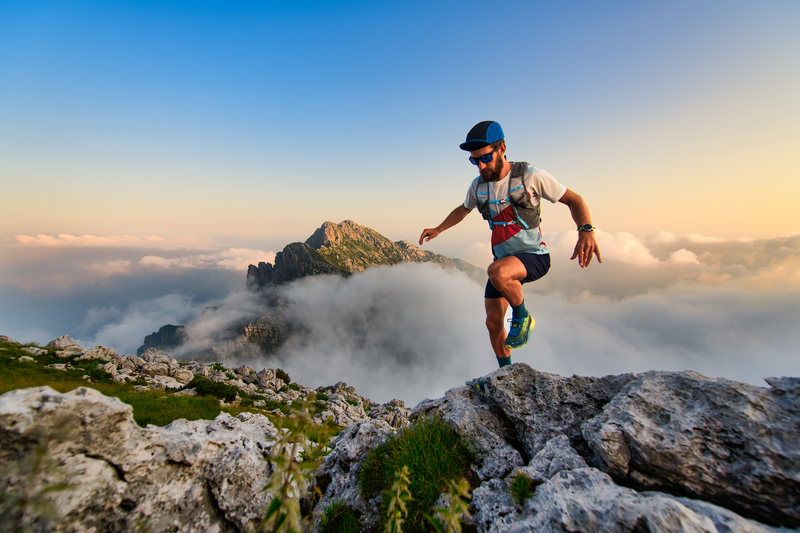
94% of researchers rate our articles as excellent or good
Learn more about the work of our research integrity team to safeguard the quality of each article we publish.
Find out more
REVIEW article
Front. Environ. Sci. , 11 September 2024
Sec. Environmental Informatics and Remote Sensing
Volume 12 - 2024 | https://doi.org/10.3389/fenvs.2024.1430818
Grassland ecosystems play a critical role in global carbon cycling and environmental health. Understanding the intricate link between grassland vegetation traits and underlying soil properties is crucial for effective ecosystem monitoring and management. This review paper examines advancements in utilizing Radiative Transfer Models (RTMs) and hyperspectral remote sensing to bridge this knowledge gap. We explore the potential of vegetation spectra as an integrated measure of soil characteristics, acknowledging the value of other remote sensing sources. Our focus is on studies leveraging hyperspectral data from proximal and airborne sensors, while discussing the impact of spatial scale on trait retrieval accuracy. Finally, we explore how advancements in global satellite remote sensing contribute to vegetation trait detection. This review concludes by identifying current challenges, outlining future research directions, and highlighting opportunities for improved understanding of the vegetation-soil property interaction.
Vegetation biophysical and biochemical properties (traits) such as chlorophyll, leaf size and carotenoids etc., are key characteristics of the vegetation that determines how they thrive in an environment. Understanding plant traits helps infer how environmental changes impact them and their underlying soil. For instance, plant presence alters soil structure, while soil properties (moisture, composition, density) affect plant growth. This link between plant traits and soil properties is crucial for understanding ecosystem function (Miedema Brown and Anand, 2022). Reliable measurements or estimates of vegetation traits are crucial for grassland monitoring. Remote sensing offers a promising tool for large-scale grassland monitoring, eliminating the need for extensive field surveys (Wang Z. et al., 2022). Advancements in proximal sensing technologies, such as handheld or vehicle-mounted multispectral and hyperspectral sensors (e.g., Analytical Spectral Devices, unmanned aerial vehicle (UAV) UAV-based hyperspectral devices, etc.), provide insights into leaf and canopy traits (pigment content, water use, etc.), crucial for grassland characterization (Aneece and Thenkabail, 2022; He et al., 2020). These sensor datasets can sample at a scale that is relevant to leaf properties and have been validated using experimental methods that utilize in situ data at comparable scales. Understanding these within-field trait characteristics is key to understanding how grasslands respond to management practices in remote areas. Over the past decade, numerous journal articles have explored using remote sensing data with statistical models to estimate vegetation traits (foliar characteristics, leaf structure) and soil properties (moisture) (Datta et al., 2022; Gholizadeh et al., 2022). However, most existing research and review studies have primarily focused on approaches for estimating and validating vegetation traits (Wang Z. et al., 2022; Dorigo et al., 2007; Berger et al., 2018a; Ustin et al., 2009). However, the extent to which these methods have advanced our understanding of seasonal relationships between vegetation traits and soil properties remains limited.
Grassland variations, shaped by management, soil properties, and plant diversity, influence how plants interact with light (Ustin and Jacquemoud, 2020). Plant functional traits (e.g., biochemistry, structure) drive these interactions, enabling adaptation to the environment. These traits affect light absorption and reflection, offering insights into plant-environment relationships through remote sensing (Ustin and Jacquemoud, 2020; Cavender-Bares et al., 2022). While remote sensing holds promise for assessing soil properties in grasslands, significant challenges remain. Multispectral and hyperspectral data have shown potential in differentiating soil and vegetation types (Adam et al., 2010; Castaldi et al., 2015; Lu B. et al., 2019), but accurately estimating soil properties is difficult due to spectral overlap, especially in areas with dense vegetation cover (Asner and Heidebrecht, 2002). Traditional masking techniques and linear unmixing methods often fall short in complex grassland environments. Although nonlinear unmixing methods offer some improvement, they are limited by restrictive assumptions (Yu et al., 2019; Khan et al., 2022). To accurately assess soil properties in grasslands using remote sensing, ideal conditions include minimal vegetation, shallow soil depth, soil types, and little plant litter (Ge et al., 2011; He et al., 2020). However, even under ideal conditions, challenges such as spectral similarity between green and non-photosynthetic vegetation, plant diseases, and ecological factors can obscure the soil-vegetation link, hindering accurate soil property retrieval. In managed grasslands, soils are often subject to practices like mowing which affect soil moisture content and compact its particle size, greatly influencing soil spectra. These complexities make characterizing soil properties in such areas difficult.
In plant remote sensing, spectra are used to estimate various plant traits by capturing the reflectance of light by the leaves or canopy (Zhang et al., 2021). Advancements in spectroscopic sensors - from laboratory to UAV-based systems - enable measurement of reflectance within the visible, near-infrared, and shortwave infrared (VIS-NIR-SWIR) spectrum (350–2,500 nm). This spectral range, covering over 90% of solar radiation reaching Earth’s surface, reveals plant-light interactions and adaptations. Established methods for retrieving vegetation traits from this spectral data fall into two categories: empirical-statistical and physical modeling (Jacquemoud et al., 2009; Ustin et al., 2009; Berger et al., 2018a). Empirical-statistical methods like vegetation indices link observed traits directly to reflectance data. However, they can be sensor-specific and may not be transferable across different conditions (Darvishzadeh et al., 2011; Verrelst et al., 2015a). Physical modeling methods, based on radiative transfer models (RTMs), directly link plant traits to spectroscopic data, offering transferability and detailed simulations of plant-light interactions (Verrelst et al., 2015a; Berger et al., 2018a). RTMs consider biochemical, structural, and geometric factors to simulate leaf or canopy reflectance. These reflectance databases generated by RTMs can then be inverted using appropriate methods to retrieve the related leaf or canopy characteristics (Gastellu-Etchegorry, 1996; Kimes et al., 2000), providing a powerful tool for studying plant functioning and responses to environmental factors. Both RTM-based methods and empirical-statistical methods have been successfully used in a range of applications, including the modeling and prediction of plant traits, species identification, and phenotype analysis (Sehgal et al., 2016; Berger et al., 2020; Schiefer et al., 2021; Kothari and Schweiger, 2022).
RTMs simulate canopy spectra by mathematically representing plant-light interactions. Their complexity varies, from one-dimensional (treating light as direct/diffuse streams) to three-dimensional models considering multiple scattering (light interaction within the canopy). The selection of an RTM is dictated by factors such as the application, data availability, and model limitations. Their accuracy generally relies on the input parameters accuracy and the modeled canopy structure. Detailed overview of some popular one- and three-dimensional models and their application to different environment can be found in these studies (Bailey et al., 2020; Yan et al., 2021). In recent years, there has been a growing body of research aimed at improving the accuracy of RTMs for various vegetation types. Among these models, PROSAIL (PROSPECT (Jacquemoud and Baret, 1990)+ SAIL (Jacquemoud et al., 2009)) has emerged as a widely used model for vegetation trait studies. PROSAIL has been used alongside multispectral images to highlight significant differences in LAI (Leaf Area Index) and LCC (Leaf Chlorophyll Content) dynamics between cultivars and nitrogen levels through high-throughput phenotyping (Impollonia et al., 2022; Sun et al., 2022) demonstrated that incorporating prior information about LAI and average leaf inclination angle can improve the accuracy of inversion of PROSAIL for leaf pigment estimation. However, if there is a high level of uncertainty, the use of prior information can result in decreased accuracy. Using the PROSAIL model to simulate future hyperspectral sensor setups, (Berger et al., 2018b), showed that significant improvements in accuracy for LCC estimation could be achieved by excluding wavelengths (optimized spectral sampling) with the highest model errors. Recent advances in UAV-based remote sensing have enabled the estimation of crop biophysical parameters like leaf area index (LAI) and chlorophyll content (Cab) through inversion frameworks that couple radiative transfer models with optimal estimation theory (Zheng et al., 2023; Ji et al., 2024). While promising, these methods require further refinement to address challenges like spectral overlap, soil variability, and atmospheric effects. A study by (Lu et al., 2021) highlighted the importance of understanding the limitations of RTMs and taking appropriate measures to improve the performance and successful application of a given model in vegetation trait studies. A systematic overview of these models and their applications towards agricultural and grassland monitoring can be found in these studies (Darvishzadeh et al., 2011; Darvishzadeh et al., 2012; Atzberger et al., 2015; Verrelst et al., 2021b; 2021a).
In this review, we analyze the advances surrounding the use of RTMs (PROSAIL) and spectroscopy to retrieve and explain the interactions between grassland vegetation traits and soil properties. We also explore existing challenges and the potential of using vegetation spectra as an integrated measure to explain underlying soil characteristics. Our focus was on studies that utilized hyperspectral spectrometer-sampled spectral reflectance data, while recognizing the value of other remote sensing data sources. Estimation of key plant traits (LCC and LAI), detectable from proximal and airborne sensor datasets, was reviewed. We also introduced a brief discussion of scale and how it affects plant trait retrieval processes. Next, we discussed how advancements in global satellite remote sensing are being utilized in trait detection. We conclude with current challenges and opportunities and provide suggestions and directions that are likely to further advance the study of vegetation-soil properties interaction.
The advancements in remote sensor technologies and data analysis methods over the past few decades have led to an increase in the use of remote sensing in grassland studies. The quality of the data produced by remote sensors depends on their spatial, spectral and temporal resolution, with low spatial and high temporal resolution data being best suited for seasonal vegetation health studies (Brown and de Beurs, 2008). However, Some studies have shown that combining low spatial and high temporal resolution data with high spatial and low temporal resolution data can provide improved results in grassland studies (Danner et al., 2021; Liu et al., 2021). Plant traits, including biochemical properties (e.g., pigments, water content), structural properties (e.g., LAI, biomass), generally govern the functioning of plants and their responses to the environment. For example, LCC, a major plant pigment, plays a significant role in regulating the amount of solar radiation absorbed by a leaf and thus impacts a plant’s photosynthetic capabilities. Chl concentrations has served as an indicator of a plant’s nutrient status. Other pigments such as carotenoids and anthocyanin also play important roles in light-harvesting and protecting leaves from excessive light (Gitelson et al., 2006; Sousa, 2022). Given their importance, spatial and temporal information on plant pigment dynamics is valuable for various scientific purposes, exploring the relationship between pigments and soil properties. Plant traits and environmental factors (e.g., soil moisture) influence how plants absorb and scatter light, affecting their spectral reflectance (Ustin and Jacquemoud, 2020). This reflectance is determined by the interaction of radiation with leaves/canopies, including leaf structure, shape, area, and orientation.
Laboratory measurement of plant pigments typically involves solvent extraction (e.g., methanol, acetone) followed by spectrophotometric analysis or high-performance liquid chromatography (Lichtenthaler, 1987; Dunn et al., 2004). For large-scale canopy analysis, extrapolating from limited point-sample measurements can introduce inaccuracies. Additionally, this approach cannot monitor temporal pigment changes, especially at the leaf level. Handheld instruments like Minolta SPAD (Markwell et al., 1995) offer a faster, non-destructive alternative for total chlorophyll estimation, but lack specificity for individual pigments. Remote sensing complements these methods by measuring upward radiation to estimate pigment content across broader areas. The signals are sampled and quantized into raw values at different wavelengths, forming spectral radiance, which can be converted to an estimate of diffuse reflectance. This allows the use of reflected radiation measurements to quantify plant pigments. Field sample spectral data can be captured by various devices equipped with proximal, airborne or spaceborne hyperspectral sensors. Such sensors range from non-imaging portable spectrometers such as the ASD (Analytical Spectral Devices, Inc. Boulder, United States), to imaging sensors such as the Micro-HyperSpec (Headwall Photonics Inc. Boston, MA, United States) which can be mounted on manned or unmanned aerial vehicles. Recently, advanced spaceborne imaging spectrometers, such as DLR’s Earth Sensing Imaging Spectrometer (DESIS) on the International Space Station (ISS), PRecursore IperSpettrale della Missione Applicativa (PRISMA), and Compact High-Resolution Imaging Spectrometer (CHRIS) on NASA’s Hyperion Earth Observing-1, have been developed with high spectral and spatial resolutions. These imaging spectrometers have provided large-scale vegetation reflectance datasets, enabling researchers to test and improve methods for estimating pigment concentrations. Forthcoming generations of operational spaceborne imaging spectrometer missions, including ESA’s Copernicus Hyperspectral Imaging Mission for the Environment (CHIME), FLuorescence EXplorer (FLEX), DLR’s Environmental Mapping and Analysis Program (EnMAP), the Italian-Israeli Spaceborne Hyperspectral Applicative Land and Ocean Mission (SHALOM) and NASA’s Surface Biology and Geology (SBG)) are expected to provide a new generation of Earth observation (EO) data and help advance a wide range of applications in grassland monitoring. The study from (Wang Z. et al., 2022) outlines some recent technological advances and applications of proximal, airborne, and spaceborne imaging spectrometry for remote sensing of grasslands. Extracting meaningful information from existing or forthcoming hyperspectral data requires rigorous preprocessing to address noise, atmospheric effects, and geometric distortions. Advanced techniques like spectral unmixing and non-negative matrix factorization are essential for separating endmembers (pure spectral signatures) and estimating their abundances within mixed pixels (Bioucas-Dias et al., 2012). By overcoming these challenges, hyperspectral remote sensing can provide valuable insights into plant traits, ecosystem dynamics, and their relationships with environmental factors.
Hyperspectral sensors capture highly detailed spectral data (hundreds/thousands of narrow bands) at high spatial resolution (cm-scale). This allows detection of subtle features linked to specific plant traits (e.g., pigments, biomass) (Eon et al., 2019; Rueda-Ayala et al., 2019; Lu et al., 2021; Wang et al., 2021). Plant pigments have unique spectral signatures, enabling their study with hyperspectral data (Figure 1). For instance, scattering within the canopy (influenced by plant structure) increases water absorption at the leaf level, raising reflectance in the NIR-SWIR region compared to other wavelength areas (Asner, 1998; Holzman et al., 2021).
Figure 1. Leaf Spectral Reflectance (350–2,400 nm) of Grasslands in Beltville, Maryland, United States (Collected on 202208–12). Key spectral regions: Visible (green), Near-Infrared (brown), and Shortwave Infrared (blue). Water absorption features indicated by blue arrows.
Chlorophyll, the main pigment in green plants, primarily affects the visible light region (400–700 nm) of the EM spectrum. It strongly absorbs light in the blue and red regions, at the same time, reflects green light, giving plants their green color. In remote sensing, the amount of light reflected in the green region (around 550 nm) can be used to estimate LCC (Gitelson et al., 1996; 2003; Gitelson and Merzlyak, 1998; Lu F. et al., 2019). Biophysical quantities such as LAI, biomass, and plant density can impact the spectral reflectance measured by remote sensing instruments. For example, For example, higher LAI values typically result in lower reflectance in the visible spectrum and higher reflectance in the near-infrared region (Darvishzadeh et al., 2008; Din et al., 2017). However, it is important to note that environmental factors like light availability, soil moisture, and atmospheric conditions can influence this relationship, making it complex. For instance, In the SWIR region, soil moisture content and roughness are known to significantly influence the reflectance spectra, thus, soil moisture can be estimated using SWIR bands (Lobell and Asner, 2002; Wang et al., 2008; Oltra-Carrió et al., 2015). However, atmospheric water vapor partially overlaps with and is close to some of the liquid water absorption bands, complicating retrievals in these regions. Soil texture has also been shown to affect reflectance at different wavelengths (Curcio et al., 2013). These changes in reflectance can be used to infer information about soil properties and vegetation-soil interactions (Asner and Heidebrecht, 2002; Effects of Ground Cover and Leaf Area on the Spectral Reflectance of Vegetation-Soil Target). By analyzing hyperspectral data, scientists can investigate the relationship between plant pigments and soil properties. However, scaling leaf-level measurements to the canopy and separating the effects of vegetation structure and chemistry remain significant challenges. To address these issues, researchers have combine leaf hemispherical reflectance and transmittance measurements with canopy radiative transfer models. (Berger et al., 2018b; Schiefer et al., 2021; Luo et al., 2022).
Sensor resolution is crucial for capturing narrow trait-related features. A spectral resolution of 10 nm band spacing, and 10 nm full-width half maximum is generally considered necessary (Schiefer et al., 2021; Chakhvashvili et al., 2022). Additionally, sensor characteristics such spectral distortion, signal-to-noise ratio should be taken into consideration. A sufficient signal-to-noise ratio is crucial for identifying important spectral features, considering random noise from sources such as detector sensitivity. Hyperspectral data requires pre-processing for trait analysis, including atmospheric correction, illumination and directional effects correction, and wavelength calibration. Calibration is essential for comparing data across sources and locations for canopy trait mapping Different sensors may have varying band centers, and these can change, especially for airborne devices, so data resampling may be necessary. This process can be data and computationally intensive and requires a thorough understanding of spectral response functions or the ability to recalibrate models to new wavelengths for precision.
Given the expansion of hyperspectral sensors, there is a growing need for methods/models that can effectively utilize such datasets to identify vegetation biochemical and structural properties. This is particularly relevant for the study of grassland trait studies, as the use of UAV-based hyperspectral systems and the new and upcoming generation of hyperspectral satellites (such as CHIME, EnMAP, HISUI, HypXIM, HyspIRI, and PRISMA etc.) are becoming more widespread in industry. To estimate pigment concentrations from hyperspectral datasets, a range of other factors (e.g., leaf internal structure, surface characteristics, soil characteristics, etc.) that influences leaf reflectance spectra must also be considered. As seen in the previous section, pigments, water, and other biochemical properties absorb certain wavelengths of radiation, thus, lowering reflectance in those regions. This offers an opportunity for pigments to be studied in a non-destructive manner (Sims and Gamon, 2002). For example, some studies have shown that assessing the functional properties or status of growth of a plant could be feasible by examining how particular traits (Chl-a, LAI, etc.) influences the plant’s optical properties within specific regions of the EM spectrum (Kattenborn and Schmidtlein, 2019; Impollonia et al., 2022; Sun et al., 2022). However, due to overlapping absorption features of leaf pigments and other constituents, relating reflectance at a single wavelength to a pigment is not advisable (Berger et al., 2018b). This has led to several studies using reflectance from multiple narrow bands to overcome the non-linearity effect between pigments and reflectance (Heckmann et al., 2017; Silva-Perez et al., 2018; Fu et al., 2019).
Leaf reflectance spectra contain many data points across wavelengths. A radiometer’s spectral resolution (wavelength of 350–2,500 nm), especially for trait studies with overlapping absorption features, is crucial for capturing subtle variations in leaf traits (Prudnikova et al., 2019). Several methods analyze specific spectral regions linked to leaf characteristics. For example, studies have investigated the influence of soil background on crop canopy reflectance in the visible and near-infrared regions (Prudnikova et al., 2019), finding the impact most pronounced between 350–500 nm and 620–690 nm. Likewise, Berger et al., (Berger et al., 2018b), used models to evaluate the potential of future hyperspectral sensors in the visible and near-infrared (VNIR) regions. Their results showing that the green visible and red edge regions had major inaccuracies due to complex interactions of variables. However, they further demonstrated that optimizing through spectral samplings improved the accuracy of LCC estimates. Although spectroscopy offers promise for mapping plant traits in remote sensing (RS), there’s no single, universally accepted method for deriving these traits due to challenges in connecting traits to RS data across scales. However, two established methods exist: (1) a physics-based radiative transfer models and (2) empirical models (Jacquemoud et al., 2009; Verrelst et al., 2012; Féret et al., 2017). Recently, a combination of these approaches, known as the (3) Hybrid method (Verrelst et al., 2019a), has emerged. We will provide a brief overview of these retrieval categories below.
Regardless of the chosen method or model for estimating vegetation traits, it must effectively convert spectral observations into biophysical properties and manage the multicollinearity of adjacent bands that convey similar information. It is important to note that the variable boundaries of retrieval categories mentioned above are never fixed/straightforward. These boundaries continually evolve with advancements in computational speed and sensor technology. For example, future missions like the Environmental Mapping and Analysis Program (EnMAP) will provide more detailed hyperspectral data for remote sensing analysis at 6.5 and 10 nm spectral sampling distances in the VNIR and SWIR regions, respectively. This will bring new insights into the biochemical and biophysical properties of different large-scale terrestrial ecosystems. While compact hyperspectral data sets are useful for pigment identification, they present a challenge in terms of spectral band redundancy. Adjacent bands often contain similar or interrelated information, making it essential that any method or model used for vegetation trait retrieval be able to handle this issue of multicollinearity.
Radiative transfer models (RTMs) are essential components of optical remote sensing and play a crucial role in connecting observations with the physical processes that generate signals in remote sensing. RTMs are widely used in various applications, including sensor radiometric calibration, atmospheric correction, and the modeling of radiation processes in vegetation canopies (Verhoef, 1984; Vermote et al., 1997; Berk et al., 2014; Verrelst et al., 2016b). In the context of vegetation studies, RTMs are mathematical models used to simulate the interaction of light with vegetation canopies. RTMs are based on the physical principles of light propagation through a medium and can be used to predict the reflectance and transmittance of light by vegetation canopies as a function of various canopy properties, such as LAI, LCC, and leaf angle distribution (Jacquemoud, 2000; Ustin and Jacquemoud, 2020). There are several RTMs used to simulate canopy spectra, including simple one-dimensional RTMs and complex three-dimensional RTMs. These models vary in their level of sophistication and accuracy, with 1-D RTMs (e.g., PROSPECT (Jacquemoud and Baret, 1990)) providing basic representation of canopy spectra, while 3-D (e.g., Monte Carlo ray tracing models (Govaerts and Verstraete, 1998)) RTMs allow for a more detailed and accurate representation of complex canopy structures. As seen in Table 1, both types of RTMs have their strengths and weaknesses and the choice between them depends on the specific requirements of each study.
For all RTMs, identifying the key input variables that affect the spectral output is crucial, even if it means simplifying the model by ignoring less important variables (Kimes et al., 2000; Verrelst et al., 2016b; Lunagaria and Patel, 2019; Impollonia et al., 2022). Simplifying RTMs enhances their usability, particularly for trait retrieval applications. RTMs are employed in both forward and inverse modes for studying grassland traits. In forward mode, RTMs generate databases of reflectance spectra by varying input parameters (e.g., LAI, chlorophyll, leaf structure). Analyzing these databases helps identify spectral features sensitive to specific traits. Examples of RTMs that have been designed for this purpose include PROSPECT (PROpriétés SPECTrales) (Jacquemoud and Baret, 1990) and LIBERTY (Leaf Incorporating Biochemistry Exhibiting Reflectance and Transmittance Yields) (Dawson et al., 1998) etc. These models vary in terms of computational complexity, input variable requirements, and how they represent the interaction between light and the leaf or canopy structure. The inverse mode of RTMs has been used to estimate plant traits such as LCC. The successful use of the PROSPECT model at both the leaf and canopy level has demonstrated the potential of this approach (Darvishzadeh et al., 2012; Zhang et al., 2017; Piegari et al., 2021). RTMs can be used to simulate the spectrum of leaves or canopies for various growth stages and conditions. However, to accurately parameterize RTMs, it is necessary to have a certain amount of input information, such as ground observations or synthetic data. It is recommended to gather input variables from a diverse range of plant, soil, and weather scenarios, preferably over different seasons. This will help to ensure that the RTM is accurate for a wide range of conditions. In cases where ground data is not available, a number of studies have used prior knowledge to set their model variables within specific bounds (Li et al., 2015; Berger et al., 2018a; de Sá et al., 2021; Wang J. et al., 2022).
The reflectance spectrum of a leaf or canopy is influenced by factors such as non-photosynthetic vegetation (NPV), non-leaf elements, shadows, and soil reflectance. In grassland management, layers of dead vegetation on the soil surface can retain moisture, prevent erosion, and reduce soil compaction (Arsenault and Bonn, 2005). Understanding spectral reflection and pigment concentrations in grasslands requires accounting for the impact of NPV and non-leaf elements. Differentiating between green and non-green grassland components in radiative transfer model (RTM) simulations is challenging, as most RTMs focus on photosynthetically active plants. To address this, non-photosynthetic plant parameters have been analyzed using RTM outputs integrated with remote sensing, meteorological, and in situ data. For example, (Berger et al., 2021a), trained Gaussian process regression (GPR) models with carbon-based constituents variable at canopy level over a simulated data from the PROSAIL-PRO model. The resulting GPR model was applied to PRISMA hyperspectral images, estimating non-photosynthetic biomass in croplands and bare soils. Such approaches also allow for the study of crop biomass (Berger et al., 2021a) and gross primary production from reflectance spectra (Cheng et al., 2020).
When studying vegetation traits, computationally expensive RTMs like Monte Carlo ray tracing models (Govaerts and Verstraete, 1998) can be limiting due to their high number of input variables and slow processing times. In contrast, more economical models like PROSAIL, which combines the leaf RTM PROSPECT (Jacquemoud et al., 2009; Féret et al., 2017) with the canopy RTM SAIL (Verhoef, 1984; Verhoef et al., 2007), require fewer input variables and have a faster processing time, making them more practical for application. Despite the computational demands, RTMs can produce high-resolution spectroscopic outputs, suitable for both imaging and non-imaging spectroscopy data, with outputs resampled to match the spectral resolution of different sensors. Thus, selecting an RTM involves balancing the model’s complexity and realism with its feasibility for inversion, as simpler models are easier to invert but may be less realistic.
Another challenge in running and inverting RTMs is that different combinations of leaf or canopy input variables can produce similar reflectance signatures, leading to an increased number of unknowns, known as the ill-posed inversion problem (Jacquemoud et al., 2009). To address this, various regularization strategies have been proposed, such as iterative numerical optimization and look-up table (LUT) based inversion methods. Both strategies aim to find the best match between measured and RTM-simulated reflectance or field-derived observations. A hybrid approach has also emerged, using LUTs as input variables for machine learning models (Jacquemoud et al., 2009; Verrelst et al., 2015a). Briefly, the numerical optimization method involves iteratively estimating the difference between measured and simulated reflectance through a cost function. While reliable, it can be time-consuming, computationally demanding, and prone to converging to a local minimum (Kimes et al., 2000; Berger et al., 2018a). The LUT-based inversion strategy, on the other hand, uses a range of possible input variable combinations to generate simulated spectral reflectance. By applying a cost function like Root Mean Square Error (RMSE), the simulated spectra can be compared to measured ones to find the closest match across all wavelengths. Recent studies suggest that using multiple cost functions can improve the accuracy of inversion in predicting leaf and canopy properties such as LCC and LAI (Darvishzadeh et al., 2012; Sehgal et al., 2016; Impollonia et al., 2022; Antonucci et al., 2023). To further mitigate the ill-posed problem and improve the accuracy of LUT-based inversion routines, various regularization strategies have been proposed. Some of which include adding white Gaussian noise to account for measurement uncertainties, utilizing prior information about input variable ranges to constrain the RTM, and applying spectral subsets (Chakhvashvili et al., 2022; Sun et al., 2022; Xie et al., 2022; Xu et al., 2022).
While this review focuses on the application of the PROSAIL RTM, it is important to note that RTMs for vegetation canopies vary widely in their features, such as plant scattering behavior and the type of medium they model. Despite this, these models are preferred due to their transferability, robustness, and flexibility in describing light-trait interactions. Although computational models have limitations, technological advancements and robust in situ data have improved RTM inversion accuracy. For example, field campaigns and laboratory measurements are essential have been heavily used for model calibration. For a comprehensive overview of plant trait retrieval methods, previous studies and reviews should be consulted (Verrelst et al., 2013; 2015a; 2016b; 2019a; Berger et al., 2018a).
Empirical methods are widely used for studying plant traits by establishing regression models between specific light bands and plant traits sensitive to those bands. This approach often requires prior knowledge of trait-spectrum relationships to select particular wavelengths and corresponding spectral vegetation indices (SVIs) for analysis (Zeng et al., 2022). By taking advantage of the sensitivity of specific bands to certain plant traits, SVIs can emphasize the expression of a particular trait while minimizing interference from other plant traits, soil reflectance, atmospheric conditions, and other factors. Most SVIs involve comparing the reflectance of red (visible) and near-infrared wavelengths, with red sensitive to chlorophyll content and near-infrared to the mesophyll structure of leaves. For example, VIs composed of these wavelengths have been correlated with LAI and LCC, helping to distinguish grassland canopies (He et al., 2006). SVIs have proven to be a valuable tool for researchers in estimating traits, including Chlorophyll (Gitelson et al., 1996), LAI (Thenkabail et al., 2000), the fraction of absorbed photosynthetic active radiation (PAR) (Kamenova and Dimitrov, 2021). Among the many indices, the ratio vegetation index (RVI), normalized difference vegetation index (NDVI), and enhanced vegetation index (EVI) are the most used. For instance, NDVI has been used to characterize canopy cover by correlating it with green canopy cover (Tenreiro et al., 2021). A variety of indices have been developed and evaluated to establish a relationship between remotely sensed data and ground truth data for predicting leaf biophysical properties (Gitelson, 2004; Kamenova and Dimitrov, 2021). Some of these indices are listed in Table 2. For more information on vegetation indices and their applications, please refer to (Bannari et al., 1995; Haboudane et al., 2002; Xue and Su, 2017). When choosing VIs, the spectral sensitivity and features of VIs and their suitability for target application should be considered. In addition, timing of data acquisition in relation to plant growth stage as well as the bandwidth and spectral resolution of sensor used for data generation should be taken into consideration.
Calculating VIs is a fast and straightforward process but testing various combinations of contiguous bands to identify the “best index” can be tedious. Moreover, this method may overlook subtle trait information in contiguous spectral bands since it only focuses on specific regions of the spectrum. Parametric approaches based on spectral shapes and spectral transformations are more effective when working with hyperspectral images, as they extract trait information from contiguous spectral bands specific to a particular trait. The spectral shape approach includes methods such as the red-edge index, which is sensitive to chlorophyll (Gitelson et al., 1996) and LAI (Dong et al., 2019). The spectral transformation approach includes continuum removal and wavelet transform methods. Continuum removal can be used to calculate LCC by focusing on the area under the spectral curve between 550 and 730 nm (Broge and Leblanc, 2001). Further example applications of these methods to enhance the accuracy of plant traits (e.g., LAI, nitrogen etc.) be found in these studies (Huang et al., 2004; Luo et al., 2022).
Parametric methods enhance spectral transformations but require a fitting function to estimate plant traits. Although mathematically simple and fast, these methods cannot perform per-pixel analysis. Non-parametric regression methods, in contrast, optimize models through a learning phase and can handle full-spectrum data without explicit parameterization. These flexible models, often referred to as linear non-parametric models, must avoid overfitting by properly defining model weights. They are sometimes used in combination with principal component regression (PCR) (Wold et al., 1987), and partial least squares regression (PLSR) (Geladi and Kowalski, 1986) for hyperspectral data dimensionality reduction purposes. This helps to mitigate the collinearity effects, which is a problem that occurs when two or more variables are highly correlated. Other common methods include the stepwise multiple linear regression (SMLR) (Draper and Smith, 1981) and the ridge regression method (Geladi and Kowalski, 1986) etc. For a more comprehensive understanding of parametric and non-parametric models, further information can be found in a study conducted by Mahmoud (Mahmoud, 2021). Table 3 summarizes their strengths and drawbacks in estimating plant traits from remote sensing data.
Parametric methods such as PLSR, MLR, and CCA are effective for vegetation trait estimation from remote sensing data, but incorporating machine learning (ML) techniques can improve accuracy (Caicedo et al., 2014; Yao et al., 2015; Kiala et al., 2016; Wang et al., 2017; Féret et al., 2019; Burnett et al., 2021). ML methods can handle nonlinear relationships between spectral data and plant traits, various data types, and establish trait-spectral relationships without prior data distribution knowledge (Verrelst et al., 2012; Berger et al., 2021b). Some examples of ML methods used in vegetation trait inversion are decision trees, artificial neural networks (ANNs), and kernel-based regression families. These methods have been shown to be more accurate than parametric methods, especially when dealing with nonlinear relationships or small training datasets (Verrelst et al., 2015a; 2019a).
Kernel ridge models are a popular type of ML method for vegetation trait estimation. They are known for their ability to handle nonlinear relationships, identify subtle grassland traits, and robustly handle outliers and data noise (Tuia et al., 2018). Gaussian process regression (GPR) is a specific type of kernel ridge model that has been widely used for vegetation trait estimation. GPR is based on a Bayesian framework and fits data through individual functions, solving regression and probabilistic classification problems (Gehler and Schlkopf, 2009). GPR has been applied alongside multispectral and hyperspectral spectroscopic data from proximal, airborne, and spaceborne sensors to map leaf, LAI, and fractional vegetation cover (Ali et al., 2021; Sun et al., 2022; Antonucci et al., 2023; Zhao et al., 2023). Studies comparing GPR to other ML methods, such as ANNs, random forest regression (RFR), and support vector regression (SVR), have shown that GPR outperforms other methods for predicting LAI and LCC from spectroscopic data (Caicedo et al., 2014; Verrelst et al., 2019a). GPR is preferred because it is easy to implement, has hyper-parameter adaptive capabilities, and flexible nonparametric inference (Verrelst et al., 2019a). Additionally, GPR provides uncertainty intervals that can be used as a metric to evaluate the performance of the model (Verrelst et al., 2013). However, effective ML algorithms may encounter issues with multicollinearity and computational expense, especially with large training datasets. Nonetheless, using spectral dimensionality reduction and active learning (semi-supervised approach) can mitigate these problems by optimizing sampling domains for the trait of interest. (Berger et al., 2021b; Danner et al., 2021; Salinero-Delgado et al., 2021).
For more details on ML approaches (e.g., ANN, SVR) and their applications in vegetation trait estimation (LCC, LAI, etc.) under diverse environmental conditions, please refer to the comprehensive descriptions in the journals by Ali et al. (Ali et al., 2021) and Danner et al. (Danner et al., 2021).
Future hyperspectral missions will generate increasingly complex data (high spatial, spectral, and temporal resolution). Processing and analyzing this data for large-scale trait estimation requires significant computing power. Hybrid approaches, combining the efficiency of machine learning (ML) with the detail of radiative transfer models (RTMs), offer a promising solution (Verrelst et al., 2019a). The hybrid method of vegetation trait estimation uses both radiative transfer models (RTMs) and machine learning (ML) methods to improve the accuracy of estimating vegetation traits. In these hybrid methods, RTMs simulate light interaction with vegetation, considering leaf properties and canopy structure. These simulations are then used to train ML models (e.g., ANNs, SVRs) to estimate traits like LCC or LAI from real spectral data.
A major advantage of the hybrid method is that it combines the accuracy of physical models provided by RTMs with the flexibility and speed of ML methods (ANNs, GPR, RFs, SVRs). This has resulted in a recent increase in studies on hybrid retrieval of plant traits using ML algorithms (Verrelst et al., 2016b; 2021a; Abdelbaki et al., 2019; Danner et al., 2021; de Sá et al., 2021). For example, a study by Berger et al. (Berger et al., 2020) employed a hybrid retrieval method that combined a physically based approach with machine learning regression to estimate crop nitrogen (N) content. The results showed that the Gaussian process (GP) model was more effective in estimating N content, and the SWIR spectral region provided the best spectral settings. Another study used active learning and spectral dimensionality reduction strategies to optimize a hybrid hyperspectral retrieval model for mapping LAI and fractional vegetation cover over a heterogeneous landscape (Pascual-Venteo et al., 2022). In addition, a hybrid retrieval scheme was tested using data from the new-generation PRISMA satellite, which involved PROSAIL-PRO radiative transfer simulations coupled with Gaussian processes regression to estimate crop traits. The results were positive for all variables investigated, demonstrating the potential of spaceborne imaging spectroscopy for crop monitoring and the possibility of routinely retrieving multiple crop traits over large areas (Tagliabue et al., 2022).
The hybrid approaches are particularly useful in situations where ground-truth measurements are difficult or costly to obtain, and where RTMs alone may not be accurate enough. Here, the hybrid method offers another significant advantage in that, since the RTM simulations are utilized to train the statistical model, the dependency of the RTM on ground input data for forward model simulations is reduced. This allows for the use of ground measurements primarily for model estimate validation. Table 4 below provides a concise summary of the advantages and disadvantages of the hybrid retrieval method compared to empirical and RTM methods.
Understanding the fundamentals of a hybrid retrieval scheme is crucial, as the determination of which methods constitute a hybrid approach is at the discretion of the researcher and depends on the vegetation properties of interest. Regardless of the specific statistical technique utilized to construct a hybrid retrieval method, it is crucial to recognize that it does not resolve the underdetermined nature of the problem associated with RTMs. Thus, the same regularization methods described earlier should be implemented to address this issue. This may involve utilizing domain knowledge to limit model variables, introducing artificial noise to accommodate model and measurement uncertainties, and/or employing multiple optimal solutions in the inversion process (Berger et al., 2018b; 2018a; Verrelst et al., 2019a; Danner et al., 2021).
This review comprehensively analyzes previous literature on vegetation trait retrieval methods, including RTM inversion and their acknowledged limitations (Jacquemoud et al., 2009; Berger et al., 2018a; Verrelst et al., 2019a). Our focus extends beyond summarizing methodologies, delving into how model vegetation trait estimates relate to changes in soil properties like moisture, as well as other physical and geotechnical properties such as density and composition. Furthermore, we assess the evolving impact of past publications and speculate on potential future developments within the field.
To assess relevant study trends, we conducted bibliometric analysis of search engine results. We identified the most relevant published papers (n = 318) through search engine database queries (Google Scholar, Scopus, Web of Science, and Dimensions) and journal-specific searches, documenting key attributes. This analysis allows us to examine trends in the application of models, research focus, and study scale over time. We specifically focus on proximal, airborne, or spaceborne hyperspectral remote sensing publications related to grasslands.
PROSAIL (PROSPECT + SAIL) is a radiative transfer model used for simulating the optical signals of vegetation. The model simulates how sunlight interacts with vegetation canopies under various conditions, including sun angles, atmosphere, and plant properties (Jacquemoud and Baret, 1990). A detailed description of the PROSAIL model can be found in (Jacquemoud et al., 2009).
The PROSPECT model is utilized at the leaf scale to estimate the biochemical and biophysical traits of vegetation by replicating the spectral response of a leaf in the VIS-NIR-SWIR range. The model assumes the leaf is composed of N parallel homogeneous layers as plates. It requires inputs of structural parameters of the leaf, such as leaf thickness, dry matter content, and number of mesophyll cell layers, as well as spectral data for the leaf being modeled. The latest version of the model, “PROPECT-PRO,” was introduced in 2020, with an improved feature of incorporating leaf protein content as an input variable (Féret et al., 2020). Table 5 summarizes the input variables for the PROSAIL model, including their symbols, units, and typical ranges for grasslands.
The SAIL radiative transfer model is a 1-D model that simulates the bidirectional reflectance of vegetation canopies (Verhoef, 1984). The model divides the canopy into several distinct layers and models the interaction between light and each layer. Each layer is characterized by its LAI, leaf angle distribution, and optical properties. The model then combines these individual layers’ reflectance to produce the overall reflectance of the canopy. The most recent variant, 4SAIL2, is designed for numerical stability and speed optimization (Verhoef and Bach, 2007). This variant is a hybrid two-layer model that has been enhanced to account for the hot spot and clumping effects, as well as improve the accuracy of the canopy absorbance output.
SAIL was first developed to analyze uniform canopies, but it has since been adapted to fit different research purposes discussed in dedicated published articles (Jacquemoud, 2000; Verhoef and Bach, 2007; Jacquemoud et al., 2009; Ustin et al., 2009; Berger et al., 2018a).
This review focuses on how PROSAIL model variants have been used to link vegetation traits with soil characteristics. PROSAIL model calculates the reflectance of vegetation canopies from 400 to 2,500 nm in 1 nm increments. The model takes up to 16 input parameters Table 5, which include factors such as pigment and water content, canopy structure, soil background, hot spot, solar diffusivity, and observation geometry.
The latest version of the PROSAIL model, PROSAIL-PRO, couples a leaf-level radiative transfer model (PROSPECT-PRO) with a canopy-level model (4SAIL). PROSPECT-PRO is a leaf model that simulates the directional-hemispherical reflectance and transmittance spectra of plant leaves in the 400–2,500 nm range based on various leaf characteristics. These characteristics include the leaf structure index (N), protein, PRO, CBC, chlorophyll content (Cab), carotenoid content (Car), anthocyanin content (Canth), equivalent water thickness (Cw), and dry matter per area (Cm). PROSPECT-PRO can be used in two modes: forward mode and inverse mode (Figure 2). In forward mode, the model is used to simulate the optical properties of leaves based on a set of input parameters. In inverse mode, the model is used to determine the input parameters based on the leaf optical properties.
The 4SAIL model (Verhoef et al., 2007), is a one-dimensional bidirectional model that uses radiative transfer equations to calculate the scattering and absorption of four fluxes at canopy level. It assumes leaves are small and randomly distributed in space, making it well-suited for grasslands and agricultural canopies (Verhoef, 1984). 4SAIL uses LAI, ALA and the hotspot (hspot, ratio of mean leaf size to height of canopy) parameters to describe the canopy structure. Coupled with PROSAIL-PRO, it simulates top-of-canopy reflectance in the 400–2,500 nm range with 1 nm resolution, based on various biophysical and biochemical parameters like LAI, ALA, Cab, Car, Cw, and soil reflectance. Table 5 provides an overview of input parameters with symbols and units.
The parameter ranges for PROSAIL-PRO forward simulations should be adjusted based on the characteristics of the study area (Berger et al., 2018a; Verrelst et al., 2019a). These ranges (Table 5) are suitable for a wide range of plant species but may need to be adjusted for specific applications (Verhoef, 1984; Verhoef and Bach, 2007; Berger et al., 2018b; 2018c). Weather information from the day of in-situ data collection is used to keep sun and view geometry angles constant. The model training process is randomized to prevent strong correlations between predicted variables, maintain aggregation benefits, and avoid overfitting.
To construct a look-up table (LUT) for the PROSAIL-PRO model, users must execute the model for various input parameters, which can be defined based on field surveys. Parameter ranges are specified by the user, and variable distributions can be uniform, Gaussian, or a combination of both to reflect realistic variability across seasons (Verrelst et al., 2016a; Féret et al., 2017; 2020; Berger et al., 2018a; Lunagaria and Patel, 2019). The model generates reflectance spectra corresponding to the specified parameter space, stored in a database serving as the LUT for future model inversions. The recommended LUT size for PROSAIL-PRO varies based on input parameters and desired accuracy. While larger LUT sizes offer enhanced precision, they demand more storage space and computational time. (Duan et al., 2014). observed comparable accuracy in LAI estimates across LUT sizes of 50,000 to 250,000 simulations. (Darvishzadeh et al., 2012). reported similar outcomes for rice chlorophyll estimation. For accurate model inversions, a LUT size of at least 10,000 spectra and above is recommended, but the optimal size depends on the study objectives and constraints (Darvishzadeh et al., 2011; 2012; Berger et al., 2018a; Danner et al., 2021).
Once generated, the LUT employs a cost function (e.g., correlation coefficient, root mean square error, mean absolute error) to identify the best-fitting simulated spectra to measured reflectance spectra for corresponding input parameter values. The choice of cost function depends on the specific application. Notably, 0 the cost function is only a measure of how well the simulated spectra fit the measured spectra. The accuracy of the estimated values depends on the quality of the LUT and the number of simulated spectra that are used.
Working with large databases and Look-Up Tables (LUTs) can be computationally expensive and prone to multicollinearity. Multicollinearity arises when variables are highly correlated, making it challenging to estimate individual variable effects accurately. Band optimization is a technique that can be used to reduce the impact of multicollinearity and computational workload. It involves selecting a subset of bands from the LUT that are most informative for the estimation of a particular variable.
There are a variety of methods that can be used for band optimization, such as ranking evaluation criteria or genetic algorithms. (Verrelst et al., 2012; 2016b; 2021a). GPR models based on Gaussian Processes (GPs) (Rasmussen, 2004) are ML approaches suitable for this purpose. GPs are data-driven models whose means and variances are functions of their input vectors. These models have been successful in solving Earth observation (EO) problems in recent years, particularly in model inversion scenarios (Verrelst et al., 2013; Berger et al., 2021a; de Sá et al., 2021; Pascual-Venteo et al., 2022; Tagliabue et al., 2022). GP models have a solid Bayesian formalism, which allows for the inclusion of prior knowledge about the problem and the quantification of uncertainty and error propagation. This can be helpful for making decisions about the accuracy of estimates. However, implementing GPs can be challenging when determining signal models and kernel/covariance functions, especially for different signal-to-noise ratio (SNR) relations. GPR models typically assume that the noise variance is constant across all observations. This assumption may not be valid in cases where the noise variance is dependent on the input variables. To address this issue, two types of GPR models can be used: standard GPR models and heteroscedastic GPR models. Standard GPR models assume that the noise variance is constant, while heteroscedastic GPR models allow the noise variance to vary across observations. The noise variance and kernel hyperparameters for standard GPR models can be estimated by maximum log-likelihood (Rasmussen, 2004). For heteroscedastic GPR models, a variational inference procedure can be used to estimate these parameters (Lázaro-Gredilla et al., 2014). Heterogeneous GPR models have been shown to slightly outperform standard GPR models in terms of accuracy (Berger et al., 2020). However, standard GPR models have also been successful in estimating vegetation trait properties (Mateo-Sanchis et al., 2018).
The process of selecting the optimal set of bands/features can be divided into four steps:
1. Subset generation: This step involves generating a set of candidate subsets of bands by sequentially removing bands from the full set of bands.
2. Evaluation of subsets: This step evaluates the performance of each candidate subset using a cost function, such as the normalized root mean square error (NRMSE).
3. Stopping criteria: This step determines when to stop the process of generating and evaluating candidate subsets.
4. Result validation: This step validates the results of the band selection process by using a holdout dataset.
The selection of bands is typically based on their reflectance sensitive regions, which can be determined through a sensitivity analysis of PROSAIL (Verrelst et al., 2019b). A sequential backward band removal (SBBR) algorithm is commonly used to generate candidate subsets of bands. This technique begins with a full set of bands and evaluates subsets based on information criterion that ranks the unconstrained length-scale parameter. The SBBR algorithm iteratively removes the band that has the least impact on the prediction error.
After each iteration, error statistics such as mean, maximum, minimum, and standard deviation are calculated for each subset of band combinations. Tenfold cross-validation is used to address any small differences between bands in a particular spectral region. Although the best combination of bands is selected based on a given cost function (e.g., least Normalized RMSE), it is important to be aware of high band correlation in outputs that may affect the regression output.
In this section of the review, we comprehensively explored studies utilizing various versions of PROSAIL RTMs to investigate photosynthetic and non-photosynthetic traits of a grassland canopy. Additionally, we evaluate studies using PROSAIL models for soil property retrieval (e.g., soil moisture, roughness, texture) from remote sensing data, if any. Relevant studies that used PROSAIL RTMs to retrieve canopy traits were identified through a systematic search of the literature. We used a combination of keywords and search operators to search the databases of Google Scholar, Scopus, Web of Science, and Dimensions. The specific keywords and search operators that we used are summarized in Table 6. In order to align with the current trends in studies, we narrowed our search to include only peer-reviewed, online-accessible articles from journals or books published between 2018 and 2023.
Our initial database search yielded hundreds of papers (Figure 3). After applying filters, we focused on studies using PROSAIL RTMs to investigate both photosynthetic (such as chlorophyll, LAI, etc.) and non-photosynthetic (including equivalent water thickness (EWT), litter, and soil moisture, etc.) traits. We also included relevant studies that aligned with our future research goals. Noteworthy contributions from some of these studies are highlighted in the discussions section.
Figure 3. Yearly distribution of studies investigating canopy properties using PROSAIL (2023 data covers publications up to December 2023)
The Zotero software was used to create and manage a local database of the relevant studies that met our search criteria. This database included key identifiers such as titles, authors, keywords, publication dates, etc. Duplicates were removed, and when necessary, the “Advanced Search” function of Zotero (Zotero, n.d.) (a free and open-source reference management software) was used to query the local database for the identification of key papers to be further discussed. This process resulted in a final selection of 318 relevant papers.
The following themes were used to screen the relevant articles:
• The purpose of the PROSAIL model application.
• Vegetation type analyzed.
• Soil properties studied.
• Leaf and canopy level biophysical and biochemical parameters retrieved.
• RTM retrieval method used.
• Use of hyperspectral data.
While hundreds of studies utilize PROSAIL for vegetation traits in grasslands, our focus here is on its potential for retrieving key soil characteristics.
Figure 4 illustrates the distribution of research papers focused on the investigation and estimation of various canopy traits using the PROSAIL model. The search results showed that the most studied canopy traits are chlorophyll and LAI. This is not surprising, given that these two traits are among the pivotal photosynthetic properties of a plant canopy. They are often studied together, possibly revealing the intricate interplay between Caband canopy structure. Conversely, our search results underscore a notable disparity in the number of studies exploring non-photosynthetic properties (leaf structure, EWT, and soil moisture). This is likely because they are not as directly related to photosynthesis.
The distinct gap in the research landscape highlighted by the plot offers a compelling opportunity for future investigations into canopy properties like soil moisture and EWT. These are both important factors that affect plant growth and productivity. These less-explored areas hold promise for uncovering valuable insights into vegetation dynamics, particularly in relation to water availability and non-photosynthetic canopy properties.
Search results showed that from 2018 to 2023, the most popular retrieval method is the LUT method, followed by the hybrid method, the parametric method, and the ML method. This analysis of the distribution of retrieval methods used to estimate canopy traits from recent peer-reviewed studies provides valuable insights into the trends and preferences within the field.
This prominence of studies using the LUT as their preferred trait retrieval method can be attributed to the inherent simplicity and efficiency of the LUT method. By utilizing a pre-calculated database of spectral signatures, the LUT method allows researchers to rapidly match observed data with known signatures, enabling quick trait retrieval. This approach is particularly effective when dealing with large datasets and offers a pragmatic solution to estimating canopy traits. Recent studies have demonstrated an increasing preference for the Hybrid retrieval method. This method integrates the strengths of both LUT databases and Machine Learning (ML) algorithms to enhance retrieval speed and accuracy. Hybrid methods leverage the extensive information present in LUT databases while employing ML techniques to fine-tune results. As research questions become more complex and dataset dimensions expand, a hybrid approach offers a well-balanced solution that combines the quick lookup capabilities of LUT databases with the adaptive learning of ML algorithms. This could be the reason behind the recent surge in adoption of this retrieval method.
In summary, most of the studies we examined employed a hybrid approach, combining the PROSAIL radiative transfer model with either empirical or machine learning algorithms, to retrieve a wide range of vegetation traits. However, as seen in Figure 5, very few studies focused their research on the potential of using PROSAIL estimates to understand aspects of grassland soil properties. Nonetheless, as demonstrated by its ability to retrieve vegetation traits, this approach has the potential for widespread use in a variety of fields. Combining PROSAIL with empirical or machine learning algorithms presents a promising avenue for future research in the retrieval of non-photosynthetic grassland properties.
Figure 5. Percentage distribution of canopy trait retrieval methods used in PROSAIL studies from 2018 to 2023.
The use of radiative transfer models (RTMs) has been growing in recent years, particularly in vegetation trait studies with synthetic and hyperspectral data. PROSAIL RTM, has played a crucial role in accurately retrieving vegetation traits and has become an essential tool in the field of remote sensing. As the demand for more comprehensive and detailed insights into vegetation-soil relationships grows, further research using RTMs like PROSAIL becomes imperative. While most research has predominantly focused on trait retrievals (Figure 4.), this section highlights recent studies that not only used and validated vegetation traits retrieval using the PROSAIL model, but also incorporated the investigation non-photosynthetic properties of their study areas, particularly soils. Studies of non-photosynthetic elements in grasslands can help us understand the complex interactions between vegetation and soil properties. We highlight these studies to inspire future research using PROSAIL and other RTMs to better understand these interactions.
The study conducted by Yu et al. (2014) aimed to develop spectral indices for estimating leaf Cab under varying natural conditions while minimizing the effects of canopy structure, soil background, and multiple scattering. Using PROSAIL, they generated a synthetic dataset of canopy reflectance to train and evaluate spectral indices. They hypothesized that a ratio of reflectance difference (RRD) index would be a more reliable chlorophyll indicator, less sensitive to soil and canopy variations than simple ratios or NDVI-like indices.
Their findings indicated that reflectance in the 660–680 nm (red) and 490–500 nm (blue) regions showed the highest variability, while the 730 nm (red edge) and 530–550 nm (green) regions exhibited the lowest, making them better suited for chlorophyll estimation. However, they noted the need for further validation to address soil background influences, especially during early growth stages when the canopy is sparse. The study emphasized the importance of validating spectral indices across diverse conditions and accounting for soil background effects in canopy reflectance data for accurate Cab estimation.
Similarly, (Darvishzadeh et al., 2008), investigated how external factors influence the estimation of vegetation LAI (LAI) using hyperspectral reflectance data. The authors proposed that narrow-band vegetation indices would exhibit higher sensitivity to LAI compared to broad-band indices, with reduced susceptibility to soil type and plant architecture influences. They executed a controlled laboratory experiment featuring four distinct plant species, two soil backgrounds, and destructive LAI measurements.
The findings revealed that the spectral contrast between leaves and soil background governs the LAI–reflectance (red/near-infrared) relationship. Notably, for light soils, a robust negative correlation existed between LAI and red reflectance. Conversely, for dark soils, this correlation was weaker. This discrepancy arises from dark soils absorbing more red light than near-infrared light, resulting in a diminished difference in reflectance between leaves and soil. Consequently, the authors underscored the importance of prior understanding of plant architecture and background soil characteristics when employing remote sensing vegetation indices for LAI estimation.
(Impollonia et al., 2022) conducted a study on hemp farming management, assessing cutting-edge precision agriculture technologies. They utilized UAV-based remote sensing technology to estimate hemp traits such as LAI and Leaf Chlorophyll Content (LCC). The study compared two PROSAIL model inversion techniques: Look-Up Table (LUT) and hybrid regression. Field measurements were employed for validation, and performance metrics like R2, bias, RMSE, and NRMSE were used to compare the methods. In the study, soil reflectance data were retrieved from a public database and adjusted to match the reflectance of the soil in the study area, which was then integrated into the PROSAIL model. This resulted in the generation of a look-up table (LUT) containing all possible values for LAI and LCC. However, they did not investigate how soil reflectance affected the trait estimates results. This is a limitation of the study, as soil reflectance can have a significant impact on the accuracy of remote sensing-based estimates of LAI and LCC. Overall, the authors suggest that hybrid regression methods are the most accurate for estimating hemp traits (LAI and LCC). However, future studies should investigate the impact of soil reflectance on the accuracy of these methods.
Live fuel moisture content (LFMC) is a crucial parameter for predicting fire behavior in shrublands. (Lai et al., 2022). compared the accuracy of physical and empirical models for estimating LFMC, considering the inherent heterogeneity of these ecosystems. Prior studies often relied on versions of the PROSAIL RTM assuming uniform shrub distribution, which Lai et al. argue is unrealistic. The authors used three RTMs (PROSAILH, PROGeoSail, and PROACRM) to simulate canopy spectra of shrublands with different levels of heterogeneity. They also calculated ten spectral indices from MODIS reflectance and used them for both empirical modeling and LFMC inversion. A unitless soil factor (psoil) was used to account for soil moisture content, but it was cautioned that psoil values may vary depending on soil type and moisture content.
The authors’ findings suggest that empirical models may be more suitable for LFMC estimation in open shrublands, and that spectral mixture analysis (SMA) or fine spatial resolution satellite images or microwave sensors may be needed to improve the accuracy of LFMC estimation in these areas. In closed shrublands, PROSAILH, PROGeoSail, and PROACRM showed similar performance in estimating LFMC. However, in open shrublands, all three models performed poorly due to mixed spectra and inadequate representation of soil properties. The study highlighted the significant influence of soil moisture on canopy spectra and LFMC estimation.
In their study, (Tian and Philpot, 2015), used soil-canopy radiative transfer models in the reflective optical domain to assess near-surface soil water content. They aimed to explore the feasibility of using optical Earth observation data for surface soil water estimation. By performing a sensitivity analysis of the PROSAIL model to soil reflectance variations and applying a simple LUT inversion technique to remote sensing and ground data from various agricultural sites, they found that these models effectively evaluate near-surface soil water. The LUT inversion technique proved valuable for estimating canopy and soil variables, with PROSAIL being sensitive to soil reflectance changes. The study recommended further research to enhance the accuracy and reliability of inversion techniques and to explore new applications of optical Earth observation data for diverse scenarios.
(Lu et al., 2021) assessed how well different PROSPECT and PROSAIL model iterations perform in estimating the spectral and biophysical characteristics of photosynthetic and non-photosynthetic vegetation in mixed grasslands. The authors found that the PROSPECT-5M model had the lowest root mean square error (RMSE: 0.015) for simulating the reflectance of non-photosynthetic leaves, followed by the PROSPECT-5 (RMSE: 0.023) and PROSPECT-D (RMSE: 0.031) models.
However, they noted that the models’ characterization of mixed canopies containing both photosynthetic and non-photosynthetic vegetation was challenging and recommended adding a parameter for decay pigments in the PROSAIL model to improve its accuracy. They also suggested further research to improve RTMs for non-photosynthetic vegetation and to test these models on a wider variety of vegetation types to enhance their applicability in mixed grasslands. Additionally, the authors acknowledged the potential confounding effects of litter and bare soil on LAI and chlorophyll estimation and called for further investigation in this regard.
(Eon and Bachmann, 2021) validated the MARMIT model for the retrieval of soil moisture content (SMC) from hyperspectral data in a field setting. The model was validated in laboratory experiments and further tested on data collected by a UAS-based hyperspectral imaging system with ground truth measurements. The MARMIT model is a radiative transfer model that accounts for how soil moisture, vegetation, and atmospheric conditions impact the reflectance of soil surface by simulating how light interacts with soil and water. The authors found that the MARMIT model was able to accurately estimate SMC from hyperspectral data, even in the presence of vegetation. The authors found that soil reflectance decreases with increasing soil moisture content (SMC), with the most significant decrease in SWIR wavelengths. This demonstrates the effectiveness of SWIR bands in characterizing water content using the MARMIT model. However, reflectance changes with SMC differ by soil type, indicating that the MARMIT model may require calibration for each soil type. Accurate retrieval of intermediate SMC values also depends on calibrating endmembers, particularly the dry and wet spectra. The model was validated with UAS-based hyperspectral imaging and ground truth measurements, showing promise for large-area SMC estimation. Nevertheless, as the study was in a controlled environment, results may not generalize to other conditions. Future research should test MARMIT in diverse environments.
(Gross et al., 2008) investigated the relationship between plant community functional traits (CFPs) and soil moisture in subalpine grasslands under different land uses. The study aimed to identify how plant traits respond to and affect soil moisture, as well as which traits mediate the relationship between plant communities and water availability. However, in the mid-late season, the effect of vegetation on soil moisture was also dependent on aboveground biomass. The authors conclude that leaf area and root length can be considered as response-effect traits, as they both respond to field abiotic conditions and affect soil moisture.
In their study, (Tian and Philpot, 2015), explored the relationship between soil water content and spectral reflectance in the SWIR region, focusing on three soil types in a lab setting. They aimed to understand how water absorption bands relate to evaporation rates and soil drying. By measuring water content and linking it to SWIR band depth, they used a simple model to illustrate the correlation between band depth and optical path. However, they noted that the true optical path involves complex reflections and refractions. Their findings suggested that SWIR band depth can estimate soil water content, though accuracy varies with soil type and particle size. They also highlighted that strong atmospheric water vapor absorption complicates field estimation.
(Schiefer et al., 2021) investigated the influence of plant phenology on trait retrieval from canopy reflectance using statistical and physically-based modeling. Their study, involving 45 herbaceous species, revealed phenology’s significant impact on model accuracy. While the hybrid inversion technique combining PROSAIL and PLSR showed slight advantages, all methods demonstrated comparable performance. However, limited ground truth data constrained generalization. The authors recommend future research with broader species representation and extended time periods, considering additional factors like soil and nutrient availability.
(Jay et al., 2017) explored the potential of ground-based multi-angular optical remote sensing for field phenotyping of sugar beet traits. Their study compared two retrieval methods: VIs and inversion of the PROSAIL RTM. They evaluated the effectiveness of both methods in remotely estimating LAI, leaf and canopy chlorophyll content, and leaf and canopy nitrogen content in sugar beet crops under field conditions. The study found that PROSAIL inversion outperformed VIs in estimating LAI, chlorophyll content, and nitrogen content in sugar beet crops. It proved to be more robust to variations in plant material and provided more accurate results. The accuracy of both methods was affected by the viewing angle, with the best results obtained from nadir and 15-degree views. This was because the nadir view provides the most direct view of the canopy, reducing the effects of atmospheric scattering and absorption, while the low angle view provides a better view of the understory of the canopy, which is crucial for plant health and productivity. The authors suggest that future research should explore the potential of multi-angular optical remote sensing using satellite or aircraft measurements for large-scale phenotyping applications.
In their study (Sinha et al., 2020), the researchers aimed to estimate LAI in a tropical broadleaved forest plantation within the Indo-Gangetic Plain of India. They assessed three techniques: vegetation indices (VIs), machine learning regression algorithms (MLRA), and physically-based inversion (LUT-inversion) using ARTMO and PROSAIL models, leveraging Sentinel-2 data. Their goal was to evaluate and compare these methods’ performance in LAI estimation and compare outputs with MODIS and Sentinel-2 LAI products to assess their reliability.
The authors found that the physically-based LUT inversion and MLRA-GPR methods outperformed VI-based parametric regression in LAI estimation. LUT inversion excelled in predicting peak season LAI, while parametric regression was superior for growing season LAI. Although MLRA-GPR had higher RMSE than LUT inversion, it provided a broader LAI range. PROSAIL proved effective in generating regional-scale spatial LAI maps through LUT inversion. The study emphasized the importance of NIR and SWIR bands in Sentinel-2 data for LAI estimation, given their sensitivity to leaf water content linked to LAI. It also noted LAI underestimation due to clumping effects in canopy imagery and recommended integrating clumping effect correction in the PROSAIL model for accurate LAI estimation.
While vegetation spectra hold immense potential for inferring underlying soil characteristics, effectively extracting soil information from mixed vegetation-soil signals presents significant challenges. Dense vegetation can significantly mask soil spectral signals, making it difficult to isolate pure soil reflectance. Additionally, soil and vegetation often exhibit overlapping spectral features, particularly in specific wavelength regions, complicating the separation of the two components.
Soil properties like moisture content, texture, and organic matter vary spatially, introducing complexity into spectral interpretation. These properties can also change with depth, affecting the spectral response and making it challenging to accurately represent subsurface conditions (Ben-Dor et al., 2009; Chabrillat et al., 2019). Vegetation canopy structure, including leaf area index and canopy cover, influences the amount of sunlight reaching the soil surface, thereby affecting soil spectral signatures (Mayel et al., 2021). Furthermore, vegetation biophysical traits, such as Chl and leaf water content, can introduce spectral variations that interfere with soil signal extraction.
Technological limitations also pose significant challenges. Traditional remote sensing sensors often lack the spectral resolution and sensitivity required to accurately capture subtle soil spectral variations. Atmospheric conditions, such as aerosols and water vapor, can distort spectral measurements, introducing noise and uncertainty into soil property estimation. To overcome these challenges, advanced spectral analysis techniques are essential (He et al., 2020; Li et al., 2022). Developing unmixing algorithms capable of separating soil and vegetation components in complex spectral mixtures is particularly crucial when dealing with hyperspectral data. Incorporating machine learning techniques can significantly enhance the accuracy and robustness an unmixing process (Bhatt and Joshi, 2020). Also, radiative transfer models to simulate spectral signatures under varying soil and vegetation conditions can enhance our understanding of spectral interactions and improve retrieval algorithms (Döpper et al., 2022).
Integrating ground-based measurements of soil properties with remote sensing data is essential for providing valuable calibration and validation insights. By combining data from different sensors, such as hyperspectral, multispectral, and radar, we can leverage their complementary strengths to enhance soil property estimation. As technological advancements in sensor innovation continue—such as the development of sensors with higher spectral resolution, wider spectral coverage, and improved signal-to-noise ratios—the capture of detailed soil spectral information becomes more precise. Coupled with the advancement of data processing algorithms and image analysis techniques, these innovations significantly improve our ability to extract accurate soil information from complex spectral data.
By addressing these challenges and capitalizing on emerging technologies, researchers can unlock the potential of vegetation spectra as a valuable tool for assessing soil properties. Continued advancements in remote sensing and data analysis will be essential for improving our understanding of soil-vegetation interactions and supporting sustainable land management practices.
Published studies often link optical properties to plant traits but typically focus on a single growth stage, often near the midpoint of the season (Croft et al., 2020). This approach overlooks the full range of conditions plant traits exhibit throughout the season. Consequently, findings may not apply across different seasons. To address this, radiative transfer models (RTMs) provide consistent optical data over time, offering insights into plant traits’ optical properties. However, linking spectral data to plant traits remains challenging due to the volume of data and variables like time, space, and observation geometry. Despite their validation, RTMs have not been extensively used to study the relationship between soil properties and overlying plant traits across various ecosystems.
To gain more insights into estimating soil properties from plant traits in grasslands, future studies could build on the advancements made in previous research. Studies like (Lai et al., 2022) can be enhanced by incorporating soil background effects into their spectral index estimation methods. By considering the interactions between vegetation traits and underlying soil properties, researchers can develop more robust and accurate models for soil estimation. Moreover, as shown in (Lu et al., 2021), the use of multiple PROSPECT and PROSAIL model iterations could be valuable for estimating non-photosynthetic elements in grasslands, offering potential clues for soil studies in similar areas. Additionally, (Schiefer et al., 2021), demonstrated the importance of considering plant phenology in trait retrieval. Future studies can improve accuracy by accounting for seasonal differences in nutrient allocation among roots and shoots, which could impact soil properties. Studies like (Orwin et al., 2010) that explore the impact of root-related traits on soil moisture content can be extended to grasslands, providing essential insights for soil property estimation.
In summary, to gain a comprehensive understanding of the intricate dynamics between vegetation traits and soil properties in grassland ecosystems, researchers should focus on modifying existing RTM techniques and incorporating soil-specific parameters (moisture content, texture, composition, density, etc.). By building upon the findings of previous studies and addressing the limitations, we can enhance the use of RTMs to estimate soil properties from plant traits effectively and contribute to the advancement of ecological research and environmental management.
WB: Conceptualization, Data curation, Formal Analysis, Funding acquisition, Investigation, Methodology, Project administration, Resources, Software, Supervision, Validation, Visualization, Writing–original draft, Writing–review and editing. AA: Funding acquisition, Project administration, Resources, Supervision, Writing–review and editing. TM: Supervision, Writing–review and editing.
The author(s) declare that no financial support was received for the research, authorship, and/or publication of this article.
The authors declare that the research was conducted in the absence of any commercial or financial relationships that could be construed as a potential conflict of interest.
All claims expressed in this article are solely those of the authors and do not necessarily represent those of their affiliated organizations, or those of the publisher, the editors and the reviewers. Any product that may be evaluated in this article, or claim that may be made by its manufacturer, is not guaranteed or endorsed by the publisher.
Abdelbaki, A., Schlerf, M., Verhoef, W., and Udelhoven, T. (2019). Introduction of variable correlation for the improved retrieval of crop traits using canopy reflectance model inversion. Remote Sens. 11, 2681. doi:10.3390/rs11222681
Adam, E., Mutanga, O., and Rugege, D. (2010). Multispectral and hyperspectral remote sensing for identification and mapping of wetland vegetation: a review. Wetl. Ecol. Manage. 18, 281–296. doi:10.1007/s11273-009-9169-z
Ali, A. M., Darvishzadeh, R., Skidmore, A., Gara, T. W., and Heurich, M. (2021). Machine learning methods’ performance in radiative transfer model inversion to retrieve plant traits from Sentinel-2 data of a mixed mountain forest. Int. J. Digital Earth 14, 106–120. doi:10.1080/17538947.2020.1794064
Aneece, I., and Thenkabail, P. S. (2022). New generation hyperspectral sensors DESIS and PRISMA provide improved agricultural crop classifications. Photogramm. Eng. remote Sens. 88, 715–729. doi:10.14358/PERS.22-00039R2
Angel, Y., and McCabe, M. F. (2022). Machine learning strategies for the retrieval of leaf-chlorophyll dynamics: model choice, sequential versus retraining learning, and hyperspectral predictors. Front. Plant Sci. 13, 722442. doi:10.3389/fpls.2022.722442
Annala, L., Honkavaara, E., Tuominen, S., and Pölönen, I. (2020). Chlorophyll concentration retrieval by training convolutional neural network for stochastic model of leaf optical properties (SLOP) inversion. Remote Sens. (Basel). 12, 283. doi:10.3390/rs12020283
Antonucci, G., Impollonia, G., Croci, M., Potenza, E., Marcone, A., and Amaducci, S. (2023). “Evaluating biostimulants via high-throughput field phenotyping: biophysical traits retrieval through PROSAIL inversion,” in Smart agricultural technology 3. doi:10.1016/j.atech.2022.100067
Araya, S. N., Fryjoff-Hung, A., Anderson, A., Viers, J. H., and Ghezzehei, T. A. (2021). Advances in soil moisture retrieval from multispectral remote sensing using unoccupied aircraft systems and machine learning techniques. Hydrol. Earth Syst. Sci. 25, 2739–2758. doi:10.5194/hess-25-2739-2021
Arsenault, É., and Bonn, F. (2005). Evaluation of soil erosion protective cover by crop residues using vegetation indices and spectral mixture analysis of multispectral and hyperspectral data. CATENA 62, 157–172. doi:10.1016/j.catena.2005.05.003
Asner, G. P. (1998). Biophysical and biochemical sources of variability in canopy reflectance. Remote Sens. Environ. 64, 234–253. doi:10.1016/S0034-4257(98)00014-5
Asner, G. P., and Heidebrecht, K. B. (2002). Spectral unmixing of vegetation, soil and dry carbon cover in arid regions: comparing multispectral and hyperspectral observations. Int. J. Remote Sens. 23, 3939–3958. doi:10.1080/01431160110115960
Atzberger, C., Darvishzadeh, R., Immitzer, M., Schlerf, M., Skidmore, A., and le Maire, G. (2015). Comparative analysis of different retrieval methods for mapping grassland leaf area index using airborne imaging spectroscopy. Int. J. Appl. Earth Observation Geoinformation 43, 19–31. doi:10.1016/j.jag.2015.01.009
Bailey, B. N., Ponce de León, M. A., and Krayenhoff, E. S. (2020). One-dimensional models of radiation transfer in heterogeneous canopies: a review, re-evaluation, and improved model. Geosci. Model Dev. 13, 4789–4808. doi:10.5194/gmd-13-4789-2020
Bannari, A., Morin, D., Bonn, F., and Huete, A. R. (1995). A review of vegetation indices. Remote Sens. Rev. 13, 95–120. doi:10.1080/02757259509532298
Ben-Dor, E., Chabrillat, S., Demattê, J. A. M., Taylor, G. R., Hill, J., Whiting, M. L., et al. (2009). Using Imaging Spectroscopy to study soil properties. Remote Sens. Environ. 113, S38–S55. doi:10.1016/j.rse.2008.09.019
Berger, K., Atzberger, C., Danner, M., D’Urso, G., Mauser, W., Vuolo, F., et al. (2018a). Evaluation of the PROSAIL model capabilities for future hyperspectral model environments: a review study. Remote Sens. 10, 85. doi:10.3390/rs10010085
Berger, K., Atzberger, C., Danner, M., Wocher, M., Mauser, W., and Hank, T. (2018b). Model-based optimization of spectral sampling for the retrieval of crop variables with the PROSAIL model. Remote Sens. 10, 2063. doi:10.3390/rs10122063
Berger, K., Hank, T., Halabuk, A., Rivera-Caicedo, J. P., Wocher, M., Mojses, M., et al. (2021a). Assessing non-photosynthetic cropland biomass from spaceborne hyperspectral imagery. Remote Sens. 13, 4711. doi:10.3390/rs13224711
Berger, K., Rivera Caicedo, J. P., Martino, L., Wocher, M., Hank, T., and Verrelst, J. (2021b). A survey of active learning for quantifying vegetation traits from terrestrial Earth observation data. Remote Sens. 13, 287. doi:10.3390/rs13020287
Berger, K., Verrelst, J., Féret, J.-B., Hank, T., Wocher, M., Mauser, W., et al. (2020). Retrieval of aboveground crop nitrogen content with a hybrid machine learning method. Int. J. Appl. Earth Observation Geoinformation 92, 102174. doi:10.1016/j.jag.2020.102174
Berger, K., Wang, Z., Danner, M., Wocher, M., Mauser, W., and Hank, T. (2018c). “Simulation of spaceborne hyperspectral remote sensing to assist crop nitrogen content monitoring in agricultural crops,” in Igarss 2018 - 2018 IEEE international geoscience and remote sensing symposium, 3801–3804. doi:10.1109/IGARSS.2018.8518537
Berk, A., Conforti, P., Kennett, R., Perkins, T., Hawes, F., and van den Bosch, J. (2014). “MODTRAN® 6: a major upgrade of the MODTRAN® radiative transfer code,” in 2014 6th workshop on hyperspectral image and signal processing: evolution in remote sensing (WHISPERS), 1–4. doi:10.1109/WHISPERS.2014.8077573
Bhatt, J. S., and Joshi, M. V. (2020). “Deep learning in hyperspectral unmixing: a review,” in Igarss 2020 - 2020 IEEE international geoscience and remote sensing symposium, 2189–2192. doi:10.1109/IGARSS39084.2020.9324546
Bioucas-Dias, J. M., Plaza, A., Dobigeon, N., Parente, M., Du, Q., Gader, P., et al. (2012). Hyperspectral unmixing overview: geometrical, statistical, and sparse regression-based approaches. IEEE J. Sel. Top. Appl. Earth Observations Remote Sens. 5, 354–379. doi:10.1109/JSTARS.2012.2194696
Broge, N. H., and Leblanc, E. (2001). Comparing prediction power and stability of broadband and hyperspectral vegetation indices for estimation of green leaf area index and canopy chlorophyll density. Remote Sens. Environ. 76, 156–172. doi:10.1016/S0034-4257(00)00197-8
Brown, M. E., and de Beurs, K. M. (2008). Evaluation of multi-sensor semi-arid crop season parameters based on NDVI and rainfall. Remote Sens. Environ. 112, 2261–2271. doi:10.1016/j.rse.2007.10.008
Burnett, A. C., Anderson, J., Davidson, K. J., Ely, K. S., Lamour, J., Li, Q., et al. (2021). A best-practice guide to predicting plant traits from leaf-level hyperspectral data using partial least squares regression. J. Exp. Bot. 72, 6175–6189. doi:10.1093/jxb/erab295
Caicedo, J. P. R., Verrelst, J., Munoz-Mari, J., Moreno, J., and Camps-Valls, G. (2014). Toward a semiautomatic machine learning retrieval of biophysical parameters. IEEE J. Sel. Top. Appl. Earth Obs. Remote Sens. 7, 1249–1259. doi:10.1109/JSTARS.2014.2298752
Castaldi, F., Casa, R., Pelosi, F., and Yang, H. (2015). Influence of acquisition time and resolution on wheat yield estimation at the field scale from canopy biophysical variables retrieved from SPOT satellite data. Int. J. REMOTE Sens. 36, 2438–2459. doi:10.1080/01431161.2015.1041174
Cavender-Bares, J., Schneider, F. D., Santos, M. J., Armstrong, A., Carnaval, A., Dahlin, K. M., et al. (2022). Integrating remote sensing with ecology and evolution to advance biodiversity conservation. Nat. Ecol. Evol. 6, 506–519. doi:10.1038/s41559-022-01702-5
Chabrillat, S., Ben-Dor, E., Cierniewski, J., Gomez, C., Schmid, T., and van Wesemael, B. (2019). Imaging spectroscopy for soil mapping and monitoring. Surv. Geophys 40, 361–399. doi:10.1007/s10712-019-09524-0
Chakhvashvili, E., Siegmann, B., Muller, O., Verrelst, J., Bendig, J., Kraska, T., et al. (2022). Retrieval of crop variables from proximal multispectral UAV image data using PROSAIL in maize canopy. Remote Sens. 14, 1247. doi:10.3390/rs14051247
Chen, J. M. (1996). Evaluation of vegetation indices and a modified simple ratio for boreal applications. Can. J. Remote Sens. 22, 229–242. doi:10.1080/07038992.1996.10855178
Cheng, R., Magney, T. S., Dutta, D., Bowling, D. R., Logan, B. A., Burns, S. P., et al. (2020). Decomposing reflectance spectra to track gross primary production in a subalpine evergreen forest. Biogeosciences 17, 4523–4544. doi:10.5194/bg-17-4523-2020
Croft, H., Chen, J. M., Wang, R., Mo, G., Luo, S., Luo, X., et al. (2020). The global distribution of leaf chlorophyll content. Remote Sens. Environ. 236, 111479. doi:10.1016/j.rse.2019.111479
Curcio, D., Ciraolo, G., D’Asaro, F., and Minacapilli, M. (2013). Prediction of soil texture distributions using VNIR-SWIR reflectance spectroscopy. Procedia Environ. Sci. 19, 494–503. doi:10.1016/j.proenv.2013.06.056
Dabboor, M., Atteia, G., Meshoul, S., and Alayed, W. (2023). Deep learning-based framework for soil moisture content retrieval of bare soil from satellite data. Remote Sens. (Basel). 15, 1916. doi:10.3390/rs15071916
Danner, M., Berger, K., Wocher, M., Mauser, W., and Hank, T. (2021). Efficient RTM-based training of machine learning regression algorithms to quantify biophysical and biochemical traits of agricultural crops. ISPRS J. Photogrammetry Remote Sens. 173, 278–296. doi:10.1016/j.isprsjprs.2021.01.017
Darvishzadeh, R., Atzberger, C., Skidmore, A., and Schlerf, M. (2011). Mapping grassland leaf area index with airborne hyperspectral imagery: a comparison study of statistical approaches and inversion of radiative transfer models. ISPRS J. Photogrammetry Remote Sens. 66, 894–906. doi:10.1016/j.isprsjprs.2011.09.013
Darvishzadeh, R., Matkan, A. A., and Dashti Ahangar, A. (2012). Inversion of a radiative transfer model for estimation of rice canopy chlorophyll content using a look-up-table approach. IEEE J. Sel. Top. Appl. Earth Obs. Remote Sens. 5, 1222–1230. doi:10.1109/JSTARS.2012.2186118
Darvishzadeh, R., Skidmore, A., Atzberger, C., and van Wieren, S. (2008). Estimation of vegetation LAI from hyperspectral reflectance data: effects of soil type and plant architecture. Int. J. Appl. Earth Observation Geoinformation 10, 358–373. doi:10.1016/j.jag.2008.02.005
Datta, D., Paul, M., Murshed, M., Teng, S. W., and Schmidtke, L. (2022). Soil moisture, organic carbon, and nitrogen content prediction with hyperspectral data using regression models. Sensors 22, 7998. doi:10.3390/s22207998
Daughtry, C. S. T., Walthall, C. L., Kim, M. S., de Colstoun, E. B., and McMurtrey, J. E. (2000). Estimating corn leaf chlorophyll concentration from leaf and canopy reflectance. Remote Sens. Environ. 74, 229–239. doi:10.1016/S0034-4257(00)00113-9
Dawson, T. P., Curran, P. J., and Plummer, S. E. (1998). LIBERTY—modeling the effects of leaf biochemical concentration on reflectance spectra. Remote Sens. Environ. 65, 50–60. doi:10.1016/S0034-4257(98)00007-8
de Sá, N. C., Baratchi, M., Hauser, L. T., and van Bodegom, P. (2021). Exploring the impact of noise on hybrid inversion of PROSAIL RTM on sentinel-2 data. Remote Sens. 13, 648. doi:10.3390/rs13040648
Din, M., Zheng, W., Rashid, M., Wang, S., and Shi, Z. (2017). Evaluating hyperspectral vegetation indices for leaf area index estimation of oryza sativa L. At diverse phenological stages. Front. Plant Sci. 8, 820. doi:10.3389/fpls.2017.00820
Dong, T., Liu, J., Shang, J., Qian, B., Ma, B., Kovacs, J. M., et al. (2019). Assessment of red-edge vegetation indices for crop leaf area index estimation. Remote Sens. Environ. 222, 133–143. doi:10.1016/j.rse.2018.12.032
Döpper, V., Rocha, A. D., Berger, K., Gränzig, T., Verrelst, J., Kleinschmit, B., et al. (2022). Estimating soil moisture content under grassland with hyperspectral data using radiative transfer modelling and machine learning. Int. J. Appl. Earth Observation Geoinformation 110, 102817. doi:10.1016/j.jag.2022.102817
Dorigo, W. A., Zurita-Milla, R., de Wit, A. J. W., Brazile, J., Singh, R., and Schaepman, M. E. (2007). A review on reflective remote sensing and data assimilation techniques for enhanced agroecosystem modeling. Int. J. Appl. Earth Observation Geoinformation 9, 165–193. doi:10.1016/j.jag.2006.05.003
Duan, S.-B., Li, Z.-L., Wu, H., Tang, B.-H., Ma, L., Zhao, E., et al. (2014). Inversion of the PROSAIL model to estimate leaf area index of maize, potato, and sunflower fields from unmanned aerial vehicle hyperspectral data. Int. J. Appl. Earth Observation Geoinformation 26, 12–20. doi:10.1016/j.jag.2013.05.007
Dunn, J. L., Turnbull, J. D., and Robinson, S. A. (2004). Comparison of solvent regimes for the extraction of photosynthetic pigments from leaves of higher plants. Funct. Plant Biol. 31, 195. doi:10.1071/FP03162
Effects of Ground Cover and Leaf Area on the Spectral Reflectance of Vegetation-Soil Target (n.d.). Available at: https://apps.dtic.mil/sti/citations/ADA119714 (Accessed February 13, 2023).
Eon, R. S., and Bachmann, C. M. (2021). Mapping barrier island soil moisture using a radiative transfer model of hyperspectral imagery from an unmanned aerial system. Sci. Rep. 11, 3270. doi:10.1038/s41598-021-82783-3
Eon, R. S., Goldsmith, S., Bachmann, C. M., Tyler, A. C., Lapszynski, C. S., Badura, G. P., et al. (2019). Retrieval of salt marsh above-ground biomass from high-spatial resolution hyperspectral imagery using PROSAIL. Remote Sens. 11, 1385. doi:10.3390/rs11111385
Farella, M. M., Barnes, M. L., Breshears, D. D., and Mitchell, J. (n.d.). Evaluation of vegetation indices and imaging spectroscopy to estimate foliar nitrogen across disparate biomes.
Féret, J.-B., Berger, K., de Boissieu, F., and Malenovský, Z. (2020). PROSPECT-PRO: a leaf radiative transfer model for estimation of leaf protein content and carbon-based constituents. oral. doi:10.5194/egusphere-egu2020-5251
Féret, J.-B., Gitelson, A. A., Noble, S. D., and Jacquemoud, S. (2017). PROSPECT-D: towards modeling leaf optical properties through a complete lifecycle. Remote Sens. Environ. 193, 204–215. doi:10.1016/j.rse.2017.03.004
Féret, J.-B., le Maire, G., Jay, S., Berveiller, D., Bendoula, R., Hmimina, G., et al. (2019). Estimating leaf mass per area and equivalent water thickness based on leaf optical properties: potential and limitations of physical modeling and machine learning. Remote Sens. Environ. 231, 110959. doi:10.1016/j.rse.2018.11.002
Fitzgerald, G., Rodriguez, D., and O’Leary, G. (2010). Measuring and predicting canopy nitrogen nutrition in wheat using a spectral index—the canopy chlorophyll content index (CCCI). Field Crops Res. 116, 318–324. doi:10.1016/j.fcr.2010.01.010
Fu, P., Meacham-Hensold, K., Guan, K., and Bernacchi, C. J. (2019). Hyperspectral leaf reflectance as proxy for photosynthetic capacities: an ensemble approach based on multiple machine learning algorithms. Front. Plant Sci. 10, 730. doi:10.3389/fpls.2019.00730
Gastellu-Etchegorry, J. (1996). Modeling radiative transfer in heterogeneous 3-D vegetation canopies. Remote Sens. Environ. 58, 131–156. doi:10.1016/0034-4257(95)00253-7
Ge, Y., Thomasson, J. A., and Sui, R. (2011). Remote sensing of soil properties in precision agriculture: a review. Front. Earth Sci. 5, 229–238. doi:10.1007/s11707-011-0175-0
Gehler, P. V., and Schlkopf, B. (2009). “An introduction to kernel learning algorithms,” in Kernel methods for remote sensing data analysis. Editors G. Camps-Valls, and L. Bruzzone (Chichester, UK: John Wiley and Sons, Ltd), 25–48. doi:10.1002/9780470748992.ch2
Geladi, P., and Kowalski, B. R. (1986). Partial least-squares regression: a tutorial. Anal. Chim. Acta 185, 1–17. doi:10.1016/0003-2670(86)80028-9
Gholizadeh, H., Friedman, M. S., McMillan, N. A., Hammond, W. M., Hassani, K., Sams, A. V., et al. (2022). Mapping invasive alien species in grassland ecosystems using airborne imaging spectroscopy and remotely observable vegetation functional traits. Remote Sens. Environ. 271, 112887. doi:10.1016/j.rse.2022.112887
Gitelson, A. A. (2004). Wide dynamic range vegetation index for remote quantification of biophysical characteristics of vegetation. J. Plant Physiology 161, 165–173. doi:10.1078/0176-1617-01176
Gitelson, A. A., Gritz †, Y., and Merzlyak, M. N. (2003). Relationships between leaf chlorophyll content and spectral reflectance and algorithms for non-destructive chlorophyll assessment in higher plant leaves. J. Plant Physiology 160, 271–282. doi:10.1078/0176-1617-00887
Gitelson, A. A., Keydan, G. P., and Merzlyak, M. N. (2006). Three-band model for noninvasive estimation of chlorophyll, carotenoids, and anthocyanin contents in higher plant leaves. Geophys. Res. Lett. 33, 2006GL026457. doi:10.1029/2006GL026457
Gitelson, A. A., and Merzlyak, M. N. (1998). Remote sensing of chlorophyll concentration in higher plant leaves. Adv. Space Res. 22, 689–692. doi:10.1016/S0273-1177(97)01133-2
Gitelson, A. A., Merzlyak, M. N., and Lichtenthaler, H. K. (1996). Detection of red edge position and chlorophyll content by reflectance measurements near 700 nm. J. Plant Physiology 148, 501–508. doi:10.1016/S0176-1617(96)80285-9
Govaerts, Y. M., and Verstraete, M. M. (1998). Raytran: a Monte Carlo ray-tracing model to compute light scattering in three-dimensional heterogeneous media. IEEE Trans. Geosci. Remote Sens. 36, 493–505. doi:10.1109/36.662732
Gross, N., Robson, T. M., Lavorel, S., Albert, C., Le Bagousse-Pinguet, Y., and Guillemin, R. (2008). Plant response traits mediate the effects of subalpine grasslands on soil moisture. New Phytol. 180, 652–662. doi:10.1111/j.1469-8137.2008.02577.x
Haboudane, D. (2004). Hyperspectral vegetation indices and novel algorithms for predicting green LAI of crop canopies: modeling and validation in the context of precision agriculture. Remote Sens. Environ. 90, 337–352. doi:10.1016/j.rse.2003.12.013
Haboudane, D., Miller, J. R., Tremblay, N., Zarco-Tejada, P. J., and Dextraze, L. (2002). Integrated narrow-band vegetation indices for prediction of crop chlorophyll content for application to precision agriculture. Remote Sens. Environ. 81, 416–426. doi:10.1016/S0034-4257(02)00018-4
Haboudane, D., Tremblay, N., Miller, J. R., and Vigneault, P. (2008). Remote estimation of crop chlorophyll content using spectral indices derived from hyperspectral data. IEEE Trans. Geoscience Remote Sens. 46, 423–437. doi:10.1109/TGRS.2007.904836
He, Y., Guo, X., and Wilmshurst, J. (2006). Studying mixed grassland ecosystems I: suitable hyperspectral vegetation indices. Can. J. Remote Sens. 32, 98–107. doi:10.5589/m06-009
He, Y., Yang, J., and Guo, X. (2020). Green vegetation cover dynamics in a heterogeneous grassland: spectral unmixing of landsat time series from 1999 to 2014. Remote Sens. 12, 3826. doi:10.3390/rs12223826
Heckmann, D., Schlüter, U., and Weber, A. P. M. (2017). Machine learning techniques for predicting crop photosynthetic capacity from leaf reflectance spectra. Mol. Plant 10, 878–890. doi:10.1016/j.molp.2017.04.009
Holzman, M. E., Rivas, R. E., and Bayala, M. I. (2021). Relationship between TIR and NIR-SWIR as indicator of vegetation water availability. Remote Sens. 13, 3371. doi:10.3390/rs13173371
Huang, Z., Turner, B. J., Dury, S. J., Wallis, I. R., and Foley, W. J. (2004). Estimating foliage nitrogen concentration from HYMAP data using continuum removal analysis. Remote Sens. Environ. 93, 18–29. doi:10.1016/j.rse.2004.06.008
Huete, A., Didan, K., Miura, T., Rodriguez, E. P., Gao, X., and Ferreira, L. G. (2002). Overview of the radiometric and biophysical performance of the MODIS vegetation indices. Remote Sens. Environ. 83, 195–213. doi:10.1016/S0034-4257(02)00096-2
Huete, A. R. (1988). A soil-adjusted vegetation index (SAVI). Remote Sens. Environ. 25, 295–309. doi:10.1016/0034-4257(88)90106-X
Huntjr, E., and Rock, B. (1989). Detection of changes in leaf water content using Near- and Middle-Infrared reflectances. Remote Sens. Environ. 30, 43–54. doi:10.1016/0034-4257(89)90046-1
Impollonia, G., Croci, M., Blandinières, H., Marcone, A., and Amaducci, S. (2022). Comparison of PROSAIL model inversion methods for estimating leaf chlorophyll content and LAI using UAV imagery for hemp phenotyping. Remote Sens. 14, 5801. doi:10.3390/rs14225801
Jacquemoud, S. (2000). Comparison of four radiative transfer models to simulate plant canopies reflectance direct and inverse mode. Remote Sens. Environ. 74, 471–481. doi:10.1016/S0034-4257(00)00139-5
Jacquemoud, S., and Baret, F. (1990). PROSPECT: a model of leaf optical properties spectra. Remote Sens. Environ. 34, 75–91. doi:10.1016/0034-4257(90)90100-Z
Jacquemoud, S., Verhoef, W., Baret, F., Bacour, C., Zarco-Tejada, P. J., Asner, G. P., et al. (2009). PROSPECT+SAIL models: a review of use for vegetation characterization. Remote Sens. Environ. 113, S56–S66. doi:10.1016/j.rse.2008.01.026
Jay, S., Maupas, F., Bendoula, R., and Gorretta, N. (2017). Retrieving LAI, chlorophyll and nitrogen contents in sugar beet crops from multi-angular optical remote sensing: comparison of vegetation indices and PROSAIL inversion for field phenotyping. Field Crops Res. 210, 33–46. doi:10.1016/j.fcr.2017.05.005
Ji, J., Wang, X., Ma, H., Zheng, F., Shi, Y., Cui, H., et al. (2024). Synchronous retrieval of wheat Cab and LAI from UAV remote sensing: application of the optimized estimation inversion framework. Agronomy 14, 359. doi:10.3390/agronomy14020359
Kamenova, I., and Dimitrov, P. (2021). Evaluation of Sentinel-2 vegetation indices for prediction of LAI, fAPAR and fCover of winter wheat in Bulgaria. Eur. J. Remote Sens. 54, 89–108. doi:10.1080/22797254.2020.1839359
Kattenborn, T., and Schmidtlein, S. (2019). Radiative transfer modelling reveals why canopy reflectance follows function. Sci. Rep. 9, 6541. doi:10.1038/s41598-019-43011-1
Khan, A., Vibhute, A. D., Mali, S., and Patil, C. H. (2022). A systematic review on hyperspectral imaging technology with a machine and deep learning methodology for agricultural applications. Ecol. Inf. 69, 101678. doi:10.1016/j.ecoinf.2022.101678
Kiala, Z., Odindi, J., Mutanga, O., and Peerbhay, K. (2016). Comparison of partial least squares and support vector regressions for predicting leaf area index on a tropical grassland using hyperspectral data. J. Appl. Remote Sens. 10, 036015. doi:10.1117/1.JRS.10.036015
Kim, Y. W., Kim, T., Shin, J., Lee, D. S., Park, Y. S., Kim, Y., et al. (2022). Validity evaluation of a machine-learning model for chlorophyll a retrieval using Sentinel-2 from inland and coastal waters. Ecol. Indic. 137, 108737. doi:10.1016/j.ecolind.2022.108737
Kimes, D. S., Knyazikhin, Y., Privette, J. L., Abuelgasim, A. A., and Gao, F. (2000). Inversion methods for physically-based models. Remote Sens. Rev. 18, 381–439. doi:10.1080/02757250009532396
Kothari, S., and Schweiger, A. K. (2022). Plant spectra as integrative measures of plant phenotypes. J. Ecol. 110, 2536–2554. doi:10.1111/1365-2745.13972
Lai, G., Quan, X., Yebra, M., and He, B. (2022). Model-driven estimation of closed and open shrublands live fuel moisture content. GIScience and Remote Sens. 59, 1837–1856. doi:10.1080/15481603.2022.2139404
Lakhankar, T., Ghedira, H., Temimi, M., Sengupta, M., Khanbilvardi, R., and Blake, R. (2009). Non-parametric methods for soil moisture retrieval from satellite remote sensing data. Remote Sens. 1, 3–21. doi:10.3390/rs1010003
Lázaro-Gredilla, M., Titsias, M. K., Verrelst, J., and Camps-Valls, G. (2014). Retrieval of biophysical parameters with heteroscedastic Gaussian processes. IEEE Geoscience Remote Sens. Lett. 11, 838–842. doi:10.1109/LGRS.2013.2279695
Li, Z., Jin, X., Wang, J., Yang, G., Nie, C., Xu, X., et al. (2015). Estimating winter wheat (Triticum aestivum) LAI and leaf chlorophyll content from canopy reflectance data by integrating agronomic prior knowledge with the PROSAIL model. Int. J. Remote Sens. 36, 2634–2653. doi:10.1080/01431161.2015.1041176
Li, Z., Shen, H., Weng, Q., Zhang, Y., Dou, P., and Zhang, L. (2022). Cloud and cloud shadow detection for optical satellite imagery: features, algorithms, validation, and prospects. ISPRS J. Photogrammetry Remote Sens. 188, 89–108. doi:10.1016/j.isprsjprs.2022.03.020
Lichtenthaler, H. K. (1987). “[34] Chlorophylls and carotenoids: pigments of photosynthetic biomembranes,” in Methods in enzymology (Elsevier), 350–382. doi:10.1016/0076-6879(87)48036-1
Liu, H., Jin, Y., Roche, L. M., O’Geen, A. T., and Dahlgren, R. A. (2021a). Understanding spatial variability of forage production in California grasslands: delineating climate, topography and soil controls. Environ. Res. Lett. 16, 014043. doi:10.1088/1748-9326/abc64d
Liu, Q., Jing, L., Lan, L., and Feng, J. (2021b). Using generalized regression neural network to retrieve bare surface soil moisture from radarsat-2 backscatter observations, regardless of roughness effect. Front. Earth Sci. 9. doi:10.3389/feart.2021.657206
Lobell, D. B., and Asner, G. P. (2002). Moisture effects on soil reflectance. Soil Sci. Soc. Am. J. 66, 722–727. doi:10.2136/sssaj2002.7220
Lu, B., He, Y., and Dao, P. D. (2019a). Comparing the performance of multispectral and hyperspectral images for estimating vegetation properties. IEEE J. Sel. Top. Appl. Earth Observations Remote Sens. 12, 1784–1797. doi:10.1109/JSTARS.2019.2910558
Lu, B., Proctor, C., and He, Y. (2021). Investigating different versions of PROSPECT and PROSAIL for estimating spectral and biophysical properties of photosynthetic and non-photosynthetic vegetation in mixed grasslands. GIScience and Remote Sens. 58, 354–371. doi:10.1080/15481603.2021.1877435
Lu, F., Bu, Z., and Lu, S. (2019b). Estimating chlorophyll content of leafy green vegetables from adaxial and abaxial reflectance. Sensors (Basel) 19, 4059. doi:10.3390/s19194059
Lunagaria, M. M., and Patel, H. R. (2019). Evaluation of PROSAIL inversion for retrieval of chlorophyll, leaf dry matter, leaf angle, and leaf area index of wheat using spectrodirectional measurements. Int. J. Remote Sens. 40, 8125–8145. doi:10.1080/01431161.2018.1524608
Luo, L., Chang, Q., Gao, Y., Jiang, D., and Li, F. (2022). Combining different transformations of ground hyperspectral data with unmanned aerial vehicle (UAV) images for anthocyanin estimation in tree peony leaves. Remote Sens. 14, 2271. doi:10.3390/rs14092271
Mahmoud, H. F. F. (2021). Parametric versus semi and nonparametric regression models. IJSP 10, 90. doi:10.5539/ijsp.v10n2p90
Markwell, J., Osterman, J. C., and Mitchell, J. L. (1995). Calibration of the Minolta SPAD-502 leaf chlorophyll meter. Photosynth Res. 46, 467–472. doi:10.1007/BF00032301
Mateo-Sanchis, A., Muñoz-Marí, J., Pérez-Suay, A., and Camps-Valls, G. (2018). Warped Gaussian processes in remote sensing parameter estimation and causal inference. IEEE Geoscience Remote Sens. Lett. 15, 1647–1651. doi:10.1109/LGRS.2018.2853760
Mayel, S., Jarrah, M., and Kuka, K. (2021). How does grassland management affect physical and biochemical properties of temperate grassland soils? A review study. Grass Forage Sci. 76, 215–244. doi:10.1111/gfs.12512
Miedema Brown, L., and Anand, M. (2022). Plant functional traits as measures of ecosystem service provision. Ecosphere 13. doi:10.1002/ecs2.3930
Mishra, S., and Mishra, D. R. (2012). Normalized difference chlorophyll index: a novel model for remote estimation of chlorophyll-a concentration in turbid productive waters. Remote Sens. Environ. 117, 394–406. doi:10.1016/j.rse.2011.10.016
Nandan, R., Bandaru, V., He, J., Daughtry, C., Gowda, P., and Suyker, A. E. (2022). Evaluating optical remote sensing methods for estimating leaf area index for corn and soybean. Remote Sens. (Basel). 14, 5301. doi:10.3390/rs14215301
North, P. R. J. (1996). Three-dimensional forest light interaction model using a Monte Carlo method. IEEE Trans. Geoscience Remote Sens. 34, 946–956. doi:10.1109/36.508411
Nur, N. B., and Bachmann, C. M. (2023). Comparison of soil moisture content retrieval models utilizing hyperspectral goniometer data and hyperspectral imagery from an unmanned aerial system. J. Geophys. Res. doi:10.1029/2023JG007381
Oltra-Carrió, R., Baup, F., Fabre, S., Fieuzal, R., and Briottet, X. (2015). Improvement of soil moisture retrieval from hyperspectral VNIR-SWIR data using clay content information: from laboratory to field experiments. Remote Sens. 7, 3184–3205. doi:10.3390/rs70303184
Orwin, K. H., Buckland, S. M., Johnson, D., Turner, B. L., Smart, S., Oakley, S., et al. (2010). Linkages of plant traits to soil properties and the functioning of temperate grassland: links of plant traits to soil properties. J. Ecol. 98, 1074–1083. doi:10.1111/j.1365-2745.2010.01679.x
Pascual-Venteo, A. B., Portalés, E., Berger, K., Tagliabue, G., Garcia, J. L., Pérez-Suay, A., et al. (2022). Prototyping crop traits retrieval models for CHIME: dimensionality reduction strategies applied to PRISMA data. Remote Sens. 14, 2448. doi:10.3390/rs14102448
Peppo, M. D., Taramelli, A., Boschetti, M., Mantino, A., Volpi, I., Filipponi, F., et al. (2021). Non-parametric statistical approaches for leaf area index estimation from sentinel-2 data: a multi-crop assessment. Remote Sens. (Basel). 13, 2841. doi:10.3390/rs13142841
Piegari, E., Gossn, J. I., Grings, F., Barraza Bernadas, V., Juárez, Á. B., Mateos-Naranjo, E., et al. (2021). Estimation of leaf area index and leaf chlorophyll content in Sporobolus densiflorus using hyperspectral measurements and PROSAIL model simulations. Int. J. Remote Sens. 42, 1181–1200. doi:10.1080/01431161.2020.1826058
Prudnikova, E., Savin, I., Vindeker, G., Grubina, P., Shishkonakova, E., and Sharychev, D. (2019). Influence of soil background on spectral reflectance of winter wheat crop canopy. Remote Sens. 11, 1932. doi:10.3390/rs11161932
Quast, R., Wagner, W., Bauer-Marschallinger, B., and Vreugdenhil, M. (2023). Soil moisture retrieval from Sentinel-1 using a first-order radiative transfer model—a case-study over the Po-Valley. Remote Sens. Environ. 295, 113651. doi:10.1016/j.rse.2023.113651
Rasmussen, C. E. (2004). “Gaussian processes in machine learning,” in Advanced lectures on machine learning: ML summer schools 2003, canberra, Australia, february 2 - 14, 2003, tübingen, Germany, august 4 - 16, 2003, revised lectures. Editors O. Bousquet, U. von Luxburg, and G. Rätsch (Berlin, Heidelberg: Springer), 63–71. doi:10.1007/978-3-540-28650-9_4
Rondeaux, G., Steven, M., and Baret, F. (1996). Optimization of soil-adjusted vegetation indices. Remote Sens. Environ. 55, 95–107. doi:10.1016/0034-4257(95)00186-7
Rosso, P., Nendel, C., Gilardi, N., Udroiu, C., and Chlebowski, F. (2022). Processing of remote sensing information to retrieve leaf area index in barley: a comparison of methods. Precis. Agric. 23, 1449–1472. doi:10.1007/s11119-022-09893-4
Rueda-Ayala, V., Peña, J., Höglind, M., Bengochea-Guevara, J., and Andújar, D. (2019). Comparing UAV-based technologies and RGB-D reconstruction methods for plant height and biomass monitoring on grass ley. Sensors 19, 535. doi:10.3390/s19030535
Salinero-Delgado, M., Estévez, J., Pipia, L., Belda, S., Berger, K., Paredes Gómez, V., et al. (2021). Monitoring cropland phenology on Google Earth engine using Gaussian process regression. Remote Sens. 14, 146. doi:10.3390/rs14010146
Schiefer, F., Schmidtlein, S., and Kattenborn, T. (2021). The retrieval of plant functional traits from canopy spectra through RTM-inversions and statistical models are both critically affected by plant phenology. Ecol. Indic. 121, 107062. doi:10.1016/j.ecolind.2020.107062
Sehgal, V. K., Chakraborty, D., and Sahoo, R. N. (2016). Inversion of radiative transfer model for retrieval of wheat biophysical parameters from broadband reflectance measurements. Inf. Process. Agric. 3, 107–118. doi:10.1016/j.inpa.2016.04.001
Silva-Perez, V., Molero, G., Serbin, S. P., Condon, A. G., Reynolds, M. P., Furbank, R. T., et al. (2018). Hyperspectral reflectance as a tool to measure biochemical and physiological traits in wheat. J. Exp. Bot. 69, 483–496. doi:10.1093/jxb/erx421
Sims, D. A., and Gamon, J. A. (2002). Relationships between leaf pigment content and spectral reflectance across a wide range of species, leaf structures and developmental stages. Remote Sens. Environ. 81, 337–354. doi:10.1016/S0034-4257(02)00010-X
Singh, A., and Gaurav, K. (2023). Deep learning and data fusion to estimate surface soil moisture from multi-sensor satellite images. Sci. Rep. 13, 2251. doi:10.1038/s41598-023-28939-9
Singhal, G., Bansod, B., Mathew, L., Goswami, J., Choudhury, B. U., and Raju, P. L. N. (2019). Comparison of parametric and non-parametric methods for chlorophyll estimation based on high-resolution UAV imagery. Curr. Sci. 117, 1874. doi:10.18520/cs/v117/i11/1874-1879
Sinha, S. K., Padalia, H., Dasgupta, A., Verrelst, J., and Rivera, J. P. (2020). Estimation of leaf area index using PROSAIL based LUT inversion, MLRA-GPR and empirical models: case study of tropical deciduous forest plantation, North India. Int. J. Appl. Earth Observation Geoinformation 86, 102027. doi:10.1016/j.jag.2019.102027
Sousa, C. (2022). Anthocyanins, carotenoids and chlorophylls in edible plant leaves unveiled by tandem mass spectrometry. Foods 11, 1924. doi:10.3390/foods11131924
Sripada, R. P., Heiniger, R. W., White, J. G., and Meijer, A. D. (2006). Aerial color infrared photography for determining early in-season nitrogen requirements in corn. Agron. J. 98, 968–977. doi:10.2134/agronj2005.0200
Sun, J., Wang, L., Shi, S., Li, Z., Yang, J., Gong, W., et al. (2022). Leaf pigment retrieval using the PROSAIL model: influence of uncertainty in prior canopy-structure information. Crop J. 10, 1251–1263. doi:10.1016/j.cj.2022.04.003
Sun, Q., Jiao, Q., Qian, X., Liu, L., Liu, X., and Dai, H. (2021). Improving the retrieval of crop canopy chlorophyll content using vegetation index combinations. Remote Sens. (Basel). 13, 470. doi:10.3390/rs13030470
Tagliabue, G., Boschetti, M., Bramati, G., Candiani, G., Colombo, R., Nutini, F., et al. (2022). Hybrid retrieval of crop traits from multi-temporal PRISMA hyperspectral imagery. ISPRS J. Photogrammetry Remote Sens. 187, 362–377. doi:10.1016/j.isprsjprs.2022.03.014
Tenreiro, T. R., García-Vila, M., Gómez, J. A., Jiménez-Berni, J. A., and Fereres, E. (2021). Using NDVI for the assessment of canopy cover in agricultural crops within modelling research. Comput. Electron. Agric. 182, 106038. doi:10.1016/j.compag.2021.106038
Thenkabail, P. S., Smith, R. B., and De Pauw, E. (2000). Hyperspectral vegetation indices and their relationships with agricultural crop characteristics. Remote Sens. Environ. 71, 158–182. doi:10.1016/S0034-4257(99)00067-X
Tian, J., and Philpot, W. D. (2015). Relationship between surface soil water content, evaporation rate, and water absorption band depths in SWIR reflectance spectra. Remote Sens. Environ. 169, 280–289. doi:10.1016/j.rse.2015.08.007
Tuia, D., Volpi, M., Verrelst, J., and Camps-Valls, G. (2018). “Advances in kernel machines for image classification and biophysical parameter retrieval,” in Mathematical models for remote sensing image processing. Editors G. Moser, and J. Zerubia (Cham: Springer International Publishing), 399–441. doi:10.1007/978-3-319-66330-2_10
Ustin, S. L., Gitelson, A. A., Jacquemoud, S., Schaepman, M., Asner, G. P., Gamon, J. A., et al. (2009). Retrieval of foliar information about plant pigment systems from high resolution spectroscopy. Remote Sens. Environ. 113, S67–S77. doi:10.1016/j.rse.2008.10.019
Ustin, S. L., and Jacquemoud, S. (2020). “How the optical properties of leaves modify the absorption and scattering of energy and enhance leaf functionality,” in Remote sensing of plant biodiversity. Editors J. Cavender-Bares, J. A. Gamon, and P. A. Townsend (Cham: Springer International Publishing), 349–384. doi:10.1007/978-3-030-33157-3_14
van der Tol, C., Verhoef, W., Timmermans, J., Verhoef, A., and Su, Z. (2009). An integrated model of soil-canopy spectral radiances, photosynthesis, fluorescence, temperature and energy balance. Biogeosciences 6, 3109–3129. doi:10.5194/bg-6-3109-2009
Verhoef, W. (1984). Light scattering by leaf layers with application to canopy reflectance modeling: the SAIL model. Remote Sens. Environ. 16, 125–141. doi:10.1016/0034-4257(84)90057-9
Verhoef, W., and Bach, H. (2007). Coupled soil–leaf-canopy and atmosphere radiative transfer modeling to simulate hyperspectral multi-angular surface reflectance and TOA radiance data. Remote Sens. Environ. 109, 166–182. doi:10.1016/j.rse.2006.12.013
Verhoef, W., Jia, L., Xiao, Q., and Su, Z. (2007). Unified optical-thermal four-stream radiative transfer theory for homogeneous vegetation canopies. IEEE Trans. Geosci. Remote Sens. 45, 1808–1822. doi:10.1109/TGRS.2007.895844
Verma, B., Prasad, R., Srivastava, P. K., Yadav, S. A., Singh, P., and Singh, R. (2022). Investigation of optimal vegetation indices for retrieval of leaf chlorophyll and leaf area index using enhanced learning algorithms. Comput. Electron. Agric. 192, 106581. doi:10.1016/j.compag.2021.106581
Vermote, E. F., Tanre, D., Deuze, J. L., Herman, M., and Morcette, J.-J. (1997). Second simulation of the satellite signal in the solar spectrum, 6S: an overview. IEEE Trans. Geoscience Remote Sens. 35, 675–686. doi:10.1109/36.581987
Verrelst, J., Berger, K., and Rivera-Caicedo, J. P. (2021a). Intelligent sampling for vegetation nitrogen mapping based on hybrid machine learning algorithms. IEEE Geosci. Remote Sens. Lett. 18, 2038–2042. doi:10.1109/LGRS.2020.3014676
Verrelst, J., Camps-Valls, G., Muñoz-Marí, J., Rivera, J. P., Veroustraete, F., Clevers, J. G. P. W., et al. (2015a). Optical remote sensing and the retrieval of terrestrial vegetation bio-geophysical properties – a review. ISPRS J. Photogrammetry Remote Sens. 108, 273–290. doi:10.1016/j.isprsjprs.2015.05.005
Verrelst, J., Malenovský, Z., Van der Tol, C., Camps-Valls, G., Gastellu-Etchegorry, J.-P., Lewis, P., et al. (2019a). Quantifying vegetation biophysical variables from imaging spectroscopy data: a review on retrieval methods. Surv. Geophys 40, 589–629. doi:10.1007/s10712-018-9478-y
Verrelst, J., Muñoz, J., Alonso, L., Delegido, J., Rivera, J. P., Camps-Valls, G., et al. (2012). Machine learning regression algorithms for biophysical parameter retrieval: opportunities for Sentinel-2 and -3. Remote Sens. Environ. 118, 127–139. doi:10.1016/j.rse.2011.11.002
Verrelst, J., Rivera, J. P., Gitelson, A., Delegido, J., Moreno, J., and Camps-Valls, G. (2016a). Spectral band selection for vegetation properties retrieval using Gaussian processes regression. Int. J. Appl. Earth Observation Geoinformation 52, 554–567. doi:10.1016/j.jag.2016.07.016
Verrelst, J., Rivera, J. P., Moreno, J., and Camps-Valls, G. (2013). Gaussian processes uncertainty estimates in experimental Sentinel-2 LAI and leaf chlorophyll content retrieval. ISPRS J. Photogrammetry Remote Sens. 86, 157–167. doi:10.1016/j.isprsjprs.2013.09.012
Verrelst, J., Rivera, J. P., Veroustraete, F., Muñoz-Marí, J., Camps-Valls, G., Moreno, J., et al. (2015b). Experimental Sentinel-2 LAI estimation using parametric, non-parametric and physical retrieval methods – a comparison. ISPRS J. Photogrammetry Remote Sens. 108, 260–272. doi:10.1016/j.isprsjprs.2015.04.013
Verrelst, J., Rivera-Caicedo, J. P., Reyes-Muñoz, P., Morata, M., Amin, E., Tagliabue, G., et al. (2021b). Mapping landscape canopy nitrogen content from space using PRISMA data. ISPRS J. Photogrammetry Remote Sens. 178, 382–395. doi:10.1016/j.isprsjprs.2021.06.017
Verrelst, J., Sabater, N., Rivera, J. P., Muñoz-Marí, J., Vicent, J., Camps-Valls, G., et al. (2016b). Emulation of leaf, canopy and atmosphere radiative transfer models for fast global sensitivity analysis. Remote Sens. 8, 673. doi:10.3390/rs8080673
Verrelst, J., Vicent, J., Rivera-Caicedo, J. P., Lumbierres, M., Morcillo-Pallarés, P., and Moreno, J. (2019b). Global sensitivity analysis of leaf-canopy-atmosphere RTMs: implications for biophysical variables retrieval from top-of-atmosphere radiance data. Remote Sens. 11, 1923 doi:10.3390/rs11161923
Villacrés, J., Fuentes, A., Reszka, P., and Cheein, F. A. (2023). Retrieval of vegetation indices related to leaf water content from a single index: a case study of Eucalyptus globulus (labill.) and pinus radiata. D. Don. doi:10.3390/plants10040697
Vincini, M., Frazzi, E., and D’Alessio, P. (2008). A broad-band leaf chlorophyll vegetation index at the canopy scale. Precis. Agric. 9, 303–319. doi:10.1007/s11119-008-9075-z
Wang, B., Chen, J., Ju, W., Qiu, F., Zhang, Q., Fang, M., et al. (2017). Limited effects of water absorption on reducing the accuracy of leaf nitrogen estimation. Remote Sens. 9, 291. doi:10.3390/rs9030291
Wang, J., Lopez-Lozano, R., Weiss, M., Buis, S., Li, W., Liu, S., et al. (2022a). Crop specific inversion of PROSAIL to retrieve green area index (GAI) from several decametric satellites using a Bayesian framework. Remote Sens. Environ. 278, 113085. doi:10.1016/j.rse.2022.113085
Wang, L., Chen, S., Peng, Z., Huang, J., Wang, C., Jiang, H., et al. (2021). Phenology effects on physically based estimation of paddy rice canopy traits from UAV hyperspectral imagery. Remote Sens. 13, 1792. doi:10.3390/rs13091792
Wang, L., Qu, J. J., Hao, X., and Zhu, Q. (2008). Sensitivity studies of the moisture effects on MODIS SWIR reflectance and vegetation water indices. Int. J. Remote Sens. 29, 7065–7075. doi:10.1080/01431160802226034
Wang, Z., Ma, Y., Zhang, Y., and Shang, J. (2022b). Review of remote sensing applications in grassland monitoring. Remote Sens. 14, 2903. doi:10.3390/rs14122903
Wold, S., Esbensen, K., and Geladi, P. (1987). Principal component analysis. Chemom. Intelligent Laboratory Syst. 2, 37–52. doi:10.1016/0169-7439(87)80084-9
Xie, J., Wang, C., Ma, D., Chen, R., Xie, Q., Xu, B., et al. (2022). Generating spatiotemporally continuous grassland aboveground biomass on the Tibetan plateau through PROSAIL model inversion on Google Earth engine. IEEE Trans. Geosci. Remote Sens. 60, 1–10. doi:10.1109/TGRS.2022.3227565
Xu, L., Shi, S., Gong, W., Shi, Z., Qu, F., Tang, X., et al. (2022). Improving leaf chlorophyll content estimation through constrained PROSAIL model from airborne hyperspectral and LiDAR data. Int. J. Appl. Earth Observation Geoinformation 115, 103128. doi:10.1016/j.jag.2022.103128
Xue, J., and Su, B. (2017). Significant remote sensing vegetation indices: a review of developments and applications. J. Sensors 2017, 1–17. doi:10.1155/2017/1353691
Yan, K., Zhang, Y., Tong, Y., Zeng, Y., Pu, J., Gao, S., et al. (2021). Modeling the radiation regime of a discontinuous canopy based on the stochastic radiative transport theory: modification, evaluation and validation. Remote Sens. Environ. 267, 112728. doi:10.1016/j.rse.2021.112728
Yao, X., Huang, Y., Shang, G., Zhou, C., Cheng, T., Tian, Y., et al. (2015). Evaluation of six algorithms to monitor wheat leaf nitrogen concentration. Remote Sens. 7, 14939–14966. doi:10.3390/rs71114939
Yu, X., Guo, Q., Chen, Q., and Guo, X. (2019). Discrimination of senescent vegetation cover from landsat-8 OLI imagery by spectral unmixing in the northern mixed grasslands. Can. J. Remote Sens. 45, 192–208. doi:10.1080/07038992.2019.1605586
Zeng, Y., Hao, D., Huete, A., Dechant, B., Berry, J., Chen, J. M., et al. (2022). Optical vegetation indices for monitoring terrestrial ecosystems globally. Nat. Rev. Earth Environ. 3, 477–493. doi:10.1038/s43017-022-00298-5
Zhang, Y., Huang, J., Wang, F., Blackburn, G. A., Zhang, H. K., Wang, X., et al. (2017). An extended PROSPECT: advance in the leaf optical properties model separating total chlorophylls into chlorophyll a and b. Sci. Rep. 7, 6429. doi:10.1038/s41598-017-06694-y
Zhang, Y., Migliavacca, M., Penuelas, J., and Ju, W. (2021). Advances in hyperspectral remote sensing of vegetation traits and functions. Remote Sens. Environ. 252, 112121. doi:10.1016/j.rse.2020.112121
Zhao, D., Zhen, J., Zhang, Y., Miao, J., Shen, Z., Jiang, X., et al. (2023). Mapping mangrove leaf area index (LAI) by combining remote sensing images with PROSAIL-D and XGBoost methods. REMOTE Sens. Ecol. CONSERVATION 9, 370–389. doi:10.1002/rse2.315
Zhao, T., Shi, J., Bindlish, R., Jackson, T., Cosh, M., Jiang, L., et al. (2015). Parametric exponentially correlated surface emission model for L-band passive microwave soil moisture retrieval. Phys. Chem. Earth 83-84, 65–74. doi:10.1016/j.pce.2015.04.001
Zheng, F., Wang, X., Ji, J., Ma, H., Cui, H., Shi, Y., et al. (2023). Synchronous retrieval of LAI and Cab from UAV remote sensing: development of optimal estimation inversion framework. Agronomy 13, 1119. doi:10.3390/agronomy13041119
Zotero (n.d.). Your personal research assistant. Available at: https://www.zotero.org/.
Keywords: soil properties, grassland traits, radiative transfer models (RTMs), vegetation-soil interactions, remote sensing spectroscopy, satellite remote sensing
Citation: Buma W, Abelev A and Merrick T (2024) Vegetation spectra as an integrated measure to explain underlying soil characteristics: a review of recent advances. Front. Environ. Sci. 12:1430818. doi: 10.3389/fenvs.2024.1430818
Received: 13 May 2024; Accepted: 28 August 2024;
Published: 11 September 2024.
Edited by:
Shawn Carlisle Kefauver, University of Barcelona, SpainReviewed by:
Nayani Ilangakoon, University of Colorado Boulder, United StatesCopyright © 2024 Buma, Abelev and Merrick. This is an open-access article distributed under the terms of the Creative Commons Attribution License (CC BY). The use, distribution or reproduction in other forums is permitted, provided the original author(s) and the copyright owner(s) are credited and that the original publication in this journal is cited, in accordance with accepted academic practice. No use, distribution or reproduction is permitted which does not comply with these terms.
*Correspondence: Trina Merrick, dHJpbmEubC5tZXJyaWNrLmNpdkB1cy5uYXZ5Lm1pbA==; Willibroad Buma, d2lsbGlicm9hZEBuYXZlci5jb20=
Disclaimer: All claims expressed in this article are solely those of the authors and do not necessarily represent those of their affiliated organizations, or those of the publisher, the editors and the reviewers. Any product that may be evaluated in this article or claim that may be made by its manufacturer is not guaranteed or endorsed by the publisher.
Research integrity at Frontiers
Learn more about the work of our research integrity team to safeguard the quality of each article we publish.