- 1Division of Environmental Health Sciences, College of Public Health, The Ohio State University, Columbus, OH, United States
- 2Department of Veterinary Preventive Medicine, The Ohio State University, Columbus, OH, United States
- 3Infectious Diseases Institute, The Ohio State University, Columbus, OH, United States
- 4Department of Food Science and Technology, The Ohio State University, Columbus, OH, United States
The dissemination of antibiotic resistance (AR) through various environments and the role of AR hotspots in public health crises are gaining increasing attention. Aquatic biofilms are speculated to play a significant role in AR spread due to their collection of diverse microorganisms and facilitation of horizontal gene transfer (HGT). However, few studies have characterized the AR genes (resistome) present in natural river biofilms. The goal of this study was to use MinION long-read sequencing to analyze the microbiome, resistome, and mobile genetic elements (MGEs) in periphyton (epilithic biofilms) (n = 56) from a multiuse watershed in Ohio, to elucidate the role of periphyton in clinically relevant AR. Key members of the periphyton microbiome included Flavobacterium and Aeromonas. Overall, periphyton microbial communities shifted with season and location. Specifically, species of Porphyrobacter and Cyanobacteria were more abundant in biofilms during the summer season. Potentially pathogenic bacteria, including the family Enterobacteriaceae, the fish pathogen Pseudomonas koreensis, and the human pathogen Shigella flexneri, were more abundant in sites downstream of the large city, Columbus, OH, than upstream. The periphyton resistome carried diverse AR genes for a variety of classes, but had minimal clinical relevance. Escherichia, Escherichia coli, and Muvirus were common hosts of AR genes (ARGs) and MGEs. Pseudomonas and Cyanobacteria were frequently MGE hosts, but not AR genes, indicating the potentially important role of these taxa in HGT within and around biofilms. While the sequencing depth in this study was relatively shallow, these findings highlight the mobility potential for the transmission of ARGs in river biofilms.
1 Introduction
Antibiotic resistance (AR) is a longstanding and widespread health concern, and its modern escalation is attributed to human activities (Finley et al., 2013). Specifically, the historical overuse, misuse, and regular use of antibiotics in medicine and agriculture have promoted the spread and selection of antibiotic resistance genes (ARGs), which enable bacteria to evade the therapeutic effects of antibiotics (Davies and Davies, 2010). This selective pressure encourages the transfer of ARGs among bacteria through horizontal gene transfer (HGT), facilitated by mobile genetic elements (MGEs) (Frost et al., 2005). In this way, ARGs are unique biological contaminants because they do not necessarily dissipate with distance from the pollution source, and they can propagate in the environment and spread between bacteria (Abe et al., 2020). Fecal contamination from sources like wastewater treatment plant (WWTP) effluent discharge and agricultural runoff is a major source of ARGs, AR bacteria (ARB), and antibiotics to the environment (Larsson and Flach, 2021; Yin et al., 2023). While the environment is increasingly recognized as a potential source of ARG evolution and transmission, its role in clinical AR remains poorly understood (Larsson and Flach, 2021).
Biofilms are hotspots of AR transmission and acquisition in the environment due to their high levels of HGT, unique structure, and ability to accumulate pollutants, such as antibiotics (Balcázar et al., 2015; Abe et al., 2020; Sentenac et al., 2021). The focus of this study, periphyton, which encompass various types of freshwater biofilms, are complex communities of microorganisms, including bacteria, algae, fungi, protozoa, and viruses surrounded by in extracellular polymeric substances (EPS) (Fernandes et al., 2020; Zhao et al., 2022). Periphyton attached to stones or other stationary surfaces are known as epilithic biofilms (Fernandes et al., 2020; Sentenac et al., 2021; Zhao et al., 2022). Compared to other aquatic matrices, including water and sediments, environmental biofilms have been found to harbor higher levels of ARGs (Reichart et al., 2021; Mills et al., 2022). Periphyton play a crucial role in freshwater ecosystems, as they significantly contribute to primary production and oxygen levels, are a key player in nitrogen and phosphorous cycling, and serve as a vital food source for aquatic animals, such as crayfish and fish (Zhao et al., 2022). Their role in nutrient cycling, particularly the removal of nitrogen and phosphorous from water, can mitigate eutrophication and combat some of the adverse effects of climate change (Zhao et al., 2022). Finally, because of periphyton’s strong responses to environmental changes and its abundance in freshwater, they are a valuable indicator of environmental quality (Zhao et al., 2022) and impact. Analyzing epilithic biofilms provides the ability to assess contamination over a longer time frame compared to more traditional monitoring matrices, like surface water, because biofilms have greater stability over time (Fernandes et al., 2020; Sentenac et al., 2021). Studying the microorganisms and ARGs in aquatic biofilms provides the opportunity to elucidate the role of these unique microbial communities in AR.
This study aimed to characterize the microbiome and resistome (collection of ARGs) of periphyton collected from various sites over a one-year period from a mixed-use river watershed in central Ohio (OH), United States. The objective was to classify the ARGs and MGEs in periphyton, as well as their microbial hosts, using long-read sequencing technology, which has not frequently been used in environmental microbiome or resistome studies. Ultimately, the purpose of this study was to use the resistome and MGE data to evaluate the clinical relevance and transmissibility of ARGs in aquatic biofilms. Previous studies analyzing the microbiome of natural river biofilms have typically used 16S rRNA gene sequencing (Zeglin, 2015; Guo et al., 2021; Tamminen et al., 2022; Bagra et al., 2023). One meta-analysis previously compiled and analyzed shotgun sequencing data to annotate the freshwater biofilm resistome, including biofilms from rivers (Yao et al., 2022). This study used publicly available metagenomic data to catalog ARGs in freshwater biofilms on a large scale. They found that the sampling location and relative human footprint (human pressure) influenced the freshwater biofilm resistome. They annotated ARGs and ARG hosts, like our study, but the scale was much larger, encompassing four countries, and they used all short read data (Yao et al., 2022). To our knowledge, the present study is the first example of metagenomic MinION long-read sequencing of freshwater periphyton communities. Our primary focus was to elucidate the potential role of periphyton in clinical AR. Our previous analysis of these samples using droplet digital PCR revealed high concentrations of clinically relevant carbapenem resistance genes (blaKPC, blaNDM, blaOXA-48) in periphyton compared to river water, sediment, and detritus (Mills et al., 2022). This subsequent study provides a comprehensive profile of the entire microbiome and resistome of periphyton from a microbial ecology perspective, examining spatial and temporal differences in this matrix.
2 Materials and methods
2.1 Sample collection
Periphyton samples were collected from 26 sites along the Scioto River watershed in OH United States, including the Scioto River, Olentangy River, and Big Darby Creek (Supplementary Figure S1). The collection methods and study sites are previously described in Mills et al. (2022). Briefly, the Scioto River watershed drains 6,513 square miles, with the northern part of the watershed being composed of mostly agricultural lands, and the large urban center, Columbus, OH, which is the largest city in the state (Ohio Environmental Protection Agency (EPA), 2014). The southern half of the watershed is mostly forests with some agricultural land (Ohio Environmental Protection Agency (EPA), 2014). Therefore, these rivers flow through many different sources of contamination. Periphyton samples were collected between October 2017 and August 2018 in four visits/seasons. The “Autumn” samples were collected between October and December 2017, “Winter” between February and March 2018, “Spring” between April and May 2018, and “Summer” between July and August 2018. Periphyton samples were collected by brushing the surface of large, submerged sediments or stones onto a tray. The detached periphyton were rinsed into an opaque plastic jar (Kautza and Sullivan, 2016). Samples were collected from near the right bank, left bank, and middle of the river for each site. Samples were transported to the lab on ice and stored at 4°C prior to DNA extraction.
2.2 DNA extraction and long-read sequencing
Periphyton DNA was extracted from 0.25 g of wet weight per sample with the DNeasy PowerSoil Kit (Qiagen, Valencia, California (CA), United States). DNA concentration was measured with the Qubit 3 Fluorometer (ThermoFisher Scientific, Waltham, Massachusetts (MA), United States). DNA quality was measured with the NanoDropTM 2000 Spectrophotometer (ThermoFisher Scientific). Periphyton DNA samples that were low in concentration, or outside the desired range of quality scores (OD260/280 = 1.8–2.0; OD260/230 = 2.0–2.2) were cleaned and concentrated with a basic ethanol precipitation protocol. The protocol is presented in Mills et al. (2023). In total, 56 periphyton samples were adequate quality and concentration for long-read metagenomic sequencing.
DNA samples were sequenced on Oxford Nanopore Technology’s (ONT) long-read MinION sequencer (ONT, Oxford, England) using Flongle flow cells (FLO-FLG001 R9.4.1). DNA libraries were prepared and barcoded with the Rapid Barcoding Kit (SQK-RBK004, ONT), following the ONT instructions for the kit, which are available on the ONT website (https://community.nanoporetech.com/). This library prep kit with the Flongle flow cells allows multiplexing of up to 12 samples with inputs of 200 ng of DNA per sample. While the input for sequencing is low, the combination of these methods allowed for low-cost analysis of many samples. This was selected for this study, given the goals of analyzing many samples at a shallower sequencing depth, and these methods and workflow had been used successfully in prior study of fecal samples to achieve similar goals (Mills et al., 2023). Sequencing was run for 24 h or until the number of reads plateaued.
2.3 Long-read sequencing bioinformatics
Raw data were basecalled and demultiplexed using Guppy (v 3.2.4). Taxonomy were annotated with What’s In My Pot (WIMP) on the EPI2ME platform (2020.05.19). TaxonKit was used to pair National Center for Biotechnology Information (NCBI) taxonomy IDs with the full taxonomy (Shen and Ren, 2021). Microbiome compositions are presented as relative abundances (RA), specifically, the number of reads annotated as a specific taxonomy divided by the total number of microbially, taxonomically annotated reads. ARGs, MGEs, and hosts were annotated with NanoARG (Arango-Argoty et al., 2019). ARG and MGE abundance are reported as read counts (gene hits) and normalized ARG abundance, based on the library size of each sample, reported in average gene counts/gigabase pair (gbp) (Ma et al., 2016). NanoARG also denotes which ARG hosts are putative pathogens, based on sequence similarity to the World Health Organization’s list of pathogens of concern for ARGs and the ESKAPE database of pathogens with multidrug resistance (Arango-Argoty et al., 2019). These annotations are reported as “potential” pathogenic hosts, given the metagenomic nature of this analysis, since higher resolution methods would be required to confirm the definite presence of pathogens (Arango-Argoty et al., 2019). Sequencing depth and library size (taxonomic annotated reads) can be found in the Supplementary Material (Supplementary Table S1). Sequencing data and metadata can be found at the NCBI Sequence Read Archive (SRA) under the accession number PRJNA1116137 (http://www.ncbi.nlm.nih.gov/bioproject/1116137).
2.4 Statistical analyses
Statistical analyses were completed in R (v 3.6.2). Periphyton microbiome data were filtered and normalized before diversity and differential abundance analyses. Only taxa classified to at least a Superkingdom level were retained in the microbiome data, and non-microbial eukaryotic reads were removed. Following this filtering, samples with libraries <100 reads were removed from the dataset prior to normalizing, which resulted in a total of 47 periphyton samples for diversity analysis (Supplementary Table S1). Prior to normalizing, the average library size from the Flongle flow cells was 609 classified, taxonomic reads, with a minimum of 120 reads and a maximum of 3,345 in a single sample. Microbiome data were normalized by scaling each sample to the median library size. Due to the wide variety in library sizes and overall shallow sequencing depth, this method was selected to preserve as much of the dataset as possible (McMurdie and Holmes, 2013; Weiss et al., 2017). This resulted in a total of 3,117 unique taxa and a library size of 385 reads/sample. Resistome and MGE data were analyzed as normalized ARG abundance (ARG counts/gbp). There were no significant Spearman or Pearson correlations between sequencing depth (bp) and Shannon index (p > 0.05), indicating that although library sizes were small, differences in the microbiome were not attributed to sequencing depth. A community-style analysis was not included for the periphyton resistome or mobilome (MGE) data, given the limited annotations of functional genes at this sequencing depth (Supplementary Table S1). Furthermore, ARG abundance was dependent on sequencing depth, even with normalization (p < 0.05), limiting the statistical reliability of the analysis.
The overall goals of the statistical testing were to identify differences in the periphyton microbiome or resistome with season or location of sample collection. Alpha and beta diversity of the microbiome were calculated using the phyloseq (McMurdie and Holmes, 2013) and vegan (Oksanen et al., 2020) packages. Shannon index was calculated for alpha diversity. Normality was tested for Shannon index with Q-Q plots and Shapiro-Wilk tests. The Levene test was used to assess homogeneity of variance. Shannon index was normally distributed with equal variance between groups. Therefore, because these data fit the assumptions of the test, differences in Shannon index were tested with a one-way ANOVA by season and location. For season, only winter, spring, and summer samples were included in statistical testing because of the small number of samples collected from autumn (n = 2). Furthermore, for location in the watershed (way upstream, upstream, downstream, way downstream), samples from Canoe Livery/Trapper John’s were excluded since these samples (n = 2) were the only taken from Big Darby Creek (Supplementary Table S1; Supplementary Figure S1). We also found that in our prior analysis (Mills et al., 2022), this site was heavily contaminated with carbapenem ARGs, likely due to its role in recreation as a canoe livery. These samples were treated as outliers and were thus removed from the statistical testing by location. In order to test microbiome alpha diversity by both location and season, a two-way ANOVA was run.
Community composition and structure were calculated with the beta diversity dissimilarity matrix, Bray Curtis, and plotted with Non-Metric Multidimensional Scaling (NMDS), which produces ordination plots that present the differences between each sample’s microbial communities by distance. Differences beta diversity were tested with Permutational Multivariate Analysis of Variance (PERMANOVA) (adonis2) tests from the vegan package (Oksanen et al., 2020), followed with pairwise adonis, to determine if microbial communities significantly differ in composition/structure by season or location. Both season and location were tested individually, then a single model was created to test both season and location simultaneously. Similar to alpha diversity statistical testing, samples collected in Autumn and from Canoe Livery/Trapper John’s were removed from comparisons made between season and location, respectively. Significance was assessed at p < 0.05 for alpha and beta diversity statistical tests.
Differences in periphyton microbiome composition (RA) were tested via Microbiome Multivariable Associations with Linear Models 2 (MaAsLin2) (Mallick et al., 2021) at a phyla, family, and species level of taxonomy to determine microorganisms that were increased in abundance in the periphyton microbiome with season or location. Models were created with both location and season as fixed effects. Only the samples included in the beta diversity testing were included in differential abundance testing. Taxa were included with a minimum RA of 0.001 and prevalence of 0.2. Chord diagrams for the functional gene host results were created in R, using the circlize package (Gu et al., 2014).
3 Results
3.1 Aquatic biofilm microbiome diversity and composition
Long-read sequencing generated an average of 4,432 reads/sample, with a minimum of 239 reads and a maximum of 20,428 reads in a single sample (Supplementary Table S1). The average sequencing depth was 2,503,173 basepairs (bp)/sample, with the smallest sample containing 1,372 bp and the largest containing 16,566,315 bp. A total of 3,116 unique taxa were identified in the 56 periphyton microbiomes in this study. This included Viruses, Fungi, Bacteria, and Archaea. At a phyla level, an average of 68.2% of the periphyton microbial community was Proteobacteria, followed by 8.70% Actinobacteria, 8.59% Cyanobacteria, 7.83% Bacteroidetes, and 2.61% Firmicutes (Figure 1C; Supplementary Figure S2). All other phyla were less than 1% of the microbiome. The ten genera with the highest RA (average) in all periphyton microbiomes were Flavobacterium (2.95%), Aeromonas (2.06%), Chondrocystis (1.89%), Escherichia (1.87%), Acinetobacter (1.25%), Burkholderia (0.68%), Bradyrhizobium (0.63%), Brevundimonas (0.56%), Acidovorax (0.53%), and Arcobacter (0.45%) (Table 1).
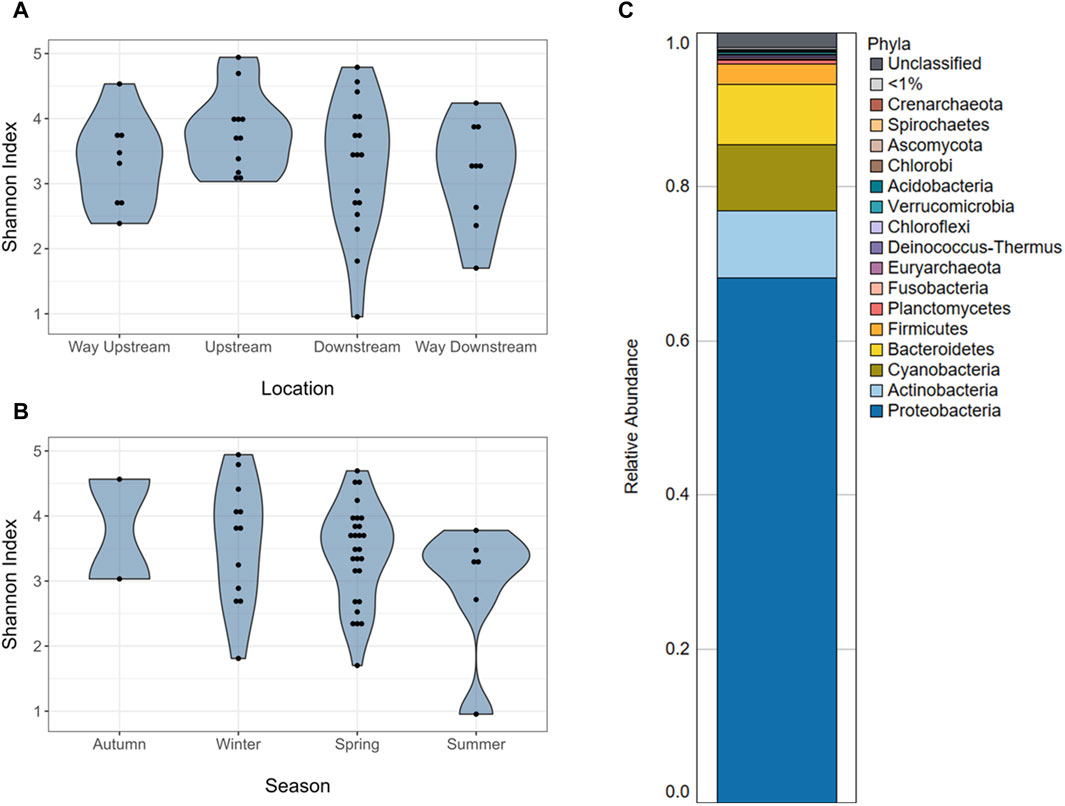
Figure 1. Microbiome alpha diversity and composition of periphyton samples. (A) Alpha diversity (Shannon index) of the microbiomes of periphyton samples by location in the watershed. (B) Alpha diversity (Shannon index) of the microbiomes of periphyton samples by season. (C) Average composition of periphyton microbiomes shown as a relative abundance at a phyla level.
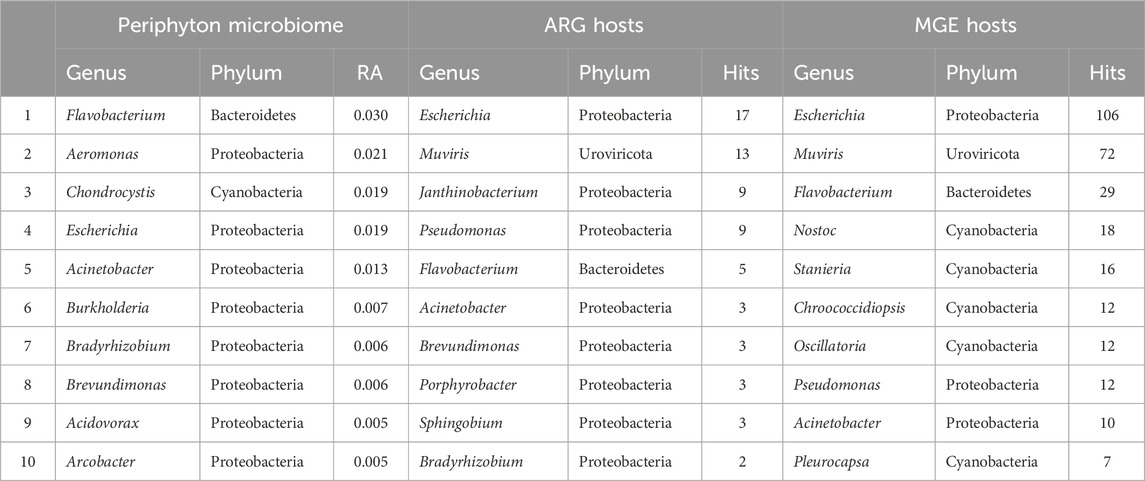
Table 1. The top ten most abundant genera in the microbiome, hosting antibiotic resistance genes, and hosting mobile genetic elements in periphyton. Genera in the microbiome are shown as a relative abundance (RA) and gene hosts are shown as the total number of hits (annotations) in all samples.
There were no differences in alpha diversity (Shannon index) of periphyton microbiome by season (winter, spring, summer) or sampling location (way upstream, upstream, downstream, way downstream) (p > 0.05; Figures 1A, B). Differences were tested by both season and location separately in one-way ANOVA analyses and together in a two-way ANOVA, and neither revealed significant differences (p > 0.05). However, there were differences in beta diversity of periphyton microbiomes with season and location. There were differences with season when tested via PERMANOVA alone (p = 0.037; Figure 2A), but there were no differences in location when tested alone (p = 0.156; Figure 2B). However, when both season and location were tested together via PERMANOVA, both were significant in structuring the biofilm microbiome. Specifically, when controlling for season (p = 0.040), location was also significant (p = 0.021). The interaction term of Season*Location was included in a PERMANOVA model, but it was not significant (p > 0.05). Pairwise tests (adonis2) revealed a significant difference between winter and summer microbiomes (p = 0.01), but there were no other significant pairwise comparisons (p > 0.05). No pairwise comparisons by location were significant (p > 0.05), however the smallest p-values were between way upstream and downstream (p = 0.075) as well as way upstream and upstream (p = 0.089).
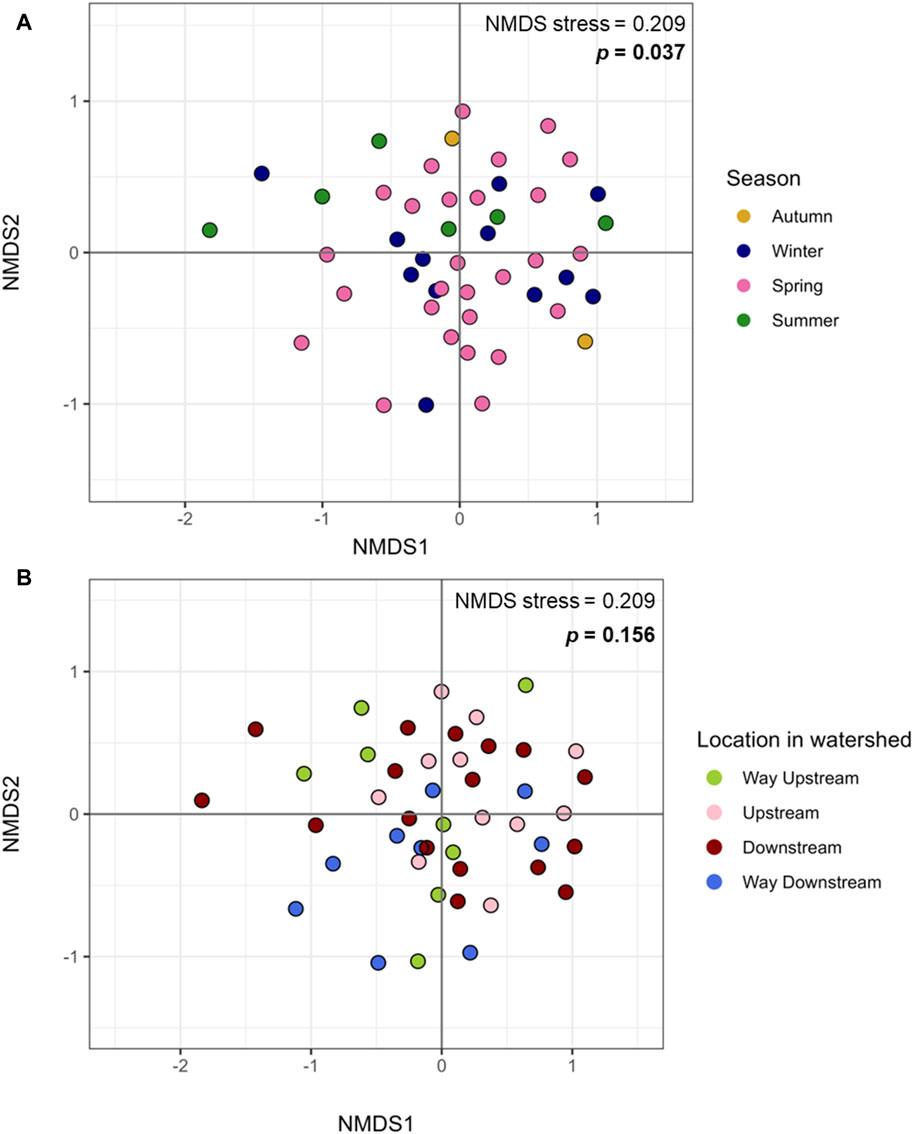
Figure 2. Microbiome beta diversity of periphyton samples. (A) Beta diversity of periphyton microbiomes by season, plotted with Bray Curtis dissimilarity and Non-Metric Multidimensional Sealing (NMDS).(B) Beta diversity of periphyton microbiomes by location in the watershed, plotted with Bray Curtis dissimilarity and NMDS.
Because both season and location were significant factors structuring the periphyton microbiome, differences in taxonomy were tested by these factors. There were no differentially abundant phyla by season or location in the periphyton microbiomes, as tested by MaAsLin2. By season, the family Phyllobacteriaceae was more abundant in winter than spring and summer (Supplementary Table S3). Cellulomonadaceae and Nocardiodaceae were more abundant in winter than summer. At a species level, Mesorhizobium loti was more abundant in winter than summer (Supplementary Table S5). Porphyrobacter sp. LM 6 and Leptolyngbya boryana were more abundant in summer than winter or spring. Porphyrobacter sp. YT40 and Porphyrobacter neustonensis were more abundant in summer than spring. Finally, Aeromonas salmonicida was more abundant in spring than winter.
By location, the families Rhizobiaceae and Sphingomonadaceae were more abundant in the way upstream and upstream sites than way downstream sites (Supplementary Table S4). Enterobacteriaceae was more abundant in the downstream compared to the way upstream sites. At a species level, Polaromonas sp. Pch P was more abundant in upstream sites than any other sites (Supplementary Table S6). Citromicrobium sp. JL477 was more abundant in upstream than downstream sites, and Tabrizicola piscis was more abundant in upstream sites than way downstream sites. Shigella flexneri was more abundant in the downstream sites than the way upstream sites. Finally, Pseudomonas koreensis was more abundant in the way downstream sites than the upstream or downstream sites.
3.2 Periphyton resistome, mobilome, and microbial hosts
In total, 88 unique ARGs were annotated in a total of 35 periphyton samples from 17 antibiotic classes. Two hundred sixteen ARGs were annotated in all biofilm samples, with an average of 6 ARGs/sample. Twenty-one samples had zero ARGs annotated, and the most ARGs annotated in a single sample was 39 (sample P43; Supplementary Table S1). Most of the ARGs identified in periphyton samples were for multidrug resistance, which are ARGs offering resistance to multiple classes of antibiotics (39.8%), followed by glycopeptides (10.6%), bacitracins (7.41%), beta-lactams (7.41%), tetracyclines (6.48%), macrolides, lincosamides, and streptogramines (MLS) (5.56%), and aminoglycosides (5.09%) (Supplementary Figure S3). The five most frequently annotated ARGs were ompR (9.26%), vanR (6.02%), bcrA (5.56%), rpoB2 (5.56%), truncated putative response regulator ArlR (5.09%), and mtrA (3.70%) (Supplementary Table S7).
For the 216 reads with an ARG annotated, 62.9% also had a microbial host annotated. ARG hosts were identified from the phyla Proteobacteria (64.7%), Urovirocota (9.56%), Bacteroidetes (8.82%), Actinobacteria (7.35%), Cyanobacteria (5.88%), Firmicutes (2.94%), and Euryarchaeota (0.74%) (Figure 3A). At a genus level, the most abundant ARG hosts were Escherichia (17.6%), Muvirus (9.56%), Janthinobacterium (6.62%), and Pseudomonas (6.62%) (Table 1). The remaining genera were all less than 5% of the ARGs annotated. Some ARG host taxa were classified to a species or even strain level, which included Escherichia coli (17.6%) as the most abundant ARG host (Table 2). One ARG host species was classified as potentially pathogenic, Acinetobacter baumannii, which hosted the multidrug resistant gene, ompR.
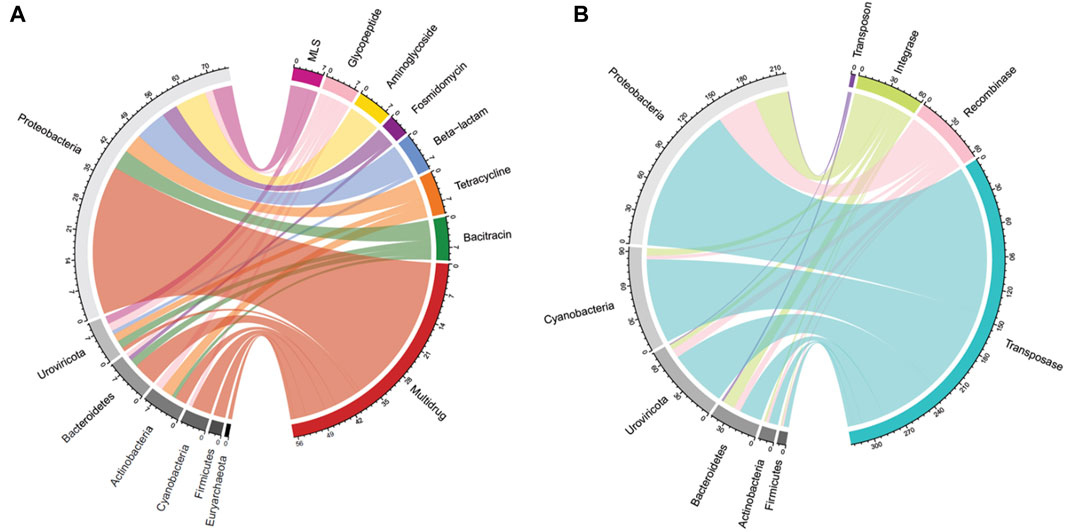
Figure 3. Periphyton antibiotic resistance gene and mobile genetic element host phyla. (A) A chord diagram of the top seven most abundant antibiotic resistance gene classes and their corresponding host phyla, shown as total antibiotic resistance gene hits. (B) A chord diagram of the types of mobile genetic elements and their corresponding top six host phyla, shown as total mobile genetic element gene hits.
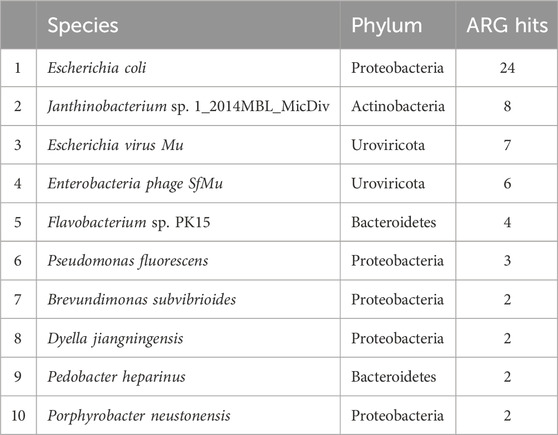
Table 2. The top ten most abundant species hosting antibiotic resistance genes in periphyton samples, shown as total gene hits in all samples.
In total, 790 unique MGEs were annotated in the 46 periphyton samples, with an average 21 MGEs/sample. The majority of the MGEs were transposases (74.3%), followed in much lower abundance by integrases (12.7%), recombinases (12.2%), transposons (0.62%), and integrons (0.21%) (Supplementary Figure S3). Out of the MGEs annotated, 47.4% were associated with a host. Among these microbial hosts, the top six most abundant phyla were Proteobacteria (48.0%), Cyanobacteria (20.6%), Uroviricota (15.8%), Bacteroidetes (9.43%), Actinobacteria (3.07%), and Firmicutes (1.75%) (Figure 3B). At a genus level, the most abundant MGE hosts were Escherichia (23.2%), Muvirus (15.8%), and Flavobacterium (6.36%) (Table 1). The remaining genera hosting MGEs were less common, comprising less than 5% of all the MGE hosts. The most abundant species hosting MGEs was E. coli (Table 3). Three species hosting MGEs were identified as potentially pathogenic, all of which contained transposases (IS630 family transposase, IS481 family transposase, IS5/IS1182 family transposase). These species were Enterococcus faecium, Klebsiella pneumoniae, and Pseudomonas aeruginosa, respectively.
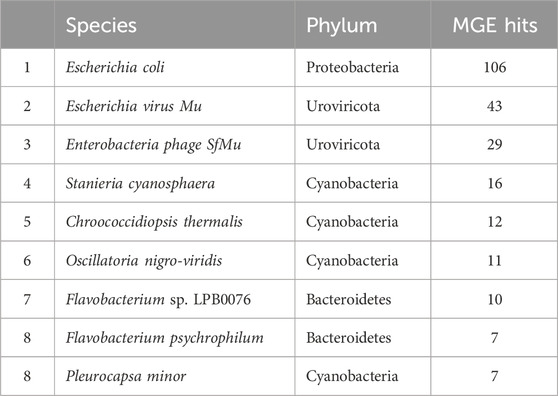
Table 3. The top ten most abundant species hosting mobile genetic elements in periphyton samples, shown as total gene hits in all samples.
Five reads within three different periphyton samples had both ARGs and MGEs annotated (Supplementary Table S8). The first read, identified as Pseudomonas syringae, contained the aminoglycoside ARG, PvrR, and an IS4 family transposase. The ARG PBP-1A for beta-lactam resistance was found on the same read as a transposase and was identified as Janthinobacterium sp. 1_2014MBL_MicDiv. The ARG vanS for glycopeptide resistance was found on the same read as three MGEs, all transposases, in an undefined host. A read carrying vanB, another glycopeptide ARG, carried a site-specific integrase for Cyanothece sp. PCC 7822 in undefined species. Finally, one read identified as a synthetic construct, which is a classification in NCBI for DNA fragments like oligonucleotides and primers, but for this study we would consider an unclassified host, contained both the multidrug ARG, smeS, and a transposase MGE (IS4/IS5 family transposase).
4 Discussion
4.1 Long-read sequencing limitations
Long-read sequencing, such as ONT’s MinION sequencing, holds much promise for the future of microbiome and resistome analysis (Boolchandani et al., 2019). While short read shotgun metagenomic sequencing requires algorithms for assembly to form longer reads for annotation, long-read sequencing does not. This limits some of the bias introduced from assembly algorithms, and it can result in obtaining results more quickly because assembly steps can be simplified (Boolchandani et al., 2019; Kamathewatta et al., 2019). Longer reads also give genetic context for the other functional genes, such as MGEs, and potential host information (Kamathewatta et al., 2019), which is extremely beneficial in studies of ARGs. A concern with long-read sequencing has been the higher error rates than short read sequencing, such as Illumina sequencing, however improvements in basecalling algorithms have improved sequencing accuracy (Logsdon et al., 2020).
In this analysis, we used small flow cells with low DNA inputs (“Flongle”), which others have referred to as “miniature” flow cells that can attain shallow sequencing depth (Mock et al., 2023; Viehweger et al., 2023). While the goal was to sequence many samples at a lower depth, our study revealed limitations with multiplexing ONT’s MinION sequencing on a Flongle flow cell, particularly when using environmental samples. Specifically, we removed nine samples from the microbiome diversity analysis due to limited annotated reads. Although normalization showed no association between diversity and sequencing depth, the library size of samples following normalization (385 reads/sample) was low. More serious limitations were present in the resistome analysis, as the shallow sequencing depth limited ARG annotations (Supplementary Table S1), meaning that a community-style ARG diversity or abundance analysis could not be completed.
Our prior study utilizing these methods of multiplexing on Flongle flow cells used fecal DNA samples (Mills et al., 2023). Using these methods to sequence fecal samples resulted in a greater proportion of reads having classified taxonomy, with an average of 1,018/3,078 reads/sample (33.1%), compared to the average of 609/4,433 reads/sample (13.7%) with annotated taxonomy from the biofilm samples. Environmental DNA sequencing can have considerable challenges because of the variable and often low biomass of environmental DNA (Harper et al., 2019) and limitations in databases for reference data to many environmental microbial taxa (Perry et al., 2022). While the current study provides a snapshot of biofilm samples from a mixed-use watershed with a relatively shallow sequencing depth, future studies should use sequencing strategies with greater depth per sample, particularly when analyzing complex environmental samples. Specifically, we advise that individuals interested in annotating functional genes should not multiplex on ONT Flongle flow cells. These “mini” flow cells are ideal for quality testing before a larger experiment, as has recently been updated as the recommendation on ONT’s website (https://nanoporetech.com/products/sequence/flongle). Other researchers have found success in using Flongle flow cells for adaptive sequencing (Viehweger et al., 2023; Wrenn and Drown, 2023), which is a targeted sequencing approach where nanopores only sequence regions of interest (Martin et al., 2022). This may be a beneficial use for Flongle flow cells in future to achieve sequencing of targeted regions, such as ARGs, while taking advantage of these flow cell’s low cost.
4.2 Periphyton microbiome shifts with location and season
Although prior studies of natural, freshwater periphyton microbiomes used 16S rRNA gene sequencing, the major phyla identified via long-read sequencing were similar, including Proteobacteria, Bacteroidetes, Actinobacteria, Firmicutes, and Cyanobacteria (Zeglin, 2015; Guo et al., 2021; Tamminen et al., 2022; Bagra et al., 2023) (Figure 1C; Supplementary Figure S2). Most prior studies do not report the microbial community composition deeper than the phyla level of taxonomy (Zeglin, 2015; Guo et al., 2021; Tamminen et al., 2022). However, one prior study reported the composition of the natural biofilms from two rivers in Saxony, Germany, at a genus level (Bagra et al., 2023). Similar to the present study, one of the most abundant genera was Flavobacterium, which composed ∼3% of both the periphyton microbiomes from Germany and the Ohio biofilms analyzed in the present study (Bagra et al., 2023) (Table 1). Flavobacterium are known biofilm formers (Jo et al., 2016). Furthermore, the species F. psychrophilum, F. columnare, and F. branchiophilum, are also known fish pathogens (Wahli and Madsen, 2018). These three species were identified in the periphyton microbiomes at various abundances. Aeromonas was the second most abundant genus in the periphyton microbiome (Table 1), and in addition to being known biofilm-formers (Talagrand-Reboul et al., 2017), these bacteria are often associated with fish infections, wastewater pollution, and urban sewage (Popovic et al., 2015). The abundance of Flavobacterium and Aeromonas in these biofilms highlights the potential One Health role of biofilms, particularly their potential relationship to animal health and wastewater. Analyzing the role of freshwater biofilm microbial communities, wastewater discharge, and fish health should be considered in future studies.
Our prior study, which analyzed a subset of these periphyton samples from the urban sites via 16S rRNA gene sequencing, showed similar major taxa as presented in this study, but at somewhat different abundances (Mills et al., 2022). This is likely due to the difference in methods and selection of different samples within the dataset, specifically the samples in this study were from the entire watershed. The biofilm microbiome composition from this larger dataset had about half the RA of Cyanobacteria (7.83% v. 13.0%) and Bacteroidetes (7.83% v. 16.0%) compared the urban subset (Mills et al., 2022) (Figure 1C). While this may be a reflection of the different sequencing methods, Cyanobacteria are known to favor water bodies with greater anthropogenic nutrient loading (Volk and Lee, 2023), which may explain the greater RA of Cyanobacteria in the urban biofilms. Furthermore, while Bacteroidetes are common inhabitants of freshwater, they are known colonizers of animal guts, and often are the majority of the bacteria that make up the human gut microbiome (Thomas et al., 2011). The higher RA of Bacteroidetes in the prior periphyton subset may be due to the increased urbanization. Both Pseudomonadaceae and Flavobacteriaceae were in the top five families in the urban, 16S rRNA gene sequencing dataset and the full watershed, long-read dataset (Mills et al., 2022) (Supplementary Table S2). Finally, Flavobacterium and Acinetobacter were both in the top five genera for both study datasets (Table 1). While Pseudomonas was the second most abundant genus in the urban, 16S rRNA sequencing microbiome data, it was not in the top ten most abundant genera in the long-read sequencing microbiome data (Mills et al., 2022). From this comparison, it is possible to deduce that while Flavobacterium and Acinetobacter may be core taxa of these river biofilms, Pseudomonas may only be a key taxon in urban areas. However, it may also reflect a bias in the differences in sequencing methods.
Microbiomes within epilithic biofilms are known to shift temporally more frequently than with location (Zeglin, 2015), so differences in periphyton microbiome diversity and composition with season were expected (Figure 3). Our prior study showed a significant difference in alpha diversity of periphyton microbiomes with season, not location (Mills et al., 2022). It was surprising that the same result was not found here (Figures 1A, B), however this may be due to limitations in diversity analysis at this sequencing depth. For beta diversity, our prior study identified significant differences with season, not location (Mills et al., 2022). Similarly, in this long- read analysis, when testing beta diversity separately, season was also significant but location was not (Figure 2), verifying that season is a stronger driver of microbial community structure and composition than the location in the watershed (Zeglin, 2015).
Among the taxa that had different abundances between seasons, several species stood out, with three distinctive species of Porphyrobacter exhibiting higher abundance in summer biofilm samples compared to spring (Supplementary Table S5). Porphyrobacter have typically been isolated from water habitats (hot spring, seawater, lake), and thrive in warm temperatures, with an optimal growth between 30°C and 35°C (Xu et al., 2018), which may explain their abundance in the summer biofilms, when temperatures in this region are the highest. The Cyanobacteria L. boryana was also more abundant in the summer than in the winter and spring, which is also likely due to Cyanobacteria thriving in warmer temperatures (Volk and Lee, 2023). A. salmonicida was the only species that was more abundant in the spring than other seasons. This is potentially because this species is mesophilic and prefers an intermediary temperature, which is found during the spring season (Charette, 2021). A. salmonicida has a genome that is known to carry many MGEs, including plasmids that carry multidrug resistant ARGs and virulence factors; this is important because this species is mainly regarded as an opportunistic fish pathogen (Charette, 2021). While no ARGs were annotated on A. salmonicada hosts in this long-read analysis, two transposase MGEs were annotated on a single read from sample P33. A. salmonicada may play an important, yet understudied role in the biofilm resistome and environmental AR, particularly considering the One Health component of this species as a fish pathogen.
When controlling for season, there were significant differences with sampling location, which confirms changes in biofilm microbial communities longitudinally, along a watershed gradient. Factors that are known to change spatially, such as land use, hydrology, metal contamination, and nutrient concentrations, have a significant impact on freshwater microbiomes (Zeglin, 2015). Specifically, the largest difference in community structure by location in the watershed was found between the sites in and around Columbus, OH (“upstream” and “downstream”), and the way upstream site. This reflects the impact of urbanization on biofilm microbiomes. Specifically of interest in the differential abundance results by location were those taxa that increased downstream the urban center of Columbus, OH. The known fecal-associated family, Enterobacteriaceae, which has incredible medical, public health, and veterinary significance (Janda and Abbott, 2021), was more abundant in the downstream sites than way upstream (Supplementary Table S4). This is likely due to the numerous sources of fecal pollution entering waterways in Columbus, such as urban wastewater and stormwater (Lee et al., 2020). Furthermore, there could be a cumulative effect of agricultural nonpoint source fecal pollution that entered the watershed upstream of Columbus that contributed to the overall increased abundance of Enterobacteriaceae. It is also clear in the relative abundance of this family, that while only the comparison of downstream v. way upstream was significantly different, the way upstream relative abundance was the lowest (0.0619), followed by the upstream location (0.0983). The downstream location RA of Enterobacteriaceae was the highest (0.1651), but the way downstream RA, was still higher than the way upstream and upstream locations (0.1164). This finding shows the accumulation of Enterobacteriaceae in freshwater biofilms from upstream to downstream in a watershed with increased anthropogenic pressure. Downstream Columbus, there was also increased RA of the fish pathogen, P. koreensis (Lau et al., 2024), and one of the causative agents of shigellosis (bacillary dysentery), S. flexneri (Nisa et al., 2020) (Supplementary Table S6). None of the species that were more abundant in upstream sites are known pathogens (Willems, 2014; Zheng et al., 2016; Han et al., 2020). Prior studies have shown the impact of polluted river water from an urban area on periphyton microbial communities (Washington et al., 2013; Liao et al., 2019).
4.3 Periphyton carries a diverse and mobile resistome
It is uncommon to find prior metagenomic studies of the river biofilm resistome. Most studies target a panel of ARGs with quantitative PCR (Proia et al., 2016; Roberto et al., 2019; Li and Zhang, 2020; Reichart et al., 2021), which limits the conclusions that can be made, since only the pre-selected panel of genes are compared. One prior study that compiled sequence data of freshwater biofilms from several different locations identified the most abundant ARG classes as bacitracin, multidrug, MLS, aminoglycoside, beta-lactam, chloramphenicol, sulfonamide, and tetracycline (Yao et al., 2022). These Ohio biofilms had a similar resistome composition, with six of the same top eight ARG classes (multidrug, bacitracin, MLS, tetracycline, beta-lactam, and aminoglycoside; Supplementary Figure S3).
An abundant ARG class in the periphyton resistome that was not expected was glycopeptide resistance. Glycopeptide antibiotics are reserved for human use against life-threatening Gram-positive bacterial infections (Yushchuk et al., 2020). The glycopeptide resistance genes were all for vancomycin resistance. However, none of these genes were identified in pathogenic bacterial hosts. Specifically, we did not find any vancomycin-resistant Enterococcus (VRE) or vancomycin-resistant Staphylococcus aureus (VRSA) (Yushchuk et al., 2020). It is most likely the vancomycin resistance in these biofilms are from naturally occurring environmental bacteria, as most of the genes identified in this study are from the biosynthetic gene clusters of environmental bacteria that naturally produce vancomycin, which are largely found on chromosomes (Yushchuk et al., 2020). Furthermore, two bacterial hosts of the vanR gene in this study were from families of bacteria known to produce vancomycin (Micromonosporaceae and Streptosporangiaceae) (Yushchuk et al., 2020). The large proportion of glycopeptide ARGs is likely due to a large presence of natural, not clinically relevant AR, so is not a major public health threat.
The most abundant MGE class in periphyton was transposase enzymes (Supplementary Figure S3), which catalyze the movement of DNA, including ARGs, between bacteria via phage or plasmids (Frost et al., 2005; Siguier et al., 2014). Transposases can also facilitate the movement of the MGEs integrons and transposons, which package mobile DNA (Frost et al., 2005; Gillings, 2014). Although this study did not analyze the proportion of the annotated functional genes that were hosted in plasmids versus chromosomes, transposases play a crucial role in plasmid gene transfer and acquisition (Frost et al., 2005). Furthermore, conjugation via plasmids is thought to be the main route of HGT in microbial communities (Abe et al., 2020), so this may explain the high abundance of transposase genes in periphyton communities.
Recombinase was the next most abundant MGE class, and these genes encode enzymes that catalyze gene movement within cells, not between cells (Frost et al., 2005). They facilitate gene acquisition by assisting in integrating DNA into the host genome, such as from phage (Wang et al., 2010). Integrase was the final major category of MGEs, and these enzymes are also important in ARG acquisition, as they catalyze integron recombination (Souque et al., 2021). Integrons carry and collect exogenous DNA on gene cassettes, which can include ARGs (Gillings, 2014). Integrons were identified in these biofilm samples, but at a much lower abundance than integrase or transposase (Supplementary Figure S2). Similarly, transposons were identified in the periphyton samples at a much lower abundance than other MGE categories. The transposon genes identified in periphyton samples exclusively encoded conjugative transposon proteins, which have great significance for AR due to their combination of transposons and transmissible plasmid traits (Clark and Padzernik, 2013). Conjugative transposons are capable of transferring genes between different species or genera as efficiently as within the same species (Scott and Churchward, 1995). This implies that in a diverse microbial population, such as an environmental biofilm, conjugative transposons greatly enhance the capacity for ARG transmission. These MGE results underscore the potential for gene mobility within biofilms. However, to fully understand the implications for ARG transmission and clinical relevance, it is essential to pair the MGE results with ARGs and their respective microbial hosts.
4.4 Periphyton have minimal clinically relevant ARG hosts
The use of long-read sequencing for the periphyton samples allowed for annotation of hosts from sequences containing ARGs without assembly or binning, which is unique compared to shotgun metagenomics (Kamathewatta et al., 2019). This study is crucial in documenting the ARG and MGE hosts within natural biofilms found in a river ecosystem. Shotgun metagenomic sequencing from freshwater biofilms identified ARG hosts via assembly methods and found that Proteobacteria were the most abundant hosts (Yao et al., 2022). Overall, 4/8 of the same phyla hosting ARGs as this study, including Proteobacteria, Bacteroidetes, Cyanobacteria, and Actinobacteria (Yao et al., 2022) (Figure 3A).
Although it might be expected that the most abundant ARG hosts are the most abundant bacteria in the microbiome, this is not the case (Table 1). Some bacteria are more common hosts than others. One study compared the abundance of horizontally transferred genes per genome and HGT hotspots between 80 different bacteria; HGT hotspots were defined as areas where horizontally transferred genes cluster in bacterial genomes (Oliveira et al., 2017). Several of the most abundant ARG and MGE hosts in this analysis had a relatively high abundance of horizontally transferred genes and hotspots, such as E. coli (Oliveira et al., 2017). Escherichia was the most abundant ARG and MGE host at a genus level (Table 1), and E. coli was the most abundant ARG and MGE host at a species level (Tables 2, 3). E. coli is known to be a common agent of HGT, transmitting and acquiring ARGs with relative ease (Hasegawa et al., 2018). Therefore, E. coli is an abundant host in periphyton because of the mobility potential in its genome, not necessarily its abundance in the microbial community. Pseudomonas was also a common ARG and MGE host, but it was not in the ten most abundant genera in the overall microbiome (Table 1). Pseudomonas may more frequently participate in HGT than other more abundant genera in the microbiome. This was demonstrated in Oliveira et al., as four different Pseudomonas species had relatively high horizontally transferred genes/genome and hotspots (2017). One species in Oliveira et al., Pseudomonas fluoroscencs, was identified several times in these periphyton samples, hosting transposase, recombinase, and integrase MGEs, as well as multidrug and phenicol ARGs (2017).
The high abundance of Cyanobacteria as hosts, specifically of MGEs is notable (Figure 3). Cyanobacteria may play a not yet fully known, but they play a critical role in the water resistome (Dias et al., 2019; Volk and Lee, 2023). Unlike other prokaryotes, Cyanobacteria form blooms and can produce hazardous toxins for human health (Cheung et al., 2012). Both factors may be important in the freshwater resistome. HGT may be increased while Cyanobacteria participate in blooms, due to the close contact and cell density during this phenomenon (Wang et al., 2020a). It has been shown that bloom-forming Cyanobacteria host a greater abundance of insertion sequence MGEs than non-bloom-forming Cyanobacteria (Lin et al., 2010). Cyanobacterial toxins, such as microcystin, also promote HGT (Xu et al., 2020). Finally, ARG abundance has been shown to increase in water during Cyanobacteria blooms compared to non-bloom periods (Wang et al., 2020a).
Another unique host of ARGs and MGEs in these periphyton samples were viruses, specifically phage (Figure 3). While previous research has called into question the nature of viruses hosting ARGs in metagenomic studies, we interpret our findings cautiously, emphasizing that these results indicate ARGs being associated with phage in these biofilm samples (Enault et al., 2017). Phage have previously been identified as a significant carrier of AR genes in the environment (Lekunberri et al., 2017; Abe et al., 2020). At a phyla level, Uroviricota viruses were the second most abundant ARG hosts and the third most abundant MGE hosts in periphyton. Phage can act as MGEs (Frost et al., 2005), and many studies demonstrate ARG transfer via phage (Abe et al., 2020). The viruses identified as hosts in this study were all from the genus Muvirus (Table 2), also known as bacteriophage Mu. They are a hybrid of both viruses and transposons, so these phage can transpose into a host genome without the use of transposons (Clark and Padzernik, 2013). Mu viruses, including the Escherichia virus mu and Enterobacteria phage SfMu, which were the two phage associated with ARGs in these biofilm samples, are common inhabitants of diverse environments, including host-associated, aquatic, and terrestrial microbial communities (Zhang et al., 2023). The phage hosts of ARG and MGEs in this study demonstrate the high HGT potential from periphyton samples.
Overall, our analysis did not identify potential pathogens hosting ARGs in periphyton. A future study with greater sequencing depth may uncover more clinically relevant ARGs and their hosts, but the primary finding from this this study is that periphyton microbial communities are not important reservoirs of clinically relevant ARGs in pathogenic bacterial hosts. Therefore, we conclude that there is not a direct threat of transmission of AR bacteria from periphyton to humans or animals with exposure in similar settings. A future goal should be to identify if aquatic biofilms harbor more dangerous AR bacteria in different settings, such as low- and middle-income countries (LMICs) with more limited water, sanitation, and hygiene (WASH) regulations. While the exact role of environmental microorganisms in the global spread and persistence of ARGs is not yet fully known, it is acknowledged that the environment is a reservoir of gene evolution and transfer (Larsson and Flach, 2021). This study reveals that periphyton samples exhibit significant potential for transmitting mobile ARGs between bacteria, due to their high microbial diversity, abundant MGEs, and varied ARGs. AR is a global public health crisis, and environmental reservoirs must be considered in a One Health approach in combatting this issue. The abundance and diversity of ARGs in biofilms contribute to the overall environmental burden of AR, thereby perpetuating the public health crisis and potentially facilitating the emergence of novel ARGs.
Data availability statement
The datasets presented in this study can be found in online repositories. Sequencing data and metadata can be found at the NCBI Sequence Read Archive (SRA) under the accession number PRJNA1116137 (http://www.ncbi.nlm.nih.gov/bioproject/1116137).
Author contributions
MM: Formal Analysis, Funding acquisition, Investigation, Methodology, Visualization, Writing–original draft. TW: Funding acquisition, Project administration, Resources, Supervision, Writing–review and editing. JL: Conceptualization, Funding acquisition, Methodology, Resources, Supervision, Writing–review and editing.
Funding
The author(s) declare that financial support was received for the research, authorship, and/or publication of this article. This study was funded by the Centers for Disease Control and Prevention and SEED from the College of Food, Agricultural and Environmental Sciences, Ohio State University.
Acknowledgments
The authors thank the assistance from Drs. Dixie Mollenkopf and Seungjun Lee in sample handling and processing, and Dr. Mažeika Patricio Sullivan and his lab for field sampling.
Conflict of interest
The authors declare that the research was conducted in the absence of any commercial or financial relationships that could be construed as a potential conflict of interest.
Publisher’s note
All claims expressed in this article are solely those of the authors and do not necessarily represent those of their affiliated organizations, or those of the publisher, the editors and the reviewers. Any product that may be evaluated in this article, or claim that may be made by its manufacturer, is not guaranteed or endorsed by the publisher.
Supplementary material
The Supplementary Material for this article can be found online at: https://www.frontiersin.org/articles/10.3389/fenvs.2024.1440635/full#supplementary-material
References
Abe, K., Nomura, N., and Suzuki, S. (2020). Biofilms: hot spots of horizontal gene transfer (HGT) in aquatic environments, with a focus on a new HGT mechanism. FEMS Microbiol. Ecol. 96 (5), fiaa031. doi:10.1093/femsec/fiaa031
Arango-Argoty, G. A., Dai, D., Pruden, A., Vikesland, P., Heath, L. S., and Zhang, L. (2019). NanoARG: a web service for detecting and contextualizing antimicrobial resistance genes from nanopore-derived metagenomes. Microbiome 7, 88. doi:10.1186/s40168-019-0703-9
Bagra, K., Bellanger, X., Merlin, C., Singh, G., Berendonk, T. U., and Klümper, U. (2023). Environmental stress increases the invasion success of antimicrobial resistant bacteria in river microbial communities. Sci. total Environ. 904, 166661. doi:10.1016/j.scitotenv.2023.166661
Balcázar, J. L., Subirats, J., and Borrego, C. M. (2015). The role of biofilms as environmental reservoirs of antibiotic resistance. Front. Microbiol. 6, 1216. doi:10.3389/fmicb.2015.01216
Boolchandani, M., D’Souza, A. W., and Dantas, G. (2019). Sequencing-based methods and resources to study antimicrobial resistance. Nat. Rev. Genet. 20, 356–370. doi:10.1038/s41576-019-0108-4
Charette, S. J. (2021). Microbe Profile: Aeromonas salmonicida: an opportunistic pathogen with multiple personalities. Microbiology 167, 5. doi:10.1099/mic.0.001052
Cheung, M. Y., Liang, S., and Lee, J. (2012). Toxin-producing Cyanobacteria in freshwater: a review of the problems, impact on drinking water safety, and efforts for protecting public health. J. Microbiol. 51 (1), 1–10. doi:10.1007/s12275-013-2549-3
Clark, D. P., and Pazdernik, N. J. (2013). “Chapter e22- Mobile DNA,” in Molecular biology. Second Edn (Amsterdam, Netherlands: Elsevier), e553–e558.
Davies, J., and Davies, D. (2010). Origins and evolution of antibiotic resistance. Microbiol. Mol. Biol. Rev. 74 (3), 417–433. doi:10.1128/MMBR.00016-10
Dias, E., Oliveira, M., Manageiro, V., Vasconcelos, V., and Caniça, M. (2019). Deciphering the role of Cyanobacteria in water resistome: hypothesis justifying the antibiotic resistance (phenotype and genotype) in Planktothrix genus. Sci. total Environ. 652, 447–454. doi:10.1016/j.scitotenv.2018.10.167
Enault, F., Briet, A., Bouteille, L., Roux, S., Sullivan, M. B., and Petit, M.-A. (2017). Phages rarely encode antibiotic resistance genes: a cautionary tale for virome analyses. ISME J. 11, 237–247. doi:10.1038/ismej.2016.90
Fernandes, G., Bastos, M. C., de Vargas, J. P. R., Le Guet, T., Clasen, B., and Dos Santos, D. R. (2020). The use of epilithic biofilms as bioaccumulators of pesticides and pharmaceuticals in aquatic environments. Ecotoxicology 29 (9), 1293–1305. doi:10.1007/s10646-020-02259-4
Finley, R. L., Colignon, P., Larsson, D. G. J., McEwen, S. A., Li, X.-Z., Gaze, W. H., et al. (2013). The scourge of antibiotic resistance: the important role of the environment. Clin. Infect. Dis. 57 (5), 704–710. doi:10.1093/cid/cit355
Frost, L. S., Leplae, R., Summers, A. O., and Toussaint, A. (2005). Mobile genetic elements: the agents of open source evolution. Nat. Rev. Microbiol. 3 (9), 722–732. doi:10.1038/nrmicro1235
Gillings, M. R. (2014). Integrons: past, present, and future. Microbiol. Mol. Biol. Rev. 78 (2), 257–277. doi:10.1128/MMBR.00056-13
Gu, Z., Gu, L., Eils, R., Schlesner, M., and Brors, B. (2014). Circlize Implements and enhances circular visualization in R. Bioinformatics 30 (19), 2811–2812. doi:10.1093/bioinformatics/btu393
Guo, K., Wu, N., Li, W., Baattrup-Pedersen, A., and Riis, T. (2021). Microbial biofilm community dynamics in five lowland streams. Sci. total Environ. 798, 149169. doi:10.1016/j.scitotenv.2021.149169
Han, J. E., Kang, W., Lee, J.-Y., Sung, H., Hyun, D.-W., Kim, H. S., et al. (2020). Tabrizicola piscis sp. nov., isolated from the intestinal tract of a Korean indigenous freshwater fish, Acheilognathus koreensis. Int. J. Syst. Evol. Microbiol. 70 (4), 2305–2311. doi:10.1099/ijsem.0.004034
Harper, L. R., Buxton, A. S., Rees, H. C., Bruce, K., Brys, R., Halfmaerten, D., et al. (2019). Prospects and challenges of environmental DNA (eDNA) monitoring in freshwater ponds. Hydrobiologia 826, 25–41. doi:10.1007/s10750-018-3750-5
Hasegawa, H., Suzuki, E., and Maeda, S. (2018). Horizontal plasmid transfer by transformation in Escherichia coli: environmental factors and possible mechanisms. Front. Microbiol. 9, 2365. doi:10.3389/fmicb.2018.02365
Janda, J. M., and Abbott, S. L. (2021). The changing face of the family Enterobacteriaceae (order: “enterobacterales”): new members, taxonomic issues, geographic expansion, and new diseases and Disease syndromes. Clin. Microbiol. Rev. 34 (2), e00174-20. doi:10.1128/CMR.00174-20
Jo, S. J., Kwon, H., Jeong, S.-Y., Lee, C.-H., and Kim, T. G. (2016). Comparison of microbial communities of activated sludge and membrane biofilm in 10 full-scale membrane bioreactors. Water Res. 101, 214–225. doi:10.1016/j.watres.2016.05.042
Kamathewatta, K. I., Bushell, R. N., Young, N. D., Stevenson, M. A., Billman-Jacobe, H., Browning, G. F., et al. (2019). Exploration of antibiotic resistance risks in a veterinary teaching hospital with Oxford Nanopore long read sequencing. PloS ONE 14 (5), e0217600. doi:10.1371/journal.pone.0217600
Kautza, A., and Sullivan, S. (2016). Anthropogenic and natural determinants of fish food-chain length in a midsize river system. Freshw. Sci. 35 (3), 895–908. doi:10.1086/685932
Larsson, D. G. J., and Flach, C.-F. (2021). Antibiotic resistance in the environment. Nat. Rev. Microbiol. 20, 257–269. doi:10.1038/s41579-021-00649-x
Lau, M. M. L., Kho, C. J. Y., Chung, H. H., and Zulkharnain, A. (2024). Isolation, identification and characterisation of Pseudomonas koreensis CM-01 isolated from diseased Malaysian mahseer (Tor tambroides). Fish and Shellfish Immunol. 148, 109518. doi:10.1016/j.fsi.2024.109518
Lee, S., Suits, M., Wituszynski, D., Winston, R., Martin, J., and Lee, J. (2020). Residential urban stormwater runoff: a comprehensive profile of microbiome and antibiotic resistance. Sci. total Environ. 723, 138033. doi:10.1016/j.scitotenv.2020.138033
Lekunberri, I., Subirats, J., Borrego, C. M., and Balcázar, J. L. (2017). Exploring the contribution of bacteriophages to antibiotic resistance. Environ. Pollut. 220, 981–984. doi:10.1016/j.envpol.2016.11.059
Li, Q., and Zhang, Q. (2020). Prevalence and pollution characteristics of antibiotic resistant genes in one high anthropogenically-impacted river. PLoS one 15, e0231128. doi:10.1371/journal.pone.0231128
Liao, K., Bai, Y., Huo, Y., Jian, Z., Hu, W., Zhao, C., et al. (2019). Use of convertible flow cells to simulate the impacts of anthropogenic activities on river biofilm bacterial communities. Sci. total Environ. 653, 148–156. doi:10.1016/j.scitotenv.2018.10.363
Lin, S., Haas, S., Zemojtel, T., Xiao, P., Vingron, M., and Li, R. (2010). Genome-wide comparison of cyanobacterial transposable elements, potential genetic diversity indicators. Gene 473, 139–149. doi:10.1016/j.gene.2010.11.011
Logsdon, G. A., Vollger, M. R., and Eichler, E. E. (2020). Long-read human genome sequencing and its applications. Nat. Rev. Genet. 21, 597–614. doi:10.1038/s41576-020-0236-x
Ma, L., Xia, Y., Li, B., Yang, Y., Li, L.-G., Tiedje, J. M., et al. (2016). Metagenomic assembly reveals hosts of antibiotic resistance genes and the shared resistome in pig, chicken, and human feces. Environ. Sci. and Technol. 50 (1), 420–427. doi:10.1021/acs.est.5b03522
Mallick, H., Rahnavard, A., McIver, L. J., Zhang, Y., Nguyen, L. H., Tickle, T. L., et al. (2021). Multivariable association discovery in population-scale meta-omics studies. PLoS Comput. Biol. 17 (11), e1009442. doi:10.1371/journal.pcbi.1009442
Martin, S., Heavens, D., Lan, Y., Horsfield, S., Clark, M. D., and Leggett, R. M. (2022). Nanopore adaptive sampling: a tool for enrichment of low abundance species in metagenomic samples. Genome Biol. 23, 11. doi:10.1186/s13059-021-02582-x
McMurdie, P. J., and Holmes, S. (2013). phyloseq: an R package for reproducible interactive analysis and graphics of microbiome census data. PLoS ONE 8 (4), e61217. doi:10.1371/journal.pone.0061217
Mills, M., Lee, S., Mollenkopf, D., Wittum, T., Sullivan, S. M. P., and Lee, J. (2022). Comparison of environmental microbiomes in an antibiotic resistance-polluted urban river highlights periphyton and fish gut communities as reservoirs of concern. Sci. total Environ. 851, 158042. doi:10.1016/j.scitotenv.2022.158042
Mills, M. C., Lee, S., Piperata, B. A., Garabed, R., Choi, B., and Lee, J. (2023). Household environment and animal fecal contamination are critical modifiers of the gut microbiome and resistome in young children from rural Nicaragua. Microbiome 11, 207. doi:10.1186/s40168-023-01636-5
Mock, A., Braun, M., Scholl, C., Fröhling, S., and Erkut, C. (2023). Transcriptome profiling for precision cancer medicine using shallow nanopore cDNA sequencing. Sci. Rep. 13, 2378. doi:10.1038/s41598-023-29550-8
Nisa, I., Qasim, M., Yasin, N., Ullah, R., and Ali, A. (2020). Shigella flexneri: an emerging pathogen. Folia Microbiol. 65, 275–291. doi:10.1007/s12223-020-00773-w
Ohio Environmental Protection Agency (EPA) (2014). Scioto River watershed. Available at: https://epa.ohio.gov/dsw/tmdl/SciotoRiver.aspx (Accessed July 3, 2014).
Oksanen, J., Blanchet, F. G., Friendly, M., Kindt, R., Legendre, P., McGlinn, D., et al. (2020). Vegan: community ecology package. R. package version 2, 5–7. doi:10.32614/CRAN.package.vegan
Oliveira, P. H., Touchon, M., Cury, J., and Rocha, E. P. C. (2017). The chromosomal organization of horizontal gene transfer in bacteria. Nat. Commun. 8, 841. doi:10.1038/s41467-017-00808-w
Perry, I., Jâms, I. B., Casas-Mulet, R., Hamutoko, J., Marchbank, A., Lendelvo, S., et al. (2022). Challenges to implementing environmental-DNA monitoring in Namibia. Front. Environ. Sci. 9. doi:10.3389/fenvs.2021.773991
Popovic, N. T., Kazazic, S. P., Strunjak-Perovic, I., Barisic, J., Klobucar, R. S., Kepec, S., et al. (2015). Detection and diversity of aeromonads from treated wastewater and fish inhabiting effluent and downstream waters. Ecotoxicol. Environ. Saf. 120, 235–242. doi:10.1016/j.ecoenv.2015.06.011
Proia, L., von Schiller, D., Sànchez-Mesió, A., Sabater, S., Borrego, C. M., Rodríguez-Mozaz, S., et al. (2016). Occurrence and persistence of antibiotic resistance genes in river biofilms after wastewater inputs in small rivers. Environ. Pollut. 210, 121–128. doi:10.1016/j.envpol.2015.11.035
Reichart, G., Hilgert, S., Alexander, J., de Azevedo, J. C. R., Morck, T., Fuchs, S., et al. (2021). Determination of antibiotic resistance genes in a WWTP-impacted river in surface water, sediment, and biofilm: influence of seasonality and water quality. Sci. total Environ. 768, 144526. doi:10.1016/j.scitotenv.2020.144526
Roberto, A. A., Van Gray, J. B., Engohang-Ndong, J., and Leff, L. G. (2019). Distribution and co-occurrence of antibiotic and metal resistance genes in biofilms of an anthropogenically impacted stream. Sci. total Environ. 688, 437–449. doi:10.1016/j.scitotenv.2019.06.053
Scott, J. R., and Churchward, G. G. (1995). Conjugative transposition. Annu. Rev. Microbiol. 49, 367–397. doi:10.1146/annurev.mi.49.100195.002055
Sentenac, H., Loyau, A., Leflaive, J., and Schmeller, D. S. (2021). The significance of biofilms to human, animal, plant and ecosystem health. Funct. Ecol. 36 (2), 294–313. doi:10.1111/1365-2435.13947
Shen, W., and Ren, H. (2021). TaxonKit: a practical and efficient NCBI taxonomy toolkit. J. Genet. genomics 48 (9), 844–850. doi:10.1016/j.jgg.2021.03.006
Siguier, P., Gourbeyre, E., and Chandler, M. (2014). Bacterial insertion sequences: their genomic impact and diversity. FEMS Microbiol. Rev. 38 (5), 865–891. doi:10.1111/1574-6976.12067
Souque, C., Escudero, J. A., and MacLean, R. C. (2021). Integron activity accelerates the evolution of antibiotic resistance. eLife 10, e62474. doi:10.7554/eLife.62474
Talagrand-Reboul, E., Jumas-Bilak, E., and Lamy, B. (2017). The social life of Aeromonas through biofilm and quorum sensing systems. Front. Microbiol. 8, 37. doi:10.3389/fmicb.2017.00037
Tamminen, M., Spaak, J., Tlili, A., Eggen, R., Tamm, C., and Räsänen, K. (2022). Wastewater constituents impact biofilm microbial community in receiving streams. Sci. total Environ. 807, 151080. doi:10.1016/j.scitotenv.2021.151080
Thomas, F., Hehemann, J.-H., Rebuffet, E., Czjzek, M., and Michel, G. (2011). Environmental and gut Bacteroidetes: the food connection. Front. Microbiol. 2, 93. doi:10.3389/fmicb.2011.00093
Viehweger, A., Marquet, M., Hölzer, M., Dietze, N., Pletz, M. W., and Brandt, C. (2023). Nanopore-based enrichment of antimicrobial resistance genes – a case-based study. GigaByte 2023, 1–15. doi:10.46471/gigabyte.75
Volk, A., and Lee, J. (2023). Cyanobacterial blooms: a player in the freshwater environmental resistome with public health relevance? Environ. Res. 216 (2), 114612. doi:10.1016/j.envres.2022.114612
Wahli, T., and Madsen, L. (2018). Flavobacteria, a never ending threat for fish: a review. Bacteriology 5, 26–37. doi:10.1007/s40588-018-0086-x
Wang, Y., Yau, Y.-Y., Perkins-Balding, D., and Thomson, J. G. (2010). Recombinase technology: applications and possibilities. Plant cell Rep. 30, 267–285. doi:10.1007/s00299-010-0938-1
Wang, Z., Chen, Q., Zhang, J., Guan, T., Chen, Y., and Shi, W. (2020a). Critical roles of cyanobacteria as reservoir and source for antibiotic resistance genes. Environ. Int. 144, 106034. doi:10.1016/j.envint.2020.106034
Washington, V. J., Lear, G., Neale, M. W., and Lewis, G. D. (2013). Environmental effects on biofilm bacterial communities: a comparison of natural and anthropogenic factors in New Zealand streams. Freshw. Biol. 58 (11), 2277–2286. doi:10.1111/fwb.12208
Weiss, S., Xu, Z. Z., Peddada, S., Amir, A., Bittinger, K., Gonzalez, A., et al. (2017). Normalization and microbial differential abundance strategies depend upon data characteristics. Microbiome 5 (1), 27. doi:10.1186/s40168-017-0237-y
Willems, A. (2014). “The family comamonadaceae,” in The prokaryotes. Editors E. Rosenberg, E. F. DeLong, S. Lory, E. Stackebrandt, and F. Thompson (Berlin, Heidelberg: Springer), 777–851.
Wrenn, D. C., and Drown, D. M. (2023). Nanopore adaptive sampling enriches for antimicrobial resistance genes in microbial communities. GigaByte 2023, 1–14. doi:10.46471/gigabyte.103
Xu, L., Wu, Y.-H., Zhou, P., Cheng, H., Liu, Q., and Xu, X.-W. (2018). Investigation of the thermophilic mechanism in the genus Porphyrobacter by comparative genomic analysis. BMC genomics 19, 385. doi:10.1186/s12864-018-4789-4
Xu, L., Zhou, Z., Zhu, L., Han, Y., Lin, Z., Feng, W., et al. (2020). Antibiotic resistance genes and microcystins in a drinking water treatment plant. Environ. Pollut. 258, 113718. doi:10.1016/j.envpol.2019.113718
Yao, Y., Liu, Z., Yip, K. K., Pu, Y., Cheng, W., Li, M., et al. (2022). Cross-regional scale pollution of freshwater biofilms unveiled by antibiotic resistance genes. Sci. total Environ. 818, 151835. doi:10.1016/j.scitotenv.2021.151835
Yin, X., Li, L., Chen, X., Liu, Y.-Y., Lam, T.T.-Y., Topp, E., et al. (2023). Global environmental resistome: distinction and connectivity across diverse habitats benchmarked by metagenomic analyses. Water Res. 235, 119875. doi:10.1016/j.watres.2023.119875
Yushchuk, O., Binda, E., and Marinelli, F. (2020). Glycopeptide antibiotic resistance genes: distribution and function in the producer Actinomycetes. Front. Microbiol. 11, 1173. doi:10.3389/fmicb.2020.01173
Zeglin, L. H. (2015). Stream microbial diversity in response to environmental changes: review and synthesis of existing research. Front. Microbiol. 6, 454. doi:10.3389/fmicb.2015.00454
Zhang, M., Hao, Y., Yi, Y., Liu, S., Sun, Q., Tan, X., et al. (2023). Unexplored diversity and ecological functions of transposable phages. ISME J. 17, 1015–1028. doi:10.1038/s41396-023-01414-z
Zhao, Y., Zhang, Y., Guo, J., Wang, J., and Li, Y. (2022). Shifts in periphyton research themes over the past three decades. Environ. Sci. Pollut. Res. 30, 5281–5295. doi:10.1007/s11356-022-24251-7
Keywords: One Health, mobile genetic elements, antibiotic resistance genes, MinION sequencing, metagenomics, freshwater, periphyton
Citation: Mills M, Wittum T and Lee J (2024) Dynamic microbiome and mobile resistome are revealed in river biofilms from a multi-use watershed through long-read sequencing. Front. Environ. Sci. 12:1440635. doi: 10.3389/fenvs.2024.1440635
Received: 03 June 2024; Accepted: 06 August 2024;
Published: 16 August 2024.
Edited by:
Visva Bharati Barua, University of North Carolina at Charlotte, United StatesReviewed by:
Connor Brown, Virginia Tech, United StatesJannatul Ferdous, University of North Carolina at Charlotte, United States
Copyright © 2024 Mills, Wittum and Lee. This is an open-access article distributed under the terms of the Creative Commons Attribution License (CC BY). The use, distribution or reproduction in other forums is permitted, provided the original author(s) and the copyright owner(s) are credited and that the original publication in this journal is cited, in accordance with accepted academic practice. No use, distribution or reproduction is permitted which does not comply with these terms.
*Correspondence: Jiyoung Lee, lee.3598@osu.edu