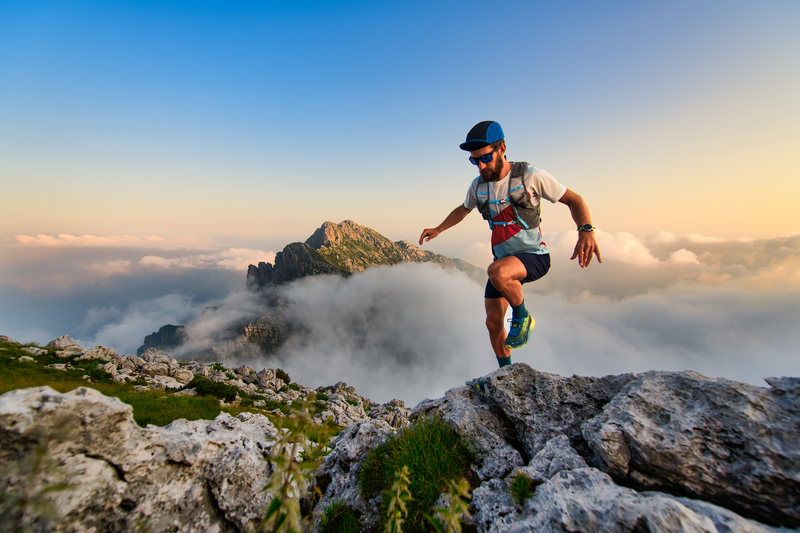
94% of researchers rate our articles as excellent or good
Learn more about the work of our research integrity team to safeguard the quality of each article we publish.
Find out more
REVIEW article
Front. Environ. Sci. , 06 September 2024
Sec. Interdisciplinary Climate Studies
Volume 12 - 2024 | https://doi.org/10.3389/fenvs.2024.1438262
Changes in wildfire regimes are of growing concern and raise issues about how well we can model risks in a changing climate. Process-based coupled fire-vegetation models, used to project future wildfire regimes, capture many aspects of wildfire regimes poorly. However, there is now a wealth of information from empirical studies on the climate, vegetation, topography and human activity controls on wildfire regimes. The measures used to quantify these controls vary among studies, but certain variables consistently emerge as the most important: gross primary production as a measure of fuel availability, vegetation cover as a measure of fuel continuity, and atmospheric humidity as a measure of fuel drying. Contrary to popular perception, ignitions are generally not a limiting factor for wildfires. In this review, we describe how empirical fire models implement wildfire processes, synthesise current understanding of the controls on wildfire extent and severity, and suggest ways in which fire modelling could be improved.
• Empirical analyses of the controls on wildfires consistently identify vegetation properties associated with fuel availability and continuity and climate factors associated with fuel drying as the most important influences on wildfire extent and severity.
• Ignitions, whether anthropogenic or natural, are generally not limiting.
• Fire size, burnt area and fire intensity are influenced by different factors; current relationships between these aspects of wildfire could become decoupled in an altered climate.
• Some hypotheses embedded in ‘process-based’ fire-vegetation models are inconsistent with empirical evidence, implying a need for a re-design.
Wildfires are unplanned fires that occur in natural ecosystems, although the ignition source can be natural (most often lightning) or anthropogenic. Wildfires occur on all vegetated continents. Current remotely-sensed based estimates suggest that something of the order of 2.6% ± 0.3% (GFED4: Giglio et al., 2013) to 5.9% ± 0.5% (GFED5: Chen et al., 2023) of the global vegetated area burns each year. Wildfires have been the most important cause of disturbance in natural ecosystems for millions of years (Scott, 2000; Scott and Glasspool, 2006; Harrison et al., 2010) and indeed are fundamental to the maintenance of many ecosystems and floras (Pausas and Keeley, 2009; Pausas et al., 2017; Harrison et al., 2021; Pausas and Keeley, 2023). The fire regime, the long-term characteristics of wildfires in specific environments (Lavorel et al., 2007; Archibald et al., 2018) as expressed in terms of fire size, intensity, extent or frequency, shapes ecosystem properties and plant reproductive traits. Some ecosystems rely on frequent wildfires for the establishment of dominant plant-types, whilst others can only support infrequent burning (Archibald et al., 2013; Archibald et al., 2018; Harrison et al., 2021; Foster et al., 2018; Simpson et al., 2021).
At a global scale, burnt area has declined in recent decades (Andela et al., 2017; Forkel et al., 2019; Jones et al., 2022; Zubkova et al., 2023). This decline is driven by changes in the tropical savannas of northern Africa and grasslands in Asia, and to a lesser extent by changes in southern Africa and Europe (Zubkova et al., 2023); other regions of the world have shown no significant trends in the past two decades. Nevertheless, recent years have been marked by extreme wildfire seasons, characterised by increased frequency, seasonality, size, intensity or severity of wildfires, in several regions, including Europe (Carnicer et al., 2022; Grünig et al., 2023), Siberia (Kharuk et al., 2022; Ponomarev et al., 2023) and the western United States (Singleton et al., 2019; Mueller et al., 2020; Jones et al., 2022; Wasserman and Mueller, 2023). These extreme wildfires have been widely attributed to anthropogenic climate change (Abatzoglou et al., 2019; Kirchmeier-Young et al., 2019; Williams et al., 2019; Bowman et al., 2020; Abram et al., 2021; van Oldenborgh et al., 2021). Model projections suggest that burnt area will increase by 13%–15% by 2030 and by 20%–40% by 2050 (UNEP, 2022). The focus on future changes in burnt area may be mis-leading, however, since some studies show that changes in other aspects of the fire regime, such as intensity, may be decoupled from changes in burnt area under altered climate states (Haas et al., 2023; Haas et al., 2024).
Wildfire regimes are controlled by factors related to climate, vegetation, landscape characteristics and human activities (Bowman et al., 2009; Harrison et al., 2010; Bowman et al., 2011). Many of these controls are incorporated in some way in global fire-enabled vegetation models (Rabin et al., 2017) or are implicit in empirically based global fire models. Nevertheless, while these models can predict the broad global patterns of burnt area, they struggle to reproduce fire season length or the interannual variability of wildfires (Hantson et al., 2020). It is unclear how well they reproduce other aspects of wildfire regimes, such as fire intensity, since this has not been a focus of model evaluation. Even though the current generation of fire-enabled vegetation models reproduce modern global patterns of burnt area, there are significant differences between them in even the sign of historic trends in burnt area reflecting differences in the sensitivity to individual forcings (Teckentrup et al., 2019) and an incomplete or inaccurate description of key processes associated with vegetation properties and anthropogenic influences on wildfire (Forkel et al., 2019). There have been many empirical studies analysing the controls on wildfires at a global scale, many conducted since the initial development of the current generation of fire-enabled vegetation models (Forkel et al., 2019) and these could provide a basis for improving model representations of key processes. This effort would require an assessment of the robustness of the findings across studies, and diagnosis of the underlying mechanisms.
The aim of this perspective is to identify key variables needed to model different aspects of wildfire regimes and how these could be incorporated in a global modelling framework. We first review how empirical (data-based) fire models treat key controls on fire processes. We then review global studies of different fire properties to assess whether there is a consensus about the importance of specific drivers of burnt area, occurrence, fire size and fire intensity. Finally, we suggest ways in which current understanding of these empirical relationships could be used to improve models and thus our ability to predict changes in fire regimes under future climate change.
Wildfires are simulated in dynamic global vegetation models (DGVMs) and land-surface models (LSMs) through specific modules (Hantson et al., 2016; Jones et al., 2022). There are two basic types: process-based (see e.g., CTM: Arora and Boer, 2005; Melton and Arora, 2016; SPITFIRE: Thonicke et al., 2010; CLM-Li: Li et al., 2012; Li et al., 2013) and empirical (see e.g., GLOBFIRM: Thonicke et al., 2001; Kloster et al., 2010; SIMFIRE-BLAZE: Knorr et al., 2014; INFERNO: Mangeon et al., 2016) modules. Process-based models simulate the behaviour of individual wildfires using theoretical equations for ignitions and fire spread, alongside parameterisations based on laboratory or field experiments, and typically produce estimates of burnt area by scaling up to the grid-cell level (generally 0.5° × 0.5° or coarser). Although much of the focus of fire modelling has been on burnt area and carbon emission, some process-based models explicitly simulate the number and size of individual fires. Empirical models do not try to simulate individual wildfires but simulate the emergent properties of the fire generally using statistical relationships between assumed drivers and fire properties (usually burnt area) across a grid. Comparisons of process-based and empirical models made in the context of the Fire Modelling Intercomparison Project (FireMIP: Hantson et al., 2016; Rabin et al., 2017) have shown that empirical models perform at least as well as process-based models in simulating global patterns of burnt area under modern conditions (Hantson et al., 2020). Both empirical and process-based fire models are incorporated in LSM components of the climate models used in the sixth phase of the Coupled Model Intercomparison Project (CMIP6), and show comparable performance in simulating modern burnt area and emissions (Li et al., 2024).
Although all the FireMIP models can reproduce the spatial patterns in burnt area under modern conditions reasonably well, they tend to overestimate the length of the fire season and do not capture the interannual variability in wildfires (Hantson et al., 2020). Perhaps more importantly, the models disagreed on the sign and magnitude of the trend in global burnt area over the 20th century (Teckentrup et al., 2019; Jones et al., 2022). Furthermore, although the models captured the emergent observed relationships between burnt area and various climate factors influencing wildfires, they failed to capture the observed relationships with other drivers (Forkel et al., 2019; Teckentrup et al., 2019). These analyses imply that the incorporation of process understanding in both empirical and process-based models is incomplete.
This incompleteness is illustrated by differences in the three empirically based models (Figure 1; Supplementary Table 1) that have been used in FireMIP: GLOBFIRM (Thonicke et al., 2001; Kloster et al., 2010), SIMFIRE-BLAZE (Knorr et al., 2014) and INFERNO (Mangeon et al., 2016). Fire occurrence is determined by ignitions in INFERNO, where the number of natural and anthropogenic ignitions is prescribed. However, ignitions are not considered to be limiting in GLOBFIRE and SIMFIRE-BLAZE and occurrence is therefore entirely determined by fuel load and climate conditions. SIMFIRE-BLAZE uses a threshold of climatological values of the fraction of absorbed photosynthetically active radiation (fAPAR) as an index of fuel load, whereas the variants of GLOBFIRM use thresholds of fuel load explicitly to determine fire occurrence. SIMFIRE-BLAZE also uses thresholds for daily precipitation and diurnal temperature range to determine fire occurrence, while GLOBFIRM only allows fire to occur when fuel moisture content is less than the moisture of extinction. The Kloster et al. (2010) variant of GLOBFIRM also imposes a daily minimum temperature threshold on occurrence. All three models consider landscape flammability, determined by plant functional type (PFT) in INFERNO, by the length of the fire season and landcover class in SIMFIRE-BLAZE and by the total number of days with a probability of fire ignition greater than one in GLOBFIRM. Fire size is also determined differently by the three models: by climate conditions in SIMFIRE-BLAZE, fire season length in GLOBFIRM and by PFT in INFERNO. The standard version of GLOBFIRM does not account for fire suppression, but the other models take account of this either through population density (SIMFIRE-BLAZE, the Kloster et al. (2010) version of GLOBFIRM and INFERNO) or through cropland area (the modified version of GLOBFIRM in Thonicke et al., 2001). The final estimate of burnt area is the product of land cover, longterm fAPAR and the length of the dry season in SIMFIRE-BLAZE, whereas it is determined by the number of ignitions, flammability and PFT-specific fire size in INFERNO, and determined by the length of the fire season in GLOBFIRM. These differences in the processes considered and in the final simulation of burnt area between the empirical models clearly highlight the uncertainties in understanding of the empirical controls on fire.
Figure 1. Flow diagram showing how processes related to the simulation of burnt area are treated in empirical models from FireMIP.
A review of the literature identified 10 empirical global studies that examined the controls on wildfires, using either machine-learning or more traditional regression-based approaches, and ranked them according to their relative importance in determining burnt area (Supplementary Table 2). Although these studies used different analytical approaches, and included different numbers of explanatory variables, they all provide an assessment of which variables are significant and a quantitative ranking of the importance of these variables (see Supplementary Table 3), allowing at least a qualitative comparison of the consistency of the results. Several other studies looked at the influence of specific controls (e.g., Knorr et al., 2014; Lasslop et al., 2015; Krawchuk and Moritz, 2011) or have derived predictions based on optimizing multiple controls without prioritizing them (e.g., Boer et al., 2021); or examined controls on interannual variability rather than burnt area (e.g., Abatzoglou et al., 2018). These studies do not provide a ranking of the importance and are therefore not considered here.
The initial selection of variables reflects hypotheses about the mechanisms involved in wildfire occurrence and spread. It is therefore not surprising that variables related to climate (often some measure of precipitation and temperature) are selected in most studies (Figure 2). More surprisingly, the relative importance of these variables does not reflect the frequency with which they are included in analyses. In particular, atmospheric humidity is consistently shown to be an important variable although it is included in analyses only half as frequently as temperature and precipitation. Field studies indicate that atmospheric humidity is a strong control on both live and dead fuel moisture (Dickman et al., 2023) and this probably explains its emergence as an important variable. However, in a modelling context, fuel moisture is more often represented by soil moisture (only indirectly related to humidity) or some kind of moisture index - both of which are shown to be relatively unimportant in these empirical analyses. Variables related to fuel availability, such as the current vegetation amount and vegetation type, are included equally frequently in these global analyses. The current amount of vegetation has a different influence on burnt area from the amount during antecedent periods (Kuhn-Régnier et al., 2021) and thus these two variables should be considered separately. Variables related to the instantaneous amount of vegetation, which impacts both fuel availability and fuel continuity, emerge as more diagnostic than vegetation type. When considering both instantaneous and antecedent conditions, vegetation amount emerges as the single most important control on burnt area, being the most important predictor in a third of the studies (Figure 2). This suggests that models that explicitly consider fuel loads or measures such as fAPAR that are closely related to vegetation production are more likely to capture the processes involved in modulating burnt area than models that rely on PFT-specific parameterisations. A number of variables related to ignitions (lightning, demographic measures) or to fire spread (wind speed, topography) are routinely included in these empirical analyses, but turn out to be less important determinants of burnt area at a global scale. This helps to explain why models that assume that ignitions are saturated perform as well as those that explicitly try to estimate the frequency of natural and human ignitions. Factors relating to landscape fragmentation (e.g., measures of agricultural activity or landscape development) are also less important than climate and vegetation factors in determining burnt area. Landscape fragmentation has an impact on limiting fire spread, but this impact is complex and depends on the nature of the fragmentation and the vegetation type (Armenteras et al., 2017; Harrison et al., 2021; Rosan et al., 2022; Harrison et al., 2024). Current measures of and assumptions about the role of fragmentation, both in empirical and process-based models, are likely over-simplified. More generally, no variable representative of human activity and its impact emerges as a strong control on burnt area. This highlights that the current ways in which we represent this impact in global models are most likely inadequate and unable to capture the desired human effects.
Figure 2. Summary of variables selected and considered important for predictions of burnt area in global empirical analyses. The individual bars show the number of studies which included the variable as a predictor (blue), the number of times the variable was selected as the most important driver of burnt area (red) and the number of times the variable was in the top three predictors (pink).
Even in the broad categories related to climate and vegetation, there are differences in the specific metrics employed in different empirical studies. Precipitation, for example, can be represented by average monthly values, total precipitation within the typical burn period or a specific season, or precipitation during an antecedent period of varying length. However, these different choices frequently relate to different processes: average monthly data is largely a measure of the immediate impact of precipitation on fuel moisture whereas antecedent precipitation is more likely to be related to the control of aridity on vegetation growth and fuel accumulation. Both the instantaneous and antecedent measures of precipitation emerge as important controls of burnt area, and they have been shown to have different (and contrasting) effects (Kuhn-Régnier et al., 2021). This poses problems in empirical analyses since the inclusion of more than one predictor variable to represent a key process means that the influence of both may be down-weighted. There is no ideal solution to this problem since there are still uncertainties about which specific processes need to be considered and which predictive variables would best represent these processes. One approach that has been used is systematic variable selection, in which a large number of individual variables are tested for their contribution in a large number of models constructed by systematically including or excluding individual variables (e.g., Tracy et al., 2018; Keeping et al., 2024).
Systematic variable selection analyses provide support for the importance of climate, vegetation and human parameters in driving fire occurrence. Figure 3 shows the frequency of variable selection, using a forward-backward selection algorithm (Keeping et al., 2024), for the prediction of fires >0.25 acres for the contiguous United States in a 12-variable model, given the selection of one initial variable, in a suite of 1,000 variable selection runs (see Supplementary Figure 1 for full matrix). Diurnal temperature range is consistently chosen in all of the runs, even when other temperature variables are selected as the initial variable. Similarly, 5-day precipitation, snow cover, antecedent GPP and rural population density are consistently selected as important in more than 90% of the selection runs. This analysis confirms the importance of the factors that emerge as important controls on fire in global analyses - including factors influencing fuel load (such a vegetation cover or GPP), fuel drying (such as relative humidity, precipitation), and fire spread (such as population density or crop cover). However, some factors that have been used as predictors of fire occurrence in regional empirical studies, such as minimum temperature or the presence of powerlines, are never selected in this analysis. This shows that some factors that might be thought of as influencing fire occurrence have no additional predictive power in the model and are thus not important at a global scale.
Figure 3. Outcome of systematic variable selection, showing the initial variable included in the model and the median and range of the number of times it was selected. The variables are: Vsoil3 – daily soil water volume in second layer (28–100 cm) (m3/m3), MinT–minimum daily temperature (°C), MaxT–maximum daily temperature (°C), rail–total length of rail lines per km2 (km/km2), BROADLEAF–the fraction of broadleaf plants (%), powerline–total length of powerlines per km2 (km/km2), drying–cumulated vapour pressure deficit in prior dry spell (Pa days), VPD_100d–mean vapour pressure deficit over prior 100 days (Pa), PopDens–population density (people/km2), trail–total length of walking trails per km2 (km/km2), road–total length of roads per km2 (km/km2), Vsoil1 – daily soil water volume in top layer (0–7 cm) (m3/m3), NEEDLELEAF–the fraction of needleleaf plants (%), GPP_150d–Gross Primary Production in prior 150 days (µ gC/m2/s), Vsoil2 – daily soil water volume in second layer (7–28 cm) (m3/m3), GPP_5y–Gross Primary Production in prior 5 years (µ gC/m2/s), dd–prior dry days (<0.1 mm precipitation) (days), VPD_night–mean vapour pressure deficit in night-time (Pa), GPP_10y–Gross Primary Production in prior 10 years (µ gC/m2/s), GPP_2y–Gross Primary Production in prior 2 years (µ gC/m2/s), sfcWind–surface windspeed (m/s), CAPE–convective atmospheric potential energy (J/kg), elevation–elevation (m, dry_CAPE–convective atmospheric potential energy if day is dry (J/kg), Vsoil1_365d–soil water volume in top layer (0–7 cm) averaged over prior 365 days (m3/m3), VRM–vector roughness metric (unitless), VPD_10d–mean vapour pressure deficit over prior 10 days (Pa), VPD_31d–mean vapour pressure deficit over prior 31 days (Pa), solar_insolation–downwelling shortwave solar radiation (J/m2/s), VPD–daily mean vapour pressure deficit (Pa), HERB–the fraction of herbaceous plants (%), TREE–the fraction of forest (%), GPP_50d–Gross Primary Production in prior 50 days (µ gC/m2/s), alpha_mean–the average annual ratio of actual to potential evapotranspiration (kg/kg), T–daily mean temperature (°C), CROP–the fraction of cropland (%), precip–daily precipitation (kg), SHRUB–the fraction of shrubland (%), GPP_100d–Gross Primary Production in prior 100 days (µ gC/m2/s), RH–relative humidity (%), GPP_1y–Gross Primary Production in prior 1 year (µ gC/m2/s), popDens_rural–population density clipped at 25 people per km2 (people/km2), snow_cover–snow cover fraction (%), precip_5d–precipitation in prior 5 days (kg), DTR–diurnal temperature range (°C).
Few studies have examined the global controls on fire properties other than burnt area. Three studies were identified that explicitly looked at the environmental controls of fire occurrence (Shmuel and Heifetz, 2022; 2023; Mukunga et al., 2023; Zhang et al., 2023) and four that examined the global controls of fire size (Hantson et al., 2015; Shmuel and Heifetz, 2022; Haas et al., 2022; Zhang et al., 2023). Despite the limited number of studies, factors relating to human activity were shown to have a less significant effect on fire occurrence than authors expected (Zhang et al., 2023; Shmuel and Heifetz, 2022) although gross domestic product (GDP) did emerge as a significant control (Mukunga et al., 2023). Cropland fraction was shown to increase ignitions in one study (Mukunga et al., 2023); this contradicts the assumption that croplands have a consistent, suppressing effect on wildfire activity as registered by fire size and burnt area. Wind speed was also shown to have a negative effect on fire occurrence above a threshold of 3–4 m/s-1 (Shmuel and Heifetz, 2023). Fire size follows a power-law (Randerson et al., 2012), and Hantson et al. (2015) used this assumption to investigate the drivers of fire size. They showed that there is a negative linear relationship between cropland cover and fire size but the relationship with population density was humped, with fire size peaking at intermediate levels of population density. Similar assumptions underpin the study of Andela et al. (2017), which suggests that agricultural expansion has been a key driver of the reduction in fire size (and the number of fires and global total burnt area) in recent decades. Zhang et al. (2023) also showed the dominant role of human activity in decreasing global BA through suppressing fire size rather than fire numbers as well as a limited effect of climate and vegetation in driving fire size (Zhang et al., 2023). This finding is in line with work showing that road density and cropland cover have a strong limiting effect on fire size, but that vegetation predictors had a less significant effect (Haas et al., 2022). These various studies make it clear that human activities influence different fire properties in different ways and that factors that increase ignitions may have the opposite impact on overall burnt area or fire intensity.
Some previous studies have focused on the relationship between different aspects of fire regimes (e.g., size, frequency, intensity) and grouped distinct clusters of these properties into fire regimes (e.g., Archibald et al., 2013; Pereira et al., 2022; Fan et al., 2023; Pias et al., 2023; Garcia et al., 2022; Yang et al., 2023). Luo et al. (2017) proposed that there is a humped relationship between fire frequency and fire intensity, with the highest-intensity fires occurring at intermediate levels of fire occurrence. This finding presumably reflects the fact that frequent fires fragment the landscape (Laurent et al., 2019) and reduce fuel load and fuel continuity (Archibald et al., 2013; Luo et al., 2017), whereas environments characterised by low fire frequency may have high fuel loads but must inevitably be too wet to burn.
Only one study (Haas et al., 2022) has separately examined the role of individual predictors on different aspects of the fire regime, comparing the drivers of burnt area, fire size and fire intensity. Starting from a common set of 16 predictors, that study showed that some variables were important for one property but either had no influence or had the opposite effect on the others. For example, both annual GPP and the seasonality of dry days have a positive effect on burnt area, but no influence on fire size and reduce fire intensity (Figure 4). Overall, whereas burnt area is primarily driven by factors influencing fuel availability and fuel dryness and is reduced by landscape fragmentation, fire size is increased by factors promoting fire spread such as wind speed but is also reduced by landscape fragmentation. Fire intensity is primarily driven by tree cover; the strong positive relationship with road density probably reflects deforestation fires. These results are broadly compatible with the emergent relationships between different fire properties shown in previous studies. However, they have strong implications for process-based fire modelling since they imply that changes in fire properties could be decoupled under changed climates (Haas et al., 2023; Haas et al., 2024) and so the processes underlying burnt area, fire size and fire intensity need to be distinguished.
Figure 4. Empirical analysis of the relative importance (as measured by t-values) of individual predictors for (A) burnt area, (B) fire size and (C) fire intensity. Green represents variables related to vegetation properties, red represents variables related to climate, and purple represents natural and anthropogenic variables related to landscape fragmentation. GPP, gross primary production; Popd, population densitiy; VRM, vector ruggedness measure: TPI, topographic position index; DD, dry days; VPD, vapour pressure deficit; DTR, diurnal temperature range; Wind, windspeed; Light, Lightning; tree, grass, shrub, crop are the fractional cover of each land type. Redrawn from Haas et al., (2022).
Consistent patterns are emerging from empirical analyses that are leading to an improved understanding of wildfire regimes and their environmental controls. These provide a basis for improving the treatment of wildfire in a modelling context. Here, we summarise the lessons learnt from these empirical analyses about what factors are important and suggest ways in which these lessons could be incorporated into process-based models.
GPP consistently emerges as the most important control on wildfires. Both total GPP and the seasonality of GPP have a positive impact on burnt area. The influence of seasonality is consistent with studies that show that antecedent GPP has an opposite effect from the GPP during the wildfire season (Forkel et al., 2017; Kuhn-Régnier et al., 2021): high GPP in the period before the fire season means that fuel loads are high, whereas high GPP at the time of a fire could only be sustained if climate conditions were favourable, i.e., the fuel is likely too wet to burn. Studies that have included measures of antecedent conditions have generally found them to be important controls on wildfires. This is also consistent with the negative impact of GPP on fire intensity. These various measures of GPP appear to be reasonably good surrogates for fuel loads. Given the limited field information available globally about fuel loads (see e.g., Pettinari and Chuvieco, 2016; McNorton and Di Giuseppe, 2024), this suggests that GPP (or net primary production, NPP) can usefully serve as a surrogate in a modelling context. However, this means that it is important to ensure that the vegetation component of process-based wildfire models simulate GPP (or NPP) accurately to ensure an accurate simulation of wildfire regimes (Forkel et al., 2019).
Vegetation cover emerges from the empirical analyses as an important influence on wildfire, particularly the relative abundance of trees versus herbaceous plants. The nature of the vegetation cover is a crucial factor in influencing fuel continuity and fire spread. Globally, grass-dominated landscapes tend to lower fire intensity but produce more burnt area. However, empirical analyses that include PFTs as predictors do not show that they have an influence on wildfire properties. Many fire models have PFT-specific parameterisations, but this arises largely because of inheriting the PFT concept from the dynamic vegetation models used to provide vegetation characteristics (Cranko Page et al., 2024). Since this multiplies the number of, often poorly specified, parameters needed, simplifying the linkages between vegetation properties and wildfire processes would be useful. There are studies suggesting that individual species are fire-promoting but, at the scale of global modelling, it seems more likely that the traits which promote fire spread, such as the presence of volatile oils or ladder-fuel structure (Blauw et al., 2017; Popović et al., 2021; Rodman et al., 2021; Chen et al., 2023a; Chen et al., 2023b), should be explicitly represented in fire-enabled vegetation models.
The empirical analyses show that climatic variables that influence atmospheric humidity, and hence fuel drying, such as vapour pressure deficit (VPD) or the diurnal temperature range consistently emerge as an important control on wildfires. Precipitation, as such, is likely chiefly influential in so far as it is a surrogate for the more direct influence of atmospheric humidity on fuel drying. In studies that include precipitation and some measure of atmospheric dryness, precipitation may also be a reflection of direct fuel wetting. Soil moisture has been widely used in a modelling context to account for fuel dryness, but empirical studies which have tested this, or used aridity indices, indicate that these are not good predictors. In the absence of explicit simulation of the fuel bed and fuel moisture, models could replace soil moisture with parameterisations based on climatic factors such as VPD, which are more closely aligned to the process of fuel drying.
Empirical analyses indicate that ignitions are not a limiting factor for wildfire occurrence at a global scale. Lightning is important for ignitions in many ecosystems. Lightning initiates ca 90% of wildfires in Siberia (Ivanov and Ivanova, 2010) and the majority of fires in Alaska (Kasischke et al., 2010) and northern Canada (Coogan et al., 2020). Changes in the incidence of lightning have been associated with increases in wildfires in these regions during the historical and recent past (e.g., Vachula et al., 2022; Veraverbeke et al., 2017). Nevertheless, even in these regions, climate and vegetation factors that influence fuel load and fuel drying play an important part in determining the occurrence of wildfires. Given this, models that prescribe anthropogenic and lightning ignitions, such as the empirical model INFERNO (Mangeon et al., 2016) and virtually all process-based models (Rabin et al., 2017), are likely focusing on the wrong process. As the differences in the prescribed relationships used by different models show (Teckentrup et al., 2019), the relationships are also poorly constrained. Most models use population density to encapsulate both anthropogenic ignitions and (direct and indirect) suppression (Rabin et al., 2017; Li et al., 2024), but this conflates two distinct processes which are influenced by different factors. Remote-sensed fire products are now available at much higher resolution, and this means that they now capture small fires, many of which are explicitly set for agricultural purposes such as preparing fields or removing waste (Millington et al., 2022). The use of total population density as a measure of anthropogenic ignitions for both wildfires and agricultural fires, ignores both differences between these two types of fire and the complexity of the cultural uses of fire.
Empirical analyses do not support the hypotheses about human impacts on wildfire currently embedded in process-based models. The peaked relationship between burnt area and population density used in many models (Rabin et al., 2017; Li et al., 2024) is an emergent property of the system and not a causal mechanism (Bistinas et al., 2014). Including other factors reflecting human transformation of the landscape, such as road density for example, transforms the relationship between burnt area and population density from negative (Bistinas et al., 2014) to positive (Haas et al., 2022). There have been attempts to provide a more nuanced picture of human impacts, through the inclusion of economic measures such as GDP (e.g., Mukunga et al., 2023), but while these factors may influence the number of ignitions they rarely turn out to be important predictors of other properties such as burnt area and do not improve the predictions of wildfire regimes. Cultural differences in human influence on fire (Pyne, 2014; Huffman, 2013; Millington et al., 2022) need to be expressed in a way that takes account of the intent and practice of deliberate and managed burning. Separate treatments of wildfires and agricultural fires are required in a modelling context, since their controls are very different.
Fragmentation is important in limiting fire spread, and this is reflected in the importance of factors such as crop cover and population density, which are indirect measures of fragmentation of the landscape, in the empirical analyses. However, a more nuanced approach to measuring fragmentation is needed if this is to be implemented in a modelling context. There is a growing understanding that the impacts of fragmentation differ between ecosystems (Armenteras et al., 2017; Harrison et al., 2021; Rosan et al., 2022; Harrison et al., 2024), and that this can be broadly explained in terms of the degree to which an ecosystem is adapted to frequent fires or not. However, more systematic analyses are required before these insights can be properly incorporated into models.
Empirical analyses of the controls on wildfire need to be more explicit about which processes are hypothesised to be controlled by specific variables. This will ensure that the variables tested are not redundant and/or acting on two different aspects of the wildfire regime. It would also be useful to adopt more systematic approaches to the evaluation of the inclusion/exclusion of specific variables and their contributions. Nevertheless, although there are gaps in our understanding of the controls on wildfires, especially related to the role of humans and the influence of landscape fragmentation, empirical analyses at the global scale already provide insights that could be used to improve process representations in process-based fire-vegetation models. The most obvious improvements are to use variables more closely aligned to the processes of generating fuel loads and of fuel drying. Separating the treatment of different aspects of the fire regime, most particularly by simulating fire intensity separately from fire size and overall burnt area, would also be a useful improvement. While the existing empirical models themselves can be useful in projecting the response of wildfires to future climate change (e.g., Haas et al., 2024), improved process-based models will be necessary in the long run to be able to examine feedbacks from fire to the climate system.
OH: Conceptualization, Investigation, Writing–review and editing. TK: Conceptualization, Investigation, Writing–review and editing. JG-D: Conceptualization, Investigation, Writing–review and editing. IP: Conceptualization, Investigation, Writing–review and editing. SH: Conceptualization, Formal Analysis, Investigation, Writing–original draft, Writing–review and editing.
The author(s) declare that financial support was received for the research, authorship, and/or publication of this article. OH, TK, ICP and SPH acknowledge support from the LEMONTREE (Land Ecosystem Models based On New Theory, obseRvations and ExperimEnts) project, funded through the generosity of Eric and Wendy Schmidt by recommendation of the Schmidt Futures programme. The grant number for the Lemontree funding is: Grant number 355.
This paper is a contribution to the work of the Vegetation-Fire giraffe team of the Leverhulme Centre for Wildfires, Environment and Society (https://centreforwildfires.org/). We thank members of the giraffe team for discussions during the development of this work.
The authors declare that the research was conducted in the absence of any commercial or financial relationships that could be construed as a potential conflict of interest.
All claims expressed in this article are solely those of the authors and do not necessarily represent those of their affiliated organizations, or those of the publisher, the editors and the reviewers. Any product that may be evaluated in this article, or claim that may be made by its manufacturer, is not guaranteed or endorsed by the publisher.
The Supplementary Material for this article can be found online at: https://www.frontiersin.org/articles/10.3389/fenvs.2024.1438262/full#supplementary-material
Abatzoglou, J. T., Williams, A. P., and Barbero, R. (2019). Global emergence of anthropogenic climate change in fire weather indices. Geophys. Res. Lett. 46, 326–336. doi:10.1029/2018gl080959
Abatzoglou, J. T., Williams, A. P., Boschetti, L., Zubkova, M., and Kolden, C. A. (2018). Global patterns of interannual climate–fire relationships. Glob. Change Biol. 24, 5164–5175. doi:10.1111/gcb.14405
Abram, N. J., Henley, B. J., Sen Gupta, A., Lippmann, T. J. R., Clarke, H., Dowdy, A. J., et al. (2021). Connections of climate change and variability to large and extreme forest fires in southeast Australia. Commun. Earth Environ. 2 (8), 8. doi:10.1038/s43247-020-00065-8
Andela, N., Morton, D. C., Giglio, L., Chen, Y., van der Werf, G. R., Kasibhatla, P. S., et al. (2017). A human-driven decline in global burned area. Science 356, 1356–1362. doi:10.1126/science.aal4108
Archibald, S., Lehmann, C. E. R., Belcher, C. M., Bond, W. J., Bradstock, R. A., Daniau, A.-L., et al. (2018). Biological and geophysical feedbacks with fire in the Earth system. Env. Res. Lett. 13, 033003. doi:10.1088/1748-9326/aa9ead
Archibald, S., Lehmann, C. E. R., Gómez-Dans, J. L., and Bradstock, R. A. (2013). Defining pyromes and global syndromes of fire regimes. Proc. Natl. Acad. Sci. U. S. A. 110, 6442–6447. doi:10.1073/pnas.1211466110
Armenteras, D., Barreto, J. S., Tabor, K., Molowny-Horas, R., and Retana, J. (2017). Changing patterns of fire occurrence in proximity to forest edges, roads and rivers between NW Amazonian countries. Biogeosci. 14, 2755–2765. doi:10.5194/bg-14-2755-2017
Arora, V. K., and Boer, G. (2005). Fire as an interactive component of dynamic vegetation models. J. Geophys. Res. 110, G02008. doi:10.1029/2005JG000042
Bistinas, I., Harrison, S. P., Prentice, I. C., and Pereira, J. M. C. (2014). Causal relationships versus emergent patterns in the global controls of fire frequency. Biogeosci 11, 5087–5101. doi:10.5194/bg-11-5087-2014
Blauw, L. G., van Logtestijn, R. S. P., Broekman, R., Aerts, R., and Cornelissen, J. H. C. (2017). Tree species identity in high-latitude forests determines fire spread through fuel ladders from branches to soil and vice versa. For. Ecol. Manage. 400, 475–484. doi:10.1016/j.foreco.2017.06.023
Boer, M. M., De Dios, V. R., Stefaniak, E. Z., and Bradstock, R. A. (2021). A hydroclimatic model for the distribution of fire on Earth. Environ. Res. Comm. 3, 035001. doi:10.1088/2515-7620/abec1f
Bowman, D. M., Balch, J., Artaxo, P., Bond, W. J., Cochrane, M. A., D’Antonio, C. M., et al. (2011). The human dimension of fire regimes on Earth. J. Biogeog. 38, 2223–2236. doi:10.1111/j.1365-2699.2011.02595.x
Bowman, D. M. J. S., Balch, J. K., Artaxo, P., Bond, W. J., Cochrane, M. A., D’Antonia, C. M., et al. (2009). Fire in the earth system. Science 324, 481–484. doi:10.1126/science.1163886
Bowman, D. M. J. S., Kolden, C. A., Abatzoglou, J. T., Johnston, F. H., van der Werf, G. R., and Flannigan, M. (2020). Vegetation fires in the anthropocene. Nat. Rev. Earth Environ. 1, 500–515. doi:10.1038/s43017-020-0085-3
Carnicer, J., Alegria, A., Giannakopoulos, C., Di Giuseppe, F., Karali, A., Koutsias, N., et al. (2022). Global warming is shifting the relationships between fire weather and realized fire-induced CO2 emissions in Europe. Sci. Rep. 12, 10365. doi:10.1038/s41598-022-14480-8
Chen, F., Si, L., Zhao, F., and Wang, M. (2023a). Volatile oil in Pinus yunnanensis potentially contributes to extreme fire behavior. Fire 6, 113. doi:10.3390/fire6030113
Chen, Y., Hall, J., van Wees, D., Andela, N., Hantson, S., Giglio, L., et al. (2023b). Multi-decadal trends and variability in burned area from the fifth version of the Global Fire Emissions Database (GFED5). Earth Syst. Sci. Data 15, 5227–5259. doi:10.5194/essd-15-5227-2023
Coogan, S. C. P., Cai, X., Jain, P., and Flannigan, M. D. (2020). Seasonality and trends in human- and lightning-caused wildfires ≥2 ha in Canada, 1959–2018. Int. J. Wildland Fire 29, 473–485. doi:10.1071/WF19129
Cranko Page, J., Abramowitz, G., De Kauwe, M. G., and Pitman, A. J. (2024). Are plant functional types fit for purpose? Geophys. Res. Lett. 51, e2023GL104962. doi:10.1029/2023GL104962
Dickman, L. T., Jonko, A. K., Linn, R. R., Altintas, I., Atchley, A. L., Bär, A., et al. (2023). Integrating plant physiology into simulation of fire behavior and effects. New Phytol. 238, 952–970. doi:10.1111/nph.18770
Fan, H., Yang, X., Zhao, C., Yang, Y., and Shen, Z. (2023). Spatiotemporal variation characteristics of global fires and their emissions. Atmos. Chem. Phys. 23, 7781–7798. doi:10.5194/acp-23-7781-2023
Forkel, M., Dorigo, W., Lasslop, G., Chuvieco, E., Hantson, S., Heil, A., et al. (2019). Recent global and regional trends in burned area and their compensating environmental controls. Environ. Res. Comm. 1, 051005. doi:10.1088/2515-7620/ab25d2
Forkel, M., Dorigo, W., Lasslop, G., Teubner, I., Chuvieco, E., and Thonicke, K. (2017). A data-driven approach to identify controls on global fire activity from satellite and climate observations (SOFIA V1). Geosci. Model Dev. 10, 4443–4476. doi:10.5194/gmd-10-4443-2017
Foster, C. N., Barton, P. S., MacGregor, C. I., Catford, J. A., Blanchard, W., and Lindenmayer, D. B. (2018). Effects of fire regime on plant species richness and composition differ among forest, woodland and heath vegetation. Appl. Veg. Sci. 21, 132–143. doi:10.1111/avsc.12345
García, M., Pettinari, M. L., Chuvieco, E., Salas, J., Mouillot, F., Chen, W., et al. (2022). Characterizing global fire regimes from satellite-derived products. Forests 13, 699. doi:10.3390/f13050699
Giglio, L., Randerson, J. T., and van der Werf, G. R. (2013). Analysis of daily, monthly, and annual burned area using the fourth-generation global fire emissions database (GFED4). J. Geophys. Res. Biogeosci. 118, 317–328. doi:10.1002/jgrg.20042
Grünig, M., Seidl, R., and Senf, C. (2023). Increasing aridity causes larger and more severe forest fires across Europe. Glob. Change Biol. 29, 1648–1659. doi:10.1111/gcb.16547
Haas, O., Prentice, I., and Harrison, S. (2024). Global wildfires on a changing planet. In revision, Nat. Commun.
Haas, O., Prentice, I. C., and Harrison, S. P. (2022). Global environmental controls on wildfire burnt area, size and intensity. Environ. Res. Lett. 17, 065004. doi:10.1088/1748-9326/ac6a69
Haas, O., Prentice, I. C., and Harrison, S. P. (2023). Examining the response of wildfire properties to climate and atmospheric CO2 change at the Last Glacial Maximum. Biogeosci 20, 3981–3995. doi:10.5194/bg-20-3981-2023
Hantson, S., Arneth, A., Harrison, S. P., Kelley, D. I., Prentice, I. C., Rabin, S. S., et al. (2016). The status and challenge of global fire modelling. Biogeosci 13, 3359–3375. doi:10.5194/bg-13-3359-2016
Hantson, S., Kelley, D. I., Arneth, A., Harrison, S. P., Archibald, S., Bachelet, D., et al. (2020). Quantitative assessment of fire and vegetation properties in simulations with fire-enabled vegetation models from the Fire Model Intercomparison Project. Geosci. Model Dev. 13, 3299–3318. doi:10.5194/gmd-13-3299-2020
Hantson, S., Pueyo, S., and Chuvieco, E. (2015). Global fire size distribution is driven by human impact and climate. Glob. Ecol. Biogeogr. 24, 77–86. doi:10.1111/geb.12246
Harrison, S. P., Marlon, J., and Bartlein, P. J. (2010). “Fire in the earth system,” in Changing climates, earth Systems and society. International year of planet earth, climate change theme. Editor J. Dodson (Legacy: Springer-Verlag), 21–48.
Harrison, S. P., Prentice, I. C., Bloomfield, K., Dong, N., Forkel, M., Forrest, M., et al. (2021). Understanding and modelling wildfire regimes: an ecological perspective. Environ. Res. Lett. 16, 125008. doi:10.1088/1748-9326/ac39be
Harrison, S. P., Haas, O., Bartlein, P. J., Sweeney, L., and Zhang, G. (in press 2024). Climate, vegetation, people: disentangling the controls of fire at different timescales. Philos. Trans. R. Soc. B.
Huffman, M. R. (2013). The many elements of traditional fire knowledge: synthesis, classification, and aids to cross-cultural problem solving in fire dependent systems around the world. Ecol. Soc. 18, 3. doi:10.5751/es-05843-180403
Ivanov, V. A., and Ivanova, G. A. (2010). Wildfires from lightning in forests of Siberia. Novosibirsk: Nauka.
Jones, M. W., Abatzoglou, J. T., Veraverbeke, S., Andela, N., Lasslop, G., Forkel, M., et al. (2022). Global and regional trends and drivers of fire under climate change. Rev. Geophys. 60, e2020RG000726. doi:10.1029/2020rg000726
Kasischke, E. S., Verbyla, D. L., Rupp, T. S., McGuire, A. D., Murphy, K. A., Jandt, R., et al. (2010). Alaska’s changing fire regime — implications for the vulnerability of its boreal forestsThis article is one of a selection of papers from the Dynamics of Change in Alaska’s Boreal Forests: resilience and Vulnerability in Response to Climate Warming. Can. J. For. Res. 40, 1313–1324. doi:10.1139/X10-098
Keeping, T., Harrison, S. P., and Prentice, I. C. (2024). Modelling the daily probability of wildfire occurrence in the contiguous United States. Environ. Res. Lett. 19, 024036. doi:10.1088/1748-9326/ad21b0
Kharuk, V. I., Dvinskaya, M. L., Im, S. T., Golyukov, A. S., and Smith, K. T. (2022). Wildfires in the siberian arctic. Fire 5, 106. doi:10.3390/fire5040106
Kirchmeier-Young, M. C., Gillett, N. P., Zwiers, F. W., Cannon, A. J., and Anslow, F. S. (2019). Attribution of the influence of human-induced climate change on an extreme fire season. Earth’s Future 7, 2–10. doi:10.1029/2018EF001050
Kloster, S., Mahowald, N. M., Randerson, J. T., Thornton, P. E., Hoffman, F. M., Levis, S., et al. (2010). Fire dynamics during the 20th century simulated by the community land model. Biogeosci 7, 1877–1902. doi:10.5194/bg-7-1877-2010
Knorr, W., Kaminski, T., Arneth, A., and Weber, U. (2014). Impact of human population density on fire frequency at the global scale. Biogeosci. 11, 1085–1102. doi:10.5194/bg-11-1085-2014
Krawchuk, M. A., and Moritz, M. A. (2011). Constraints on global fire activity vary across a resource gradient. Ecology 92, 121–132. doi:10.1890/09-1843.1
Kuhn-Régnier, A., Voulgarakis, A., Nowack, P., Forkel, M., Prentice, I. C., and Harrison, S. P. (2021). The importance of antecedent vegetation and drought conditions as global drivers of burnt area. Biogeosci 18, 3861–3879. doi:10.5194/bg-18-3861-2021
Lasslop, G., Hantson, S., and Kloster, S. (2015). Influence of wind speed on the global variability of burned fraction: a global fire model’s perspective. Int. J. Wildland Fire 24, 989–1000. doi:10.1071/wf15052
Laurent, P., Mouillot, F., Moreno, M. V., Yue, C., and Ciais, P. (2019). Varying relationships between fire radiative power and fire size at a global scale. Biogeosci 16, 275–288. doi:10.5194/bg-16-275-2019
Lavorel, S., Flannigan, M. D., Lambin, E. F., and Scholes, M. C. (2007). Vulnerability of land systems to fire: interactions among humans, climate, the atmosphere, and ecosystems. Mitig. Adapt. Strateg. Glob. Change 12, 33–53. doi:10.1007/s11027-006-9046-5
Li, F., Levis, S., and Ward, D. S. (2013). Quantifying the role of fire in the earth system – Part 1: improved global fire modeling in the community earth system model (CESM1). Biogeosci 10, 2293–2314. doi:10.5194/bg-10-2293-2013
Li, F., Song, X., Harrison, S. P., Marlon, J. R., Lin, Z., Leung, L. R., et al. (2024). Evaluation of global fire simulations in CMIP6 Earth system models. Geosci. Model Dev. doi:10.5194/gmd-2024-85
Li, F., Zeng, X. D., and Levis, S. (2012). A process-based fire parameterization of intermediate complexity in a Dynamic Global Vegetation Model. Biogeosci 9, 2761–2780. doi:10.5194/bg-9-2761-2012
Luo, R., Hui, D., Miao, N., Liang, C., and Wells, N. (2017). Global relationship of fire occurrence and fire intensity: a test of intermediate fire occurrence-intensity hypothesis. J. Geophys. Res. Biogeosci. 122, 1123–1136. doi:10.1002/2016jg003722
Mangeon, S., Voulgarakis, A., Gilham, R., Harper, A., Sitch, S., and Folberth, G. (2016). INFERNO: a fire and emissions scheme for the UK Met Office’s Unified Model. Geosci. Model Dev. 9, 2685–2700. doi:10.5194/gmd-9-2685-2016
McNorton, J. R., and Di Giuseppe, F. (2024). A global fuel characteristic model and dataset for wildfire prediction. Biogeosci 21, 279–300. doi:10.5194/bg-21-279-2024
Melton, J. R., and Arora, V. K. (2016). Competition between plant functional types in the Canadian Terrestrial Ecosystem Model (CTEM) v. 2.0. Geosci. Model Dev. 9, 323–361. doi:10.5194/gmd-9-323-2016
Millington, J. D., Perkins, O., and Smith, C. (2022). Human fire use and management: a global database of Anthropogenic fire impacts for modelling. Fire 5, 87. doi:10.3390/fire5040087
Mueller, S. E., Thode, A. E., Margolis, E. Q., Yocom, L. L., Young, J. D., and Iniguez, J. M. (2020). Climate relationships with increasing wildfire in the southwestern US from 1984 to 2015. For. Ecol. Manage. 460, 117861. doi:10.1016/j.foreco.2019.117861
Mukunga, T., Forkel, M., Forrest, M., Zotta, R. M., Pande, N., Schlaffer, S., et al. (2023). Effect of socioeconomic variables in predicting global fire ignition occurrence. Fire 6, 197. doi:10.3390/fire6050197
Pais, C., Gonzalez-Olabarria, J. R., Elimbi Moudio, P., Garcia-Gonzalo, J., González, M. C., and Shen, Z. J. M. (2023). Global scale coupling of pyromes and fire regimes. Comm. Earth & Environ. 4, 267. doi:10.1038/s43247-023-00881-8
Pausas, J. G., and Keeley, J. E. (2009). A burning story: the role of fire in the history of life. BioSci 59, 593–601. doi:10.1525/bio.2009.59.7.10
Pausas, J. G., and Keeley, J. E. (2023). Evolutionary fire ecology: an historical account and future directions. BioSci. 73, 602–608. doi:10.1093/biosci/biad059
Pausas, J. G., Keeley, J. E., and Schwilk, D. W. (2017). Flammability as an ecological and evolutionary driver. J. Ecol. 105, 289–297. doi:10.1111/1365-2745.12691
Pereira, J. M., Oom, D., Silva, P. C., and Benali, A. (2022). Wild, tamed, and domesticated: three fire macroregimes for global pyrogeography in the Anthropocene. Ecol. Applic. 32, e2588. doi:10.1002/eap.2588
Pettinari, M. L., and Chuvieco, E. (2016). Generation of a global fuel data set using the Fuel Characteristic Classification System. Biogeosci 13, 2061–2076. doi:10.5194/bg-13-2061-2016
Ponomarev, E. I., Zabrodin, A. N., Shvetsov, E. G., and Ponomareva, T. V. (2023). Wildfire intensity and fire emissions in Siberia. Fire 6, 246. doi:10.3390/fire6070246
Popović, Z., Bojović, S., Marković, M., and Cerdà, A. (2021). Tree species flammability based on plant traits: a synthesis. STOTEN 800, 149625. doi:10.1016/j.scitotenv.2021.149625
Pyne, S. J. (2014). World fire: the culture of fire on earth. Seattle, Washington, United States: University of Washington Press.
Rabin, S., Melton, J. R., Laslop, G., Bachelet, D., Forrest, M., Hantson, S., et al. (2017). The Fire Modeling Intercomparison Project (FireMIP), phase 1: experimental and analytical protocols with detailed model descriptions. Geosci. Model Dev. 10, 1175–1197. doi:10.5194/gmd-10-1175-2017
Randerson, J., Chen, Y., van der Werf, G. R., Rogers, B., and Morton, D. (2012). Global burned area and biomass burning emissions from small fires. J. Geophys. Res.-Biogeo. 117, G04012. doi:10.1029/2012JG002128
Rodman, K. C., Veblen, T. T., Andrus, R. A., Enright, N. J., Fontaine, J. B., Gonzalez, A. D., et al. (2021). A trait-based approach to assessing resistance and resilience to wildfire in two iconic North American conifers. J. Ecol. 109, 313–326. doi:10.1111/1365-2745.13480
Rosan, T. M., Sitch, S., Mercado, L. M., Heinrich, V., Friedlingstein, P., and Aragão, L. E. O. C. (2022). Fragmentation-driven divergent trends in burned area in Amazonia and Cerrado. Front. For. Glob. Change 5. doi:10.3389/ffgc.2022.801408
Scott, A. C. (2000). The Pre-Quaternary history of fire. Palaeogeogr. Palaeoclimatol. Palaeoecol. 164, 281–329. doi:10.1016/s0031-0182(00)00192-9
Scott, A. C., and Glasspool, I. J. (2006). The diversification of Paleozoic fire systems and fluctuations in atmospheric oxygen concentration. Proc. Natl. Acad. Sci. U. S. A. 103, 10861–10865. doi:10.1073/pnas.0604090103
Shmuel, A., and Heifetz, E. (2022). Global wildfire susceptibility mapping based on machine learning models. Forests 13, 1050. doi:10.3390/f13071050
Shmuel, A., and Heifetz, E. (2023). Empirical evidence of reduced wildfire ignition risk in the presence of strong winds. Fire 6, 338. doi:10.3390/fire6090338
Simpson, K. J., Jardine, E. C., Archibald, S., Forrestel, E. J., Lehmann, C. E. R., Thomas, G. H., et al. (2021). Resprouting grasses are associated with less frequent fire than seeders. New Phytol. 230, 832–844. doi:10.1111/nph.17069
Singleton, M. P., Thode, A. E., Sanchez Meador, A. J., and Iniguez, J. M. (2019). Increasing trends in high-severity fire in the southwestern USA from 1984 to 2015. For. Ecol. Manage. 433, 709–719. doi:10.1016/j.foreco.2018.11.039
Teckentrup, L., Harrison, S. P., Hantson, S., Heil, A., Melton, J. R., Forrest, M., et al. (2019). Response of simulated burned area to historical changes in environmental and anthropogenic factors: a comparison of seven fire models. Biogeosci 16, 3883–3910. doi:10.5194/bg-16-3883-2019
Thonicke, K., Spessa, A., Prentice, I. C., Harrison, S. P., Dong, L., and Carmona-Moreno, C. (2010). The influence of vegetation, fire spread and fire behaviour on biomass burning and trace gas emissions: results from a process-based model. Biogeosci 7, 1991–2011. doi:10.5194/bg-7-1991-2010
Thonicke, K., Venevsky, S., Sitch, S., and Cramer, W. (2001). The role of fire disturbance for global vegetation dynamics: coupling fire into a dynamic global vegetation model. Glob. Ecol. Biogeog. 10, 661–677. doi:10.1046/j.1466-822x.2001.00175.x
Tracy, J. L., Trabucco, A., Lawing, M., Giermakowski, J. T., Tchakerian, M., Drus, G. M., et al. (2018). Random subset feature selection for ecological niche models of wildfire activity in Western North America. Ecol. Model. 383, 52–68. doi:10.1016/j.ecolmodel.2018.05.019
United Nations Environment Programme (UNEP) (2022). Spreading like wildfire – the rising threat of extraordinary landscape fires. Nairobi: A UNEP Rapid Response Assessment.
Vachula, R. S., Liang, J., Sae-Lim, J., and Xie, H. (2022). Ignition frequency and climate controlled Alaskan tundra fires during the Common Era. Quat. Sci. Rev. 280, 107418. doi:10.1016/j.quascirev.2022.107418
van Oldenborgh, G. J., Krikken, F., Lewis, S., Leach, N. J., Lehner, F., Saunders, K. R., et al. (2021). Attribution of the Australian bushfire risk to anthropogenic climate change. Nat. Hazards Earth Syst. Sci. 21, 941–960. doi:10.5194/nhess-21-941-2021
Veraverbeke, S., Rogers, B., Goulden, M., Jandt, R., Miller, C., Wiggins, E., et al. (2017). Lightning as a major driver of recent large fire years in North American boreal forests. Nature Clim. Change. Nat. Clim. Chang. 7, 529–534. doi:10.1038/nclimate3329
Wasserman, T. N., and Mueller, S. E. (2023). Climate influences on future fire severity: a synthesis of climate-fire interactions and impacts on fire regimes, high-severity fire, and forests in the western United States. Fire Ecol. 19, 43. doi:10.1186/s42408-023-00200-8
Williams, A. P., Abatzoglou, J. T., Gershunov, A., Guzman-Morales, J., Bishop, D. A., Balch, J. K., et al. (2019). Observed impacts of anthropogenic climate change on wildfire in California. Earth's Future 7, 892–910. doi:10.1029/2019EF001210
Yang, X., Zhao, C., Zhao, W., Fan, H., and Yang, Y. (2023). Characterization of global fire activity and its spatiotemporal patterns for different land cover types from 2001 to 2020. Environ. Res. 227, 115746. doi:10.1016/j.envres.2023.115746
Zhang, Y., Mao, J., Ricciuto, D. M., Jin, M., Yu, Y., Shi, X., et al. (2023). Global fire modelling and control attributions based on the ensemble machine learning and satellite observations. Sci. Remote Sens. 7, 100088. doi:10.1016/j.srs.2023.100088
Keywords: wildfire regimes, drivers of wildfire, empirical modelling, fuel availability, fuel continuity, fuel drying, wildfire modelling
Citation: Haas O, Keeping T, Gomez-Dans J, Prentice IC and Harrison SP (2024) The global drivers of wildfire. Front. Environ. Sci. 12:1438262. doi: 10.3389/fenvs.2024.1438262
Received: 25 May 2024; Accepted: 22 August 2024;
Published: 06 September 2024.
Edited by:
Chenghao Wang, University of Oklahoma, United StatesReviewed by:
Huilin Huang, Pacific Northwest National Laboratory (DOE), United StatesCopyright © 2024 Haas, Keeping, Gomez-Dans, Prentice and Harrison. This is an open-access article distributed under the terms of the Creative Commons Attribution License (CC BY). The use, distribution or reproduction in other forums is permitted, provided the original author(s) and the copyright owner(s) are credited and that the original publication in this journal is cited, in accordance with accepted academic practice. No use, distribution or reproduction is permitted which does not comply with these terms.
*Correspondence: Sandy P. Harrison, cy5wLmhhcnJpc29uQHJlYWRpbmcuYWMudWs=
Disclaimer: All claims expressed in this article are solely those of the authors and do not necessarily represent those of their affiliated organizations, or those of the publisher, the editors and the reviewers. Any product that may be evaluated in this article or claim that may be made by its manufacturer is not guaranteed or endorsed by the publisher.
Research integrity at Frontiers
Learn more about the work of our research integrity team to safeguard the quality of each article we publish.