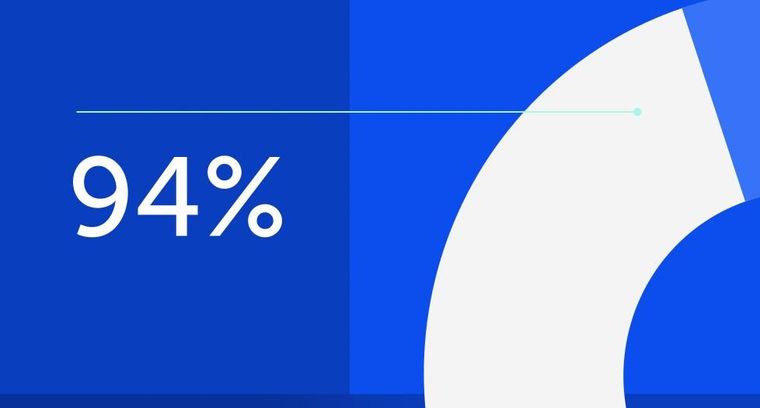
94% of researchers rate our articles as excellent or good
Learn more about the work of our research integrity team to safeguard the quality of each article we publish.
Find out more
ORIGINAL RESEARCH article
Front. Environ. Sci., 03 July 2024
Sec. Environmental Informatics and Remote Sensing
Volume 12 - 2024 | https://doi.org/10.3389/fenvs.2024.1435714
This article is part of the Research TopicTerritorial Spatial Evolution Process and Its Ecological Resilience, volume IIView all 10 articles
The rapid development of the digital economy is driving transformative changes in a multifaceted collaborative environmental governance system. From the perspective of collaborative governance between government and the public, this study employs double fixed-effects models, spatial econometric models, and instrumental variables methods to empirically explore how the digital economy influences environmental pollution, using panel data from 30 provinces in China spanning 2011 to 2022. The results demonstrate that the digital economy significantly lowers environmental pollution. The primary mechanism is through the government’s environmental governance behaviors, which are positively moderated by public environmental concerns, enhancing effectiveness. Additionally, the digital economy induces a spatial spillover effect on environmental pollution. This promotion of collaborative management between the government and the public is poised to become a pivotal direction in future environmental governance.
As one of the world’s largest energy consumers and carbon emitters, improving environmental pollution in China holds significant importance for the global environment. Yet, China’s environmental challenges are extremely severe. To tackle this, it’s crucial to actively embrace the new development concept, focus on building and enhancing an ecological civilization system, and unwaveringly pursue a green, circular, and low-carbon economic growth path.
The digital economy, which involves economic activities created by connecting individuals, organizations, and information systems via digital technology, is rapidly evolving (Carlsson, 2004; Sturgeon, 2021; Zhen et al., 2021; Zou and Deng, 2022). The influence of the digital economy on environmental pollution is a key focus among scholars. The digital economy reduces environmental pollution by fostering technological innovation and refining industrial structures (Zhang et al., 2023). It also enhances the integration of the real economy with industry using production factors such as knowledge, information, and IT, playing a vital role in boosting production efficiency (Pan et al., 2022), reducing carbon emissions (Xie et al., 2024), and advancing green transformations (Liu and Zhao, 2024). Technologies like artificial intelligence, big data, and industrial robots are reshaping industrial chains (Moyer and Hughes, 2012)and enhancing green total factor productivity (Chen et al., 2023; Wang et al., 2024), thus driving high-quality development (Wang et al., 2024). From this perspective, studying the impact of the digital economy on environmental pollution and the underlying mechanisms behind it is of significant importance for achieving green and sustainable development.
However, the synergistic mechanisms between government environmental governance and social public participation have not received adequate attention, presenting an opportunity to expand this area of research. The use of big data platforms and Internet technology can enhance the informatization of government environmental oversight, the scientific basis of environmental protection decisions, and deepen social participation (Wei and Zhang, 2023). These advancements improve the government’s capacity to manage the environment and further reduce environmental pollution levels. Notably, a diverse and shared environmental governance model is emerging in China, where the government leads and public participation supports. Public involvement acts as an informal environmental regulation (Tan and Eguavoen, 2017), compelling local governments to focus on environmental protection and increase invest-ments in environmental pollution control (Ge et al., 2021), which contributes to environmental improvement (Wang et al., 2023).
This study investigates how the development of digital economy impacts regional environmental pollution governance through collaborative efforts between the government and the public. The findings indicate that the digital economy significantly reduces environmental pollution levels. This reduction prompts local governments to increase investments in environmental pollution control, enhance their focus on environmental governance, and enforce environmental protection penalties, thereby improving regional environmental quality. These conclusions remain robust after various analyses, including the instrumental variable method and substituting explanatory variables. Public environmental concern also positively moderates the relationship between the digital economy and environmental pollution, indicating that the digital economy enhances environmental governance effectiveness through increased government and public interaction and participation.
The potential marginal contribution of this paper is twofold: Firstly, we use the co-management by multiple environmental stakeholders as the entry point, positioning the digital economy, governmental environmental governance, public environmental concern, and environmental pollution prevention within the same analytical framework to systematically assess the governance effects of the digital economy on environmental pollution. This encourages us to consider the synergistic effects of government environmental governance and social public participation, offering a fresh perspective for deeply understanding how the digital economy contributes to green development. Secondly, this paper delves into the specific transmission mechanisms through the interaction between local governments and the public, aiming to explore the long-term mechanisms of environmental governance facilitated by collaborative efforts between the government and the public.
The structure of the paper is as follows: Section 1 introduces the study. Section 2 reviews relevant literature and presents theoretical analyses. Section 3 outlines the research design, including variable selection, data sources, and model construction. Section 4 analyzes empirical results, covering baseline regression outcomes, impact mechanisms, moderating effects, and tests for spatial effects. Section 5 conducts robustness tests, utilizing approaches like the instrumental variables method, substitution of explanatory variables, and heterogeneity analysis. Finally, Section 6 offers conclusions and policy recommendations.
The rapid advancement and widespread adoption of digital information technologies—such as the Internet, big data, and artificial intelligence—not only inject new momentum into economic development but also facilitate the restructuring of the environmental governance system encompassing government, businesses, and society. This restructuring supports the economy’s transition to green and low-carbon operations. Specifically, within enterprises, the innovative breakthroughs in digital technology serve as a key driver for eco-friendly economic practices. As primary agents of pollution control, businesses utilize digital technologies to gather information and consolidate resources, enabling informed production decisions and enhancing operational efficiency (Zhang Rongwu et al., 2022). Moreover, the digital economy enhances knowledge dissemination efficiency in electronic equipment, communication networks, and information processing, encouraging enterprises to adopt green production models. This undoubtedly fosters technological innovation and industrial upgrading, ultimately contributing to both pollution reduction and green development (Xu et al., 2023).
In terms of governmental environmental governance, digital technology facilitates the development and application of ecological and environmental data, effectively collecting, integrating, and sharing critical information like pollution levels and environmental carrying capacity. This data supports dynamic assessments and supervision of governmental environmental efforts, improving pollution perception and early warning capabilities (Fang et al., 2024), and enhancing the precision and effectiveness of environmental supervision. This provides a robust data foundation for crafting environmental policies and refining the ecological regulation framework, thereby elevating the government’s role in environmental management (Shin and Choi, 2015).
Regarding public supervision and participation, the digital economy simplifies ac-cess to environmental information and raises public environmental awareness by enhancing public service platforms and fostering information exchange between the government and the community. The public can engage in environmental oversight through avenues such as social media, ensuring adherence to environmental regulations and governance policies, and supervising pollution activities and enforcement. This transformation of environmental consciousness into action facilitates collaborative governance of environmental pollution by both government and the public (Yang et al., 2020).
In conclusion, the development of digital economy impacts environmental pollution by promoting green production in enterprises, refining governmental environmental regulatory frameworks, and enhancing social and public oversight. Based on these insights, this paper proposes the following research hypothesis:
Hypothesis 1:. The development of digital economy will reduce environmental pollutant emissions, i.e., digital economic development contributes to effective environmental pollution management.
Reducing environmental pollution is a systematic project requiring the participation and synergy of multiple actors, including the government, enterprises, and the public. From the perspective of interactive synergy between the government and the public, analyzing the impact of the digital economy on the environmental governance behaviors of local governments can deepen our understanding of China’s environmental pollution governance model.
Firstly, the digital economy enables the government to efficiently collect, integrate, and share environmental data, scientifically assess government environmental governance performance, and enhance the accuracy and effectiveness of environmental supervision, thus boosting the government’s regulatory capacity (Zhao Shuliang et al., 2023). Additionally, the government can use digital technology to expand communication channels for knowledge diffusion, support environmental regulation, and improve policy formulation and implementation, which also increases government transparency (Peng et al., 2023), and improves environmental governance (Ahlers and Shen, 2018).
Secondly, investment in environmental pollution management reflects the commitment and effort of local governments in environmental governance. The digital economy drives local governments to increase their investments in environmental governance through digitized knowledge, information, technology, and other production factors, thereby elevating the level of ecological and environmental governance (Su et al., 2018; Zhu and Li, 2020).
Thirdly, the digital economy aids the development and utilization of environmental data and information, reducing information asymmetry between various government departments, businesses, and the public. This breaks down data barriers, forms a comprehensive ecological and environmental data system, and improves the transparency of local government environmental protection (Ahlers and Shen, 2018). The digital economy also enhances government environmental supervision and law enforcement capabilities, enabling the government to impose penalties on non-compliant businesses (Wu et al., 2024), strengthen the investigation and handling of environmental violations and penalties (Li Mingxian et al., 2023; Liu et al., 2024), and deter environmental non-compliance by businesses, which in turn reduces environmental pollution emissions.
Based on the above analysis, the following hypothesis is proposed:
Hypothesis 2:. The digital economy affects environmental pollution governance through the pathways of government environmental governance attention, environmental pollution governance investment, and environmental protection administrative enforcement efforts.
Social public participation, supplementing environmental regulation, supervises and influences local government environmental governance behaviors. With the rapid advancement of digital technology, the public can share social resources and create public service platforms using the Internet and big data. This platform model enables the public to easily access environmental information, express their opinions, and voice their dissatisfaction with pollution issues and demand for environmental quality improvement (Tan and Eguavoen, 2017). The theory of government responsiveness suggests that government environmental governance behaviors are influenced by and respond to regional public opinion, aligning environmental policies with public preferences (Arantes, 2023). In response to public environmental demands, the government reduces Environmental pollution by increasing attention to environmental governance, boosting investment in pollution control, and imposing administrative penalties for environmental protection (Sun et al., 2023; Liu et al., 2024).
On the other hand, under the Chinese environmental governance model, public satisfaction with environmental governance is a crucial metric for assessing local government performance. The digital economy promotes public environmental demands, participation, and supervision, compelling higher-level governments to motivate and oversee lower-level government environmental policies and behaviors, which assists in reducing local environmental pollution (Niu et al., 2024). As the primary responder, local governments attend to public environmental demands and actively respond through their governance practices, adjusting their environmental policy preferences, which undoubtedly enhances the environmental governance performance of local governments. The digital economy influences local government environmental policy adjustments and governance behaviors by elevating public environmental concerns and transforming public demands into active participation in environmental protection activities (Arantes, 2023).
The above logical mechanism is summarized as follows: The digital economy contributes to the interaction between the government and the public, influences public environmental awareness and behavior, and guides the public to pay attention to and participate in the process of environmental governance, thus fostering a governance system that is scientific in decision-making, refined in supervision, and convenient in service. By influencing local government environmental governance behaviors, public environmental demands compel local governments to enhance their focus on environmental governance, increase investments in environmental pollution control and enforcement, and ultimately aid in regional environmental pollution control.
As a result, the following hypothesis is derived:
Hypothesis 3:. Public environmental concern positively moderates the relationship between the digital economy and environmental pollution, enhancing the impact of digital economy on government environmental regulation.
The spatial spillover effect of the digital economy and environmental pollution is a crucial prerequisite for spatial measurement research. The spatial spillover effect of the digital economy has been extensively studied (Li Guangqin et al., 2023; Hou et al., 2023; Xu, 2024), with scholars exploring its impacts on rural revitalization (Li Guangqin et al., 2023) and industrial green innovation (Li Mingxian et al., 2023).
Conversely, the spatial spillover effect of environmental factors has also garnered significant attention (Liu et al., 2020; Zhang Maomao et al., 2022; Zhao Feng et al., 2023). This includes research on water pollution (Liu, et al., 2020), the interplay between urbanization and environmental pollution (Zhao Feng et al., 2023), the relationship between industrial agglomeration and environmental pollution (Zhang Maomao et al., 2022), and the mismatch of land resources contributing to environmental issues (Wan and Shi, 2022). Given that both the digital economy and environmental pollution exert influences on surrounding areas, it is conceivable that the digital economy might also impact environmental pollution in neighboring regions through spatial spillovers.
Thus, the following hypothesis is proposed:
Hypothesis 4:. The digital economy will exert spatial spillover effects on environmental pollution.
Environmental pollution level (Pol). The entropy weighting method is used to calculate a comprehensive environmental pollution index for regional industrial wastewater, sulfur dioxide emissions, and solid waste emissions as a measure of the level of environmental pollution in each region.
The explanatory variable in this paper is the digital economy (Dtf). Currently, defining the connotation of the digital economy is challenging, with many scholars understanding it as the sum of economic activities based on modern information technology (Carlsson, 2004; Sturgeon, 2021; Zhen et al., 2021; Zou and Deng, 2022). In this paper, we build on the work of Wang et al. (2021) to construct a digital economy development index using the entropy weight method. We select indicators from four dimensions: digital infrastructure, digital industrialization, industrial digitization, and digital innovation capability. The specific indicators are presented in Table 1.
This study identifies three core mechanistic variables to dissect the behavioral patterns of local governments in environmental governance. First, the degree of pre-existing attention to environmental governance (en) is quantified by analyzing the frequency of environmental and haze-related terms in the annual work reports of provincial governments. Secondly, the financial commitment to environmental pollution control (ei) is gauged by the total provincial investment in this area. Finally, the post-action intensity of enforcing environmental regulations (ez) is measured through the tally of environmental administrative penalty cases. These variables are designed to provide a comprehensive evaluation of the government’s dedication and efficacy in environmental protection.
The moderating variable, public environmental concern (pf), is represented by the Baidu haze search index. This choice is primarily due to Baidu’s status as the largest Chinese search engine, which offers extensive coverage and high data availability, allowing for detailed regional analysis based on search frequency and trends. Haze, as an environmental issue, tends to register a higher level of public awareness compared to other issues like environmental pollution, making it an ideal measure of environmental concern.
In a bid to thoroughly examine the digital economy’s influence on environmental pollution, this study introduces various control variables: fiscal freedom (ff), gauged by the ratio of fiscal revenue to fiscal expenditures; financial development level (fin), defined by the urban financial employment per 10,000 people; infrastructure (inf), assessed through the ratio of highway kilometers to developed area; medical care level (sin), measured by the number of practicing assistant physicians per 10,000 people; science and technology investment (tec), represented by the ratio of industrial enterprises’ R&D expenditures to regional GDP; education level (edu), based on the average higher education enrollment per 10,000 population; old-age burden (old), using the elderly dependency ratio; and parenting burden (chi), determined by the child dependency ratio.
Based on the results of the Hausman test (test value of 39.472, p-value of 0), the fixed effect model is deemed appropriate. Given that the data are panel data, and drawing on the methodology of Zhang et al. (2023), a double fixed-effect model is employed to analyze the impact of the digital economy on environmental pollution. The specific model (1) is presented as follows.
In Eq. 1, Poli,t represents the level of environmental pollution in province i during period t; Dtfi,t denotes the level of the digital economy in province i during the same period; the vector Zi,t includes a series of control variables for environmental pollution; μi symbolizes the individual fixed effect, while δt controls for the time fixed effect; εi,t is the random disturbance term.
Secondly, to explore the mechanisms through which the digital economy impacts environmental pollution, a transmission effect model is introduced as depicted in Eqs 2, 3. Here, itvi,t represents a series of mechanism variables through which the digital economy influences environmental pollution.
Thirdly, to assess the moderating effect of public environmental concern on the mechanism variables, this effect is captured in Eq. 4.
Additionally, the explanatory variables and the cross-multiplication term of each control variable with the spatial weight matrix are integrated into Eq. 1 to construct the Spatial Durbin Model (SDM), as detailed in Eq. 5. Here, φ2 represents the spatial spillover coefficient, and W is the spatial weight matrix.
Drawing on the approach of Zhang et al. (2023), 30 provinces in China (excluding Tibet, Taiwan, Hong Kong, and Macau) are selected as the research sample, covering the period from 2011 to 2022. The selected provinces are shown in Figure 1. The data for the dependent variables are sourced from the China Environmental Yearbook. Composite indicators for the dependent variables are derived from the digital finance Index of Peking University, the China Statistical Yearbook, and the respective statistical yearbooks of each province. The data for the control variables are also obtained from the China Statistical Yearbook and the provincial statistical yearbooks. Descriptive statistics for each variable are presented in Table 2.
Table 3 illustrates the results of a regression analysis on the impact of the digital economy on environmental pollution. As control variables were incrementally added to the regression model, the estimated coefficient for the core explanatory variable, the digital economy index (Dtf), consistently showed a significant negative effect. This strongly supports the hypothesis that the digital economy significantly mitigates or reduces environmental pollution, confirming Hypothesis 1.
Among the control variables, fiscal freedom’s impact on environmental pollution is also negative, suggesting that increased fiscal freedom provides local governments with more resources to combat environmental pollution. Financial development negatively correlates with environmental pollution; higher financial development levels likely channel more funds towards sustainable practices, thereby reducing pollution levels. However, the influence of infrastructure development on environmental pollution was found to be statistically insignificant. Medical care levels also negatively affect environmental pollution, implying that higher levels of healthcare lead to greater public awareness and concern for health, which in turn discourages environmental pollution. The impact of investments in science and technology on environmental pollution is also negative, reinforcing the idea that technological advancements drive energy efficiency and pollution reduction. Similarly, higher education levels correlate with reduced environmental pollution, as they foster more expertise in pollution prevention and control. The old-age dependency ratio negatively affects environmental pollution. Elderly populations, being more health-sensitive, tend to reside in areas with better environmental conditions, thus places with higher elderly care levels experience lower pollution. In contrast, the child dependency ratio has a positive impact on environmental pollution. In regions with higher fertility rates, which typically have more outdated production methods, environmental pollution is more severe.
Hypothesis 2 asserts that the development of the digital economy impacts the level of environmental pollution through pathways such as increased government focus on environmental governance, greater investment in pollution control, and more stringent environmental administrative law enforcement. This section provides an empirical examination of these mechanisms, with regression outcomes detailed in Table 4. Results from columns (7), (9), and (11) reveal that the digital economy substantially enhances government attention to environmental governance, boosts investment in environmental pollution control, and strengthens environmental administrative law enforcement. Furthermore, data from columns (6), (8), and (10) indicate that public environmental concern, environmental regulation level, green innovation level, and industrial structure significantly diminish environmental pollution levels. Consequently, the digital economy mitigates environmental pollution through mechanisms that influence government attention, investment in pollution control, and the enforcement of environmental laws, thus confirming Hypothesis 2.
Hypothesis 3 posits that public environmental concern positively moderates the relationship between the digital economy and environmental pollution, enhancing the impact of digital economy on government environmental regulation.
Table 5, column (12), demonstrates that the impact of the digital economy on public environmental concern is significantly positive at the 1% level, indicating that the digital economy boosts public environmental awareness. Columns (13), (14), and (15) show that after incorporating the interaction term between the digital economy and public environmental concern, the digital economy positively affects local government attention to environmental protection, investment in environmental pollution control, and environmental law enforcement efforts, all significant at least at the 10% level.
Moreover, the interaction term between the digital economy and public environmental concern is significantly positive, highlighting that the digital economy promotes government attention to environmental protection, environmental pollution control investment, and law enforcement efforts by increasing public awareness. In essence, the development of digital economy enhances environmental quality by fostering the synergy of public environmental concerns and prompting local governments to intensify their environmental governance efforts. This process is indicative of China’s evolving pattern of diverse and collaborative environmental governance, driven by the government with widespread public involvement, which plays a pivotal role in controlling environmental pollution and outlines the future trajectory of environmental governance. Hypothesis 3 is validated.
Hypothesis 4 believes that the digital economy will have a spatial spillover effect on environmental pollution. This part examines the spatial spillover effect of the digital economy. It is mainly divided into three parts: comparative analysis of spatial distribution maps, spatial autocorrelation test, and spatial econometric regression. The specific process is as follows:
Figure 2 shows the spatial distribution of the environmental pollution in 2011, 2016, and 2022. Overall, from 2011 to 2022, the environmental pollution showed a downward trend, indicating that the environmental pollution in China is improving. The provinces with a relatively high degree of environmental pollution are concentrated in the northern part of China, such as Inner Mongolia, Shanxi, and Hebei. The environmental pollution in the eastern coastal provinces is relatively low. The environmental pollution of each province shows certain characteristics of spatial agglomeration.
Figure 3 shows the spatial distribution of the digital economy of various provinces in China in 2011, 2016, and 2022. It can be seen that the level of China’s digital economy has achieved relatively large development from 2011 to 2022. Moreover, the development level of the digital economy in northern provinces is lower than that in the south. The development level of the digital economy in southeastern coastal provinces is relatively high, and there is also a certain degree of spatial agglomeration in the development level of the digital economy in space. By comparing Figure 2, it can be found that the environmental pollution of Inner Mongolia, Shanxi, Hebei and other provinces is at a relatively high position in the whole country, and the development level of the digital economy is at a relatively low position in the whole country. The environmental pollution of coastal provinces is at a relatively low level in the whole country, and the development level of the digital economy is at a relatively high position in the whole country. There is a certain correspondence in the spatial distribution between the two. In the following text, the spatial autocorrelation level of the environmental pollution and the development of the digital economy will be further tested.
The premise of the spatial econometric model is the existence of spatial correlation among the study variables. The Moran’s Index is used to test the spatial autocorrelation between the digital economy and environmental pollution from 2011 to 2022. Table 6 presents the Moran indices for both digital economy and environmental pollution levels using a geographical distance matrix. The results show significant spatial autocorrelation at the 1% level for the period studied, justifying further spatial econometric regression.
The outcomes from spatial measurement regressions are reported in columns (16) to (19) of Table 7, utilizing four different spatial weight matrices: neighborhood distance, geographic distance, economic distance, and an economic-geographic nested matrix. The results across these matrices are consistent. However, the analysis focuses on the economic-geographic nested matrix shown in column (19), which combines both economic and geographic factors. Note that the spatial econometric model is uniquely characterized, particularly in column (19), rows 3 and 4, which highlight the regression coefficients for the digital economy and its interaction with the spatial weights. The significance of these coefficients primarily indicates whether the digital economy directly influences environmental pollution. However, the actual existence and extent of its spatial impacts require further analysis using spatial econometric modeling techniques, such as partial differentiation; the actual values of the coefficients themselves are less critical here. Detailed examination of the direct and spillover effects is required through partial differentiation, presented in rows 6 and 7 of column (19).
As evident from the third row of column 19, the direct impact of the digital economy on environmental pollution is negative, indicating that the growth of the digital economy within a region is likely to reduce its environmental pollution. Similarly, the fourth row reveals a negative coefficient for the interaction term between the digital economy and the spatial weight matrix. This suggests that while the digital economy may decrease environmental pollution within a region, it can concurrently mitigate pollution in surrounding areas as well. This may stem from the demonstrative effect of the digital economy, where as it prompts an increase in environmental regulatory efforts by local governments, neighboring governments may also face pressure from performance evaluation and public attention, leading to enhanced environmental pollution management. Consequently, when the digital economy reduces environmental pollution in a given region, it tends to have a similar effect in neighboring areas. Thus, Hypothesis 4 is confirmed.
Drawing on the methodology of Nunn and Qian (2014) and Zhang et al. (2023), we use historical data on post and telecommunications from provinces in 1984 as a basis, combined with the number of Internet users in the country from 2011 to 2022, to construct an instrumental variable. This approach is chosen because the development of the digital economy is closely related to local infrastructure such as postal and telecommunication services, thus satisfying the requirement for correlation between the instrumental variable and the explanatory variables. Additionally, the impact of postal and telecommunication infrastructure on environmental pollution has become negligible over time, meeting the exogeneity requirement of the instrumental variable. Please refer to Table 8 for the relevant regression results.
The results from Table 8 confirm that the digital economy continues to have a significant negative impact on environmental pollution, even after addressing the endogeneity issue. Furthermore, the instrumental variables cleared the LM test with F-values exceeding 10, affirming their statistical validity and appropriateness. These findings robustly support a deeper exploration of the interplay between the digital economy and environmental pollution.
We substituted the main explained variable for its subcomponents sulfur dioxide emissions, water pollutants, and solid pollutant discharge to verify the robustness of our regression analysis. The modified regression outcomes, presented in Table 9, demonstrate that the results remain robust even after this substitution. This further solidifies the reliability of our regression findings.
At present, various regions in China are at different stages of industrialization and economic development levels, which leads to differences in both environmental pollution and the development of the digital economy. Therefore, in accordance with China’s regional planning standards, specifically as shown in Figure 4, it is necessary to analyze the sub-samples from the three regions in order to better understand these differences. The regression results are presented in Table 10.
Table 10 reveals that the impact of the digital economy on environmental pollution is negative across eastern, central, and western regions. However, the most substantial negative impact is observed in the western region, followed by the central and the least in the eastern region. This trend may be attributed to the high level of environmental pollution and the relatively undeveloped the digital economy in the western region, resulting in the largest marginal utility of the digital economy interventions on environmental pollution. In contrast, the digital economy in the eastern region, being more developed and shows a diminishing marginal effect due to lower levels of environmental pollution. Despite this, the development of digital economy remains crucial in the eastern region, which possesses more experience in reducing environmental pollution through digital means. Therefore, the central and western regions could benefit significantly from adopting the eastern region’s strategies.
This study constructs a digital economy development indicator system for 30 provinces spanning from 2011 to 2022, taking into account both government environmental governance and public environmental concerns as the starting points. Utilizing fixed-effect models, spatial econometric models, and an instrumental variable system, it examines the mechanisms of how digital economy development contributes to environmental pollution control. The key findings are as follows: 1) The development of the digital economy significantly reduces environmental pollution, promoting regional green transformation and development. 2) This reduction in environmental pollution is achieved through the government’s pre-event focus on environmental governance, in-event investment in pollution control, and post-event enforcement efforts. 3) Public environmental concern positively moderates the relationship between the digital economy and environmental pollution, enhancing the impact of the digital economy on government environmental regulations. 4) The impact of the digital economy on environmental pollution exhibits a spatial spillover effect, where the development of the digital economy reduces pollution in a given region while also lowering pollution levels in surrounding areas.
According to the conclusion, the following suggestions are made:
(1) Vigorously promote the development of the Dtf and improve relevant policies and safeguard systems. Promote the Dtf: strengthen the construction of infrastructure such as 5G and big data, promote the digital transformation of industry, develop new business forms such as platform economy, innovate the application of technology, improve data management and laws and policies, optimize the business environment, train digital talents, narrow the digital divide, and build a healthy and sustainable Dtf ecology.
(2) Give full play to the guiding role of the Dtf in green development through government environmental governance. We will strengthen local governments’ attention to environmental governance and enhance their environmental supervision capabilities. We will increase investment in Pol control and improve the government’s ability to improve the ecological environment. Strengthen government environmental law enforcement and administrative penalties through information and technology, so as to promote Pol reduction.
(3) Improve the construction of government public service platforms and improve the channels for the public to express environmental demands. The Dtf will guide the public to pay attention to and participate in environmental governance. With the help of digital technologies and platforms, local governments and social forces are encouraged to interact and cooperate in Pol control, and a collaborative environmental governance system with government supervision as the leading role and public participation as the auxiliary role is better established, so as to improve the public’s participation in and supervision of government environmental governance, and thus strengthen the effect of Pol control.
(4) To harness the positive spatial spillover effects of the digital economy on environmental pollution, we should enhance inter-regional communication and cooperation. Through regular dialogues, information sharing, personnel training, and exchange programs, we can facilitate the transfer of technology and expertise from advanced regions to less-developed ones. Additionally, leveraging the technological advantages of the digital economy, we should promote green digital technologies, optimize industrial layouts, and strengthen environmental oversight, ultimately achieving the coordinated development of the digital economy and environmental protection.
Publicly available datasets were analyzed in this study. This data can be found here: https://idf.pku.edu.cn/yjcg/zsbg/index.htm.
KL: Conceptualization, Software, Writing–original draft. FM: Data curation, Methodology, Writing–review and editing.
The author(s) declare that no financial support was received for the research, authorship, and/or publication of this article.
The authors declare that the research was conducted in the absence of any commercial or financial relationships that could be construed as a potential conflict of interest.
All claims expressed in this article are solely those of the authors and do not necessarily represent those of their affiliated organizations, or those of the publisher, the editors and the reviewers. Any product that may be evaluated in this article, or claim that may be made by its manufacturer, is not guaranteed or endorsed by the publisher.
Ahlers, A. L., and Shen, Y. (2018). Breathe easy? Local nuances of authoritarian environmentalism in China's battle against air pollution. China Q. 234 (8), 299–319. doi:10.1017/s0305741017001370
Arantes, V. (2023). DING, iza. 2022. The performative state: public scrutiny and environmental governance in China. Ithaca: cornell university press. China Perspect. 132, 75–76. doi:10.4000/chinaperspectives.14719
Carlsson, Bo (2004). The Digital Economy: what is new and what is not? Struct. Change Econ. Dyn. 15 (3), 245–264. doi:10.1016/j.strueco.2004.02.001
Chen, H., Ma, Z., Xiao, H., Li, J., and Chen, W. (2023). The impact of digital economy empowerment on green total factor productivity in forestry. Forests 14 (1729), 1729. doi:10.3390/f14091729
Fang, J., Zhao, L., and Li, S. (2024). Exploring open government data ecosystems across data, information, and business. Gov. Inf. Q. 41 (2), 101934. doi:10.1016/j.giq.2024.101934
Ge, T., Xionglei, H., and Li, J. (2021). Effects of public participation on environmental governance in China: a spatial Durbin econometric analysis. J. Clean. Prod. 321, 129042. doi:10.1016/j.jclepro.2021.129042
Hou, L., Tian, C., Xiang, R., Wang, C., and Gai, M. (2023). Research on the impact mechanism and spatial spillover effect of digital economy on rural revitalization: an empirical study based on China’s provinces. Sustainability 15 (15), 11607. doi:10.3390/su151511607
Li, G., Li, X., and Huo, L. (2023a). Digital economy, spatial spillover and industrial green innovation efficiency: empirical evidence from China. Heliyon 9, e12875. doi:10.1016/j.heliyon.2023.e12875
Li, M., Zou, S., and Jing, P. (2023b). Spatial spillover effect of water environment pollution control in basins—based on environmental regulations. Water 15 (21), 3745. doi:10.3390/w15213745
Liu, J., and Zhao, Q. (2024). Mechanism testing of the empowerment of green transformation and upgrading of industry by the digital economy in China. Front. Environ. Sci. 11. doi:10.3389/fenvs.2023.1292795
Liu, X., Deng, L., Dong, X., and Li, Q. (2024). Dual environmental regulations and corporate environmental violations. Finance Res. Lett. 62 (8), 105230. doi:10.1016/j.frl.2024.105230
Liu, X., Sun, T., and Feng, Q. (2020). Dynamic spatial spillover effect of urbanization on environmental pollution in China considering the inertia characteristics of environmental pollution. Sustain. Cities Soc. 53, 101903. doi:10.1016/j.scs.2019.101903
Moyer, J. D., and Hughes, B. B. (2012). ICTs: do they contribute to increased carbon emissions? Technol. Forecast. Soc. Change 79 (5), 919–931. doi:10.1016/j.techfore.2011.12.005
Niu, X., Ma, Z., Ma, W., Yang, J., and Mao, T. (2024). The spatial spillover effects and equity of carbon emissions of digital economy in China. Journal of Cleaner Production 434, 139885. doi:10.1016/j.jclepro.2023.139885
Nunn, N., and Qian, N. (2014). US food aid and civil conflict. Am. Econ. Rev. 104 (6), 1630–1666. doi:10.1257/aer.104.6.1630
Pan, W., Xie, T., Wang, Z., and Ma, L. (2022). Digital economy: an innovation driver for total factor productivity. J. Bus. Res. 139, 303–311. doi:10.1016/j.jbusres.2021.09.061
Peng, M., Peng, S., Jin, Y., and Wang, S. (2023). Government environmental information disclosure and corporate carbon performance. Front. Environ. Sci. 11, 1204970. doi:10.3389/fenvs.2023.1204970
Shin, D.-H., and Choi, M. J. (2015). Ecological views of big data: perspectives and issues. Telematics Inf. 32 (2), 311–320. doi:10.1016/j.tele.2014.09.006
Sturgeon, T. (2021). Upgrading strategies for the digital economy. Glob. Strategy J. 11 (1), 34–57. doi:10.1002/gsj.1364
Su, P., Lin, D. G., and Qian, C. (2018). Study on air pollution and control investment from the perspective of the environmental theory model: a case study in China, 2005-2014. Sustainability 10 (7), 2181. doi:10.3390/su10072181
Sun, J., Hu, J., Wang, H., Shi, Y., Wei, Z., and Cao, T. (2023). The government’s environmental attention and the sustainability of environmental protection expenditure: evidence from China. Sustainability 15 (11163), 11163. doi:10.3390/su151411163
Tan, J., and Eguavoen, I. (2017). Digital environmental governance in China: information disclosure, pollution control, and environmental activism in the yellow river delta. Water Altern. - Interdiscip. J. Water Polit. Dev. 10 (1), 910–929.
Wan, Q., and Shi, D. (2022). Smarter and cleaner: the digital economy and environmental pollution. China and World Econ. 30 (6), 59–85. doi:10.1111/cwe.12446
Wang, J., Zhu, J., and Luo, X. (2021). Measurement of China's digital economy development level and evolution (in Chinese). Quantitative Econ. Tech. Econ. Res. 38, 26–42. doi:10.13653/j.cnki.jqte.2021.07.002
Wang, L., Liu, B., He, Y., Dong, Z., and Wang, S. (2023). Have public environmental appeals inspired green total factor productivity? empirical evidence from Baidu Environmental Search Index. Environ. Sci. Pollut. Res. 30 (11), 30237–30252. doi:10.1007/s11356-022-23993-8
Wang, S., Zheng, Y., and Yang, H. (2024). Digital economy and green total factor productivity in China. PloS one 19 (3), e0299716. doi:10.1371/journal.pone.0299716
Wei, J., and Zhang, X. (2023). The role of big data in promoting green development: based on the quasi-natural experiment of the big data experimental zone. Int. J. Environ. Res. public health 20 (5), 4097. doi:10.3390/ijerph20054097
Wu, Y., Hu, J., Irfan, M., and Hu, M. (2024). Vertical decentralization, environmental regulation, and enterprise pollution: An evolutionary game analysis. J. Environ. Manage. 349, 119449. doi:10.1016/j.jenvman.2023.119449
Xie, B., Liu, R., and Dwivedi, R. (2024). Digital economy, structural deviation, and regional carbon emissions. J. Clean. Prod. 434, 139890. doi:10.1016/j.jclepro.2023.139890
Xu, J. (2024). Spatial spillover and threshold effects of digital rural development on agricultural circular economy growth. Front. Sustain. Food Syst. 8 (24). doi:10.3389/fsufs.2024.1337637
Xu, Q., Li, X., Dong, Y., and Guo, F. (2023). Digitization and green innovation: how does digitization affect enterprises’ green technology innovation? J. Environ. Plan. Manag., 0964–0568. doi:10.1080/09640568.2023.2285729
Yang, J., Li, X., and Huang, S. (2020). Impacts on environmental quality and required environmental regulation adjustments: a perspective of directed technical change driven by big data(Article). J. Clean. Prod. 275, 124–126. doi:10.1016/j.jclepro.2020.124126
Zhang, M., Tan, S., Pan, Z., Daoqing, H., Zhang, X., and Chen, Z. (2022a). The spatial spillover effect and nonlinear relationship analysis between land resource misallocation and environmental pollution: evidence from China. J. Environ. Manag. 321, 115873. doi:10.1016/j.jenvman.2022.115873
Zhang, R., Fu, W., and Yingxu, K. (2022b). Can digital economy promote energy conservation and emission reduction in heavily polluting enterprises? Empirical evidence from China. Int. J. Environ. Res. public health 19 (16), 9812. doi:10.3390/ijerph19169812
Zhang, X., Zhong, J., and Wang, H. (2023). Does the development of digital economy affect environmental pollution? Sustainability 15 (2), 9162. doi:10.3390/su15129162
Zhao, F., Yi, S., and Zhang, J. (2023a). Does industrial agglomeration and environmental pollution have a spatial spillover effect? taking panel data of resource-based cities in China as an example. Environ. Sci. Pollut. Res. Int. 30 (31), 76829–76841. doi:10.1007/s11356-023-27852-y
Zhao, S., Shuliang, Z., Linjiao, T., Arkorful, V. E., and Hui, H. (2023b). Impacts of digital government on regional eco-innovation: moderating role of dual environmental regulations. Technol. Forecast. Soc. Change 196, 122842. doi:10.1016/j.techfore.2023.122842
Zhen, Z., Yousaf, Z., Radulescu, M., and Yasir, M. (2021). Nexus of digital organizational culture, capabilities, organizational readiness, and innovation: investigation of SMEs operating in the digital economy. Sustainability 13, 720. doi:10.3390/su13020720
Zhu, X., and Li, B. (2020). Convergence of efficiency of environmental pollution control investment in the coastal areas of China. J. Coast. Res. 105, 171–175. doi:10.2112/jcr-si105-036.1
Keywords: digital economy, environmental pollution, environmental governance, coordinated governance, spatial spillover
Citation: Liu K and Ma F (2024) The impact of the digital economy on environmental pollution: a perspective on collaborative governance between government and Public. Front. Environ. Sci. 12:1435714. doi: 10.3389/fenvs.2024.1435714
Received: 20 May 2024; Accepted: 12 June 2024;
Published: 03 July 2024.
Edited by:
Xiao Ouyang, Hunan University of Finance and Economics, ChinaReviewed by:
Bo Liu, Hunan Agricultural University, ChinaCopyright © 2024 Liu and Ma. This is an open-access article distributed under the terms of the Creative Commons Attribution License (CC BY). The use, distribution or reproduction in other forums is permitted, provided the original author(s) and the copyright owner(s) are credited and that the original publication in this journal is cited, in accordance with accepted academic practice. No use, distribution or reproduction is permitted which does not comply with these terms.
*Correspondence: Fanglin Ma, Mjg1ODQ2MzAwN0BxcS5jb20=
Disclaimer: All claims expressed in this article are solely those of the authors and do not necessarily represent those of their affiliated organizations, or those of the publisher, the editors and the reviewers. Any product that may be evaluated in this article or claim that may be made by its manufacturer is not guaranteed or endorsed by the publisher.
Research integrity at Frontiers
Learn more about the work of our research integrity team to safeguard the quality of each article we publish.