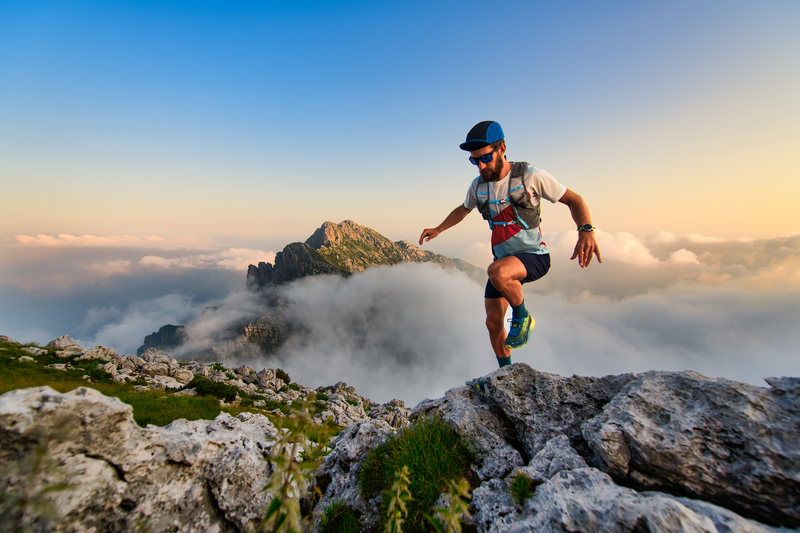
94% of researchers rate our articles as excellent or good
Learn more about the work of our research integrity team to safeguard the quality of each article we publish.
Find out more
ORIGINAL RESEARCH article
Front. Environ. Sci. , 30 August 2024
Sec. Environmental Economics and Management
Volume 12 - 2024 | https://doi.org/10.3389/fenvs.2024.1432534
This article is part of the Research Topic Urban Carbon Emissions and Anthropogenic Activities View all 14 articles
Digital technology plays a vital role in driving toward a zero-carbon future. This paper explores whether new infrastructure, serving as carriers for digital technology, contributes to carbon reduction and efficiency gains in cities. Drawing on panel data from 280 Chinese cities spanning 2011 to 2019, we establish a theoretical analytical framework to investigate how new infrastructure influences urban carbon performance through the perspectives of influence, threshold, and spatial spillover effects. The study reveals that new infrastructures development has a contributing effect on the total factor carbon productivity of cities, with consistent results across various testing methods. Additionally, the threshold effect test suggests that the dual threshold of regional energy consumption influences the enhancing effect of new infrastructure on total factor carbon productivity, exhibiting nonlinear characteristics. Furthermore, the spatial spillover effect test suggests that new infrastructure construction accelerates the local carbon emission performance, while having a positive spillover effect on neighboring cities. This study provides innovative ideas and experiences from China for the global realization of simultaneous promotion of digital economy development and energy conservation and emission reduction.
With the worldwide acknowledgement of the necessity to control greenhouse gas emissions, 151 countries have committed to carbon-neutral climate goals, encompassing 94% of the global economies and 86% of the world’s population (Matthews et al., 2009; ICON, 2023). International green initiatives are expanding in scale and influence to achieve a sustainable global future (Arbolino et al., 2018). Major global economies have clarified their decarbonization schedules and are actively progressing toward a zero-carbon future.
Despite this, the tension between economic growth and carbon emissions persists globally. Any effective climate change policy must simultaneously aim to stabilize greenhouse gas levels in the atmosphere and promote sustained economic growth, thereby fostering the development decoupled from CO2 emissions. Developing nations’ economies, such as China, are shifting from high growth to high-quality development. Improvement in factor productivity serves as a core indicator for assessing the quality of economic transformation (Solow, 1957; Khanna and Sharma, 2021). Compared with traditional productivity, total factor carbon productivity (TFCP) serves as a link between economic development and carbon emissions. It has become an important metric for reconciling economic growth with changes in carbon emissions. TFCP is also gaining traction in academic research. Studies have demonstrated that the advances in green technology advances, renewable energy innovations, and the opening of high-speed railways can serve as TFCP engines (Du and Li, 2019; Zhou and Tang, 2021; Lin and Jia, 2022).
In December 2018, the concept of “new infrastructure (NI) ” was introduced at China’s Central Economic Work Conference and included in the government’s work report. NI is an infrastructure system based on the concept of new development, driven by technological innovation and supported by information networks. It can be instrumental to economic growth (Fernández-Portillo et al., 2020), technological innovation (Koutroumpis et al., 2020), industrial structure upgrading (Wu et al., 2023), and enhanced factor productivity (Mitra et al., 2016). Overall, NI has become a crucial indicator of national competitiveness in the digital economy age. However, there is an ongoing debate about the effectiveness of NI in promoting low carbon development. Numerous studies focusing on specific types of NI have suggested that digitization can lead to win-win situation for national prosperity and carbon reduction (Madlener et al., 2022; Kou and Xu, 2022). However, some scholars believe that the construction and operation of NI may promote energy-intensive production, leading to a rebound effect that exacerbates energy consumption and potentially decreases carbon efficiency (Wang et al., 2022). Consequently, the question of whether NI can enhance TFCP still needs to be answered in some instances.
This study utilizes data from city-level studies in China to investigate the impact of developing NI on TFCP. The motivation for this research stems from several factors. First, global carbon dioxide emissions reach a record high of 37.4 billion tons in 2023, according to the data of CO2 emission in 2023 releasing by the International Energy Agency (IEA). Among them, carbon emissions of China are 12.6 billion tons, and ranking first in the world. As the world’s largest energy consumer, China is committed to achieving the highest reduction rate in carbon emission intensity globally and bears a significant responsibility to accelerate the adoption of low-carbon and green industries. Cities are the main geographical unit of carbon emissions in China, accounting for about 85% of the total carbon emissions (Mi et al., 2016), so improving carbon performance at the city level in China is the key to realize low carbon development. Second, unlike developed nations, developing countries face the dual challenges of environmental preservation and economic growth, which necessitates striking a balance between economic development and climate change. Third, as a rapidly emerging economy, China possesses considerable investment and consumption demands. Its NI construction has yielded substantial results through systematic development and large-scale deployment. According to the data provided by the Ministry of Industry and Information Technology (MIIT, 2024), as of 2023, China has 64.32 million km of fibre-optic cable lines, and over 3.377 million 5G base stations. Moreover, China is developing NI for the construction of the “Belt and Road.” to promote deeper global integration.
In summary, with China as the research object, the present study seeks to address the following questions: How effective is the development of NI in reducing carbon at the city level? Is there a heterogeneous threshold effect for NI’s lifting effect on TFCP? Are there spatial spillovers from NI influencing TFCP, and what is its regional radiative effect? The present study aims to address these question. The research results will provide additional empirical support for the rise of emerging economies, global responses to climate change, and the transition of development modes.
Compared with existing literature, this paper makes several distinct contributions. First, according to the connotation of NI, the paper assesses NI levels at the city level, employing a nuanced scale encompassing information, integration, and innovation dimensions. Second, it extends the dividend effect of NI from the economic realm to the low-carbon domain. By effectively integrating measures of economic growth and carbon emissions, it comprehensively examines the impact of NI on reducing carbon emissions through the TFCP indicator. Third, using a threshold econometric model, the paper reveals that the promotional effect of NI on urban TFCP is influenced by dual threshold of regional energy consumption, exhibiting nonlinear characteristics. As energy usage decreases, the positive promotional impact of NI on urban carbon performance gradually strengthens. Applying the Durbin spatial model, the study reveals that NI not only positively impacts the region’s carbon performance improvement but also generates positive spillover impacts on neighboring cities in terms of relieving pressure on carbon emissions.
The rest of the paper is organized as follows: Section 2 reviews pertinent literature. Section 3 outlines the theoretical framework and necessary hypotheses. Section 4 delineates the research design, encompassing the econometric model, variables, and data. Section 5 presents empirical findings from the benchmark regression. Section 6 further examines threshold and spatial effects based on empirical results. The final section presents conclusions with policy recommendations.
Currently, academics base their empirical analyses on the economic efficiency effects of new digital infrastructures, affirming their dual role in enhancing the speed and quality of economic advancement. At the macro level, Czernich et al. (2011) in their examination of a sample of OECD data, confirmed that broadband infrastructure enables high-speed Internet access, thereby positively influencing economic growth through increased penetration. Du et al. (2022) observed that new infrastructure investment can propel sustainable economic advancement by leveraging technological innovation, upgrading industrial structures, and increasing production efficiency as transmission pathways. At the micro level, Mitra et al. (2016) posited that information infrastructure offers novel avenues for firms to acquire and utilize information resources, consequently fostering technological efficiency and enhancing factor productivity.
In terms of environmental effects, studies at the macro level have found that the development of new digital infrastructures can enhance the efficient use of energy, thus leading to a reduction in pollution (Wu et al., 2021). At the micro level, studies have shown that the development of digital infrastructure can promote green transformation of enterprises (Guo et al., 2024b).
As the emphasis on carbon neutrality in the digital age increases, scholars are beginning to discuss the carbon reduction effects of NI. Most studies have assessed the climate impact of specific types of NI on carbon emissions. Danish et al. (2018), Yang et al. (2022), and Ai and Yan, 2024 employed direct measures of NI, such as length of fiber-optic cable lines, the Broadband China Policy and capital stock. However, their findings regarding the influence of NI on the total carbon emissions of developing nations have been inconsistent. Some researchers have explored the impact of NI on urban per capita carbon emissions and carbon intensity by constructing an NI composite index (Tang and Yang, 2023; Zhang et al., 2023a).
The relation between economic development and zero-carbon targets is viewed as integrated and mutually reinforcing. Carbon performance has garnered scholarly attention because it connects enhanced economic performances with reduced carbon emissions. However, research on the impact of NI on carbon performance is still in its early stages. Given the limitations of assessing carbon performance by using a single metric (such as carbon productivity or carbon intensity), few scholars have initiated an exploratory investigation into the comprehensive effects of NI on ecological performance from the TFCP perspective. Hu et al. (2023) discovered that developing NI positively affects urban low-carbon development, demonstrating beneficial spatial spillover effects. This “low-carbon dividend” exhibits a Matthew effect and is prominent only in regions with abundant low-carbon endowments. Lan and Zhu (2023) argued that the impact of NI on TFCP exhibits nonlinear characteristics, and the rebound effect complicates the role of NI in carbon emission reduction.
Synthesizing the results of existing research, it can be inferred that NI is a new engine to promote high-quality economic development; however, there are limitations in the research on its low-carbon effect: First, much of the existing studies have either focused on NI’s economic performance at national, regional, and enterprise levels or explored its environmental impact through single indicators such as total carbon emissions and carbon intensity. This approach makes it challenging to accurately assess NI’s comprehensive effects on a city’s carbon reduction and efficiency enhancement behaviors. Second, some studies have used specific proxies such as Internet penetration or policy dummy variables to represent NI, which may not accurately capture the level of developing NI at the city level. Due to limitations of previous studies regarding the relation between NI and carbon performance, the causal relation between the two remains to be clarified. There is a need to comprehensively characterize NI and its positive impact on urban green, low-carbon, and high-quality development. Third, the adverse effects of NI on carbon emission reduction have garnered more attention, and its positive impacts and the role of spatial spillovers in alleviating emission reduction pressures need further analysis.
In view of this, this paper links carbon emissions to economic development and measures the impact of NI on the efficiency of urban low-carbon economic development through TFCP indicator that considering multiple input and output factors. We innovatively constructed a system of indicators to measure the level of infrastructure development at the city level from the three dimensional layers of information, integration and innovation. The enhancement effect of NI on urban TFCP is also empirically examined, further revealing the positive spillover impact of NI on the low-carbon development of neighboring regions.
NI serves as the cornerstone of intelligent city construction and is fundamental for enabling the digital economy to bring about environmental improvements. Focusing on the connotation dimension of NI, we emphasize three aspects, namely information, integration, and innovation, which are aimed at promoting carbon reduction in cities.
First, information infrastructure lays the foundation for the comprehensive development of the urban digital economy. It bears the transmission of various information technologies such as big data, the Internet of things, artificial intelligence, and digital twins, establishing a collaborative network across the city. According to the resource-energy hypothesis, resources and energy are endogenously substitutable (Acemoglu et al., 2012). This implies that as the scale of information capital expands, information infrastructure can be dematerialized by high-tech information technologies, thereby optimizing and replacing enterprise production, processing, marketing, and management processes. Consequently, the result in reduced production costs and energy consumption reduce, thereby contributing to energy conservation and decarbonization (Zhong et al., 2022; Fu et al., 2023).
Second, the deep integration of digital technology into production and life scenarios plays a crucial role in fostering low-carbon urban development by reshaping industrial processes and promoting intelligent living. On one hand, the integration of digital technology within industries has effectively promoted specialized labor divisions in production. This integration has deeply permeated crucial carbon-emitting industries like petrochemicals, electricity, industry, construction, and transportation, facilitating whole-industry and whole-chain digital transformation and low-carbon industrial process re-engineering, concurrently leading to improvements in both carbon emissions and production efficiency (Dedrick et al., 2013). On the other hand, integrating NI into smart city frameworks encourages the gradual transformation of residents’ lives toward paperless, advanced, and low-carbon alternatives. NI facilitates the realization of intelligent living, including digital payments, smart travel, and online healthcare, streamlining residents’ daily behaviors and thereby reducing urban carbon emissions by improving travel efficiency and promoting low-carbon consumption (Mouratidis et al., 2021).
Third, innovation infrastructure with public goods attributes bolsters scientific research, technical advancement, and environmental protection, while furnishing technological dividends for urban units to engage in environmental governance. This enables environmental regulators to dynamically and promptly monitor production emissions, thereby achieving the process control of carbon emissions. Establishing a precise carbon information service platform through digital innovation lays the groundwork, which will work together with energy-consuming rights trading policy and environmental information disclosure system, for enhancing governmental digital carbon management and the effectiveness of digital carbon reduction efforts (Guo et al., 2024a; Fang et al., 2022; Wang et al., 2024). In light of these observations, this study proposes Hypothesis 1.
H1:. Developing NI can enhance urban TFCP.
During the industrialization phase, developing countries have heavily relied on extensive resource consumption in exchange for GDP growth, leading to a steady rise in carbon emissions. This rough development model poses challenges to the sustainability of economic growth and may lead to “productivity paradox”. Consequently, the efficacy of NI to promote TFCP is difficult to release. Additionally, developing countries often lack a robust foundation for advancing the digital economy, leading to increased energy consumption in pre-development stage of NI and a delay in carbon reduction efforts. In the early stages of development, the digital transformation of resource-dependent industries by NI, may boost their production efficiency, thereby stimulating higher energy consumption and impeding the Improvement in TFCP.
With dual-control constraints on the total amount and intensity of energy consumption, NI is propelled by digital advancements, instigating profound transformations within the energy sector, thereby diminishing the reliance of economic growth on energy consumption. Cities boasting larger economies, diverse industry types, and lower resource dependence, facilitating the scaling up of NI, are more prone to reap the benefits from the positive effects of NI on carbon performance (Du et al., 2023). Therefore, the impact of NI on TFCP may be constrained by the characteristics of regional energy consumption thresholds. The amalgamation of NI and energy constraints can effectively bolster TFCP.
H2:. The effect of carbon emission reduction from new infrastructure development depends heterogeneously on regional energy consumption. NI’s positive facilitating effect on enhancing urban carbon performance gradually increases as energy consumption decreases.
As the cornerstone of the digital economy, NI’s roles in enhancing the efficiency of regional economic development have been validated. Drawing from Metcalfe’s law of network technology development, NI effectively transcends the spatial and temporal constraints of information exchange, facilitating the cross-regional flow of high-value elements and generating spatial spillover effects (Du et al., 2023; Yan et al., 2022). With its universality and shareability, NI empowers the digital economy to break through geographical constraints, thereby unleashing the diffusion effect and influencing “local-neighborhood” economic activities (Bronzini and Piselli, 2009). Consequently, NI not only impacts the region’s TFCP but also enhances the carbon performance of neighboring regions.
H3:. Developing NI exhibits a spatial spillover effect on TFCP in the neighboring areas.
The theoretical framework is illustrated in Figure 1.
This study establishes the following benchmark regression model to assess the direct impact of NI on TFCP. The detailed calculation is as Equation 1.
Where i denotes city; t denotes year; TFCP denotes total factor carbon productivity; NI denotes the level of new infrastructure development; Controlit denotes a series of city-level control variables over time, including resourcing level (RAL), financial development level (FDL), level of investment in assets (IFA), economic development level (EDL), and degree of industrialization (IDD); λ denotes individual fixed effects; η denotes time fixed effect; and ε is the random perturbation term.
To further explore whether there are nonlinear effects and threshold conditions for the contribution of new infrastructure development to total factor carbon productivity in cities, this study established the threshold effect model on Model (1) as the Equation 2 (Hansen and Seo, 2002):
In the equation, EC denotes the threshold variable energy consumption, γ denotes the threshold, and I denotes an indicator function that assumes the value 0 or 1.
Furthermore, to explore the spatial spillover effect of carbon emission reduction of new infrastructure construction, Model (1) has been expanded into a spatial panel model by adding a spatial interaction element as the Equation 3 (Elhorst, 2012):
Here, W denotes the spatial weight matrix, ρ denotes spatial effect coefficients, and μ1 and μ2 denote the spatial lag terms’ coefficients for the primary explanatory and controlling variables, respectively.
Carbon productivity can link carbon emissions to economic development, and has emerged as a critical metric for gauging the efficiency of a country’s (region’s) low-carbon economic development. A single-indicator measurement approach emphasizes the direct correlation between carbon dioxide emissions and GDP. However, this method fails to capture the intrinsic relations between CO2 emissions and factors such as capital, labor, and energy, thereby hindering the comprehensive assessment of multiple inputs and outputs. The TFCP indicator, which integrates various relevant factors, considers offers account both economic efficiency gains and environmental carbon reductions (Li and Liao, 2022; Chen and Yao, 2024). It is more appropriate for evaluating the win-win performance of carbon reduction and efficiency gains.
Among the various methods for measuring TFCP, nonparametric data envelopment analysis (DEA) stands out for its ability to effectively address issues related to different scales and angular bias. DEA does not require the construction of production functions and has found widespread application in efficiency measurement studies (Zhou et al., 2017).
The present study employs a nonradial, nonangular Slacks-Based Measure (SBM) model to measure urban TFCP, drawing on the work of Tone and others (Tone, 2001). The indicator system comprises input and output components. The input component includes capital, labor, and energy. The fixed capital stock reflects the capital input and is processed using the perpetual inventory method (PIM) (Nehru et al., 1995). The formula of capital stock used for calculation is as follows:
Existing literature has mainly assessed NI development based on individual indicators such as the total volume of postal and telecommunication services, length of fiber-optic cable lines, Internet penetration rate, and the “Broadband China” strategy (Danish et al., 2018; Yang et al., 2022; Xiao et al., 2024). While these indicators provide insights into specific aspects of NI development, they may not offer a comprehensive measure of overall NI levels in a particular region.
Based on the definition of new infrastructure from the Commission on National Development and Reform of China, and according to the research ideas of Ndubuisi et al. (2021), Lan and Zhu (2023) and Chang et al. (2024). This research constructs an evaluation index system for China’s city-level new infrastructure from three dimensions: information, convergence, and innovation, to thoroughly mirror the reality of China’s urban new infrastructure development. Information infrastructure pertains to the communication network infrastructure reliant on new-generation information technology. Converged infrastructure combines information technology and traditional infrastructure to address deficiencies in the modern era. Innovation infrastructure empowers various sectors including science, education, and industry with digital innovation technology. By integrating the dimensions of information, convergence, and innovation, the level of NI development at the city level is determined using the entropy weight method. Table 1 reports the specific indicators.
The threshold variable is energy consumption (EC), measured as electricity consumption per capita for society, which is calculated as the ratio of total electricity consumption for the year to the number of people in the area (Khanna and Sharma, 2021).
The rest of the data are control variables for this paper. Drawing on the research methodology of Li et al., 2012, Rehman et al. (2019) and Fang et al. (2020), the main control variables are as follows: (1) Resource allocation level (RAL), represented by road space per capita; (2) financial development level (FDL), indicated by the year-end deposit and loan balances of the financial sector as a share of GDP; (3) level of asset investment (IFA), quantified as investment in fixed assets per capita; (4) economic advancement level (EDL), measured as regional GDP per capita; (5) degree of industrialization development (IDD), expressed as the share of output in the secondary sector to the share of employees in the secondary sector.
To account for the missing data of some cities and ensure the continuity and availability of sample data, this study includes 280 prefecture-level cities in China from 2011 to 2019. A balanced panel of data from 2,520 cities is then formed. Data are sourced from the China Urban Statistical Yearbook, China Energy Statistical Yearbook, Statistical Yearbook of Urban Construction in China, Annual Development Report of China’s Electric Power Industry, provincial and municipal statistical yearbooks and statistical bulletins, as well as the Juchao Information Network. We employed interpolation to manage data lacunae. Some variables are logarithmic to ensure scale uniformity. Descriptive statistics for each variable are presented in Table 2.
Based on the theoretical analyses and hypotheses, first, this study explores the performance of the fundamental role of new infrastructure construction in carbon emission reduction. Table 3 presents the results of the benchmark regression of Model (1), controlling for the individual and time-fixed effects. Notably, the contribution of NI to urban TFCP appears relatively robust, with all impact coefficients being significant at 1% confidence level. Specifically, each 1% increase in the level of NI corresponds to an average 0.48% increase in TFCP. Although the effect decreases after incorporating control variables, it remains significant, affirming that developing NI bolster urban TFCP. This suggests that NI possess environmentally friendly characteristics and can support low-carbon sustainable development in cities (Vaka et al., 2020). These results verify Hypothesis 1 of this study.
Meanwhile, ecnonmic development level (EDL) and degree of industrialization development (IDD) also contribute to TFCP. Financial development level (FDL) and investment in assets (IFA) are negatively correlated with TFCP and both are significant at 1% level. This result suggests that the level of economic and industrialization development is an important factor in urban carbon reduction and efficiency, while controlling the level of financial development and asset investment is crucial.
Furthermore, this study employs lagged orders of NI for regression to mitigate possible causal inversion and endogenous disturbances stemming from unobservable factors in the benchmark regression (Hu et al., 2023; Lan and Zhu, 2023). As depicted in columns (3) and (4) of Table 3, the effect of lagged NI on TFCP remains significant. The robustness of the results is indicated by the positive and negative attributes of the coefficient values and the significance of the estimation outcomes, confirming the lagged effect of NI’s influential role.
Lagged first and second orders of new infrastructure are employed as instrumental variables (IV) and estimated using two-stage least squares (2SLS) to further mitigate variable endogeneity (Li and Liu, 2022). The results in Column (5) of Table 3 indicate that the promotional effect of NI on TFCP persists under this approach.
This study recalculates explained variables (TFCP) by expanding the dimensions and reconducts regression tests by comprehensively considering the input and output performances of the production factors (Kong et al., 2024). In this context, two additional resource factors, water and land, are considered alongside the existing capital, labor, and energy input factors. In addition to the desired outputs of economic value, the wellbeing of life is considered, with the green space per capita in parks serving as a proxy for this aspect. The TFCP is recalculated using the super-efficient SBM model and labeled as TFCP1, and the parameters of the benchmark regression model are re-estimated. The results are displayed in column (1) of Table 4, which indicate that findings are stable and valid.
The informativeness method is employed as an alternative approach to evaluate the level of NI development, and further regression tests are conducted (Ndubuisi et al., 2021; Yang et al., 2021). The results are presented in Column (2) of Table 4. The positive effect of NI on TFCP remains significant. This finding reaffirms the beneficial role of NI in enhancing the city’s TFCP.
Acknowledging the distinctive characteristics of directly governed cities, which fall under the direct administration of the central government, and coastal cities, which often benefit from preferential national policies, it is evident that their infrastructure configuration, economic development level, and degree of information differ from those of other prefecture-level cities. To enhance the generalizability of the research results, the sample data from directly governed and coastal cities are excluded. Regression tests are conducted again (Chang et al., 2024; Li et al., 2024). The positive and negative attributes of the coefficient values in columns (3) and (4) of Table 4 demonstrate the robustness of the findings of this study.
This study empirically tests the threshold effect of regional energy consumption by using a panel threshold model to further explore whether NI affects TFCP nonlinearly. According to Model (2), a self-sampling threshold effect test (bootstrap) is initially conducted with 1,000 repeated samples to confirm the existence of a threshold. The results show that energy consumption has passed the single-threshold and double-threshold tests at 1% significance level. It indicates that the contribution of NI to TFCP exhibits heterogeneity under different levels of energy consumption. Graphs depicting changes in the likelihood ratio (LR) statistics of the threshold variables clearly illustrate the threshold estimates and their confidence intervals, as detailed in Table 5 and Figure 2.
The results presented in Table 6 indicate that NI contributes to urban TFCP, and energy consumption exhibits a double threshold effect on this relation, passing the 1% significance level test. Specifically, when the level of energy consumption is below 5.213, every 1% increase in the level of NI construction will lead to 10.643% increase in TFCP; when the level of energy consumption in the city exceeds the threshold, the impact coefficient of NI decreases to 3.888; and when the level of energy consumption exceeds the second threshold, the estimated coefficient of the impact index of NI decreases to 0.354. It indicates that the impact of NI on urban carbon performance is affected by the regional energy consumption threshold. The effect is obvious in cities with low energy consumption, while in cities with strong resource dependence, the carbon reduction effectiveness of NI is difficult to be realized.
Many cities characterized by high levels of energy consumption are in a rough development mode, relying heavily on industries with high energy consumption and emissions for economic growth. This situation leads to significant contradictions concerning resources, environment, climate, and security. The convergence of new infrastructures and resource-dependent industries plays a limited role in such cities. Even if the manufacturing efficiency of traditional resource-based industries improves through digital technology, it often results in further driving regional energy consumption, with a minimal pull effect on the city’s carbon performance or even exacerbating low-carbon hazards (Hu et al., 2023). However, cities with a low level of energy consumption usually have a larger scale of national innovation investment and a higher level of digital technology application and innovation. Therefore, the investment in digital infrastructure construction in low-energy cities is more massive, and providing richer application scenarios for them to promote the transformation and upgrading of energy structure and the green and low-carbon development of industries (Zhang et al., 2023a).
In the traditional theory of economic growth, abundant natural resources are the basis for the start of industrialization and the guarantee of sustainable economic development. Resource dependent cities and Resource undependent cities differ in terms of resource endowment and energy use efficiency. Thus, the sample has been divided into 109 resource-based cities and 171 nonresource-based cities to verify the energy consumption threshold effect for both types of cities. The findings demonstrate that in the sample of resource-based cities, the threshold effect of regional energy consumption fails the significance test. The industrial structure of resource cities is mostly high energy consumption and high pollution. Their reliance on traditional resources is characterized by typical “path dependence” and “lock-in effect”. The persistence of their high-energy consumption pattern hinders the green transformation of traditional industries and the development of new energy industries, thus weakening the carbon-reducing effect of new infrastructures (Du et al., 2023).
A sample test of non-resource cities shows that new infrastructure development has a positive impact on the increase of total factor carbon productivity in cities, and that energy consumption has a double-threshold effect on this positive impact. With the rapid progress of industrialization, the demand for coal, electricity and other energy consumption of the whole society remains high in the early stage of urban development. At this stage, the digitalization level of cities is relatively backward, and the new infrastructure construction fails to follow up, which makes it difficult to release its effect on carbon performance. Industrial change promotes the diversification of the development mode of non-resource cities, and the level of energy consumption of the whole society is gradually reduced. At this stage, as the level of digital technology application in cities gradually increases, it creates more favorable conditions for the integration and penetration of new infrastructures (Pan et al., 2022; Peng et al., 2024). This has also led to digital infrastructure and digital platforms even better in cities, which makes it easier to upgrade industrial structure and green technological innovation, and strive to improve the efficiency of energy utilization, thus curbing carbon emissions (Liao and Liu, 2024).
In addition, this paper also replaces the explained and explanatory variables during the threshold test and the robustness test shows that the research conclusions are still valid. In addition, this paper also replaces the explained and explanatory variables in threshold test during the threshold test. The robustness test shows that the research conclusions are still valid.
To examine the existence of a spatial influence and linkage between NI and urban TFCP changes, first, we assess the spatial correlation of the core variables by calculating the global Moran’s Index (Moran’ I). The Moran’ I index is calculated using the Formula 4 (Tiefelsdorf and Boots, 1997):
Where n denotes the number of sample cities; xi, xj are TFCPs of cities i and j;
The construction of spatial weight matrix is the basis for spatial measurement correlation analysis. In order to reflect the degree of spatial correlation between cities and the spatial effect of NI on the impact of TFCP (Elhorst, 2012). Considering that the research content and explanatory variables in this paper are related to environmental pollution and energy consumption, and therefore related to spatial distance. So this paper chooses to construct the weight matrix based on the spatial neighboring of cities (W1) and geographic distance (W2). The detailed calculation is as Equation 5 and Equation 6.
Here, dij denotes the surface distance between cities calculated by longitudinal and latitudinal position, with greater weighting given to closer geographic proximity.
The results are presented in Table 7. The values of global Moran’s I index for both NI and TFCP are positive, indicating significant spatial autocorrelation between the two variables. To further explore the spatial effects, this study selects the spatial Durbin model (SDM) with double fixed effects based on the LM, LR, Wald and Hausmann tests (Elhorst, 2014).
Table 8 displays the spatial autocorrelation regression coefficients ρ of the two models, which are 0.141 and 0.541, respectively. Both coefficients pass the 1% significance level test, indicating that the city’s TFCP is affected by the NI levels in geospatially proximate cities. This finding confirms that NI exhibits a radiative effect on regional carbon emission performance, consistent with the findings of selected scholars (Zhang et al., 2023b). The positive effect persists in the regression coefficients of the spatial interaction terms. The coefficient of the interaction term with geographical distance is even more significant, indicating that NI enhances the TFCP of neighboring cities, and this cross-regional spillover effect is closely related to geographical distance.
NI enables the continuous adaptation of a city’s internal space through the aggregation of multiple functions and the transmission of massive amounts of information, effectively connecting the city’s external space. While some studies have suggested a spatial-geographical decay law of knowledge spillover (Fischer et al., 2009), the high permeability of NI determines its role in blurring the traditional geographic boundaries and facilitating knowledge sharing. NI breaks through the limitations of geospatial distance; promote flow of knowledge, information, data, and other innovation factors across regions; and reduce information asymmetry caused by geographic distance (Raghupathi et al., 2014). This strengthens the correlation between a city’s carbon emission performance and the level of NI development in neighboring cities. In that case the NI of neighboring cities significantly contributes to local low carbon development through elemental correlation.
Because of a significant and nonzero ρvalue, the direct use of regression coefficients cannot adequately explain the effects of the two variables. Therefore, the total marginal effect should be divided into direct and indirect effects to more comprehensively analyze the effects of each factor on urban TFCP (Lesage and Pace, 2009).
Regarding the total effect (see Table 9), NI facilitates the improvement of the TFCP index in the region and neighboring cities under two spatial weight matrices of neighborhood and geography. The deployment and utilization of new infrastructure in the region radiate the integration and development of digital industries in neighboring regions. The application of information technology enhances productivity by reducing energy loss, while reducing emissions of pollutants such as CO2, thereby promoting low-carbon development in the local area and neighboring regions (Khan et al., 2018).
From the results reflecting by the direct and indirect effects, the direct and spillover impact coefficients of NI on urban TFCP are significantly positive at 1% confidence level. This indicates that NI significantly positively affects carbon reduction and improve efficiency in the region. Its diffusion effect surpasses the echo effect, enhancing the green, low-carbon, and high-quality development of neighboring regions. Notably, the spatial spillover effect under the geographic weighting matrix is substantially more pronounced than the direct effect, with a difference in the coefficient of influence of NI being 3.408. This suggests that the geographic distance significantly amplifies the promotional effect of NI on TFCP of neighboring regions. Moreover, NI promotes high-efficiency concentration and diffusion of information technology factors between regions through spatial clustering and production networking. It also drives the greening, digitalization, and energy-saving upgrading of production processes and techniques. The accelerated establishment of a wide-coverage intelligent transport and fast-track system, along with the rapid flow of production factors across regions and the continuous reduction of logistics and transaction costs, contribute to the formation of an organic community among cities (Hepburn et al., 2021).
Digital technologies play a crucial role in driving towards a zero-carbon future. This paper explores whether new infrastructures as vehicles for digital technologies can play a role in carbon reduction and efficiency enhancement in cities. Drawing on panel data from 280 Chinese cities spanning 2011–2019, this study investigates the effect of NI on urban TFCP, offering insights to complement existing literature and create policy rationale toward low-carbon development through digitization. The main findings of the study are as follows: (1) The fundamental role analysis shows that NI positively influences urban TFCP, with robustness of the result affirmed through various testing methodologies. (2) As the level of energy consumption decreases, the positive contribution of NI to TFCP gradually increases. This effect is obvious in non-resource cities, while the carbon reduction effect of new infrastructure is difficult to realize in cities with strong resource dependence. (3) The spatial spillover effect analysis indicates that NI can accelerate local carbon performance and exerts a positive spillover effect on neighboring cities through emission reduction and pressure reduction, thereby promoting large-scale urban integration.
Based on the empirical tests provided above, we present the following policy recommendations:
In view of the significant impact of NI on the improvement of urban carbon performance, On one hand, it is recommended to advance the layout of NI appropriately, increase the penetration rate of information infrastructure such as 5G base stations, data centers, and the Internet of Things, so as to enhance the new energy level of the digital pedestal. The “NI” should be emphasized the effectiveness of investment, and localities make a prediction of investment prospects. It is necessary to design the profit model rationally and arrange the financing method. It should be made feasible for commercial investment, enhance development momentum and form high-quality assets that can be reused in the long term. Meanwhile, it is necessary to increase financial support to support the relevant fields of universities, research institutes and enterprises to carry out common technology research jointly and solve technical problems of industries involved in NI. On the other hand, strengthening the innovation infrastructure that supports scientific research and promoting the application of digital technology in urban low-carbon management. Carbon emissions are monitored and analyzed through big data, and the effects of environmental policy implementation are assessed. This improves the efficiency and responsiveness of urban governance and creates conditions for enhancing the effectiveness of digital carbon management and digital carbon reduction by the government.
Furthermore, considering that the carbon emission reduction effect of NI is affected by regional energy consumption thresholds, cities should strengthen energy constraints and other key supporting factors and seize energy consumption’s “threshold point” in NI development. On one hand, accelerate the deep integration of information technology and resource-based industries, and lead the transformation and upgrading of traditional energy industries with digital technology. Cities introduce policies and norms to promote the synergistic development of digital infrastructure and green energy actively. promote the rational distribution of green energy, and improve the energy utilization efficiency of the digital industry. On the other hand, the Government has scientifically designed and deployed an accurate energy control mechanism, built a modern smart grid and promoted the digitalization of energy control. Through real-time monitoring and analysis of energy use data, the visualization of energy use is realized, ultimately improving energy use efficiency and reducing energy consumption.
Finally, NI has spatial spillover effects on neighboring cities in terms of carbon reduction and efficiency, and contributes to the construction of ecological civilization in urban agglomerations by upgrading innovation capacity and accelerating urban-rural integration. However, the prevalence of information silos and the lack of effective integrated planning have led to different cities promoting NI projects independently without unified coordination, resulting in scattered resources and duplication of construction. At the starting line of the construction of smart city clusters, a top-level design with a far-reaching orientation is crucial. The Government should strengthen interregional cooperation in the development of the digital economy and coordinate the construction layout of interregional data centers, arithmetic algorithms, industrial Internet and other infrastructures to maximize their effectiveness. The carbon-reducing effects of new infrastructure can cross traditional urban governance boundaries by industrial integration, corporate cooperation and factor sharing. This creates a green and intensive regional integration pattern and improves the overall green economy efficiency among cities.
Although this study uses 280 cities in China as the sample, the findings are equally helpful for other emerging countries. Like China, most developing economies are still in the early stages of NI. Expanding effective investment and advancing the layout of NI will significantly impact the economic growth of these countries.
This study also has some limitations. On the one hand, we focus on the fundamental role, nonlinear effect and spatial spillover effect of NI impact on TFCP. This research line of thought weakens the differences manifested under different city characteristics. Future research can further explore the impact, such as different city sizes, factor endowments, and geographic locations. On the other hand, in the complex relationship of NI affecting urban TFCP, whether there are other factors that play a moderating role besides being constrained by the threshold characteristics of energy consumption. Which can be further explored at a later stage to explore the role of technological accumulation, human capital, and so on, in enhancing the empowering effect of digital investment.
This data can be found here: Data are sourced from the China Urban Statistical Yearbook, China Energy Statistical Yearbook, provincial and municipal statistical yearbooks and statistical bulletins. Available at: “https://data.cnki.net/” and “https://www.stats.gov.cn/sj/ndsj/”.
YL: Conceptualization, Methodology, Writing–original draft. YW: Data curation, Software, Writing–original draft. BY: Writing–review and editing. HZ: Data curation, Writing–original draft.
The author(s) declare that financial support was received for the research, authorship, and/or publication of this article. This research was funded by Ministry of Education, Humanities and social science projects of China (No. 22YJC790086), as well as High-level Talent Cultivation Project of Heilongjiang Provincial Undergraduate Colleges and Universities with Central Support for the Reform and Development Funds of Local Colleges and Universities (No. 14011202101).
The authors declare that the research was conducted in the absence of any commercial or financial relationships that could be construed as a potential conflict of interest.
All claims expressed in this article are solely those of the authors and do not necessarily represent those of their affiliated organizations, or those of the publisher, the editors and the reviewers. Any product that may be evaluated in this article, or claim that may be made by its manufacturer, is not guaranteed or endorsed by the publisher.
Acemoglu, D., Aghion, P., Bursztyn, L., and Hemous, D. (2012). The environment and directed technical change. Am. Econ. Rev. 102, 131–166. doi:10.1257/aer.102.1.131
Ai, K., and Yan, X. (2024). Can green infrastructure investment reduce urban carbon emissions:empirical evidence from China. Land 13 (2), 226. doi:10.3390/land13020226
Arbolino, R., Carlucci, F., Cirà, A., Yigitcanlar, T., and Ioppolo, G. (2018). Mitigating regional disparities through microfinancing: an analysis of microcredit as a sustainability tool for territorial development in Italy. Land Use Policy 70, 281–288. doi:10.1016/j.landusepol.2017.10.042
Bronzini, R., and Piselli, P. (2009). Determinants of long-run regional productivity with geographical spillovers: the role of R&D, human capital and public infrastructure. Reg. Sci. Urban Econ. 39, 187–199. doi:10.1016/j.regsciurbeco.2008.07.002
Chang, X., Li, J., and Zheng, Q. (2024). Does new-type infrastructure improve total factor carbon productivity? Experimental evidence from China. J. Clean. Prod. 460, 142620. doi:10.1016/j.jclepro.2024.142620
Chen, W., and Yao, L. (2024). The impact of digital economy on carbon total factor productivity: a spatial analysis of major urban agglomerations in China. J. Environ. Manag. 351, 119765. doi:10.1016/j.jenvman.2023.119765
Czernich, N., Falck, O., Kretschmer, T., and Woessmann, L. (2011). Broadband infrastructure and economic growth. Econ. J. 121, 505–532. doi:10.1111/j.1468-0297.2011.02420.x
Danish, N. K., Baloch, M. A., Shah Saud, T. F., Saud, S., and Fatima, T. (2018). The effect of ICT on CO2 emissions in emerging economies: does the level of income matters? Environ. Sci. Pollut. Res. 25, 22850–22860. doi:10.1007/s11356-018-2379-2
Dedrick, J., Kraemer, K. L., and Shih, E. (2013). Information technology and productivity in developed and developing countries. J. Manage. Inf. Syst. 30, 97–122. doi:10.2753/MIS0742-1222300103
Du, K., and Li, J. (2019). Towards a green world: how do green technology innovations affect total-factor carbon productivity. Energy Policy 131, 240–250. doi:10.1016/j.enpol.2019.04.033
Du, X., Zhang, H., and Han, Y. (2022). How does new infrastructure investment affect economic growth quality? Empirical evidence from China. Sustainability 14, 3511. doi:10.3390/su14063511
Du, Y., Zhou, J., Bai, J., and Cao, Y. (2023). Breaking the resource curse: the perspective of improving carbon emission efficiency based on digital infrastructure construction. Resour. Policy 85, 103842. doi:10.1016/j.resourpol.2023.103842
Elhorst, J. P. (2012). Dynamic spatial panels: models, methods, and inferences. J. Geogr. Syst. 14 (1), 95–119. doi:10.1007/978-3-642-40340-8_4
Elhorst, J. P. (2014). Matlab software for spatial panels. Int. Reg. Sci. Rev. 37, 389–405. doi:10.1177/0160017612452429
Fang, Z., Gao, X., and Sun, C. (2020). Do financial development, urbanization and trade affect environmental quality? Evidence from China. J. Clean. Prod. 259, 120892. doi:10.1016/j.jclepro.2020.120892
Fang, Z., Razzaq, A., Mohsin, M., and Irfan, M. (2022). Spatial spillovers and threshold effects of internet development and entrepreneurship on green innovation efficiency in China. Technol. Soc. 68, 101844. doi:10.1016/j.techsoc.2021.101844
Fernández-Portillo, A., Almodóvar-González, M., and Hernández-Mogollón, R. (2020). Impact of ICT development on economic growth. A study of OECD European Union countries. Technol. Soc. 63, 101420. doi:10.1016/j.techsoc.2020.101420
Fischer, M. M., Scherngell, T., and Reismann, M. (2009). Knowledge spillovers and total factor productivity: evidence using a spatial panel data model. Geogr. Anal. 41, 204–220. doi:10.1111/j.1538-4632.2009.00752.x
Fu, L., Zhang, L., and Zhang, Z. (2023). The impact of information infrastructure construction on carbon emissions. Sustainability 15, 7693. doi:10.3390/su15097693
Guo, B., Feng, Y., and Wang, X. (2024b). The effect of environmental information disclosure on carbon emission. Pol. J. Environ. Stud. 33, 1681–1691. doi:10.15244/pjoes/173106
Guo, B., Hu, P., and Lin, J. (2024a). The effect of digital infrastructure development on enterprise green transformation. Int. Rev. Financial Analysis 92, 103085. doi:10.1016/j.irfa.2024.103085
Hansen, B. E., and Seo, B. (2002). Testing for two-regime threshold cointegration in vector error-correction models. J. Econ. 110, 293–318. doi:10.1016/S0304-4076(02)00097-0
Hao, Y., and Liu, Y. (2016). The influential factors of urban PM2.5concentrations in China: a spatial econometric analysis. J. Clean. Prod. 112, 1443–1453. doi:10.1016/j.jclepro.2015.05.005
Hepburn, C., Qi, Y., Stern, N., Ward, B., Xie, C., and Zenghelis, D. (2021). Towards carbon neutrality and China's 14th Five-Year Plan: clean energy transition, sustainable urban development, and investment priorities. Environ. Sci. Ecotechnology 8, 100130. doi:10.1016/j.ese.2021.100130
Hu, J., Zhang, H., and Irfan, M. (2023). How does digital infrastructure construction affect low-carbon development? A multidimensional interpretation of evidence from China. J. Clean. Prod. 396, 136467. doi:10.1016/j.jclepro.2023.136467
ICON (2023). Annual progress report on global carbon neutrality 2023. Beijing: Institute for Carbon Neutrality (Accessed September 23, 2023).
Khan, Z., Linares, P., Rutten, M., Parkinson, S., Johnson, N., and García-González, J. (2018). Spatial and temporal synchronization of water and energy systems: towards a single integrated optimization model for long-term resource planning. Appl. Energy 210, 499–517. doi:10.1016/j.apenergy.2017.05.003
Khanna, R., and Sharma, C. (2021). Does infrastructure stimulate total factor productivity? A dynamic heterogeneous panel analysis for Indian manufacturing industries. Quart. Rev. Econ. Finance 79, 59–73. doi:10.1016/j.qref.2020.08.003
Kong, L., Li, Z., Liu, B., and Xu, K. (2024). The impact of environmental protection tax reform on low-carbon total factor productivity: evidence from China’s fee-to-tax reform. Energy 290, 130216. doi:10.1016/j.energy.2023.130216
Kou, J., and Xu, X. (2022). Does internet infrastructure improve or reduce carbon emission performance?A dual perspective based on local government intervention and market segmentation. J. Clean. Prod. 379, 134789. doi:10.1016/j.jclepro.2022.134789
Koutroumpis, P., Leiponen, A., and Thomas, L. D. W. (2020). Small is big in ICT: the impact of R&D on productivity. Telecommun. Policy 44, 101833. doi:10.1016/j.telpol.2019.101833
Lan, M., and Zhu, Y. (2023). Digital infrastructure construction, carbon total factor productivity, and carbon rebound effect. Environ. Sci. Pollut. Res. 30, 88968–88985. doi:10.1007/s11356-023-28738-9
LeSage, J. P., and Pace, R. K. (2009). Introduction to spatial econometrics. Chapman and Hall/CRC. doi:10.1201/9781420064254
Li, G., and Liao, F. (2022). Input digitalization and green total factor productivity under the constraint of carbon emissions. J. Clean. Prod. 377, 134403. doi:10.1016/j.jclepro.2022.134403
Li, H., Wang, L., Shen, L., and Chen, F. (2012). Study of the potential of low carbon energy development and its contribution to realize the reduction target of carbon intensity in China. Energy Policy 41, 393–401. doi:10.1016/j.enpol.2011.10.061
Li, J., and Liu, A. (2022). Impact of urbanization on total factor carbon productivity in Central Asia. Sustainability 14, 15379. doi:10.3390/su142215379
Li, X., Yang, G., Shao, T., Yang, D., and Liu, Z. (2024). Does digital infrastructure promote individual entrepreneurship? Evidence from a quasi-natural experiment on the “Broadband China” strategy. Tech Fore & Soci Chan 206, 123555. doi:10.1016/j.techfore.2024.123555
Liao, K., and Liu, J. (2024). Digital infrastructure empowerment and urban carbon emissions: evidence from China. Telec Policy 48, 102764. doi:10.1016/j.telpol.2024.102764
Lin, B., and Jia, H. (2022). Does the development of China's high-speed rail improve the total-factor carbon productivity of cities? Transp. Res. Part D. Transp. Environ. 105, 103230. doi:10.1016/j.trd.2022.103230
Madlener, R., Sheykhha, S., and Briglauer, W. (2022). The electricity-and CO2-saving potentials offered by regulation of European video-streaming services. Energy Policy 161, 112716. doi:10.1016/j.enpol.2021.112716
Matthews, H. D., Gillett, N. P., Stott, P. A., and Zickfeld, K. (2009). The proportionality of global warming to cumulative carbon emissions. Nature 459, 829–832. doi:10.1038/nature08047
Mi, Z., Zhang, Y., Guan, D., Shan, Y., Liu, Z., Cong, R., et al. (2016). Consumption-based emission accounting for Chinese cities. Appl. Energy 184, 1073–1081. doi:10.1016/j.apenergy.2016.06.094
MIIT (2024). Launch of China's industrial and informatisation development in 2023. Beijing: Ministry of Industry and Information Technology.
Mitra, A., Sharma, C., and Véganzonès-Varoudakis, M. A. (2016). Infrastructure, information & communication technology and firms’ productive performance of the Indian manufacturing. J. Policy Model. 38, 353–371. doi:10.1016/j.jpolmod.2016.02.013
Mouratidis, K., Peters, S., and van Wee, B. (2021). Transportation technologies, sharing economy, and teleactivities: implications for built environment and travel. Transp. Res. Part D. Transp. Environ. 92, 102716. doi:10.1016/j.trd.2021.102716
Ndubuisi, G., Otioma, C., and Tetteh, G. K. (2021). Digital infrastructure and employment in services: evidence from Sub-Saharan African countries. Tele. Pol. 45 (8), 102153. doi:10.1016/j.telpol.2021.102153
Nehru, V., Swanson, E., and Dubey, A. (1995). A new database on human capital stock in developing and industrial countries: sources, methodology, and results. J. Dev. Econ. 46, 379–401. doi:10.1016/0304-3878(94)00054-G
Pan, W., Xie, T., Wang, Z., and Ma, L. (2022). Digital economy: an innovation driver for total factor productivity. J. Bus. Res. 139, 303–311. doi:10.1016/j.jbusres.2021.09.061
Peng, H., Ling, K., and Zhang, Y. (2024). The carbon emission reduction effect of digital infrastructure development: evidence from the broadband China policy. J. Clean. Prod. 434, 140060. doi:10.1016/j.jclepro.2023.140060
Raghupathi, W., Wu, S. J., and Raghupathi, V. (2014). The role of information and communication technologies in global sustainability: a review. J. Manage. Glob. Sustain. 2, 123–145. doi:10.13185/JM2014.02106
Rehman, A., Rauf, A., Ahmad, M., Chandio, A. A., and Deyuan, Z. (2019). The effect of carbon dioxide emission and the consumption of electrical energy, fossil fuel energy, and renewable energy, on economic performance: evidence from Pakistan. Environ. Sci. Pollut. Res. 26 (21), 21760–21773. doi:10.1007/s11356-019-05550-y
Shan, Y., Guan, D., Zheng, H., Ou, J., Li, Y., Meng, J., et al. (2018). China CO2 emission accounts: 1997-2015. Sci. Data 5, 170201. doi:10.1038/sdata.2017.201
Solow, R. M. (1957). Technical change and the aggregate production function. Rev. Econ. Stat. 39, 312–320. doi:10.2307/1926047
Tang, K., and Yang, G. (2023). Does digital infrastructure cut carbon emissions in Chinese cities? Sustain. Prod. Consum. 35, 431–443. doi:10.1016/j.spc.2022.11.022
Tiefelsdorf, M., and Boots, B. (1997). A note on the extremities of local Moran's I(i)s and their impact on global Moran's I. Geogr. Anal. 29 (3), 248–257. doi:10.1111/j.1538-4632.1997.tb00960.x
Tone, K. (2001). A slacks-based measure of efficiency in data envelopment analysis. Eur. J. Oper. Res. 130, 498–509. doi:10.1016/S0377-2217(99)00407-5
Vaka, M., Walvekar, R., Rasheed, A. K., and Khalid, M. (2020). A review on Malaysia’s solar energy pathway towards carbon-neutral Malaysia beyond Covid’19 pandemic. J. Clean. Prod. 273, 122834. doi:10.1016/j.jclepro.2020.122834
Wang, K., Sun, T., Xu, R., Miao, Z., and Cheng, Y. (2022). How does internet development promote urban green innovation efficiency? Evidence from China. Technol. Forecast. Soc. Change 184, 122017. doi:10.1016/j.techfore.2022.122017
Wang, M., Wang, Y., Yang, Z., and Guo, B. (2024). Does energy-consuming rights trading policy achieve urban pollution and carbon reduction? A quasi-natural experiment from China. Front. Environ. Sci. 12, 1430031. doi:10.3389/fenvs.2024.1430031
Wu, H., Hao, Y., Ren, S., Yang, X., and Xie, G. (2021). Does internet development improve green total factor energy efficiency? Evidence from China. Energy Policy 153, 112247. doi:10.1016/j.enpol.2021.112247
Wu, W., Ji, Z., and Liang, H. (2023). The impact of new infrastructure construction on optimization and upgrading of industrial structure. Buildings 13, 2580. doi:10.3390/buildings13102580
Xiao, X., Liu, C., and Li, S. (2024). How the digital infrastructure construction affects urban carbon emissions—a quasi-natural experiment from the “Broadband China” policy. Sci. Total Environ. 912, 169284. doi:10.1016/j.scitotenv.2023.169284
Yan, X., Bai, J., Zhang, Y., and Hu, S. (2022). Can the ecological environment reverse feed renewable energy technology innovation?Heterogeneity test from the Yangtze River Economic Belt. Renew. Energy 195, 1381–1392. doi:10.1016/j.renene.2022.06.124
Yang, Y., Chen, W., and Gu, R. (2021). How does digital infrastructure affect industrial eco-efficiency? Considering the threshold effect of regional collaborative innovation. J. Clean. Prod. 427, 139248. doi:10.1016/j.jclepro.2023.139248
Yang, Z., Gao, W., Han, Q., Qi, L., Cui, Y., and Chen, Y. (2022). Digitalization and carbon emissions: how does digital city construction affect China's carbon emission reduction? Sustain. Cities Soc. 87, 104201. doi:10.1016/j.scs.2022.104201
Zhang, J., Wu, G., and Zhang, J. (2004). Estimation of provincial physical capital stocks in China: 1952–2000. Econ. Res. 10, 35–44.
Zhang, W., Fan, H., and Zhao, Q. (2023a). Seeing green: how does digital infrastructure affect carbon emission intensity? Energy Econ. 127, 107085. doi:10.1016/j.eneco.2023.107085
Zhang, W., Zeng, M., Zhang, Y., and Su, C. W. (2023b). Reducing carbon emissions: can high-speed railway contribute? J. Clean. Prod. 413, 137524. doi:10.1016/j.jclepro.2023.137524
Zhong, M. R., Cao, M. Y., and Zou, H. (2022). The carbon reduction effect of ICT: a perspective of factor substitution. Technol. Forecast. Soc. Change 181, 121754. doi:10.1016/j.techfore.2022.121754
Zhou, L., and Tang, L. (2021). Environmental regulation and the growth of the total-factor carbon productivity of China's industries: evidence from the implementation of action plan of air pollution prevention and control. J. Environ. Manage. 296, 113078. doi:10.1016/j.jenvman.2021.113078
Keywords: total factor carbon productivity, new infrastructure, carbon reduction, efficiency gains, energy consumption
Citation: Liu Y, Wang Y, Yan B and Zhang H (2024) The impact of new infrastructure development on urban total factor carbon productivity-empirical evidence based on Chinese cities. Front. Environ. Sci. 12:1432534. doi: 10.3389/fenvs.2024.1432534
Received: 14 May 2024; Accepted: 19 August 2024;
Published: 30 August 2024.
Edited by:
Ji Zheng, The University of Hong Kong, Hong Kong SAR, ChinaReviewed by:
Bingnan Guo, Jiangsu University of Science and Technology, ChinaCopyright © 2024 Liu, Wang, Yan and Zhang. This is an open-access article distributed under the terms of the Creative Commons Attribution License (CC BY). The use, distribution or reproduction in other forums is permitted, provided the original author(s) and the copyright owner(s) are credited and that the original publication in this journal is cited, in accordance with accepted academic practice. No use, distribution or reproduction is permitted which does not comply with these terms.
*Correspondence: Yang Liu, eWxpdUBuZXB1LmVkdS5jbg==
Disclaimer: All claims expressed in this article are solely those of the authors and do not necessarily represent those of their affiliated organizations, or those of the publisher, the editors and the reviewers. Any product that may be evaluated in this article or claim that may be made by its manufacturer is not guaranteed or endorsed by the publisher.
Research integrity at Frontiers
Learn more about the work of our research integrity team to safeguard the quality of each article we publish.