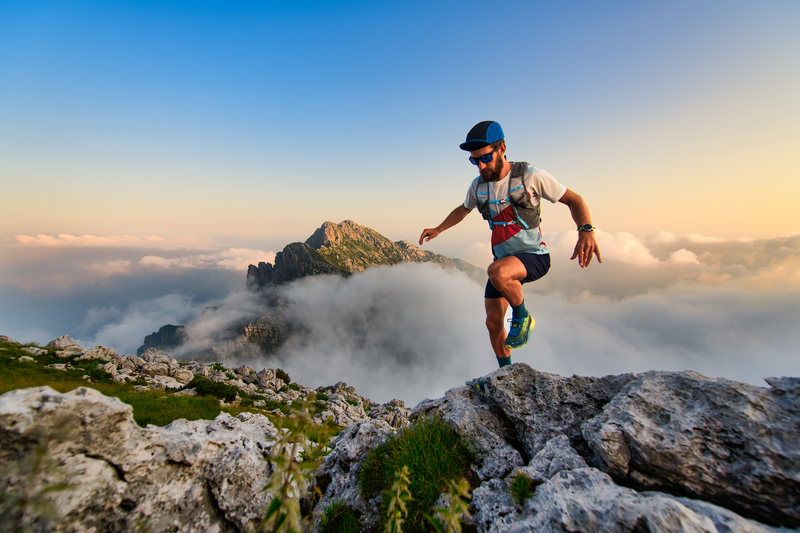
94% of researchers rate our articles as excellent or good
Learn more about the work of our research integrity team to safeguard the quality of each article we publish.
Find out more
ORIGINAL RESEARCH article
Front. Environ. Sci. , 07 January 2025
Sec. Environmental Economics and Management
Volume 12 - 2024 | https://doi.org/10.3389/fenvs.2024.1439927
This article is part of the Research Topic Financial Development, Institutions, and Markets in the Era of Climate Change View all articles
This paper examines the relationship between the changes in air temperature over recent decades and the economic performance of the European Union (EU) countries. It provides the climate-economic model of EU countries, considering the NUTS 3 regions to capture the within-country differences in air temperatures and economic growth at the regional level because of evident differences in the geographical landscape—elevation and climate. The sample covers 1,200 units at the NUTS 3 level from 2000 to 2022, using a panel data approach. In addition to the analysis provided at the NUTS 3 level, the paper’s uniqueness also lies in focusing on temperature variations using the Cooling index, a weather-based technical measure designed to describe the need for air cooling (or air conditioning). The findings confirm an inverse relationship between air temperatures and economic performance. These results contribute to the climate-economic literature that provides policy implications to shape public policies dealing with the impacts and risks of climate change.
The literature on climate change and climate-economic models considers increasing air temperature to be one of the representative effects of global warming, which has several negative consequences for the world economy, as well as the economy of individual countries. One of the most common economic consequences is a change in economic production due to impacts on agriculture, energetics, infrastructure, and many other economic areas. These consequences may vary depending on geographic location, economic sector, and a country’s ability to adapt to or mitigate the effects of climate change (Petrovic, 2023).
In general, the increase in air temperature represents a significant threat to economic stability and development. Global warming and related temperature changes lead to adverse weather events, which are also related to threats associated with extreme temperatures, which harms agricultural production and GDP in many countries worldwide (United Nations, 2018; World Bank, 2016). Temperature affects production at the global (Burke et al., 2015), national (Deryugina and Hsiang, 2017), and regional levels (Kalkuhl and Wenz, 2020) and influences the country, regardless of its economic or globalization level.
Currently, we are witnessing increasing temperatures in the EU (Patterson, 2023), and the discussion regarding the detrimental effects of climate change on economic outcomes is viral. However, empirical research provides inconclusive findings regarding the inverse relationship between the changes in air temperature and economic performance. While several studies confirm the negative relationship between climate change and economic performance, others contradict this conclusion (Burke et al., 2015; Dell et al., 2012; Cattaneo and Peri, 2016).
Since the consequences of increasing air temperatures may vary depending on geographic location (as mentioned by Colacito et al., 2019; Petrovic, 2023), we were stimulated to examine these effects not only between EU countries but also within them because of evident differences in the geographical landscape—elevation and climate within EU countries, except for observed sound economic and population heterogeneities.
Considering the within-country differences, the novelty of the paper lies in the examination of the relationship between the changes in air warming over recent decades and the economic performance of the EU countries at the NUTS 3 level, which is a small region for specific diagnoses, with a population ranging from 150,000 to 800,000.
We aim to address the research question of whether higher air temperatures hamper the economic growth of EU countries when considering the NUTS 3 regions. In the climate-economic model, the NUTS 3 level is considered for the analyses to provide us with deeper insights into the problem. It allows us to consider within-country differences pointing to the geography in terms of countries’ largeness and the elevation among their regions, corresponding to different temperatures within the country. In addition to the analysis provided at the NUTS 3 level, the paper’s uniqueness also lies in focusing on temperature variations using the Cooling index, a weather-based technical measure designed to describe the need for air cooling (or air conditioning). The sample covers 1,200 units at the NUTS 3 level from 2000 to 2022, using a panel data approach. To capture the nature of the relationship in question, we conduct a regression analysis based on static and dynamic estimation techniques.
The remainder of this paper is organized as follows: Section 2 presents a literature review that focuses on related empirical research and presents the main research hypothesis. Section 3 describes the methods and data used in the research. The main research findings are presented and discussed in Section 4. Finally, Section 5 provides the conclusion.
To investigate the economic consequences of changes in air temperatures, panel econometric estimates are used, mainly using the temperature variable (Hsiang, 2016; Auffhammer, 2018). Most of the research in this area has made a breakthrough in examining the relationship between weather conditions and economic aggregates such as gross domestic product (Dell et al., 2012; Hsiang and Narita, 2012; Burke et al., 2015; Burke et al., 2018). The relationship between climate change and GDP development through econometric models is an increasingly popular scientific problem (Field et al., 2014; Hsiang, 2016; Cashin et al., 2017). Nordhaus and Moffat (2017) and Tol (2012) highlighted the impact of climate change on the world economy, but in recent years, authors such as Hsiang (2010); Barrios et al. (2010); Deryugina (2011); Hsiang and Narita (2012); Burke et al. (2015); and Burke et al. (2018) have focused on the impact of weather and its changes on the specific economic aggregate of GDP. Hsiang (2010) confirmed the impact of extremely hot days with average temperatures of 27°C–29°C on national production in the Caribbean and Central America.
In general, there are several groups of estimates of changes in the GDP of economies due to warming. The first group takes a conservative approach, projecting a reduction in the global GDP per capita of 7–13 percent by 2,100 (varying between economies depending on the temperature increase rate and climate condition variability) (Kahn et al., 2021). The second group provides mid-range estimates, suggesting a 7–14 percent reduction in GDP (Kalkuhl and Wenz, 2020), and the third group estimates a loss of approximately 20 percent (Burke et al., 2015). Deryugina and Hsiang (2014) and Deryugina and Hsiang (2017) also confirmed a non-linear relationship between temperature and the level of GDP in the United States. Colacito et al. (2019) confirmed their results, stating that the increase in temperature has the most significant effect on the GDP of the United States, especially in summer and autumn. Dell et al. (2012), on the contrary, confirmed no significant correlation between an increase in temperature and the slowdown in economic growth in developed countries. The findings of Petrovic (2023) partially agree with those of Dell et al. (2012). They stated that the relationship between climate change and economic outcomes varies among countries and that the negative impact is still very rare. In developing countries, however, the relationship is significant. Fankhauser and Tol (2005) highlighted the effects of climate change by measuring capital accumulation and the related propensity of people to save, which, in turn, reduces economic growth.
According to Nordhaus and Moffat (2017), a general average temperature increase of 3°C will reduce economic output by approximately 2 percent. The importance of measuring the relationship between temperature and GDP is also emphasized by the Swiss Re (2021), which warns that climate change could decrease the GDP of the worldwide economy by 2050 if global temperatures increase by 3.2°C. Under this scenario, China could lose nearly 24% of its GDP, while the United States, Canada, and the United Kingdom each could face a 10% loss, and Europe could experience a 11% decrease.
Agarwala et al. (2021) mentioned that the macroeconomic consequences of climate change hamper the public finance outcomes and fiscal (debt) sustainability of countries through various transmission channels. Considering the macroeconomic impacts of climate change on state budgeting, similar approaches have been performed by Acevedo Mejia and Acevedo Mejia (2014); Koetsier (2017); Kahn et al. (2021); Kotz et al. (2021); and Beirne et al. (2021). The authors also point to risks of macroeconomic nature, the consequences of which are linked to the disruption of economic activity, which can negatively affect tax revenues or social transfers from various government levels (Schuler et al., 2018), inflation, and interest rates due to the effects of exchange rates (Farhi and Gabaix, 2016). According to Arawatari et al. (2023) and Semieniuk et al. (2021), changes in weather conditions and air temperatures can cause aggregate supply and demand shocks, which lead to instability in employment, public finances, and production; thus, they also lead to overall structural changes in the economy. The consequences of the impact of temperature changes on the economy can also be defined as financial vulnerability, which is negatively transmitted to the public sector through the instability of the financial sector (Acharya et al., 2014; Farhi and Tirole, 2018; Gennaioli et al., 2018). The impacts of climate change and related changes in climate policies also have an impact on international trade and financial flows within the economy. Problems arising from the balance of payments issues can lead to debt crises (Bouchet et al., 2018).
The direct consequences of warming on productivity are mainly caused by the impact on human, physical, and natural capital, while the volatility of temperatures is also related to deteriorating results of national production (Day et al., 2019). Kahn et al. (2021) or Dasgupta et al. (2021), however, draw attention to differences in production in individual sectors (differences if the economy is oriented toward agriculture, i.e., exterior-orientated economy, or, on the contrary, an economy-oriented to factories, i.e., work to interiors). Physical productivity should not be neglected, including, for example, infrastructure. Watson et al. (2021) provided the example of reduced train speeds in Great Britain due to extreme hot weather or power outages in Texas (United States). Agarwala et al. (2021) assessed these threats as macroeconomic risks caused by changes in the climatic environment. At the same time, other research studies have confirmed that the costs resulting from reduced worker productivity due to increased temperatures in their work environment are greater than the negative effects of any other work environment-related impacts (DARA, 2012; Deryugina and Hsiang, 2014). In an earlier work, Sala-i-Martin (1997) employed the variable of a country’s absolute latitude, finding a positive sign of the beta estimate when examining the economic growth determinants.
A certain group of research is also focused on monitoring the relationship between changing air temperatures and the economic results of economies due to efforts to create programs that mitigate the negative impacts of global warming or provide solutions to address these impacts promptly (Burke et al., 2018).
In general, the current empirical research assumes a negative effect of air warming on the economic performance of countries, providing analyses at the country level and using the air temperature as an explanatory variable.
However, as mentioned by Colacito et al. (2019) and Petrovic (2023), the consequences of increasing air temperatures may vary depending on the geographic location. When focusing on the EU, this leads us to consider the evident geographical differences within the EU landscape, which directs our attention away from other heterogeneities characteristic of the EU (initial economic circumstances and ethnic, linguistic, and religious diversities).
By revealing the research gap in the climate economic literature when considering the within-country differences, the novelty of the paper lies in the examination of the relationship between the changes in air temperature over recent decades and the economic performance of the EU countries at the NUTS 3 level regions, which are territorially bounded areas usually smaller than the area of the state. However, the number of NUTS 3 regions in the country depends on the population size. Using the NUTS 3 classification allows us to consider within-country differences, pointing to the geography in terms of countries’ largeness and the elevation among their regions, corresponding to different temperatures within the country. Our sample includes 1,200 NUTS 3 regions of the EU countries, while we assume that temperature also affects production at the regional level (Kalkuhl and Wenz, 2020). Furthermore, the various impacts of air temperatures in different regions are also considered by Colacito et al. (2019). In addition to the analysis provided at the NUTS 3 level, the paper’s novelty also lies in focusing on temperature variations using the Cooling index, a weather-based technical measure designed to describe the need for air cooling (or air conditioning) instead of expressing the air temperatures by standard metrics (as °C).
In this research, we conduct a regression analysis to examine the relationship between changes in air temperature and the economic growth of EU countries. When working with longitudinal data composed of cross-sectional and time-series data, the panel data approach is applied (similar to the approach used by Dell et al., 2014; Hsiang, 2016; Colacito et al., 2019).
Emphasizing the novelty of this research, the cross-sectional units are presented by NUTS 3 regions. These areas are defined by the classification based on the population size known as NUTS (Nomenclature of Territorial Units for Statistics) classification from the methodology described by Eurostat (2024). At the NUTS 3 level, there are small regions for specific diagnoses, with a population ranging from 150,000 to 800,000. There are significant differences among countries in terms of their size (population) within the EU, so different numbers of NUTS 3 regions are observed.
Data are collected for the time period 2000–2022. This period witnesses the hottest decade in the EU (between 2011 and 2020) (European Commission, undated; Patterson, 2023). From the economic point of view, it covers two serious external shocks that might affect the economic growth of the EU countries. The choice of the period is also influenced by the data availability.
The main source of data is the Eurostat Database, which also provides data on regional statistics by NUTS classification. Since the number of NUTS 3 regions differs in individual sub-sections of the Eurostat database, we include those NUTS 3 regions in the final sample, which are present in all considered data tables. The final sample covers 1,200 NUTS 3 regions of EU countries. The distribution of the NUTS 3 regions across the EU is projected in Figure 1.
When analyzing the relationship between increasing air temperatures in the EU countries and their economic performance at the NUTS 3 level, we express the dependent research variable as the growth of Gross Domestic Product per capita at current market prices (hereafter labeled as gGDPpc). It is considered a standard measure of economic growth (Sala-i-Martin, 1997; Checherita-Westphal and Rother, 2012).
In the literature on the economics of climate change, the variables are usually expressed in terms of greenhouse gases, fossil fuels, temperature variations, and natural disasters or extreme weather events (Hsiang, 2016; Auffhammer, 2018; Agarwala et al., 2021; Kahn et al., 2021; Kotz et al., 2022; Petrovic, 2023). In this research, we also use the variable focusing on temperature variations. We use Eurostat’s Cooling index as an explanatory variable, which is calculated from the base temperature (the highest daily average air temperature at which it is not necessary to cool the air) (Eurostat, 2024). The index expresses the severity of the heat at a specific time, taking into consideration the outdoor temperature and average room temperature (the need for cooling). Eurostat performs these calculations daily, and they are added up to a calendar month and calendar year. Annual data are calculated as the sum of monthly data.
In the literature on economic growth determinants, various variables are employed. As mentioned by Petrovic (2023), their selection and use are in general arbitrary because the growth theories do not provide answers on the use of explanatory variables. However, an exhaustive review of economic growth determinants is provided by Sala-i-Martin (1997). In this paper, the selection of control variables or economic growth determinants is strongly related to limited data availability at the NUTS 3 level. However, we focus on all available data provided by the Eurostat database at the NUTS 3 level (Eurostat, 2024) that correspond to the economic growth determinants mentioned by Sala-i-Martin (1997) and many other authors such as Barro (2003); Eliot (2012); Checherita-Westphal and Rother (2012); Fetahi-Vehapi et al. (2015); Simionescu et al. (2017); and Rapacki and Próchniak (2019). To describe the socio-economic conditions of the regions at the NUTS 3 level of the countries in the sample, we draw inspiration from the above-mentioned authors and employ variables such as the size of the population, natural change of population, live births, and employed persons. To capture the size of the cross-sectional unit/region, we refer to the land area variable, as suggested by Brito (2015), while the population size is also considered in relation to the country’s size.
We also included a variable referring to the external shocks observed during the monitored period, labeled Crises. This variable is constructed as a dummy binary variable, taking the value 1 in the crisis period and 0 otherwise. When considering the Global Financial Crisis (GFC), it has affected the economic conditions in EU countries since 2009, as mentioned by FitzGerald (2013), Amstad and Packer (2015), and Rapacki and Próchniak (2019). The literature on the GFC mentions 2012 as the year of economic recovery (Paulus et al., 2017; Makrevska Disoska et al., 2020). For this reason, in the context of GFC, we set a value of 1 to the years 2009, 2010, and 2011. During the period monitored in this paper, another crisis occurred. The multicrisis, starting with the COVID-19 crisis in 2020 in the EU and followed by the energy crisis and war conflict in Ukraine, affected the economic activity of EU countries (Ingham, 2023). The threat of economic downturn lasted several years, and according to the Center on Budget and Policy Priorities (CBPP), the economies began recovering in 2023 (CBPP, 2024). For this reason, in the context of multicrisis, we set a value of 1 to 2020, 2021, and 2022, while 2023 is not covered in our sample.
As Sala-i-Martin (1997), Bassanini and Scarpetta (2001), and Barro (2003) mentioned other determinants of economic growth related to government expenditure, fiscal policy, inflation, and financial systems, we also employ variables such as government expenditure, inflation rate, and interest rates into our research. In the case of government expenditure, in line with the Keynesian approach, a positive relationship between government expenditure and economic growth is expected (Parui, 2020; Buthelezi, 2023). However, discussing the relationship in the question invokes a lack of consensus among researchers and admits both positive and negative influences (IMF, 1995; Arawatari et al., 2023; Buthelezi, 2023). The related literature distinguished between developed and developing countries, while the positive impact of government expenditure on economic growth is present in developing countries (IMF, 1995; Poku et al., 2022; Rahman et al., 2023). In developed countries, Loizides and Vamvoukas (2005) also observed a positive relationship, while Arawatari et al. (2023) observed a non-linear relationship between government expenditure and economic growth. Arawatari et al. (2023) discussed the composition of public expenditure, aligning their findings with those of IMF (1995) and Bassanini and Scarpetta (2001), particularly focusing on government expenditure on research and development as a focal point of their interest.
The literature on the impact of the inflation rate on economic growth also provides conflicting results (Batrancea et al., 2022). Rodríguez-Pose and Krøijer (2009) and Thießen (2003) observed a negative relationship between the inflation rate and economic growth, while Davoodi and Zou (1998) observed a positive relationship. Further related research discussed the non-linear relationship (Aydın et al., 2016; Ekinci et al., 2020), concluding that the negative impact of the inflation rate on economic growth is observed above a certain threshold; below this threshold, it is either not significant (Ekinci et al., 2020) or positive (Aydın et al., 2016).
The impact of financial systems on economic growth, considering the interest rates, is analyzed (Petkovski and Kjosevski, 2014; Shaukat et al., 2019; Batrancea et al., 2022). The negative impact of interest rates on economic growth is expected because higher interest rates discourage taking out loans to fund new investment projects. This is observed by Petkovski and Kjosevski (2014) and Shaukat et al. (2019).
The dependent variable (gGDPpc), the main research variable, explanatory variable (Cooling index), and control variables (live births, natural change, land area, employed persons, and population) are sourced from the Eurostat databases, except for the binary variable crises. The labeling, characteristics, and source of data on the variables included in the research are listed in Table 1.
We conduct an econometric regression on panel data to examine the relationship between the Cooling index and gGDPpc. We consider several estimation techniques.
In the first step, we run static panel data models, the fixed-effect model (FEM), and the random-effect model (REM), which differ in an assumption that in FEM, individual differences between countries are expressed by their own fixed intercepts. In this research, in both FEM and REM, the estimates are adjusted by heteroskedasticity and autocorrelation consistent (HAC) covariance matrix estimation proposed by Arellano (2003). In the REM, the Swamy–Arora/Baltagi and Chang transformation for unbalanced panels is applied (Baltagi and Chang, 1994). REM is tested against FEM using the Hausman test (Hausman, 1978).
For static panel data models, we use Equation 1 for FEM and Equation 2 for REM:
where
For robustness checks and to deal with potential endogeneity in the model, in the second step, we run the dynamic panel data models based on the GMM estimator (GMM, generalized method of moments). In addition to the default first difference GMM estimator introduced by Arellano and Bond (1991), we also run the dynamic panel data regression based on the system GMM estimator (introduced by Arellano and Bover (1995); Blundell and Bond (1998)) level equations. As mentioned by Roodman (2009), in panels with small T (time period) and large N (number of cross-sectional units), the use of the GMM system estimator is proposed due to its higher efficiency compared to the first difference GMM estimator. The dynamic panel data models are tested for first- and second-order serial correlation (Arellano–Bond tests for first- and second-order serial correlation, Roodman, 2009), and the validity of the instruments is assessed using the Sargan test (Sargan, 1958). These models help control for unobserved heterogeneity and potential endogeneity, providing robust estimates of the impact of temperature changes on GDP. Two types of tests are provided to test the collinearity: the Belsley, Kuh, and Welsch collinearity diagnostics (Belsley et al., 1980) and the variance inflation factor test of collinearity (Neter et al., 1990).
For dynamic panel data models, we use Equation 3:
where
Table 2 provides an overview of the descriptive statistics of the analyzed variables, including the dependent variable (gGDPpc), the explanatory variable (Cooling index), and all control variables used in the following regression analyses.
To demonstrate the changes in the air temperatures using the Cooling index, Figures 2, 3 are projected. In Figures 2, 3, we graphically display the values of the Cooling index at the NUTS 3 level across the EU countries during the period 2000–2022, where we divided the analyzed period into four shorter periods (2000–2005, 2006–2011, 2012–2017, and 2018–2022). In these sub-periods, we calculated the average values of the index for individual regions according to the NUTS 3 classification and displayed the values in the following maps.
Figure 2. Average values of the Cooling index in countries of the European Union (period 2000–2005, left side; period 2006–2011, right side). Source: own processing.
Figure 3. Average values of the Cooling index in countries of the European Union (period 2012–2017, left side; period 2018–2022, right side). Source: own processing.
From the displayed maps, it is possible to observe increasing average values of the index in the NUTS 3 regions of the EU (more dark areas on maps showing later periods (Figure 3) compared to maps capturing earlier periods (Figure 2)).
As mentioned before, the main goal of the paper is to analyze the relationship between the variable referring to the air temperature variations (measured by the Cooling index) and the economic performance of the country (measured by the growth of the GDP per capita) considering the NUTS 3 level, which allows considering the within-country differences in climate conditions. For this purpose, we conduct the regression analyses, the results of which are provided in Tables 3, 4. When examining the relationship between the mentioned variables, panel estimation techniques are used in line with related research (Dell et al., 2014; Hsiang, 2016; Colacito et al., 2019).
In the first step of the analysis, FEM and REM estimations were conducted. When including all the variables mentioned in Table 1 into the estimations, the problem of multicollinearity was detected. For this reason, several control variables, such as the natural change of population and population, were excluded from the final estimations. For the same reason, in the case of the variable on employed persons, the logs are used. The results of FEM and REM are displayed in Table 3. However, the Hausman test suggests the adequacy of the FEM against the REM.
A statistically significant negative relationship between the Cooling index and gGDPpc is observed in both models (see Table 3). The increase in the need for cooling causes a decrease in the GDPpc growth. The result that GDP tends to decrease as temperatures increase supports the hypothesis that higher temperatures would decrease economic performance. Our main finding is in line with the research assumption of an inverse relationship between air warming and GDP per capita growth based on the findings of Hsiang (2010); Burke et al. (2015); Colacito et al. (2019); Kalkuhl and Wenz (2020); and Kahn et al. (2021).
Regarding the control variables, the results correspond to the related research findings on the economic growth determinants.
The variable number of live births, reflecting the birth rate, has a negative and significant effect on GDPpc growth. These findings suggest that higher birth rates are associated with lower growth of the GDP per capita, potentially due to the increased economic burden of higher dependency rates. According to Eliot (2012), increased live births mirrored in the increased fertility rate indicate that the correspondent part of female individuals is not included in the production process. However, the negative impact of higher fertility rates on economic growth is observed by Barro (2003). The negative impact of population growth on economic performance is also mentioned by Checherita-Westphal and Rother (2012) and Rapacki and Próchniak (2019).
The level of employment, captured by the variable number of employed people, has a positive and significant effect on the GDPpc growth in both models, indicating that a higher level of employment contributes to a higher growth of the GDP per capita; this highlights the key role of the labor market in economic growth. Our findings are in line with the findings of Simionescu et al. (2017), while the effect of the labor force is also discussed by Eliot (2012) and Rapacki and Próchniak (2019).
The effect of land area on GDP is mixed. The coefficient in the FEM model indicates that land area has no effect on the GDPpc growth but is not statistically significant. In the REM model, the coefficient is positive and statistically significant, indicating that larger land areas are associated with higher GDP per capita growth in this model. Relating to this, Brito (2015) mentioned that smaller countries (measured by their population and area) are more vulnerable to external shocks and disasters, often leading to lower levels of GDP. Although the same author admits that there are certain exceptions where small countries perform at high levels of GDP per capita, in general, better economic performance tends to be associated with larger countries. Our findings also confirm this assumption.
Our findings show the obvious statistically significant negative effect of the variable referring to crises on GDPpc growth. This effect is present in both models, in line with the findings of FitzGerald (2013); Amstad and Packer (2015); Rapacki and Próchniak (2019); and Ingham (2023). This consistent result underlines the harmful impact of economic crises on GDP and highlights the importance of economic stability for growth.
The results on the relationship between inflation and economic growth show a positive effect in line with the findings of Davoodi and Zou (1998) and Aydın et al. (2016) but contrary to those of Thießen (2003) and Rodríguez-Pose and Krøijer (2009).
In conducted estimations (FEM and REM), the impact of government expenditure on economic growth is mixed. In the case of FEM, the estimate of the variable is positive. In the case of REM, it is negative. Although the Hausman test suggests the adequacy of the FEM against the REM, the results are in line with the Keynesian approach about a positive relationship between government expenditure and economic growth, as observed by Parui (2020) and Buthelezi (2023). A statistically significant negative estimate of the interest rate variable confirms the assumption regarding the detrimental effect of increasing interest rates on economic growth, as observed by Petkovski and Kjosevski (2014) and Shaukat et al. (2019).
Subsequently, we continued with dynamic panel estimations based on GMM and system GMM estimators (see Table 4). At a glance, results of dynamic panel data models, like results of FEM and REM estimations (see Table 3), confirm the expectations about the inverse relationship between the Cooling index and GDPpc growth in line with the findings of a myriad of studies, as mentioned by Hsiang (2010); Burke et al. (2015); Colacito et al. (2019); Kalkuhl and Wenz (2020); and Kahn et al. (2021). However, the model based on the first-difference GMM estimator experiences second-order serial correlation (the p-value of the AR 2 test is lower than the standard significance level; Roodman, 2009), and its results are thus misleading. When considering the results of the dynamic panel data model based on the system GMM estimator, the findings concerning the main explanatory variable (Cooling index) and control variable correspond to those observed in the case of FEM and REM estimations. The variable on crises has a statistically significant negative impact on GDPpc growth, as assumed (FitzGerald, 2013; Amstad and Packer, 2015; Rapacki and Próchniak, 2019; Ingham, 2023). The variable referring to live births shows a statistically significant negative relationship with GDPpc growth, similar to the results observed by Barro (2003); Eliot (2012); Checherita-Westphal and Rother (2012); and Rapacki and Próchniak (2019). The level of employment measured by the variable employed persons shows a statistically significant positive impact on the GDPpc growth in line with statements and findings mentioned by Eliot (2012), Simionescu et al. (2017), and Rapacki and Próchniak (2019). These results point to the decisive role of employment in managing economic growth. The land area variable, representing the country’s area, has a statistically significant positive effect on the economic performance of NUTS 3 regions of EU countries, confirming the expectations mentioned by Brito (2015). The inflation rate in the dynamic panel data model based on the system GMM estimator is not statistically significant, as observed by Ekinci et al. (2020). In the same estimation, the impact of government expenditure on economic growth is negative, contrary to the results of previous FEM estimation, signaling the potential problem of endogeneity in a static model. Furthermore, focusing on the composition of government expenditure (as proposed by IMF (1995) and Bassanini and Scarpetta (2001) might help solve the puzzling problem. In a system GMM model, a statistically significant negative estimate of the interest rates variable confirms the assumption about the detrimental effect of increasing interest rates on economic growth, as observed by Petkovski and Kjosevski (2014) and Shaukat et al. (2019).
Unfortunately, in the model using the system GMM estimator, as well as in the model using the GMM estimator, the results of the Sargan test signalize the problems of the model specification mentioned by Petrovic (2023) referring to Sala-i-Martin (1997). They point to the problems of model misspecification when certain further economic growth determinants are omitted. In our case, as mentioned before, we face the problem with data on potential determinants at the NUTS 3 level. However, our findings are in line with the related literature on climate-economic models and models on economic growth determinants.
This research provides a thorough analysis of the impact of increasing air temperature, which is one of the most significant representations of global warming, on the economic production of the countries of the EU. Observed results support the assumptions given by the literature on climate-economic models. We found out that observed air temperature changes have a statistically significant impact on the production of the economies, which together affect the overall economic productivity of the EU at the NUTS 3 level regions.
The results of this paper are important for academia, society, and policymakers. The observed results of our research do not only confirm the hypothesis of the negative impact of increasing air temperatures measured using the Cooling index on the growth of the gross domestic product per capita but also point to the presence of the need for adaptation of countries and their individual regions to mitigate the negative impact of climate change.
For this reason, further public policies in the field of climate change should cover the strategy at the national level and regional/local level of the government. Policymakers should consider the within-country differences in geographical landscapes and, by respecting the principle of subsidiarity, allow regional/local authorities to address place-based adaptations to climate changes and related risks. Lower government levels are not only closer to citizens but also closer to the local environment, with a better understanding of the local needs, specifics, and preferences. The decentralization of competencies in environmental protection is present in the EU countries, and in this context, the regions/localities should also prepare themselves for the transformation toward sustainable regions/cities. However, vertical fiscal imbalance worsens the fiscal position of lower government levels compared to the national government and thus hinders green project investments at the regional/local level. To deal with this problem, the national government should support the lower levels of government financially, if possible, and in other fields. Other forms of support should come from expertise services as lower levels of government may lack professional capacities to address specific solutions. Some issues that could be solved at the national level, although they damage regional/local landscapes, are negative externalities produced outside the region/locality but affecting regional/local conditions. Equally important is considering the unequal distribution of natural resources and industry across countries, which affects regional/local conditions, but is often not a result of activities within the regions/localities, especially in small open economies with foreign direct investments, which make up a non-negligible part of EU countries.
This paper focuses on the relationship between the changes in air temperature over recent decades and the economic performance of the EU countries. The unique approach of the paper lies in the analysis conducted on NUTS 3 regions and using the Cooling index. We use the NUTS 3 regions, aiming to capture the within-country differences in air temperatures and economic growth at the regional level because of evident differences in the geographical landscape—elevation and climate. The Cooling index is a weather-based technical measure designed to describe the need for air cooling (or air conditioning) constructed by the EU. We conduct a regression analysis on the panel of 1,200 units at the NUTS 3 level in the period from 2000 to 2022, employing the FEM, REM, and dynamic panel data model using the GMM and GMM system estimators.
The results confirm the expectation about the inverse relationship between air temperatures and economic performance measured as GDP per capita growth. They support efforts to address the adaptation needs of individual regions when mitigating these changes. This result suggests that the adverse effects of increasing temperatures, such as increased energy costs, health impacts, and productivity losses, outweigh any potential economic benefits.
Limitations to the research lie in the complexity of the analyzed period. Observed increases in the air temperature were obvious in the second decade of the 21st century, and economic turbulences almost simultaneously might bias the results. Thus, it would be beneficial to consider longer periods. However, the availability of data at the NUTS 3 level is limited, and some datasets have only been produced in Eurostat since 2014. Another limitation to the research is that the effects of climate change are only expressed through the Cooling index, related to the air temperature. Climate change also brings other changes in weather, such as disasters like droughts and floods. They can also have an impact on the economic performance of affected regions.
Despite this, the results contribute to the climate economic literature and can serve as a basis for further research and the creation of regional/local policies, aiming to improve economic performance through analyses in the field of global warming. Policymakers, as well as further research, should consider these findings when designing strategies to mitigate the economic impacts of climate change for particular regions and identify adaptive measures to counteract these effects. Although climate change is usually discussed as a global topic, further research should focus on place-based adaptations to climate changes and risks due to within-country differences in geographical landscapes. National governments should consider decentralizing the competencies in environmental fields to lower government levels, which are not only closer to citizens but also closer to the local environment, with a better understanding of the local needs and local specifics.
Publicly available datasets were analyzed in this study. These data can be found at: Eurostat database, Eurostat (2024)—https://ec.europa.eu/eurostat/data/database.
DH: project administration, validation, and writing–review and editing. DJ: data curation, formal analysis, writing–review and editing. LM: formal analysis, funding acquisition, resources, supervision, and writing–review and editing. JV: conceptualization, data curation, investigation, methodology, software, visualization, and writing–original draft.
The author(s) declare that no financial support was received for the research, authorship, and/or publication of this article.
The article is published with the support of the Scientific Grant Agency of the Ministry of Education, Science, Research, and Sport of the SR and the Slovak Academy of Sciences within the projects 1/0681/22 and 1/0646/23 and internal grant for PhD. students granted by the Economic faculty of the Technical University of Košice.
The authors declare that the research was conducted in the absence of any commercial or financial relationships that could be construed as a potential conflict of interest.
All claims expressed in this article are solely those of the authors and do not necessarily represent those of their affiliated organizations, or those of the publisher, the editors, and the reviewers. Any product that may be evaluated in this article, or claim that may be made by its manufacturer, is not guaranteed or endorsed by the publisher.
Acevedo Mejia, S., and Acevedo Mejia, S. (2014). Debt, growth and natural disasters A caribbean trilogy. IMF Work. Pap. 14, 1. doi:10.5089/9781498337601.001
Acharya, V., Drechsler, I., and Schnabl, P. (2014). A pyrrhic victory? Bank bailouts and sovereign credit risk. J. Finance 69, 2689–2739. doi:10.1111/jofi.12206
Agarwala, M., Burke, M., Klusak, P., Mohaddes, K., Volz, U., and Zenghelis, D. (2021). Climate change and fiscal sustainability: risks and opportunities. nier 258, 28–46. doi:10.1017/nie.2021.37
Amstad, M., and Packer, F. (2015). Sovereign ratings of advanced and emerging economies after the crisis. BIS Q. Rev. Bank for International Settlements.
Arawatari, R., Hori, T., and Mino, K. (2023). Government expenditure and economic growth: a heterogeneous-agents approach. J. Macroecon. 75, 103486. doi:10.1016/j.jmacro.2022.103486
Arellano, M. (2003). Panel data econometrics. 1st Edn. Oxford University PressOxford. doi:10.1093/0199245282.001.0001
Arellano, M., and Bond, S. (1991). Some tests of specification for panel data: Monte Carlo evidence and an application to employment equations. Rev. Econ. Stud. 58, 277. doi:10.2307/2297968
Arellano, M., and Bover, O. (1995). Another look at the instrumental variable estimation of error-components models. J. Econ. 68, 29–51. doi:10.1016/0304-4076(94)01642-D
Auffhammer, M. (2018). Quantifying economic damages from climate change. J. Econ. Perspect. 32, 33–52. doi:10.1257/jep.32.4.33
Aydın, C., Esen, Ö., and Bayrak, M. (2016). Inflation and economic growth: a dynamic panel threshold analysis for Turkish republics in transition process. Procedia - Soc. Behav. Sci. 229, 196–205. doi:10.1016/j.sbspro.2016.07.129
Baltagi, B. H., and Chang, Y.-J. (1994). Incomplete panels. J. Econ. 62, 67–89. doi:10.1016/0304-4076(94)90017-5
Barrios, S., Bertinelli, L., and Strobl, E. (2010). Trends in rainfall and economic growth in africa: a neglected cause of the african growth tragedy. Rev. Econ. Statistics 92, 350–366. doi:10.1162/rest.2010.11212
Barro, R. J. (2003). Determinants of economic growth in a panel of countries. Ann. Econ. Finance 4, 231–274.
Bassanini, A., and Scarpetta, S. (2001). The driving forces of economic growth: panel data evidence for the OECD countries. OECD Econ. Stud. 33, 9–56. doi:10.1787/16097491
Batrancea, L., Rathnaswamy, M. K., and Batrancea, I. (2022). A panel data analysis on determinants of economic growth in seven non-BCBS countries. J. Knowl. Econ. 13, 1651–1665. doi:10.1007/s13132-021-00785-y
Beirne, J., Renzhi, N., and Volz, U. (2021). Feeling the heat: climate risks and the cost of sovereign borrowing. Int. Rev. Econ. and Finance 76, 920–936. doi:10.1016/j.iref.2021.06.019
Belsley, D. A., Kuh, E., and Welsch, R. E. (1980). Regression diagnostics: identifying influential data and sources of collinearity. 1st Edn. Wiley. doi:10.1002/0471725153
Blundell, R., and Bond, S. (1998). Initial conditions and moment restrictions in dynamic panel data models. J. Econ. 87, 115–143. doi:10.1016/S0304-4076(98)00009-8
Bouchet, M. H., Fishkin, C. A., and Goguel, A. (2018). Managing country risk in an age of globalization: a practical guide to overcoming challenges in a complex world. Cham: Springer International Publishing. doi:10.1007/978-3-319-89752-3
Brito, J. (2015). Country size and determinants of economic growth: a survey with special interest on small states. Germany: University Library of Munich. MPRA Paper No. 61273.
Burke, M., Davis, W. M., and Diffenbaugh, N. S. (2018). Large potential reduction in economic damages under UN mitigation targets. Nature 557, 549–553. doi:10.1038/s41586-018-0071-9
Burke, M., Hsiang, S. M., and Miguel, E. (2015). Global non-linear effect of temperature on economic production. Nature 527, 235–239. doi:10.1038/nature15725
Buthelezi, E. M. (2023). Impact of government expenditure on economic growth in different states in South Africa. Cogent Econ. and Finance 11. doi:10.1080/23322039.2023.2209959
Cashin, P., Mohaddes, K., and Raissi, M. (2017). Fair weather or foul? The macroeconomic effects of El Niño. J. Int. Econ. 106, 37–54. doi:10.1016/j.jinteco.2017.01.010
Cattaneo, C., and Peri, G. (2016). The migration response to increasing temperatures. J. Dev. Econ. 122, 127–146. doi:10.1016/j.jdeveco.2016.05.004
CBPP (2024). Chart book: tracking the recovery from the pandemic recession. Cent. Budg. Policy Priorities. Available at: https://www.cbpp.org/research/economy/tracking-the-recovery-from-the-pandemic-recession.
Checherita-Westphal, C., and Rother, P. (2012). The impact of high government debt on economic growth and its channels: an empirical investigation for the euro area. Eur. Econ. Rev. 56, 1392–1405. doi:10.1016/j.euroecorev.2012.06.007
Colacito, R., Hoffmann, B., and Phan, T. (2019). Temperature and growth: a panel analysis of the United States. J. Money Credit Bank. 51, 313–368. doi:10.1111/jmcb.12574
DARA. (2012) Climate vulnerable forum. Clim. Vulnerability Monit. Available at: https://daraint.org/wp-content/uploads/2012/09/CVM2ndEd-FrontMatter.pdf [Accessed May 26, 2024].
Dasgupta, S., Van Maanen, N., Gosling, S. N., Piontek, F., Otto, C., and Schleussner, C.-F. (2021). Effects of climate change on combined labour productivity and supply: an empirical, multi-model study. Lancet Planet. Health 5, e455–e465. doi:10.1016/S2542-5196(21)00170-4
Davoodi, H., and Zou, H. F. (1998). Fiscal decentralization and economic growth: a crosscountry study. J. Urban Econ. 43, 244–257. doi:10.1006/juec.1997.2042
Day, E., Fankhauser, S., Kingsmill, N., Costa, H., and Mavrogianni, A. (2019). Upholding labour productivity under climate change: an assessment of adaptation options. Clim. Policy 19, 367–385. doi:10.1080/14693062.2018.1517640
Dell, M., Jones, B. F., and Olken, B. A. (2012). Temperature shocks and economic growth: evidence from the last half century. Am. Econ. J. Macroecon. 4, 66–95. doi:10.1257/mac.4.3.66
Dell, M., Jones, B. F., and Olken, B. A. (2014). What do we learn from the weather? The new climate-economy literature. J. Econ. Literature 52, 740–798. doi:10.1257/jel.52.3.740
Deryugina, T. (2011). The dynamic effects of hurricanes in the US: the role of non-disaster transfer payments. MIT Cent. Energy Environ. Policy Res.
Deryugina, T., and Hsiang, S. (2014). Does the environment still matter? Daily temperature and income in the United States. Cambridge, MA: National Bureau of Economic Research. doi:10.3386/w20750
Deryugina, T., and Hsiang, S. (2017). The marginal product of climate. Cambridge, MA: National Bureau of Economic Research. doi:10.3386/w24072
Ekinci, R., Tuzun, O., and Ceylan, F. (2020). The relationship between inflation and economic growth: experiences of some inflation targeting countries. Stud. Financ. Financ. Stud. 24, 6–20.
Eliot, T. S. (2012). “Determinants of economic growth,” in Devaluing to prosperity: misaligned currencies and their growth consequence. Editor S. S. Bhalla (Washington, DC: Peterson Institute for International Economics), 11–32. Available at: https://climate.ec.europa.eu/climate-change/causes-climate-change_en.
Eurostat (2024). Eurostat database. Brussels: European Commission. Available at: https://ec.europa.eu/eurostat/statistics-explained/index.php?title=Heating_and_cooling_degree_days_-_statistics#Heating_and_cooling_degree_days_by_NUTS_3_regions (Accessed May 26, 2024).
Fankhauser, S., and Tol, S. J. (2005). On climate change and economic growth. Resour. Energy Econ. 27, 1–17. doi:10.1016/j.reseneeco.2004.03.003
Farhi, E., and Gabaix, X. (2016). Rare disasters and exchange rates. Q. J. Econ. 131, 1–52. doi:10.1093/qje/qjv040
Farhi, E., and Tirole, J. (2018). Deadly embrace: sovereign and financial balance sheets doom loops. Rev. Econ. Stud. 85, 1781–1823. doi:10.1093/restud/rdx059
Fetahi-Vehapi, M., Sadiku, L., and Petkovski, M. (2015). Empirical analysis of the effects of trade openness on economic growth: an evidence for south east European countries. Procedia Econ. Finance 19, 17–26. doi:10.1016/S2212-5671(15)00004-0
FitzGerald, J. (2013). Financial crisis, economic adjustment and a return to growth in the EU. Rev. l’OFCE N° 127, 275–302. doi:10.3917/reof.127.0275
Gennaioli, N., Martin, A., and Rossi, S. (2018). Banks, government bonds, and default: what do the data say? J. Monetary Econ. 98, 98–113. doi:10.1016/j.jmoneco.2018.04.011
Hausman, J. A. (1978). Specification tests in econometrics. Econometrica 46, 1251. doi:10.2307/1913827
Hsiang, S. M. (2010). Temperatures and cyclones strongly associated with economic production in the Caribbean and Central America. Proc. Natl. Acad. Sci. U.S.A. 107, 15367–15372. doi:10.1073/pnas.1009510107
Hsiang, S. M. (2016). Climate econometrics. Annu. Rev. Resour. Econ. 8, 43–75. doi:10.1146/annurev-resource-100815-095343
Hsiang, S. M., and Narita, D. (2012). Adaptation to cyclone risk: evidence from the global cross-section. Clim. Change Econ. 03, 1250011. doi:10.1142/S201000781250011X
IMF (1995). “Unproductive public expenditures A pragmatic approach to policy analysis,” in Pamphlet series No. 48 (1995). Washington, D.C: International Monetary Fund. Available at: https://www.imf.org/external/pubs/ft/pam/pam48/pam4803.htm.
Ingham, H. (2023). COVID-19, the Great recession and economic recovery: a tale of two crises. J. Common Mark. Stud. 61, 469–485. doi:10.1111/jcms.13383
Intergovernmental Panel on Climate Change (2014). in Climate change 2014: impacts, adaptation, and vulnerability: working Group II contribution to the fifth assessment report of the Intergovernmental Panel on Climate Change. Editors C. B. Field, and V. R. Barros (New York, NY: Cambridge University Press).
Kahn, M. E., Mohaddes, K., Ng, R. N. C., Pesaran, M. H., Raissi, M., and Yang, J.-C. (2021). Long-term macroeconomic effects of climate change: a cross-country analysis. Energy Econ. 104, 105624. doi:10.1016/j.eneco.2021.105624
Kalkuhl, M., and Wenz, L. (2020). The impact of climate conditions on economic production. Evidence from a global panel of regions. J. Environ. Econ. Manage. 103, 102360. doi:10.1016/j.jeem.2020.102360
Koetsier, I. (2017). “The fiscal impact of natural disasters,” in Tjalling C. Koopmans research Institute discussion paper 17-17. Utrecht: Utrecht University.
Kotz, M., Levermann, A., and Wenz, L. (2022). The effect of rainfall changes on economic production. Nature 601, 223–227. doi:10.1038/s41586-021-04283-8
Kotz, M., Wenz, L., Stechemesser, A., Kalkuhl, M., and Levermann, A. (2021). Day-to-day temperature variability reduces economic growth. Nat. Clim. Chang. 11, 319–325. doi:10.1038/s41558-020-00985-5
Loizides, J., and Vamvoukas, G. (2005). Government expenditure and economic growth: evidence from trivariate causality testing. J. Appl. Econ. 8, 125–152. doi:10.1080/15140326.2005.12040621
Makrevska Disoska, E., Tevdovski, D., Toshevska-Trpchevska, K., and Stojkoski, V. (2020). Evidence of innovation performance in the period of economic recovery in Europe. Innovation Eur. J. Soc. Sci. Res. 33, 280–295. doi:10.1080/13511610.2018.1524288
Neter, J., Wasserman, W., and Kutner, M. H. (1990). Applied linear statistical models. 3rd Edn. Boca Raton, FL: CRC Press.
Nordhaus, W., and Moffat, A. (2017). A survey of global impacts of climate change: replication, survey methods, and a statistical analysis. Cambridge, MA: National Bureau of Economic Research. doi:10.3386/w23646
Parui, P. (2020). Government expenditure and economic growth: a post-Keynesian analysis. Int. Rev. Appl. Econ. 35, 597–625. doi:10.1080/02692171.2020.1837744
Patterson, M. (2023). North-West Europe hottest days are warming twice as fast as mean summer days. Geophys. Res. Lett. 50, e2023GL102757. doi:10.1029/2023GL102757
Paulus, A., Figari, F., and Sutherland, H. (2017). The design of fiscal consolidation measures in the European Union: distributional effects and implications for macro-economic recovery. Oxf. Econ. Pap. 69, gpw054–654. doi:10.1093/oep/gpw054
Petkovski, M., and Kjosevski, J. (2014). Does banking sector development promote economic growth? An empirical analysis for selected countries in Central and South Eastern Europe. Econ. Research-Ekonomska Istraživanja 27, 55–66. doi:10.1080/1331677X.2014.947107
Petrovic, P. (2023). Climate change and economic growth: plug-in model averaging approach. J. Clean. Prod. 433, 139766. doi:10.1016/j.jclepro.2023.139766
Poku, K., Opoku, E., and Agyeiwaa Ennin, P. (2022). The influence of government expenditure on economic growth in Ghana: an Ardl approach. Cogent Econ. and Finance 10. doi:10.1080/23322039.2022.2160036
Rahman, M., Nath, S., Siddqu, M., and Hossain, S. (2023). The impact of government expenditure on economic growth: a study of saarc countries. Open J. Bus. Manag. 11, 1691–1703. doi:10.4236/ojbm.2023.114095
Rapacki, R., and Próchniak, M. (2019). EU membership and economic growth: empirical evidence for the CEE countries. Eur. J. Comp. Econ. 16 (1), 3–40. doi:10.25428/1824-2979/201901-3-40
Rodríguez-Pose, A., and Krøijer, A. (2009). Fiscal decentralization and economic growth in central and eastern Europe. London: The London School of Economics and Political Science.
Roodman, D. (2009). A note on the theme of too many instruments. Oxf. Bull. Econ. Stat. 71, 135–158. doi:10.1111/j.1468-0084.2008.00542.x
Sargan, J. D. (1958). The estimation of economic relationships using instrumental variables. Econometrica 26, 393. doi:10.2307/1907619
Schuler, P., Oliviera, L. E., Mele, G., and Antonio, M. (2018). Managing the fiscal risks associated with natural disasters. Washington, DC: World Bank. doi:10.1596/978-1-4648-1358-0
Semieniuk, G., Campiglio, E., Mercure, J., Volz, U., and Edwards, N. R. (2021). Low-carbon transition risks for finance. WIREs Clim. Change 12, e678. doi:10.1002/wcc.678
Shaukat, B., Zhu, Q., and Ijaz Khan, M. (2019). Real interest rate and economic growth: a statistical exploration for transitory economies. Phys. A Stat. Mech. its Appl. 534 (122193), 122193. doi:10.1016/j.physa.2019.122193
Simionescu, M., Lazányi, K., Sopková, G., Dobeš, K., and Balcerzak, A. P. (2017). Determinants of economic growth in V4 countries and Romania. J. Compet. 9, 103–116. doi:10.7441/joc.2017.01.07
Swiss Re (2021). World economy set to lose up to 18% GDP from climate change if no action taken, reveals Swiss Re Institute’s stress-test analysis. Zurich: Swiss Re. Available at: https://www.swissre.com/media/press-release/nr-20210422-economics-of-climate-change-risks.html.
Thießen, U. (2003). Fiscal decentralisation and economic growth in high income OECD countries. Fisc. Stud. 24, 237–274. doi:10.1111/j.1475-5890.2003.tb00084.x
Tol, R. S. J. (2012). On the uncertainty about the total economic impact of climate change. Environ. Resour. Econ. 53, 97–116. doi:10.1007/s10640-012-9549-3
United Nations (2018). Goal 13: take urgent action to combat climate change and its impacts. Available at: http://www.un.org/sustainabledevelopment/climate-change-2 (Accessed May 26, 2024).
Watson, K. P., Cross, R., and Jones, M. P. (2021). The winter storm of 2021. Houston: University of Houston. Available at: https://uh.edu/hobby/winter2021/storm.pdf (Accessed May 26, 2024).
Keywords: GDP, cooling index, NUTS3 regions, European Union, climate-economic model
Citation: Hadačová D, Jusková D, Maličká L and Vancová J (2025) The climate-economic model of 27 EU countries at the NUTS 3 level. Front. Environ. Sci. 12:1439927. doi: 10.3389/fenvs.2024.1439927
Received: 28 May 2024; Accepted: 27 November 2024;
Published: 07 January 2025.
Edited by:
Hai Hong Trinh, Massey University Business School, New ZealandReviewed by:
Nabila Khurshid, COMSATS University, Islamabad Campus, PakistanCopyright © 2025 Hadačová, Jusková, Maličká and Vancová. This is an open-access article distributed under the terms of the Creative Commons Attribution License (CC BY). The use, distribution or reproduction in other forums is permitted, provided the original author(s) and the copyright owner(s) are credited and that the original publication in this journal is cited, in accordance with accepted academic practice. No use, distribution or reproduction is permitted which does not comply with these terms.
*Correspondence: Dominika Jusková, ZG9taW5pa2EuanVza292YUB0dWtlLnNr
Disclaimer: All claims expressed in this article are solely those of the authors and do not necessarily represent those of their affiliated organizations, or those of the publisher, the editors and the reviewers. Any product that may be evaluated in this article or claim that may be made by its manufacturer is not guaranteed or endorsed by the publisher.
Research integrity at Frontiers
Learn more about the work of our research integrity team to safeguard the quality of each article we publish.