from 13.34% to 38.55%, while industrial land values increased from 0% to 2.12%. These changes resulted in a decline in the high-habitat quality class from 73.93% to 70.81%, while the low-habitat quality class increased from 21.03% to 25.84%. Again, between 2015 and 2020, community built-up exponentially grew from 38.55% to 57.28%, while industrial land grew slightly from 2.12% to 2.44%. This huge rise resulted in a dramatic decrease in the spatial values of the high-habitat quality class, from 70.81% to 62%, while the spatial values of the low-habitat quality class grew from 25.84% to 33.89% (Table 9). Over these periods, the spatial values of the medium-habitat quality class slightly decrease. Specifically, the consistent declines in the high-habitat quality class and the rise in the low-habitat quality class from 2000 to 2020 indicated pervasive habitat degradation across the GAW area. Figure 7 shows the spatial distribution of threat factors from 2000 to 2020.
Figure 7. The spatial distribution of threat factors in 2000, 2015, and 2020: (A) Community built-up, (B) Bare land, and (C) Industrial land.
This study revealed the emergence of industrial activities and the apparent expansion of communities, which led to a decrease in the wetland and consequent impacts on the area’s habitat quality. A study by the USAID GHANA (2017), reported that about 17 ha of wetland vegetation (mangroves and swamps) in Ellembelle were lost from 2016 to 2017 but failed to single out the causes of the wetland loss. However, in this study, we advanced a related work showing the percentages of wetland loss caused by industrialization and rural community expansions within 2 decades using remote sensing observations. Further literature reviewed shows that globally, urban development poses severe threats to wetlands in metropolitan areas, such as those in central Florida in the United States of America (McCauley et al., 2013), South Korea (Murray et al., 2014), Inner Mongolia (Tao et al., 2015), Shuangtai estuary (Tian et al., 2017), and Jiangsu in China (Cui et al., 2018; Mao et al., 2018). Notwithstanding the scale and spatial extent of this study, the findings strongly agree with the conclusion that the inadequate assessment of wetland conservation and ecological security globally is a result of the limited studies on urbanization-induced wetland loss.
Within the last decade, the population of the Ellembelle district has increased tremendously, primarily due to the large-scale gold mining activities and the discovery and subsequent production of oil and gas in 2010 (Baah, 2015; Ghana Statistical Service, 2014). The establishment of the Ghana Gas processing plant in Ellembelle district (Atuabo) in 2011 has led to the concentration of many industries and the setting up of hospitality and recreational firms in the coastal part of the district in subsequent years. These factors remarkably drove the expansion of coastal communities in the district. As an industrial hotspot area with increasing industrialization in its coastal part, wetland degradation was evident in the GAW (Figure 2), where large tracts of the wetland have been converted to industrial and residential lands over the past decade. The continued population growth of coastal communities and the increasing demand for space for residences and infrastructure resulted in the encroachment and degradation of various wetlands categories, especially the wetland vegetation (mangroves and swamps) and lakes in the area (Table 5) (Mpoano, 2018; Yevugah et al., 2017).
The study found that over the past 2 decades, community built-up lands, including residential land, community electrification expansion projects, community roads, recreational land, and gardens, have posed a significant threat to the GAW. Community built-up land mainly encroaches on the wetland vegetation category: mangroves and swaps. Rural communities are directly engaged in destroying wetland vegetation by continuously cutting mangrove trees for firewood, charcoal production, and other domestic purposes (Ablo and Asamoah, 2018; USAID GHANA, 2017; Yevugah et al., 2017). The commencement of industrial activities in 2010 worsened the destruction of wetlands in the area. Ghana Gas Company Ltd.'s oil and gas exploration has dragged other oil companies such as Quantum LPG Terminal and ENI Oil and Gas Exploration and Production to the area in 2015. The increase in the oil production base led to the construction of more private roads and pipelines to transport gases to various stations. Unlike community built-up land, industrial land negatively affected all wetland categories such as river, lake, and wetland vegetation (Table 5). The degradation of wetland vegetation, shrinkages, and diversions of lake and river flows by private industrial roads and pipeline constructions resulted in −31.10% wetland loss in stage 1 of the study period. Increasing industrialization and rapid community expansion caused massive fragmentation and wetland loss of −42.53% in stage 2 of the study period (Table 6 and Supplementary Figure 2).
Developmental lands absorbed significant portions of the wetland area, and the extent of landscape fragmentation intensified. The area’s excessive use and exploitation of wetland ecological resources resulted in a dramatic drop in natural asset reserves and the supply of wetland ecosystem services, while demand for such services increased, posing severe dangers to the wetland (Mpoano, 2018; USAID GHANA, 2017). The severe deforestation and a steady decline in the area’s high-habitat quality (Table 8; Figure 6) affect ecological services, including the protection of species variety and water conservation. This has resulted in the extinction and emigration of wetland animal species such as monkeys, birds, rabbits, and crabs which are usually found at the available tourist site (Nzulezo silt village) within the wetland area, thereby negatively affecting tourism activities (Mpoano, 2018; USAID GHANA, 2017).
Meanwhile, artificial alterations to the wetland environment, such as industrial activities and community land usage, present significant threats to the survival of the wetland vegetative species like mangroves and swamps. The persistent encroachment on the wetland ecosystem has a detrimental effect on the wetland’s hydrological functions and processes, weakening its ability to adjust to rainfall, resulting in environmental catastrophes such as urban flooding and severe droughts (Jafari, 2009; Mao et al., 2018; Yang and Pang, 2006). Wetlands significantly contribute to the optimization of the built environment by mitigating the impact of the urban heat island effect, haze, sand storms, degradation, and ongoing threats to the quality of the wetland habitat, thereby restricting its functions (Ablo and Asamoah, 2018), and these are evidenced by the constant rise in temperature in the district’s oil and gas refinery zones.
Additionally, the continuous deterioration of the wetland’s habitat quality has created a significant supply-demand mismatch for ecological services, including a decrease in the wetland’s capacity to sequester carbon in response to global climate change. An existing study postulated that the cumulative carbon sequestration anticipated for 2015 based on the wetland’s size was 1.550 Mt, with significant reductions expected in the following years due to severe human effects on the wetland (Allred et al., 2015; Yevugah et al., 2017). This study’s results show that the spatial value of the GAW keeps declining as community expansions and industrialization continue to boom in the area.
The irreversible transformation of wetlands into industrial and residential lands, unlike aquaculture land usage and agricultural reclamation, implies the permanent loss of certain critical wetland ecological services, leading to a variety of long-term human health consequences. Fortunately, wetland shrinkage and degradation have attracted the attention of governments, local authorities, and NGOs. To prevent further degradation, it became critical to delineate and map the exact surface area of GAW (USAID GHANA, 2017; Yevugah et al., 2017). Wetland mapping is essential for various reasons, including decision-making in resource management and monitoring the impact of human and natural factors on resources over the years. Between 2014 and 2018, seventeen communities had their wetland areas mapped, ten of which fell within the Ellembelle District. The mapping helped authorities identify degraded portions of the wetland, which became the target for restoration (Mpoano, 2018).
Again, re-planting degraded wetland vegetation, such as mangroves, is an effective wetland restoration method in the area. The Ellembelle District Assembly collaborated with a wetland conservation NGO (Hen Mpoano) to establish a mangrove nursery center to train the community conservation committee members in mangrove nurseries and planting techniques. From 2017 to 2018, this mangrove nursery center raised about 10,000 mangrove seedlings to be re-planted, and about 20.5 ha of mangrove were re-planted in Bakanta, Kamgbunli, and Ampain wetland areas (USAID GHANA, 2017). In addition, awareness creation on wetlands occurs through media engagement and community video show programs. Most communities in the wetland area are particularly aware of the benefits of mangrove services, such as building poles and domestic fuelwood for cooking. Therefore, the wetland was historically managed as a communal resource, paying little attention to its sustainability. Efforts to engage individuals in awareness-raising events resulted in minimal participation until the implementation of a “community video show program” in 2016. This program aired video presentations in different communities and schools, aimed at educating people about the extensive advantages of the wetland and the enduring effects of its degradation (USAID GHANA, 2017). This served as a platform to create a larger audience for education to ensure wetland sustainability.
Despite significant efforts to protect wetlands and address community expansion and industrial-induced ecological issues, the rate of wetland loss from developmental lands escalated after 2015 due to high industrial-induced wetland loss. According to this study’s findings, the spatial value of the GAW continues to decline as the area’s community expansion and industrialization expand. Our results show that the coastal areas were the hotspots of wetland loss due to communities and industrial development (Figure 2). The NDVI and HQM analyses (Tables 7, 9; Figures 5, 7) reveal a rapid increase in areas with low density and low habitat quality, while areas with high vegetation density and high habitat quality have continued to decrease since 2015. After the 2015 period, the degradation levels of most wetland categories, except rivers, started increasing (Table 8). All these areas are located within the coastal zones of wetland ecosystems, where the loss of wetlands accounted for over 75% of the GAW’s losses between 2000 and 2020. Therefore, it is crucial to prioritize and implement restoration measures in these areas.
This study demonstrates that integrating GIS and remote sensing with the InVEST habitat quality model provides more advantages for analyzing land use changes and their effects on wetlands than using GIS and RS alone. The InVEST model leverages remote sensing data to assess wetland habitat quality through various ecological indicators, such as vegetation cover and hydrology. This combination yields clear visualizations through the density, threat, and habitat quality maps, helping stakeholders understand the extent and impact of degradation. Furthermore, it offers a superior understanding of wetland degradation compared to studies like Mao et al. (2018), Kaku et al. (2021), Cui et al. (2018), and others that relied solely on GIS and remote sensing. This approach enhances data accuracy, identifies key stressors, and facilitates effective management, ultimately improving conservation outcomes for these vital ecosystems.
Nonetheless, the study encountered considerable limitations that we intend to address in future studies. Specific significant wetland categories like mangroves and swamps were combined as wetland vegetation due to their close nature and the resolution of the satellite images (30 m), which was not high enough to distinguish these two wetland categories. Similar studies by Ekumah et al. (2020) conducted in the study region also encountered the same problems and merged important LULC categories into one. The study’s two time intervals (2000–2015 and 2015–2020) were unequal. Although Landsat satellite images have been accessible since 1972, most did not match the study’s criteria due to too many clouds, making acquiring required images difficult. When performing NDVI analysis, the choice of image acquisition months may pose a significant constraint, depending on the study’s objectives and the geographical area of interest (Huang et al., 2021; Lyon et al., 1998b; Lyon et al., 1998a). Consequently, choosing image acquisition months spanning various seasons (from summer to winter) is prudent to attain precise results. The three image acquisition months (December, January, and February) used by this study fall within the same season, which could impact the NDVI analysis, especially when applied in different regions. Significantly, because the study area is located in a tropical zone with evergreen vegetation, the study’s analysis was unaffected by an uneven time interval or the months of image collection. However, to ensure accurate NDVI results, future studies should use images that span all seasons in the year. This will also improve the accuracy of habitat quality results since InVEST’s Habitat Quality module heavily relies on the LULC map for analysis.
This study utilized multisource satellite images to evaluate the effects of industrial establishments and community expansion on wetland loss in rural areas. The study reveals the relationship between wetlands and habitat quality losses, along with the threat factors of industrialization and community expansion. According to our analysis, the degradation of 108.7 ha of the GAW occurred between 2000 and 2020. Wetland degradation reached its peak in 2015, largely due to the start of industrial operations in 2011 and subsequent years. However, community built-up causes more wetland loss than industrial activity. Throughout the study period, the overall habitat quality of the GAW area continued to deteriorate.
Despite recent significant efforts made to conserve wetlands in recent years, a lot more needs to be done by responsible authorities to ensure the GAW’s sustainability. As a result, we recommend that the Town and Country Planning Department and the EPA should control the rapid expansion of community lands by thoroughly examining community land use plans before issuing land use permits to enhance the efficiency of community land use. Authorities should formulate policies to restrict wetland encroachment for communities and industrial activities to be directed to the bare lands in the area, ensuring effective stakeholder coordination in the management of the wetland, and practicing natural-artificial vegetation recovery technique by introducing a new suitable plant species to the wetland area and managing it for the degraded vegetation to be gradually restored.
The original contributions presented in the study are included in the article/Supplementary Material, further inquiries can be directed to the corresponding author.
UD: Conceptualization, Data curation, Writing–original draft, Writing–review and editing, Investigation. CY: Funding acquisition, Project administration, Resources, Supervision, Writing–review and editing. YA-M: Data curation, Formal Analysis, Methodology, Software, Writing–review and editing. AA-A: Data curation, Formal Analysis, Methodology, Software, Writing–review and editing. HA: Funding acquisition, Resources, Project administration, Supervision, Writing–review and editing. JN: Data curation, Formal Analysis, Methodology, Writing–review and editing. YZ: Formal Analysis, Methodology, Resources, Software, Visualization, Writing–review and editing. HT: Data curation, Formal Analysis, Methodology, Resources, Writing review and editing.
The author(s) declare that no financial support was received for the research, authorship, and/or publication of this article. This research was supported by King Saud University, Riyadh, Saudi Arabia. Researchers Supporting Project number (RSP2024R425).
We would like to extend our gratitude to Mohammed D. Abekah, Douglas A. Arhinkorah, Joseph Yeboah, and Rose Nkasala for their unwavering support throughout the study.
The authors declare that the research was conducted in the absence of any commercial or financial relationships that could be construed as a potential conflict of interest.
All claims expressed in this article are solely those of the authors and do not necessarily represent those of their affiliated organizations, or those of the publisher, the editors and the reviewers. Any product that may be evaluated in this article, or claim that may be made by its manufacturer, is not guaranteed or endorsed by the publisher.
Abdullah, A. (2018). Ghana and her wonderful wetlands - ambassador report - our actions. Tunza eco generation. Available at: https://tunza.eco-generation.org/ambassadorReportView.jsp?viewID=44359 (Accessed September 29, 2021).
Ablo, A. D., and Asamoah, V. K. (2018). Local participation, institutions and land acquisition for energy infrastructure: the case of the Atuabo gas project in Ghana. Energy Res. Soc. Sci. 41 (June 2017), 191–198. doi:10.1016/j.erss.2018.03.022
Ackah, C., Adjasi, C., and Turkson, F. (2014). Scoping study on the evolution of industry in Ghana, 1–37. WIDER Working Paper, 2014/075(1).
Adams, S., Adom, P. K., and Klobodu, E. K. M. (2016). Urbanization, regime type and durability, and environmental degradation in Ghana. Environ. Sci. Pollut. Res. 23 (23), 23825–23839. doi:10.1007/s11356-016-7513-4
Addae, B., and Oppelt, N. (2019). Land-use/land-cover change analysis and urban growth modelling in the greater accra metropolitan area (GAMA), Ghana. Urban Sci. 3 (1), 26. doi:10.3390/urbansci3010026
Alex, E. C., Ramesh, K. V., and Sridevi, H. (2017). Quantification and understanding the observed changes in land cover patterns in Bangalore. Int. J. Civ. Eng. Technol. 8 (4), 597–603.
Allred, B. W., Smith, W. K., Twidwell, D., Haggerty, J. H., Running, S. W., Naugle, D. E., et al. (2015). Ecosystem services lost to oil and gas in North America. Science 348 (6233), 401–402. doi:10.1126/science.aaa4785
Ashiagbor, G., Amoako, C., Asabere, S. B., and Quaye-Ballard, J. A. (2019). Landscape transformations in rapidly developing peri-urban areas of accra, Ghana: results of 30 years. Open Geosci. 11 (1), 172–182. doi:10.1515/geo-2019-0014
Baah, K. A. (2015). Maximization of natural gas liquids Recovery of the Ghana national gas plant-atuabo. Kumasi, Ghana: Kwame Nkrumah University of Science and Technology.
Bernard, E., Armah, F. A., Afrifa, E. K. A., Aheto, D. W., Odoi, J. O., and Afitiri, A. R. (2020). Assessing land use and land cover change in coastal urban wetlands of international importance in Ghana using Intensity Analysis. Wetl. Ecol. Manag. 28 (2), 271–284. doi:10.1007/s11273-020-09712-5
Bolca, M., Turkyilmaz, B., Kurucu, Y., Altinbas, U., Esetlili, M. T., and Gulgun, B. (2007). Determination of impact of urbanization on agricultural land and wetland land use in Balçovas’ Delta by remote sensing and GIS technique. Environ. Monit. Assess. 131 (1–3), 409–419. doi:10.1007/s10661-006-9486-0
Cao, L., and Anthony, D. (2009). Birds and people both depend on China’s wetlands. Nature 460 (7252), 173. doi:10.1038/460173a
Cazenave, A., and Robert, J. N. (2010). Sea-level rise and its impact on coastal zones. J. Coast. Res. 30 (6), 1287–1306. Available at: http://www.jstor.org/stable/40656419.
Choubin, B., Soleimani, F., Pirnia, A., Sajedi-Hosseini, F., Alilou, H., Rahmati, O., et al. (2019). Effects of drought on vegetative cover changes: investigating spatiotemporal patterns. Extreme Hydrology Clim. Var. Monit. Model. Adapt. Mitig. 2, 213–222. doi:10.1016/B978-0-12-815998-9.00017-8
Coastal Resources Center (2013). Managing our coastal wetlands: lessons from the western region. USAID integrated coastal and fisheries governance program for the western region of Ghana. Narragansett RI: Coastal Resources Center, University of Rhode Island.
Cui, L., Li, G., Ouyang, N., Mu, F., Yan, F., Zhang, Y., et al. (2018). Analyzing coastal wetland degradation and its key restoration technologies in the coastal area of Jiangsu, China. Wetlands 38 (3), 525–537. doi:10.1007/s13157-018-0997-6
Diallo, Y., Hu, G., and Wen, X. (2010). Assessment of land use cover changes using ndvi and dem in puer and simao counties, yunnan Province, China. Rep. Opin. 2 (9), 7–16.
Douglas, I. (2017). “Ecosystems and human well-being,” in Encyclopedia of the anthropocene (Elsevier), 185–197. doi:10.1016/B978-0-12-809665-9.09206-5
Drisya, J., KumarD, S., and Thendiyath, R. (2018). “Spatio-temporal variability of soil moisture and drought estimation using a distributed hydrological model,” in Integrating disaster science and management (Elsevier Inc), 451–460. doi:10.1016/B978-0-12-812056-9/00027-0
Ehsan, S., and Kazem, D. (2013). Analysis of land use-land covers changes using normalized difference vegetation index (NDVI) differencing and classification methods. Afr. J. Agric. Res. 8 (37), 4614–4622. doi:10.5897/ajar11.1825
Faisal, A., Hossain, M., Haque, S., Shaunak, M., and Kafy, A. (2019). “Remote sensing approach in wetland and land degradation assessment: a scenario of modhumoti model Town, savar, Bangladesh,” in 1st International Conference on Urban and Regional Planning (Bangladesh: Bangladesh Institute of Planners).
Finlayson, C. M., Horwitz, P., and Weinstein, P. (2015). Wetlands and human health. Wetl. Hum. Health, 1–263. doi:10.1007/978-94-017-9609-5
Ghanadistricts.com (2019). Ghana districts: a repository of all local Assemblies in Ghana. Available at: http://www.Ghanadistricts.Com/Home/LinkData/7188 (Accessed August 22, 2021).
Ghana Statistical Service (2014). 2010 population and housing census, district analytical survey, Ellembelle district. Republic of Ghana: Ministry of Food and Agriculture.
Gibson, J. F., Stein, E. D., Baird, D. J., Max, F. C., Zhang, X., and Hajibabaei, M. (2015). Wetland ecogenomics – the next generation of wetland biodiversity and functional assessment. Wetl. Sci. Pract. 32 (1), 27–32. doi:10.1672/ucrt083-282
Grumbine, R. E. (2014). Assessing environmental security in China. Front. Ecol. Environ. 12 (7), 403–411. doi:10.1890/130147
He, C., Liu, Z., Tian, J., and Ma, Q. (2014a). Urban expansion dynamics and natural habitat loss in China: a multiscale landscape perspective. Glob. Change Biol. 20 (9), 2886–2902. doi:10.1111/gcb.12553
He, Q., Bertness, M. D., Bruno, J. F., Li, B., Chen, G., Coverdale, T. C., et al. (2014b). Economic development and coastal ecosystem change in China. Sci. Rep. 4, 5995–5999. doi:10.1038/srep05995
Hilson, G., and Nyame, F. (2006). Gold mining in Ghana’s forest reserves: a report on the current debate. Area 38 (2), 175–185. doi:10.1111/j.1475-4762.2006.00670.x
Holland, C. C., Honea, J. E., Gwin, S. E., and Kentula, M. E. (1995). Wetland degradation and loss in the rapidly urbanizing area of Portland, Oregon. Wetlands 15 (4), 336–345. doi:10.1007/BF03160888
Huang, S., Tang, L., Hupy, J. P., Wang, Y., and Shao, G. (2021). A commentary review on the use of normalized difference vegetation index (NDVI) in the era of popular remote sensing. J. For. Res. 32 (1), 1–6. doi:10.1007/s11676-020-01155-1
Jafari, N. (2009). Ecological integrity of wetland, their functions and sustainable use. J. Ecol. Nat. Environ. 1 (3), 45–54. Available at: http://www.academicjournals.org/JENE.
Junk, W. J., An, S., Finlayson, C. M., Gopal, B., Květ, J., Mitchell, S. A., et al. (2013). Current state of knowledge regarding the world’s wetlands and their future under global climate change: a synthesis. Aquat. Sci. 75 (1), 151–167. doi:10.1007/s00027-012-0278-z
Kaku, A. (2018). The socio-economic impact of oil and gas discovery in the Ellembelle district of Ghana. Legon, Accra, Ghana: University of Ghana. (Issue 10111447).
Kaku, D. U., Cao, Y., Al-Masnay, Y. A., and Nizeyimana, J. C. (2021). An integrated approach to assess the environmental impacts of large-scale gold mining: the nzema-gold mines in the ellembelle district of Ghana as a case study. Int. J. Environ. Res. Public Health 18 (13), 7044. doi:10.3390/ijerph18137044
Kentula, M. E., Gwin, S. E., and Pierson, S. M. (2004). Tracking changes in wetlands with urbanization: sixteen years of experience in Portland, Oregon, USA. Wetlands 24 (4), 734–743. doi:10.1672/0277-5212(2004)024[0734:TCIWWU]2.0.CO;2
Kuchay, N. A., and Bhat, M. S. (2014). Urban sprawl of srinagar city and its impact on wetlands- A spatio-temporal analysis. Int. J. Environ. Bioenergy 9 (2), 122–129.
Kulawardhana, R. W. (2011). Remote sensing of vegetation: principles, techniques and applications. By Hamlyn G. Jones and Robin A Vaughan. J. Veg. Sci. 22 (6), 1151–1153. doi:10.1111/j.1654-1103.2011.01319.x
Kumi, J., Kumi, M., and Apraku, A. (2015). Threats to the conservation of wetlands in Ghana: the case of songor ramsar site. J. Sci. Res. Rep. 6 (1), 13–25. doi:10.9734/jsrr/2015/13906
Li, F., Wang, L., Chen, Z., Clarke, K. C., Li, M., and Jiang, P. (2018). Extending the SLEUTH model to integrate habitat quality into urban growth simulation. J. Environ. Manag. 217, 486–498. doi:10.1016/j.jenvman.2018.03.109
Li, Y., Shi, Y., Zhu, X., Cao, H., and Yu, T. (2014). Coastal wetland loss and environmental change due to rapid urban expansion in Lianyungang, Jiangsu, China. Reg. Environ. Change 14 (3), 1175–1188. doi:10.1007/s10113-013-0552-1
Lyon, J. G., Yuan, D., Lunetta, R. S., and Elvidge, C. D. (1998a). A change detection experiment using vegetation indices. Photogrammetric Eng. Remote Sens. 64 (2), 143–150.
Lyon, J. G., Yuan, D., Lunetta, R. S., and Elvidge, C. D. (1998b). A change detection experiment using vegetation indices. Photogrammetric engineering and remote sensing. Am. Soc. Photogranometry Remote Sens. 64 (2), 143–150.
Mao, D., Wang, Z., Wu, J., Wu, B., Zeng, Y., Song, K., et al. (2018). China’s wetlands loss to urban expansion. Land Degrad. Dev. 29 (8), 2644–2657. doi:10.1002/ldr.2939
McCauley, L. A., Jenkins, D. G., and Quintana-Ascencio, P. F. (2013). Isolated wetland loss and degradation over two decades in an increasingly urbanized landscape. Wetlands 33 (1), 117–127. doi:10.1007/s13157-012-0357-x
Mcveagh, P., Yule, I., and Grafton, M. (2012). “Pasture yield mapping from your groundspread truck,” in Advanced nutrient management: gains from the past - goals for the future. Editors L. D. Currie, and C. L. Christensen (Florida, USA: Fertilizer and Lime Research Centre). Occasional Re-port No. 25.
Mensah, A. K., Mahiri, I. O., Owusu, O., Mireku, O. D., Wireko, I., and Kissi, E. A. (2015). Environmental impacts of mining: a study of mining communities in Ghana. Appl. Ecol. Environ. Sci. 3 (3), 81–94. doi:10.12691/aees-3-3-3
Mpoano, H. (2018). Initiative to conserve the greater Amanzule wetlands (gaw) launched on world wetland day. Available at: https://www.facebook.com/HenMpoano/photos/initiative-to-conserve-the-greater-amanzule-wetlands-gaw-launched-on-world-wetla/1763046803727427 (Accessed October 17, 2021).
Murray, N. J., Clemens, R. S., Phinn, S. R., Possingham, H. P., and Fuller, R. A. (2014). Tracking the rapid loss of tidal wetlands in the Yellow Sea. Front. Ecol. Environ. 12 (5), 267–272. doi:10.1890/130260
Ochoa-Gaona, S., Kampichler, C., de Jong, B. H. J., Hernndez, S., Geissen, V., and Huerta, E. (2010). A multi-criterion index for the evaluation of local tropical forest conditions in Mexico. For. Ecol. Manag. 260 (5), 618–627. doi:10.1016/j.foreco.2010.05.018
Owusu-Adjapong, E. (2018). Oil and gas production in Ghana blessing or curse. Available at: http://large.stanford.edu/courses/2018/ph240/owusu-adjapong2/.
Owusu-Nimo, F., Mantey, J., Nyarko, K. B., Appiah-Effah, E., and Aubynn, A. (2018). Spatial distribution patterns of illegal artisanal small scale gold mining (Galamsey) operations in Ghana: a focus on the Western Region. Heliyon 4 (2), e00534. doi:10.1016/j.heliyon.2018.e00534
Ramsar Convention Secretariat (2013). The ramsar convention manual: a guide to the convention on wetlands. 6th edition. Ramsar, Iran: Ramsar Convention Bureau, 109.
Sallustio, L., Toni, A. D., Strollo, A., Di, M., Gissi, E., Vizzarri, M., et al. (2017). Assessing habitat quality in relation to the spatial distribution of protected areas in Italy. J. Environ. Manag. 201, 129–137. doi:10.1016/j.jenvman.2017.06.031
Sharp, R., Douglass, J., Wolny, S., Arkema, K., Bernhardt, J., Bierbower, W., et al. (2020). “InVEST user ’ s guide,” in The natural capital project. Editors R. Sharp, J. Douglass, and S. Wolny (Stanford University, University of Minnesota, The Nature Conservancy, and World Wildlife Fund).
Shuangao, W., Padmanaban, R., Mbanze, A. A., Silva, J. M. N., Shamsudeen, M., Cabral, P., et al. (2021). Using satellite image fusion to evaluate the impact of land use changes on ecosystem services and their economic values. Remote Sens. 13 (5), 851. doi:10.3390/rs13050851
Sun, X., Jiang, Z., Liu, F., and Zhang, D. (2019). Monitoring spatio-temporal dynamics of habitat quality in Nansihu Lake basin, eastern China, from 1980 to 2015. Ecol. Indic. 102 (March), 716–723. doi:10.1016/j.ecolind.2019.03.041
Tao, S., Fang, J., Zhao, X., Zhao, S., Shen, H., Hu, H., et al. (2015). Rapid loss of lakes on the Mongolian Plateau. Proc. Natl. Acad. Sci. U. S. A. 112 (7), 2281–2286. doi:10.1073/pnas.1411748112
Terrado, M., Sabater, S., Chaplin-Kramer, B., Mandle, L., Ziv, G., and Acuña, V. (2016). Model development for the assessment of terrestrial and aquatic habitat quality in conservation planning. Sci. Total Environ. 540, 63–70. doi:10.1016/j.scitotenv.2015.03.064
Tian, Y., Luo, L., Mao, D., Wang, Z., Li, L., and Liang, J. (2017). Using Landsat images to quantify different human threats to the Shuangtai Estuary Ramsar site, China. Ocean Coast. Manag. 135, 56–64. doi:10.1016/j.ocecoaman.2016.11.011
Uerbach, N. A. A. A., Ulloch, A. Y. I. T. T., and Possingham, H. P. (2014). Informed actions: where to cost effectively manage multiple threats to species to maximize return on investment. Ecol. Appl. 24 (6), 1357–1373. doi:10.1890/13-0711.1
United State Geological Survey (2021). EarthExplorer. Available at: http://earthexplorer.usgs.gov (Accessed October 22, 2021).
Wang, X., Yan, F., and Su, F. (2020). Impacts of urbanization on the ecosystem services in the Guangdong-Hong Kong-Macao greater bay area, China. Remote Sens. 12 (19), 3269. doi:10.3390/rs12193269
Wu, C. F., Lin, Y. P., Chiang, L. C., and Huang, T. (2014). Assessing highway’s impacts on landscape patterns and ecosystem services: a case study in Puli Township, Taiwan. Landsc. Urban Plan. 128, 60–71. doi:10.1016/j.landurbplan.2014.04.020
Wu, Y., and Ke, Y. (2016). Landslide susceptibility zonation using GIS and evidential belief function model. Arabian J. Geosciences 9 (17), 697. doi:10.1007/s12517-016-2722-1
Xu, L., Chen, S. S., Xu, Y., Li, G., and Su, W. (2019). Impacts of land-use change on habitat quality during 1985-2015 in the Taihu Lake Basin. Sustain. Switz. 11 (13), 3513. doi:10.3390/su11133513
Yang, X., and Pang, J. (2006). Implementing China ’ s “ water agenda 21 ” in a nutshell. Front. Ecol. Environ. 2. doi:10.1890/1540-9295(2006)004[0362:ICWA]2.0.CO;2
Yevugah, L. L., Osei, E. M., Ayer, J., and Osei, J. (2017). Spatial mapping of carbon stock in riverine mangroves along Amanzule River in the ellembelle district of Ghana. Earth Sci. Res. 6 (1), 120. doi:10.5539/esr.v6n1p120
Zaitunah, A., Samsuri, S., Ahmad, A. G., and Safitri, R. A. (2018). Normalized difference vegetation index (ndvi) analysis for land cover types using landsat 8 oli in besitang watershed, Indonesia. IOP Conf. Ser. Earth Environ. Sci. 126 (1), 012112. doi:10.1088/1755-1315/126/1/012112
Zhai, T., Wang, J., Fang, Y., Qin, Y., Huang, L., and Chen, Y. (2020). Assessing ecological risks caused by human activities in rapid urbanization coastal areas: towards an integrated approach to determining key areas of terrestrial-oceanic ecosystems preservation and restoration. Sci. Total Environ. 708, 135153. doi:10.1016/j.scitotenv.2019.135153
Keywords: wetland loss, GIS and remote sensing, land-use land-cover, normalization difference vegetation index, industrialization, invest-habitat quality model
Citation: Dawuda UK, Yonghong C, Al-Masnay YA, Al-Aizari AR, Alzahrani H, Nizeyimana JC, Zhang Y and Tong H (2024) Assessment of wetland loss through industrialization and community expansion: a case study of the greater amanzule wetland in the Ellembelle district of Ghana. Front. Environ. Sci. 12:1451394. doi: 10.3389/fenvs.2024.1451394
Received: 20 June 2024; Accepted: 25 September 2024;
Published: 05 November 2024.
Edited by:
Teresa Ferreira, University of Lisbon, PortugalReviewed by:
Stamatis Zogaris, Hellenic Centre for Marine Research (HCMR), GreeceCopyright © 2024 Dawuda, Yonghong, Al-Masnay, Al-Aizari, Alzahrani, Nizeyimana, Zhang and Tong. This is an open-access article distributed under the terms of the Creative Commons Attribution License (CC BY). The use, distribution or reproduction in other forums is permitted, provided the original author(s) and the copyright owner(s) are credited and that the original publication in this journal is cited, in accordance with accepted academic practice. No use, distribution or reproduction is permitted which does not comply with these terms.
*Correspondence: Hassan Alzahrani, aHNhbkBLU1UuRURVLlNB
Disclaimer: All claims expressed in this article are solely those of the authors and do not necessarily represent those of their affiliated organizations, or those of the publisher, the editors and the reviewers. Any product that may be evaluated in this article or claim that may be made by its manufacturer is not guaranteed or endorsed by the publisher.
Research integrity at Frontiers
Learn more about the work of our research integrity team to safeguard the quality of each article we publish.