- 1Finance and Economics, University of Southampton, Southampton, United Kingdom
- 2School of Urban and Regional Sciences, Shanghai University of Finance and Economics, Shanghai, China
The purpose of this paper is to examine whether the development of carbon finance can promote sustainable economic growth in China through spatial spillover effects. On the basis of a theoretical analysis of this mechanism, this paper takes 30 provincial-level administrative regions in China as the research object and uses a variety of spatial econometric analysis techniques to empirically test them. It assesses sustainable economic development measured by green total factor productivity (GTFP). This study documents a significant and positive impact of province-level carbon finance improvement on sustainable economic growth through improving technological progress and increasing technological market turnover. The economic influence of carbon finance on sustainable economic growth is still positive after considering possible endogeneity concerns. Results reveal heterogeneity and spatial spillover effects of carbon finance on GTFP, notably stronger in eastern regions compared to central and western ones. We find that the technological progress and technological market turnover have significant and positive promotional effects on GTFP with increasing levels of carbon finance. This paper provides policy implications for improving sustainable economic development.
1 Introduction
“The Paris Agreement,” signed by 173 countries in 2015 and implemented in 2016, is an imperative provision for environment protection and the reduction of CO2 emissions. China, the world’s second largest economy, has taken on the role of green technological innovator and announced the goals of carbon peaking and carbon neutrality in 2021, in order to achieve a sustainable future. China has already carried out implementation plans for peaking carbon dioxide emissions in important areas and sectors, as well as a number of supporting measures, and will carry out a “1 + N” policy framework for carbon peaking and neutrality in order to meet the targets for both carbon peaking and neutrality. Meanwhile, in order to realize green technological innovation and economic development at the same time, China has launched a series of financial products, such as carbon finance, green credit, green insurance and green investment. Carbon finance, as an emerging financial instrument, aims to promote carbon emission reduction and green economic development through market mechanisms. It includes various forms such as carbon emission right trading, carbon credit trading, green bonds, etc. It helps enterprises and governments to realize economic benefits while reducing carbon footprints. Through carbon finance, it can not only provide financial support for environmental protection projects, but also incentivize enterprises to carry out technological innovation, improve energy efficiency, and reduce pollution emissions, thus promoting the overall economic development in the direction of low-carbon and sustainable development. In recent years, the carbon finance market has been developing rapidly, and the volume and value of transactions in the global carbon market have increased significantly, becoming one of the important means to address climate change. Especially in China, with the vigorous promotion of national policies, the carbon financial market has been gradually improved, and the coverage and trading activity of the carbon trading market have been increasing, providing a strong guarantee for the realization of carbon peak and carbon neutral goals. Carbon finance not only helps to achieve the goal of environmental protection, but also promotes the optimization and upgrading of the economic structure and the overall competitiveness of the economy by promoting the research and development and application of green technologies.
With the global economy standing at a crossroads, where the imperatives of growth and sustainability often seem at odds, the exploration of carbon finance mechanisms offers a promising avenue for achieving a harmonious balance. Given the important role of carbon finance in promoting the development of a green economy, many scholars have begun to study the relationship between carbon finance and economic growth and environmental protection. Many foreign scholars have used DEA index models, panel data models, time series analysis and other methods to study the correlation between energy consumption, environmental pollution and economic growth (Liddle, 2013). In this context, the theory of sustainable development and the concept of high-quality economy, which are committed to reducing ecological and environmental risks and improving the quality of economic development, have gradually matured (Jabbour et al., 2018). Studies over the past two decades have provided important information on economic sustainability, environmental protection, and green economic development (Wang et al., 2022). Wang and Su (2020) pointed out in their study that carbon finance can effectively improve green total factor productivity (GTFP) by optimizing resource allocation and promoting technological innovation. In addition, Zhang and Da, 2015 found through empirical analysis that the development of carbon finance can significantly reduce the carbon emission intensity of the industrial sector, thus promoting a win-win situation for environmental protection and economic growth. Lee and Brahmasrene (2014) emphasized the important role of the carbon finance market in promoting the diffusion of low-carbon technologies and improving the energy efficiency of enterprises. In addition, some scholars have explored the effects of carbon finance implementation in different countries and regions. Liu and Bae (2018) found that in emerging market economies, the effects of carbon finance may vary depending on the strength of policy implementation and market maturity. Not only that, the development of carbon finance is also closely related to the policy environment. Liang and Li (2013) emphasize that the government plays a key role in the development of the carbon finance market, and that the market efficiency and environmental benefits of carbon finance can be significantly enhanced through the formulation and implementation of effective environmental policies. Chen and Lee (2020) further point out that the government’s financial support and incentive measures are crucial in promoting the rapid development of the carbon finance market. In the terms of green total factor productivity (Shahbaz M et al., 2013), it is a key indicator to measure the coordinated development of resources, environment and economy of a country or region all the time (Feng and Zhang, 2017). In the past, high economic growth in China was accompanied by serious waste of resources and environmental pollution. In this context, the whole country has begun to re-examine environmental issues, and green and low-carbon economic development is imperative (Chong and Lei, 2023). The collection of climate policies in China was predominantly made up of regulatory measures and investments led by the state (Jotzo and Löschel 2014; Bumpus and Liverman, 2008) demonstrate how carbon offsets embody strategies for capital accumulation that transfer governance of the atmosphere to market forces and actors beyond national and state control. The implementation of these policies and measures has together contributed to China’s remarkable progress in green technology innovation and environmental protection.
Based on the existing literature, this paper proposes a new research methodology to deeply explore the specific impact mechanism of carbon finance on green total factor productivity through empirical analysis. Most of the previous studies stayed at the theoretical level, and little studies explored the internal mechanism of carbon finance and sustainable economic development from the empirical aspect. The novelty of our approach lies in leveraging the Romer Endogenous Economic Growth Model to quantitatively assess the impact of carbon finance on sustainable economic development. Unlike prior studies that predominantly focus on theoretical underpinnings of green finance, our investigation hones in on carbon finance’s direct and spatial effects on GTFP across 30 Chinese provinces. This geographic specificity, combined with a rigorous methodological framework, enables us to capture the dynamics of carbon finance development with high precision. Through these innovative approaches, this paper aims to explore in depth the specific role of carbon finance in enhancing green total factor productivity and to provide empirical evidence for policy makers.
My study is propelled by the hypothesis that carbon finance, through its influence on technological progress and market dynamics, plays a crucial role in enhancing GTFP. By meticulously analyzing provincial data, we aim to uncover the heterogeneous and spatially varied impacts of carbon finance, thereby offering empirical evidence to guide policy formulation and implementation. This research not only contributes to the existing body of knowledge by providing a detailed empirical analysis of carbon finance’s role in green economic efficiency but also paves the way for future studies to explore the intricate linkages between financial innovations and sustainable development. In doing so, it offers valuable insights for policymakers, financial institutions, and businesses striving to align economic objectives with environmental imperatives in the pursuit of a sustainable future.
The rest of the paper is arranged as follows. Section 2 shows recent studies on carbon finance and green total factor productivity. Section 3 describes theoretical analyses and research hypotheses through integrating the Romer Endogenous economic growth model. Section 4 shows the research design including the selection of variables, data descriptions, and the construction of relevant models. Section 5 exhibits the results of the empirical analyses. Section 6 describes research suggestions and conclusions.
2 Literature review
Carbon finance is an important field that has emerged in recent years in response to the growing problem of global climate change. However, at present, there is no clear and unified conceptual definition of carbon finance at both domestic and international levels. Generally speaking, carbon finance covers all financial transaction activities related to carbon emission reduction, including trading and investment in carbon emission rights and their derivatives, investment and financing activities for green and low-carbon energy projects, as well as related guarantee and consulting services. Its core is to promote the reduction of carbon emissions through market mechanisms, thereby promoting the development of a green economy. Since the European Climate Exchange (European Climate Ex, change, ECX) launched the futures and options on carbon emission rights in 2005, carbon emission has gradually become an important means of combating climate change and carbon emission rights have the attributes of financial products. According to foreign studies, Lohmann, 2009 explored the advantages and disadvantages of carbon market mechanisms, pointing out that although carbon trading can improve the efficiency of emission reduction, there are also the problems of market speculation and unfair distribution. Bumpus and Liverman (2008) further analyzed the operation mechanism of the carbon offset market and emphasized the role of the market in capital accumulation and global carbon governance. China, as one of the largest carbon emitters in the world, actively promotes the development of carbon finance. Zhang and Da (2015) found through empirical analysis that the development of carbon finance can significantly reduce the carbon emission intensity of the industrial sector, thus promoting a win-win situation for environmental protection and economic growth. In addition, some studies have analyzed the impact of regional differences on the effectiveness of carbon finance. Studies have shown that in emerging market economies, the effect of carbon finance varies depending on the strength of policy implementation and market maturity. For example, a study by Jiang and Ma (2019) points out that financial development significantly increases carbon emissions from a global perspective, and this effect is more pronounced in emerging markets and developing countries. Overall, existing studies have shown that carbon finance plays an important role in promoting global and regional carbon emission reduction and the development of a green economy. However, the carbon finance market still faces many challenges, such as the improvement of market mechanisms, the strength of policy support and the depth of international cooperation. Future research should pay more attention to the long-term mechanism and multi-level impacts of carbon finance, so as to provide a more solid theoretical and empirical basis for policymaking.
Green Total Factor Productivity (GTFP), as an important indicator for measuring the degree of coordination between economic development and environmental protection, has received widespread attention in academia in recent years. GTFP not only focuses on the input and output efficiencies of traditional factors of production, but also incorporates consideration of the utilization of environmental resources and ecological damages, so as to reflect the sustainability of economic activities in a more comprehensive manner. The definition of Green Total Factor Productivity (GTFP) is mainly based on the traditional Total Factor Productivity (TFP) with the inclusion of environmental factors to make the productivity measurement more environmentally friendly. Methods such as Data Envelopment Analysis (DEA) and Stochastic Frontier Analysis (SFA) are generally used to measure GTFP. Chung et al. (1997) proposed a DEA model with environmental variables for calculating green productivity. This approach is widely used to measure GTFP across countries or regions. On the basis of the gradual improvement and maturity of theories related to high-quality economy, more and more scholars have begun to pay attention to the realization path. For example, in relevant foreign studies, some scholars believe that ecological and environmental pollution is the main factor that reduces the quality of the economy, so industrial transformation and environmental protection are the key to improving economic quality (Shafik, 1994). Some scholars also point out that engineering quality education, human resources and technological innovation are important factors affecting the high quality of the economy (Dobrenel et al., 2016). Meanwhile, technological innovation is also a key driver of GTFP enhancement, and studies have shown that green technology innovation can significantly improve resource utilization efficiency and reduce pollution emissions (Zhou et al., 2010; Guo et al., 2024 use the generalized Luenberger productivity index and its parameter decomposition method to study the green total factor productivity (GTFP) of Chinese cities. Through empirical analysis of Chinese cities, the study finds that technological progress and environmental protection measures are the main drivers for enhancing urban GTFP. The study also points out that there are significant differences in GTFP between cities, with economically developed regions having significantly higher GTFP than less developed regions. In addition, some scholars believe that finance, as the core of the market economy, can promote the transformation of industrial structure and improve the quality of economic development by optimizing resource allocation. In addition, the optimization of industrial structure, such as the transformation to low-pollution and high-value-added industries, also helps to improve GTFP (Wang et al., 2019). Environmental policies, on the other hand, play an important guiding role by incentivizing firms to adopt environmentally friendly technologies (Zhang et al., 2017). A large number of empirical studies have shown that there are significant differences in GTFP across countries and regions. Ouyang and Sun, 2015 studied the green efficiency of Chinese cities and showed that the strictness of environmental policies has a significant impact on the improvement of GTFP.
Although the impact of carbon finance on green total factor productivity (GTFP) has attracted academic attention, there are still several shortcomings and gaps in existing research. First, most of the existing studies stay at the theoretical level and lack empirical analysis to reveal in depth the specific mechanism of action between carbon finance and GTFP. Second, early academic research focused more on green finance, while carbon finance was only regarded as an indicator for constructing the green finance index, and there was a lack of research on the independent impact of carbon finance. In addition, the existing studies less consider the spatial heterogeneity of carbon finance development and fail to reveal the differences in the impact of carbon finance on GTFP in different regions. Finally, the specific path of how carbon finance can enhance GTFP through technological progress and increased trading volume in the technology market is unclear. By introducing the Romer endogenous economic growth model, this thesis provides an in-depth empirical discussion on the specific impact mechanism of carbon finance on green total factor productivity. Unlike previous studies that mainly focus on theoretical discussions, this study takes 30 provincial-level administrative regions in China as the research object, and uses spatial econometric models to empirically analyze the relationship between carbon finance and GTFP, especially the spatial heterogeneity of carbon finance development. It is found that carbon finance significantly contributes to the enhancement of GTFP through technological progress and the increase in the volume of technology market transactions, and this effect is particularly significant in eastern China. Through these innovative approaches, this thesis aims to deeply reveal the specific role of carbon finance in enhancing green total factor productivity and provide empirical evidence for policy formulation.
3 Theoretical basis and hypotheses
To evaluate the impact mechanisms and characteristics of sustainable economic development and carbon finance, this study introduces another key factor called natural capital, which includes natural resource consumption and pollution discharge (Guo and Tan, 2023). Natural capital is the sum of natural resource consumption and pollution emissions, where energy consumption is calculated by converting coal, electricity, oil, and gas consumption into “10,000 tons of standard coal” and pollution emission is calculated by emissions of sulfur dioxide (SO2) and nitrogen oxides (NOx) (tons/year). Technological advances increase the efficiency of resource utilization and reduce resource consumption per unit of output. Examples include energy efficient technologies, new energy technologies and circular economy models. The development of advanced pollution control technologies, such as wastewater treatment technologies and air pollutant filtering technologies, can reduce pollution emissions and improve environmental quality (Maria et al., 2017). A high level of human capital helps drive technological innovation and the development of new environmentally friendly technologies and processes, further reducing dependence on natural capital. Economic growth is usually accompanied by increased resource consumption and rising pollution emissions, but it may also be possible to mitigate this effect through increased environmental protection investment and technological research and development. By adjusting the industrial structure to reduce the share of highly polluting and energy-consuming industries and increase the share of green and service industries, a win-win situation for both economic growth and environmental protection can be realized. The link between carbon finance and green total factor productivity is then examined in this article using the deduction and inference of the Romer Model (Chandra, 2022).
3.1 Final production department
The final production department is composed of several independent companies but is viewed as an integrated company. When this department is combined with labor, human resources, natural capital investment, and a few intermediate capital products, it produces final goods.
The Romer endogenous economic growth model (Chandra, 2022) provides a framework for the significant influences of technological advancement and knowledge production on economic development. This framework can be succinctly expressed by the endogenous economic growth production function as follows:
where 0 < α, β, η < 1, Y is the total output; H, L, E respectively represent human capital, labor force and natural capital input. Natural capital input includes natural resource consumption N and pollution emission O and is an increase function of natural resource consumption and a decrease function of pollution emissions; xi is the ith special inter-mediate product purchased; A represents the total number of intermediate product types developed by the R&D department and produced by the intermediate Product Department. Technological progress is expressed in the form of an increase in the number of specialized intermediate product category A. It is assumed that the R&D department can achieve technological innovation, and then the final production department combines the newest technology with the intermediate product A to produce the final product. The production and scale of A require readjustment of the level of technology and knowledge stock. As mentioned in the previous paragraph, E in the production function includes natural resource consumption and pollution emissions, so A can also reflect the level of green total factor productivity.
When the final product price is set to 1, the profit function of the final product producer can be expressed as follows:
where πY represents the profit of the final product sector, WH represents the wages of R&D personnel, WL represents the wages of labor personnel, PE represents the price of natural capital, and Pxi represents the price of the ith intermediate product. Assume that in Equation 2 the final product manufacturer is in perfect competition, and WH, WL, PE and Pxi are given, the demand function of the intermediate product can be obtained by the first-order condition of maximizing the profit of the manufacturer:
3.2 Intermediate product department
It is assumed that the intermediate production department is in a monopolistic competitive market, and at the same time, the products produced by any company in the intermediate production department cannot be replaced by other companies. There are countless such intermediate manufacturers between [0, A].
Suppose that the intermediate product manufacturer needs to make use of the new technology of the R&D department and invest in the final product to produce the intermediate product and lease the intermediate product to the final production department. The production of 1 unit of the intermediate product needs to invest μ units of final products, and the application cost of new technologies is k times of the consumed final products, the profit function of intermediate products is as follows:
where πi is the profit of the ith intermediate product, and the first-order conditional combination Equation 3 maximized by Equation 4 gives the demand function of the intermediate good:
Substituting Equation 5 into Equation 3, the price of the intermediate product can be obtained:
Substituting Equation 6 into Equation 4, the maximum profit of intermediate manufacturers is:
Let
whether or not an intermediate product producer adopts a new technology for production in Equation 8 depends on the revenue Vt of the products they produce, which is equal to the discounted value of the intermediate product’s profit, i.e.,:
in Equation 9, where r is the deposit rate. From the capital market no-arbitrage principle, it can be expressed as:
where
3.3 R&D department
Two factors are introduced into the production function of Equation 1 above, including natural resource consumption and pollution emission, and Equation 7 shows that the profits of the intermediate production sector are directly related to natural re-source consumption and pollution emission. Therefore, as Lei et al., 2023 states, whether the intermediate production department adopts the new technology provided by the R&D department should consider the constraints of the environment and re-sources. It is assumed that the R&D department will take green technology innovation into account when making technological progress. If the R&D capacity of the R&D department depends on the available technical knowledge and the amount of human capital invested, then the production function of the R&D department is as follows:
in Equation 11, where HA represents the human capital invested in the R&D department, A represents the stock of existing technical knowledge, and
In the process of developing a new product, the R&D department is faced with two stages of choice. In the first phase, the R&D department should decide whether or not to invent. The R&D department will continue to invent new technology when the expected profit from the invention of new technology is greater than the R&D cost. In the second phase, the R&D department should determine the optimal price for the inter-mediate goods department. Assume that the R&D department is in a perfectly competitive market, the patent price is set as PA, and all new technologies of the R&D department belong to patents. Combined with Equation 7, the price function of new technologies of the R&D department is as follows:
Equation 12 indicates that the price of R&D patents is related to natural resource consumption and pollution emissions, so the R&D department first considers “green” technological innovation in the technological innovation process.
3.4 Introduce new products into carbon finance
Suppose that the initial capital for establishing an intermediate goods manufacturing enterprise consists of two parts. The first part is start-up costs, and the second part is the initial stock of technical knowledge. The more technical knowledge the enterprise has at the beginning, the better the production technology base of the enterprise, and can reduce the search cost of the enterprise. Therefore, the investment function of establishing an intermediate product company is set in Equation 13 as follows:
where M0 represents start-up cost, including the purchase of patents, materials, plants, and hired human capital, and A represents the existing stock of technical knowledge.
Suppose that a newly created intermediate goods company has no spare capital for production and needs to organize production through financing. Considering that China’s financial system is dominated by commercial banks, and the production costs of enterprises are all provided by banks, then assume that the unit cost of corporate financing is rl, and the discounted value of interest paid by external financing is:
where r is the deposit interest rate. Since the profits of the intermediate goods sector are constrained by natural capital, it is assumed that the new enterprises will carry out green technological innovation. In addition, due to the implementation of the carbon emission reduction policy, commercial banks will support carbon emission reduction, the utilization of resources in universities and the rapid marketization of green technologies and will give enterprises a “green carbon loan interest rate,” which is lower than the market loan interest rate, that is, loans to enterprises that meet the carbon emission reduction project. This rate is closely related to the level of development of carbon finance. The higher the emphasis on carbon finance, the lower the interest rate. The level of carbon finance is expressed in ξ, assuming that:
According to Equation 15,
since the intermediate product market is monopolized in Equation 16, each intermediate product company usually has a unique intermediate product, so only when
By differentiating both sides of Equation 17 with respect to time, we get:
From Equations 10, 17, 18:
where gA represents the growth rate of A.
Take the derivative of Equation 19 with respect to ξ:
Equation 20 shows that the higher the level of carbon finance development, the more conducive to the growth of green GTFP. It can be seen that under financing constraints, investment in green technology R&D will affect scientific research output, thus hindering the improvement of green TFP. Carbon finance loans take corporate carbon emission reduction projects into consideration, provide finance support for projects and companies that meet the standards, and then affect the decision-making effect and technological progress capacity of companies. After the capital threshold of intermediate product enterprises to enter the market is lowered, enterprises compete to buy the patented technology of the research and development department to form a monopoly position, so the marketization cycle of scientific research achievements is shortened, and the research and development rate and quantity of the research and development department are greatly increased, thus accelerating the speed of technology upgrading and application, and improving the GTFP.
The paper of Jiang et al., 2023 explores the relationship between carbon finance and high-quality economic development through the evidence from China. The evidence shows that carbon finance has a spatial spillover effect on economic development. Zhou et al., 2023 studies how green finance and technological progress promote total factor productivity. Zeng et al., 2023 analyses the impact path that green finance influences green total factor productivity, including technology transaction market and financial development. According to the research of prior researchers and based on the results and analyses of these deductions and inferences above, this paper proposes hypotheses below and carries out empirical analyses.
H1: The development of carbon finance influences the level of green total factor productivity to promote sustainable economic development.
H2a: The development of carbon finance improves the level of green total factor productivity through technological progress.
H2b: The development of carbon finance improves the level of green total factor productivity through the increase in technology market turnover.
H3: Carbon finance has a spatial spillover effect on green total factor productivity, which can not only improve the green total factor productivity of the local region, but also affect the green total factor productivity of neighboring regions.
4 Research design
4.1 Variables and data description
4.1.1 Dependent variable
The dependent variable of this paper is green total factor productivity (GTFP). Green total factor productivity not only takes into account the inputs and outputs of traditional factors of production (e.g., labor and capital), but also incorporates the impact of environmental factors and resource consumption, providing a comprehensive picture of the efficiency of the green economy. The SBM-super efficiency model addresses the problem that the SBM model is unsolved in the case of multiple valid decision units and is widely used in the measurement of GTFP.
This paper uses SBM—Malmquist to measure the GTFP of 30 provinces in the case of variable returns to scale.
The input variables and output variables of the measurement of green total factor productivity are as follows:
Supposing that three production factors are devoted in production, including labor, capital and energy. This paper uses the total employed population of the region at the end of the year of 30 provinces in China to present labor input. Energy consumption is calculated by converting coal, electricity, oil, and gas consumption into “10,000 tons of standard coal.” Zhang Jun (2004)’s perpetual inventory method is adopted for the estimation of capital stock, as follows:
where i is provincial areas, t is years from 2010 to 2020, k is physical capital stock, I is gross capital formation for the year, δ is fixed asset depreciation rate. In Equation 21, the fixed asset investment price index is converted to constant prices in 2004 for each year; The depreciation rate is set to 9.6% with reference to Zhang et al., 2024; and the capital stock in the base period is calculated using the total investment in fixed assets.
Both of acceptable and unpleasant output are output variables. Prior to that, desired production is translated into GDP at constant 2004 prices. Wastewater discharge, sulfur dioxide discharge, dust discharge, and soot discharge are examples of undesired output. The National Bureau of Statistics of China and the statistics yearbooks of several Chinese provinces and cities are where the data is sourced from.
4.1.2 Independent variable
Carbon finance is an important driver of the green economy transition by promoting the reduction of carbon emissions by enterprises and individuals through economic incentives and promoting the innovation and application of green technologies. Studying its mechanism of action will help to understand the path of green economy transition. Referring to Chen et al., 2024, this paper selects the development of carbon finance as an independent variable and uses the ratio of carbon dioxide emissions (10,000 tons) to the loan balance (100 million RMB), in other words, the intensity of carbon dioxide emission loans, to measure the level of carbon finance development.
4.1.3 Controls variables
To reduce the influence of omitted variables on this research, this paper refers to Zhou et al., 2020 for the selection of control variables, including human capital level, foreign direct investment, the degree of industrialization, the degree of opening-up, government support, and industrial structure upgrading. The human capital level is measured by the average school year. Foreign direct investment is measured by the relationship between the actual utilization of FDI and provincial GDP (Zhou et al., 2019). The degree of industrialization is measured by the relationship between industrial value added and provincial GDP (Li et al., 2023). The degree of opening-up is measured by the ratio of the total import and export volumes of the province in the current period to the total GDP of the province in the current period. Government support is measured by the ratio of government spending to provincial GDP. Industrial structure upgrading is measured by the ratio of the third industrial sector to provincial GDP. The data comes from NBS, the China Statistic Yearbook, the China Employment Statistic Yearbook, the China Industry Statistic Yearbook, and so on. Aver school year function is as follows:
in Equation 22, P is the number of people at each level of education.
4.1.4 Mediator variables
According to Geng et al., 2023 methodology, labor productivity—or the ratio of the regional GDP to all employment—is used to gauge the advancement of technology. As technological progress is an important means of raising total factor productivity. Through innovation and the introduction of new technologies, enterprises can utilize resources more efficiently and increase the efficiency of output, thereby promoting economic growth. In addition, advances in green technologies can help reduce polluting emissions in the production process. For example, the application of clean energy technologies can reduce reliance on fossil fuels, thereby reducing emissions of carbon dioxide and other harmful gases. Meanwhile, carbon finance provides financial support for enterprises’ green technology R&D, reduces the financing cost of green technology innovation, and incentivizes enterprises to carry out green technology R&D and innovation. Many countries and regions encourage technological innovation, especially the R&D and application of green technologies through policies. Carbon finance, as one of the policy tools, directly promotes the progress of green technology by providing preferential loans and financing support. The China Publication and Employment Statistics Yearbook and the China Statistic Yearbook are the sources of the information. The circulation space for technical advancements and the total of their trade linkages make up the technology market turnover. The development of carbon finance has contributed to the dynamism of the green technology market. Through the provision of green loans and financial support, more enterprises have been able to participate in the research, development and trading of green technologies, increasing the volume of transactions and the speed of transformation in the technology market. This accelerated market turnover not only promotes the widespread application of green technologies, but also enhances the green total factor productivity (GTFP) of the entire economy through the technology diffusion effect. The China Statistic Yearbook is where the data is sourced.
4.1.5 Data description
Panel data from 30 Chinese provinces from 2010 to 2020 is used for empirical studies. The China Insurance Yearbook which provides basic statistics on the economy, population, environment, etc. for each province, the China Publication Statistic Yearbook, the Almanac of China’s Finance and Banking, the Wind economic database, the China Employment statistics Yearbook which provides data on employment in each province, which is used to measure labor input, the China Industrial Statistics Yearbook which provides data on industrial output value and other data for each province to measure the degree of industrialization, and CSMAR are the primary sources for the original data for all variables. The study uses panel data from 2010 to 2020, and while it helps to control for fixed effects, autocorrelation and trend effects in time-series data can lead to endogeneity problems. This paper uses lagged variables and differencing to mitigate this problem. The data for this study came from several different statistical yearbooks and databases, and the collection process was complex. In this study, a few missing data points are filled up using linear interpolation and the near mean. The variables that are used in the baseline regression are summarized in Table 1.
4.2 Model selection and construction
4.2.1 Benchmark model construction
Referring to Twum et al., (2022), considering that the green total factor productivity data measured by the ultra-efficient SBM model has the characteristics of left union at 0, if OLS is used, parameter estimation bias will be caused, so the Tobit model is used to analyze the benchmark relationship between green finance and green total factor productivity. The model function is as follows:
in Equation 23, gftp presents green total factor productivity, cfi presents the development of carbon finance, controls presents control variables, including human capital level, foreign direct investment, the degree of opening-up, the degree of industrialization (Yang and Hao, 2023), government support, and industrial structure upgrading, i presents 30 provinces in China between 2010 and 2020, t presents years from 2010 to 2020, uit presents individual error terms, and εit presents random error terms.
4.2.2 Mediation effect model construction
This paper mainly explores the impact mechanisms and characteristics of carbon finance on green total factor productivity, adding technology progress and the increase in technology market turnover as mediator variables.
By combing through previous studies, it can be seen that most scholars test the existence of the mediation effect by testing the regression coefficient step by step. MacKinnon et al., 2002; Fairchild and Fritz, 2007, as well as Hayes, 2009 argue that when the mediating effect is not strong, the test power using the stepwise test coefficient is also not strong. MacKinnon et al., 2002 conducted a simulation study on this, and the results showed that the test method proposed by Sobel, 1987 was more accurate and stronger in testing the mediating effect. To investigate the impact mechanisms and characteristics of carbon finance on green total factor productivity, this paper uses a causal stepwise regression test to build a mediation effect model builds model (5) and model (6) on the basis of the main model (4):
In addition, in order to ensure the validity of the results, the Sobel test is applied on the basis of the stepwise analysis method. In Equations 24, 25, Med presents mediator variable, including technological progress and the increase in technology market turnover; i presents 30 Chinese provinces except Tibet, Hong Kong, Macao, and Taiwan; and t presents years between 2010 and 2020.
The path is as follows in Figure 1:
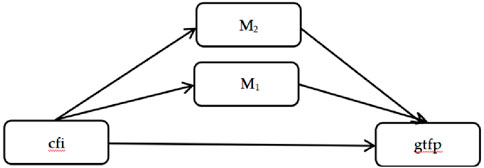
Figure 1. where M1 is technological progress; M2 is the increase in technology market turnover. There are two paths.
4.2.3 Spatial auto-correlation test
4.2.3.1 Spatial weighting matrix
To further inspect the spatial effects of carbon finance on green total factor productivity, we should first examine whether there are spatial effects between carbon finance and GTFP. Referring to the study of Ren, 2020 this paper uses the difference between the per capita GDP of the two provinces from 2010 to 2020 to make a matrix of economic distance weights. The function of the matrix of economic distance weights is as follows:
In Equation 26, Weco is is the matrix of economic distance weights, and pgdp is the mean of the per capita GDP of 30 provinces from 2010 to 2020.
4.2.3.2 Spatial correlation tests
This section uses Moran’s I (Anselin, 1988) to inspect the global spatial correlations of cfi and GTFP and uses the Moran scatterplots to inspect the local spatial correlations of cfi and GTFP. The value of Moran’s I is generally [−1,1]. If Moran’s I is > 0, it indicates a positive spatial correlation; if Moran’s I is < 0, it indicates a negative spatial correlation. Moran scatterplots are two-dimensional scatterplots of Wz (displaying the standardized variable’s spatial lag values), W (representing the spatial weight matrix), and z (representing standardized observations).
4.2.4 Spatial econometric model construction
According to theoretically analyze, the level of carbon finance development not only has influences on the development of GTFP but also has spatial spillover effects on the development of GTFP in surrounding provinces to a certain extent, so by building three spatial econometric models and conducting a series of hypotheses, tests and empirical analyses, this paper selects the optimal spatial econometric model for research. In order to solve the estimation bias problem of spatial econometric models due to the left-connected feature, this paper combines the Tobit model and the spatial econometric models.
In equation 27 and 28, ρ, θ1, θ2 are spatial term coefficients; this model is a traditional OLS model when ρ = θ1 = θ2 = 0; this model is SDM when λ = 0; this model is SAR when θ1 = θ2 = 0; this model is SEM when ρ = 0, θ1 = θ2 = 0.
5 Empirical analysis results
5.1 The features of CFI and GTFP
5.1.1 The features of carbon finance
This paper takes the level of carbon finance development (the ratio of carbon di-oxide emissions to the loan balance) as an independent variable, measures the strength of carbon finance development level by carbon loan intensity, and uses 30 provinces in China from 2010 to 2020 as observation samples. From the perspective of time, according to Figure 2, except for a few provinces in the northwest, the level of carbon finance development in most provinces has significantly improved, among which the increases in Guangdong Province, Zhejiang Province, Shanghai Municipality, and Beijing Municipality are most pronounced. From the perspective of region, according to Figure 2, the level of carbon finance in China is uneven, among which Beijing, Shanghai, Zhejiang, Guangdong, Sichuan, Fujian, and Chongqing are developing well, while the level of carbon finance in the northwest is generally lagging, such as Inner Mongolia, Ningxia, and Xinjiang. In China, the level of carbon finance is unbalanced in different regions.
5.1.2 The characteristics of GTFP
In order to compare the differences and trends of GTFP in each province and region, GTFP in 2010, 2012, 2014, 2016, 2018, and 2020 is represented by Figure 3. In the time dimension, the GTFP of most provinces in China has improved, and the provinces with the most significant improvement are Hebei, Chongqing, Shandong, Liaoning, Shanghai, Guizhou, Beijing, Tianjin, Jiangsu, etc. In the regional dimension, China’s GTFP has significant regional differences, with a higher GTFP in Jiangsu, Beijing, Tianjin, Shanghai, etc., and a lower GTFP in Xinjiang, Shaanxi, and Gansu. In China, there is a significant imbalance in green total factor productivity at the provincial and regional levels.
5.2 The influence of carbon finance on GTFP under static panel model
5.2.1 Benchmark regression analysis
Conduct empirical analysis of the connection between carbon finance and GTFP through the Tobit regression model (Table 2). First, the positive impact of the development of carbon finance on green total factor productivity passes the significance test at the level of 1%; the regression coefficient is 0.1554. This means GTFP increases by 0.1554 for every unit of carbon finance added and verifies the hypothesis H1. Second, positive relationships exist between control variables and dependent variable, including human capital level, the degree of opening-up, and industrial structure upgrading; however, a negative relationship exists between foreign direct investment and GTFP.
5.2.2 Mediating effects analysis
According to the benchmark regression results above, the development of carbon finance significantly promotes GTFP. To explore the mechanisms through which carbon finance affects GTFP, this paper adopts a causal stepwise regression test to construct a mediating effect model for analyses Lee and Lee (2022). On the one hand, columns 1 and 2 in Table 2 show that carbon finance coefficients are significant, and the regression results (column 3) express that the coefficients of technological progress and carbon finance are all significant. The coefficients of carbon finance reduce from 0.1554 to 0.0965, which means a partial mediating effect of technological progress exists in the relationship between carbon finance and GTFP. That is, the effect path of “carbon finance—technology progress—GTFP” is valid, which verifies the hypothesis H2a. On the other hand, the coefficient of carbon finance passes the significance test in column 4, and the coefficients of carbon finance and technology market turnover are both significantly positive in column 5. The coefficients of carbon finance reduce from 0.1554 to 0.0136, which means that a partial mediating effect of technology market turnover also exists in the relationship between the level of carbon finance development and GTFP. That is, the effect path of “carbon finance—the increase in technology market turnover—GTFP” is valid, which verifies the hypothesis H2b.
In summary, technological progress and technology market turnover can be seen as mediator variables and exist in a positive impact relationship in the influence of carbon finance on GTFP, accounting for 12.53% and 12.20%, respectively. While the Sobel test results show that the mediating effect is significant, indicating that carbon finance affects GTFP through two paths: technological progress and technology market turnover.
5.2.3 Endogeneity testing
In order to avoid the endogeneity problem caused by the reverse causal relationship, referring to the research of Xingjian and Zhou, (2018), the product of the first phase of carbon finance lag (L.cfi) and the first-order difference of carbon finance (D.cfi) was selected as the instrumental variable (L.cfi×D.cfi) for 2SLS regression, the results are shown in Table 3, and it can be seen that there is no weak instrumental variable problem in the selected tool variables, indicating that the selected tool variables are effective. After considering the endogeneity problem, the results are shown in Table 3, and carbon finance still shows a significant positive effect on the green total factor productivity.
5.3 The spatial effects of carbon finance on GTFP
5.3.1 Spatial auto-correlation test
Calculate Moran’s I of carbon finance and GTFP in 30 provinces in China from 2010 to 2020 and make Moran’s I trend plots over time (Figure 4) and Moran scatterplots (Figure 5). Conduct a spatial correlation test for carbon finance and GTFP under the economic distance weight matrix (Table 3). Moran’s I of carbon finance and GTFP both passes the significant test and shows positive correlation in all years from 2010 to 2020, which means carbon finance and GTFP have positive spatial agglomeration effects on spatial weight distribution. Figures 4, 5 indicate that both carbon finance and GTFP are spatially correlated; therefore, this paper builds a spatial econometric model and use it for testing.
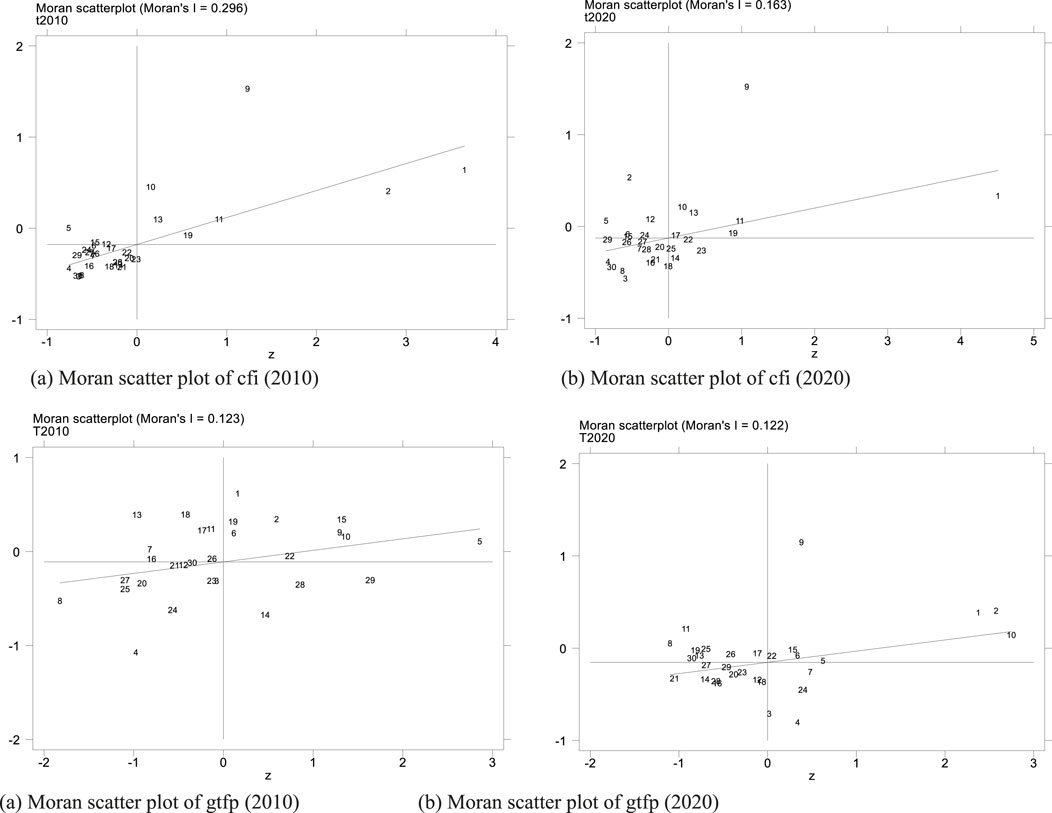
Figure 5. Moran scatterplots of cfi and gtfp in 2010 and 2020. (A) Moran scatter plot of gtfp (2010) (B) Moran scatter plot of gtfp (2020)
5.3.2 Econometric model selection
This paper conducts the LM test, LR test, Wald test, and Hausman test under the spatial weights of the economic distance matrix (Table 4) so as to select the optimal spatial econometric model for testing Yang et al., 2023. According to the testing results, the LM test rejects the null hypotheses at a significant level of 1% when the economic distance weight matrix is used. In other words, the Spatial Durbin Model is suitable for this study; the LR test and the Wald test both reject the null hypotheses, which means the Spatial Durbin Model is more suitable for the research than SAR and SEM. The Hausman test rejects the null hypothesis that a random effect is better than a fixed effect under the economic distance weight matrix. Therefore, this paper reports the regression results of the SDM of fixed individual effects when using the economic distance matrix.
5.3.3 The empirical analysis of spatial effects
After using SDM to conduct the test, spatial auto-regression coefficient rho passes the significant test and are all positive under economic distance weight matrix in Table 5, which means GTFP exists a significant positive spatial correlation effect and shows obvious spatial agglomeration characteristics in China.
According to the SDM, first, when other relevant variables known to influence GTFP, such as human capital level and foreign direct investment, have been appropriately controlled, in Table 6, for every 1% increase in the level of carbon finance, GTFP increases by 0.0879, which once again shows that the level of carbon finance significantly increases regional GTFP. Second, the spatial lag term of SDM investigates the relationship between the level of carbon finance and GTFP. The results of coefficients show that the level of carbon finance passes the significance test at the level of 1%, indicating that the development of carbon finance has a positive spatial spillover effect. The development of carbon finance in neighboring regions can improve the GTFP of the region, and the cross-regional flow of financial resources can significantly raise the GTFP in the adjacent region. But the results show that carbon finance has different spatial spillover effect, what is the reason for it? Firstly, an important feature of carbon finance is its cross-regional flow of capital and technology. This means that financial resources and carbon financial products from developed regions can flow into relatively underdeveloped regions through market mechanisms, thus promoting GTFP enhancement in these regions. As the economy and financial markets in the eastern region are more developed, carbon financial resources are more likely to flow across regions and generate spillover effects. The statistical results show that for every 1% increase in the level of carbon finance, there is a significant increase in GTFP by 0.0879% (p < 0.01), which indicates that the enhancement of GTFP by carbon finance is statistically significant. Meanwhile, the development of carbon finance promotes the R&D and application of green technologies. When enterprises in a certain region obtain carbon financial support to carry out technological innovation, these new technologies can be diffused to neighboring regions through the market, leading to the enhancement of the overall GTFP in the region. According to the results of the Spatial Durbin Model (SDM), the coefficient of the spatial lag term is 0.204 (p < 0.05), indicating that carbon finance also has a significant positive effect on GTFP in neighboring regions. Secondly, the eastern region is economically developed, with very strong inter-regional economic ties and factor mobility. This efficient mobility makes the positive effects of carbon finance not only limited to a single region, but also able to quickly spread to the whole region through the market mechanism, driving up the GTFP of neighboring regions. The SDM results show that the direct effect coefficient of carbon finance on GTFP in the eastern region is 0.122 (p < 0.01), the indirect effect coefficient is 0.253 (p < 0.01), and the total effect coefficient is 0.375 (p < 0.01). In contrast, the central and western regions have a lower level of economic development and poorer mobility of financial resources and technology. Therefore, the positive effects of carbon finance spread slower in these regions, and the spatial spillover effect is not as significant as in the eastern region. The direct effect coefficient in the central region is −0.161 (p < 0.01), indicating that carbon finance in the central region has a limited effect on the enhancement of GTFP. Thirdly, the eastern region is endowed with sound infrastructure, high-quality talents and a favorable financial environment, which are all important factors in enhancing GTFP. The development of carbon finance in this region can lead to efficient green technology innovation and industrial upgrading, further enhancing its demonstration and driving role in the country. The central and western regions are geographically remote, with backward infrastructure and low levels of economic development, resulting in a lack of attraction for carbon finance and green technologies. Although carbon finance policies have been implemented to some extent in these regions, their effect on GTFP enhancement is limited due to the weak economic foundation. The results verify H3. Third, the coefficients of the degree of opening-up, industrial structure upgrading, and foreign direct investment are negative, indicating that the degree of opening-up, industrial structure upgrading, and foreign direct investment result in the reduction of GTFP. The improvements in the degree of opening-up, industrial structure upgrading ignore the environmental quality in the surrounding regions and are not conducive to the improvement of GTFP in the short term. However, the improvements in human capital level, the degree of industrialization, and government support lead to the improvement of GTFP because GTFP is a total factor productivity that considers energy and environmental problems and is a key indicator reflecting economic growth under the playground of energy conservation and emission reduction. The upgrading of regional industrialization drives the improvement of GTFP in neighboring areas, and government capital investment and policy support for environmental protection and effective utilization of resources also have positive influences on GTFP in surrounding areas.
5.3.4 Heterogeneity analysis of spatial effects
In view of the regional heterogeneity of carbon finance and GTFP in China, this paper continues to verify whether there is spatial heterogeneity in the impact of carbon finance development on GTFPs.
First, as far as the eastern region is concerned (Table 7), GTFP has significant positive spatial correlation effects under the economic distance weight matrix. The GTFP in eastern regions has spatial agglomeration characteristics. The level of carbon finance has significant positive influences and positive spatial spillover effects on GTFP. The direct effect and indirect effect of GTFP pass the significance test at the level of 1%, and the indirect effect is greater than the direct effect. Second, as far as the central region is concerned (Table 8), although the influence of carbon finance on GTFP passes significance test, the spatial auto-regression coefficient rho is not significant under economic distance weight matrix. Third, as far as the western region is concerned (Table 9), the main effect, spatial effect, and effect decomposition of carbon finance on GTFP are all not significant under the economic distance weight matrix.
In summary, there is a regional heterogeneity in the impact of carbon finance on GTFP, with the reason for the large differences in the eastern, central, and western regions as follows:
First, the eastern region provides strong external conditions for the improvement of GTFP with its abundant carbon finance products, perfect carbon finance system, and good finance environment when compared with the central and western regions; Car-bon finance and GTFP represent obvious spatial effects because of close economic ties and a high-efficient flow of financial factors among eastern regions; With a better development platform and perfect infrastructure, the eastern region gathers a large number of compound talents, who can increase the nation’s capacity for technological innovation generally, draw clean industries with “low pollution and high output,” and raise the GTFP standard. Second, compared with the eastern region that has the most pronounced spatial spillover effects, the implementation effect of carbon finance policies in the western region has a certain lag; Financial resources and carbon financial products are still in the process of continuous improvement; the flow efficiency of financial factors is relatively low; and the spatial effects of carbon finance and GTFP performance are weak. The remote geographical location and the small scale of eco-nomic development lead to excessive reliance on high-energy-consuming industries with low input-output efficiency and an unreasonable energy consumption structure, which has a certain negative impact on the development of GTFP.
5.4 Robustness test
To further investigate the reliability of the effects of carbon finance on GTFP in the Tobit model and spatial econometric model, this paper uses the four approaches below to conduct a robustness test (Table 10). First, drawing on the practices of Zhang et al., 2018, rebuild the key independent variable. Using the ratio of CO2 emission to provincial GDP to measure the level of carbon finance, the regression results are still congruent. Second, add a control variable. Coal is the main energy consumption, high input and low output increase the carbon dioxide emission in China and have negative influences on the development of GTFP. The regression results are consistent with the analyses in the previous paragraph after adding total energy consumption as a control variable. Third, change the spatial weight matrix. Considering that the impact of the level of carbon finance on GTFP may be different under different weight matrices, this paper replaces the economic distance weight matrix with an in-verse distance square matrix to explore the spatial effects of carbon finance on GTFP. The results show that the sign of the local effect is consistent with the previous paragraph and the significance and signs of direct, indirect, and total effects are consistent with the previous results. In summary, the research findings in this paper are fairly robust.
6 Conclusions and policy implications
This article draws the following conclusions, by analyzing the impact mechanisms of carbon finance on GTFP, building the variable index system of the level of carbon finance and GTFP, using the Tobit model and spatial econometric model based on economic distance weight matrix, and empirically analyzing the influence effects of carbon finance on GTFP and empirically mediating effects of technological progress and technology market turnover. The current study mainly focuses on 30 provincial-level administrative regions in China for empirical analysis. Future studies can consider extending this analysis method to other countries or regions to verify whether the impact of carbon finance on green total factor productivity (GTFP) is generalizable. For example, countries with different levels of economic development can be selected for comparative analysis to understand the performance of carbon finance in different economic environments. Extending the study to other countries and regions, several representative countries can be selected, including developed countries, emerging economies and developing countries, so that the similarities and differences in carbon finance implementation and GTFP enhancement in different economies can be compared. Firstly, some developed countries can be chosen, such as the United States, Germany and Japan, which have relatively mature carbon finance markets and high levels of environmental protection policies and technological innovations, and the study of these countries can help to understand the mechanism of the impact of carbon finance on GTFP under high levels of economic development. Secondly, some emerging economies can be selected, such as Brazil, India and South Africa, which are developing rapidly and their carbon financial markets are still in the growth stage, and the study of these countries can reveal the role and effect of carbon finance in the process of economic transformation. Finally, some developing countries, such as Nigeria, Bangladesh and Kenya, can be selected, these countries have a relatively weak economic foundation and relatively insufficient environmental protection technology and financial support, and the study of these countries can provide valuable experience on carbon finance to promote GTFP under the condition of limited resources. In the terms of variables, which could influence GTFP, research in future could take policy factors, economic factors, social factors, and environmental factors into consideration, such as environmental protection policies in different regions, carbon emission standards, economic growth rate, urbanization level, availability of natural resources, and level of environmental pollution. Meanwhile, in the future, researchers could also further refine the indicators of technological progress and consider the specific impact of different types of technological innovations, such as clean energy technologies and energy efficiency technologies, on GTFP.
Firstly, by analyzing in detail the data of 30 provincial-level administrative regions in China between 2010 and 2020, this paper finds that the development of carbon finance has shown a continuous and steady upward trend. This trend reflects the growing emphasis on and positive response to the goals of a low-carbon economy and sustainable development in each region. Green Total Factor Productivity (GTFP) also shows a significant increase during this period, indicating a continuous improvement in the efficiency of the utilization of environmental resources in economic activities, along with a gradual reduction in pollution emissions. There are obvious differences in the development level of carbon finance and GTFP between different regions. Specifically, the level of carbon finance and GTFP in the eastern coastal regions is significantly higher than that in the central and western regions due to the higher level of economic development, more mature financial markets and the implementation of environmental protection policies. This regional difference reflects the differences in geographic location, economic foundation, policy implementation and technological innovation capacity, which enables the eastern region to more effectively utilize carbon finance tools to promote the development of green economy. In contrast, the development of carbon finance in the central and western regions has been relatively slow due to the relatively lagging economic development, weak infrastructure and technological innovation capacity, resulting in a relatively limited increase in GTFP as well. Therefore, in the process of promoting the development of national carbon finance, we should focus on narrowing the regional gap and formulating differentiated policies for the specific conditions of different regions, so as to comprehensively enhance the level of green development in each region.
Secondly, the results of empirical analysis clearly show that the level of development of carbon finance has a significant role in promoting green total factor productivity (GTFP). As the government and financial institutions continue to promote green finance and carbon finance policies, these policies not only help to reduce carbon emissions and environmental pollution, but also effectively promote the coordinated development of economic technology and the environment. Specifically, carbon finance affects GTFP in a number of ways: first, it provides enterprises with the financial support they need to carry out green technology R&D and innovation, and incentivizes them to invest in new technologies, processes and clean energy, thereby achieving technological progress. These technological advances not only improve resource utilization efficiency, but also reduce pollution emissions in the production process. Second, carbon finance promotes the widespread application and diffusion of green technologies by increasing the volume of transactions in the technology market. With the support of carbon finance, enterprises are not only able to develop more green technologies, but also quickly spread these technologies to other enterprises and industries through the technology market, forming a technology diffusion effect and further enhancing the GTFP of the whole society. The mediation effect analysis further reveals the specific mechanism of carbon finance on GTFP. The study shows that carbon finance has an indirect effect on GTFP through the two mediating variables of promoting technological progress and increasing technology market turnover. This means that carbon finance not only directly improves the green technology R&D capability of enterprises, but also enhances the transformation and application effect of technology achievements by activating the technology market. This finding emphasizes the important role of technological progress and technology market in the relationship between carbon finance and GTFP, indicating that carbon finance policies should pay more attention to the support and improvement of technological innovation and market mechanisms in promoting green economic development.
Thirdly, by analyzing the spatial econometric model using the economic distance weight matrix, we find that the mechanism of carbon finance’s impact on green total factor productivity (GTFP) has a significant spatial spillover effect. This means that the development of carbon finance not only enhances GTFP in the region, but also positively affects neighboring regions through cross-regional capital and technology flows. This finding suggests that carbon finance plays an important role in promoting interregional synergistic development and optimal resource allocation.
Fourthly, the analysis of regional heterogeneity in spatial effects reveals that there are significant differences in the improvement effect of carbon finance on green total factor productivity (GTFP) in different regions. Specifically, the development of carbon finance shows significant positive impacts in the more economically developed eastern regions, while its impacts are relatively limited in the relatively economically backward central and western regions. This regional heterogeneity reflects the differences in infrastructure, technological innovation capacity and financial market maturity across regions, leading to significant differences in the effects of carbon finance across regions. In the eastern region, due to the better economic foundation, more mature financial market and stronger government support for carbon finance, these regions not only have perfect infrastructure and strong technological innovation ability, but also have sufficient capital liquidity and favorable market environment. These advantages make carbon finance policies in the eastern region can be quickly implemented and play a significant effect. Enterprises in the eastern region can make better use of carbon finance tools to invest in technological innovation and green projects, thus significantly increasing GTFP. meanwhile, due to the high density and frequency of economic activities in the eastern region, the technological advances and market effects brought by carbon finance can also be spread to neighboring regions more quickly, forming a strong spatial spillover effect. However, in the central and western regions, these regions face more challenges in attracting carbon finance resources and implementing carbon finance policies due to the relative lag in economic development, relatively weak infrastructure development and technological innovation capacity, and less mature financial markets. Although the government has also launched a series of carbon finance policies and support measures in the central and western regions, due to the lack of sufficient economic and technological foundations, the effect of carbon finance on the enhancement of GTFP in these regions is not significant. In addition, the low capital mobility and relatively imperfect market mechanism in the central and western regions also limit the spatial spillover effect of carbon finance. This makes it difficult for these regions to realize significant green economic benefits through carbon finance as in the eastern region.
Finally, based on the conclusions of this study, the following suggestions are put forward on how to achieve the improvement of sustainable economic development.
First, accelerate the innovation of carbon financial products, improve the carbon finance evaluation mechanism, and continue to empower green emerging industries. Continuously promote the reform of the financial system, consider incorporating carbon finance assessment into the macroprudential assessment framework, and guide financial institutions to continuously innovate carbon financial products and services. Encourage environmental protection enterprises to go public and raise funds, launch carbon financial products, introduce more social funds into green production, increase the empowerment of carbon finance to emerging industries and green environmental protection industries, and achieve constant improvement of the green environment and sustainable economic development. The following actions could be taken into consideration. Set up a special carbon fund to attract social capital to invest in low-carbon projects. The carbon fund should mainly invest in carbon emission reduction technologies, carbon capture and storage (CCS) projects, and low-carbon infrastructure construction. Promote the listing of environmental enterprises in the stock market to broaden their financing channels. The government should provide counseling and support for environmental enterprises to be listed, help them meet the listing conditions, and simplify the listing approval process. Develop loan products specifically targeting carbon emission reduction projects and provide enterprises with low-interest rate and long-cycle financial support. Carbon credit should prioritize support for energy-saving renovation, clean energy development and other projects with significant carbon emission reduction benefits. Promote the development of a carbon credit trading market that allows enterprises to buy and sell carbon credits to achieve their carbon emission targets. The carbon credit trading market should have a transparent and fair-trading mechanism to protect the rights and interests of market participants. From the perspective of the government, establish a green project subsidy fund to provide financial subsidies to eligible green projects and reduce investment costs for enterprises. The subsidies should focus on supporting new energy development, pollution control, energy-saving renovation and other areas. Publish a green investment guide to clarify the criteria for recognizing green projects and the direction of investment and guide the flow of social capital to projects that are in line with the national environmental protection strategy. The Government should regularly assess and update the guidelines to ensure that they are in line with the latest environmental policies and technological developments. Carbon finance cases should be diverted to different types of courts, and many environmental resources trial judges who are proficient in carbon finance-related businesses should be trained. Carbon finance cases that seriously endanger the ecological and environmental protection should be referred to the Environmental Resources Tribunal for trial and environ-mental public interest litigation cases related to the carbon finance case should be heard together.
Second, accelerate green technological innovation and continue to promote industrial transformation and upgrading. Lower the criteria for financial institutions to enter the carbon market, allow financial institutions to enter the carbon market to trade, and clarify carbon assets as qualified low collateral, and financial institutions can trade carbon assets on behalf of customers in the future. Guide financial institutions to increase financial support for projects with strong innovation and high technology content, promote enterprises to carry out substantive green technology innovation, and achieve coordinated improvement of enterprise economic, environmental, social, and economic benefits. For example, establish a special green technology innovation fund focused on supporting research and development of technologies with high environmental benefits, such as renewable energy technologies, energy-saving technologies and pollution control technologies. The fund should cover all stages from basic research to applied development and provide long-term stable financial support. Establish a national or regional-level green technology promotion platform to centralize the display of the latest green technologies and solutions. The platform should provide technology docking services to help enterprises find suitable technology providers and facilitate technology transfer and cooperation. Regular green technology exchanges, seminars and training courses should be organized, and experts, scholars and industry leaders should be invited to share the latest technological achievements and application experience, to enhance enterprises’ knowledge of green technologies and their application capacity. Energy audits and diagnostics are conducted for high energy-consuming enterprises to identify inefficient energy utilization and technological bottlenecks, and detailed energy-saving reform proposals are provided to enterprises. The Government can encourage enterprises to conduct energy audits through subsidies or tax incentives. Encourage the use of green technology to solve environmental problems in economic development, accelerate the green transformation of industries, encourage the development of clean, low-carbon, and environmental-friendly industries, transform and upgrade the traditional industries of the “three highs”, reduce pollution emissions and improve energy efficiency.
Third, give full play to the demonstration and diffusion effects of the eastern region, improve the GTFPs of the central and western regions, and achieve green development and economic growth for mutual benefit and win-win results. Establish several green technology demonstration zones in economically developed regions in the east to centralize the demonstration and application of the latest green technologies. These demonstration zones should cover a wide range of fields, such as renewable energy, energy conservation and environmental protection, and new materials, and be equipped with advanced infrastructure and technical support services. Governments in the eastern region should formulate specialized support policies, such as tax incentives, financing support and subsidies for technological research and development, to attract more enterprises to move into the demonstration zones and to promote the clustering and development of green technologies and industries. Local governments should carefully consider each region’s geographic location and economic development characteristics when forming pertinent policies because the impact of carbon finance on GTFP has a spatial spillover effect that is still present in the eastern region but is less obvious in the central and western regions and promote the coordinated development of various regions through differentiated policies and measures to avoid homogeneous competition. Relying on its advantages in the economy, geographical location, human capital, technological innovation level, and technological market turnover, the eastern region should actively play a leading role in improving the GTFP of the region and neighboring regions. For western and central areas, A special fund for green development in the central and western regions should be established, focusing on supporting green technology research and development, energy-saving and emission reduction projects and ecological restoration projects. The government should reduce the financing costs of enterprises through subsidies and low-interest loans to encourage them to make green investments. Increase investment in green infrastructure in the central and western regions, improve energy, transportation, and environmental protection facilities, and create a favorable environment for green development. Focus will be placed on the construction of clean energy power stations, smart grids, green transportation networks and other infrastructure projects. Meanwhile, through the cooperation platform, advanced enterprises and research institutions in the eastern region can provide technology transfer, joint research and development and financial support to the central and western regions, helping them to rapidly upgrade their green technologies. Governments should encourage and support such cross-regional technological and financial cooperation.
Forth, relevant regulatory authorities should develop their understanding of the carbon finance market and cooperate with multiple parties to promote the development of the carbon finance market. Regulators can strengthen the construction of relevant personnel and improve the approval and supervision capacity of carbon finance market business, for example, set up carbon finance training bases in key universities or research institutions to provide long-term professional training courses and cultivate high-quality carbon finance talents. The training bases should be equipped with comprehensive teaching facilities and internship platforms and provide a training mode that combines theory and practice; To create a favorable external environment for the growth and innovation of the carbon finance market, carbon futures trading will be implemented gradually in the early stages of the development of the national carbon market. Additionally, carbon financial derivatives will be innovated and scaled based on carbon futures. Encourage financial institutions and research institutes to design more innovative carbon financial derivatives, such as carbon index futures, carbon bond options, etc., to enrich the market product line and enhance the depth and breadth of the market. Select several representative enterprises and regions to carry out pilot projects on carbon financial derivatives, summarize the experience and gradually promote them. The government should provide policy support and financial subsidies to reduce the trial-and-error costs of pilot enterprises. This requires the Ministry of Ecology and Environment to cooperate and communicate with financial regulators such as the “One Bank and Two Sessions” and coordinate with multiple parties to delineate their respective regulatory responsibilities and coordinate supervision to standardize and reduce the risk of the development of the carbon finance market.
Data availability statement
The original contributions presented in the study are included in the article/supplementary material, further inquiries can be directed to the corresponding author.
Author contributions
YC: Conceptualization, Data curation, Formal Analysis, Funding acquisition, Investigation, Methodology, Project administration, Resources, Software, Supervision, Validation, Visualization, Writing–original draft. GM: Data curation, Formal Analysis, Methodology, Software, Writing–review and editing.
Funding
The author(s) declare that no financial support was received for the research, authorship, and/or publication of this article.
Conflict of interest
The author declares that the research was conducted in the absence of any commercial or financial relationships that could be construed as a potential conflict of interest.
Publisher’s note
All claims expressed in this article are solely those of the authors and do not necessarily represent those of their affiliated organizations, or those of the publisher, the editors and the reviewers. Any product that may be evaluated in this article, or claim that may be made by its manufacturer, is not guaranteed or endorsed by the publisher.
References
Bumpus, A. G., and Liverman, M. D. (2008). Accumulation by decarbonization and the governance of carbon offsets. Econ. Geogr. 84, 127–155. doi:10.1111/j.1944-8287.2008.tb00401.x
Chandra, R. (2022). “Paul romer and modern endogenous growth theory,” in Endogenous growth in historical perspective. Palgrave studies in economic history (Cham: Palgrave Macmillan).
Chen, F., Zeng, X., and Guo, X. (2024). Green finance, climate change, and green innovation: evidence from China. Finance Res. Lett. 63, 105283. doi:10.1016/j.frl.2024.105283
Chen, Y., and Lee, C. C. (2020). Does technological innovation reduce CO2 emissions? Cross-country evidence. J. Clean. Prod. 263, 121550. doi:10.1016/j.jclepro.2020.121550
Chong, W., and Lei, W. (2023). Green credit and industrial green total factor productivity: the impact mechanism and threshold effect tests. J. Environ. Manag. 331, 117266. doi:10.1016/j.jenvman.2023.117266
Chung, Y. H., Färe, R., and Grosskopf, S. (1997). Productivity and undesirable outputs: a directional distance function approach. J. Environ. Manag. 51, 229–240. doi:10.1006/jema.1997.0146
Dobrenel, T., Caldana, C., Hanson, J., Robaglia, C., Vincentz, M., Veit, B., et al. (2016). TOR signaling and nutrient sensing. Annu. Rev. Plant Biol. 67, 261–285. doi:10.1146/annurev-arplant-043014-114648
Fairchild, A. J., and Fritz, M. S. (2007). Mediation analysis. Annu. Rev. Psychol. 58, 593–614. doi:10.1146/annurev.psych.58.110405.085542
Feng, J., and Zhang, S. Q. (2017). The measurement of China’s provincial green total factor productivity based on DEA method: does the choice of DEA model make difference. Acta Sci. Nat. Univ. Pekin. 53, 151–159. doi:10.13209/j.0479-8023.2016.119
Geng, L., Hu, J., and Shen, W. (2023). The impact of carbon finance on energy consumption structure: evidence from China. Environ. Sci. Pollut. Res. 30, 30107–30121. doi:10.1007/s11356-022-24303-y
Guo, B., Yu, H., and Jin, G. (2024). Urban green total factor productivity in China: a generalized Luenberger productivity indicator and its parametric decomposition. Sustain. Cities Soc. 106, 105365. doi:10.1016/j.scs.2024.105365
Guo, L., and Tan, W. (2023). Relationship between green credit and high-quality development of green and green economy—“pull effect” or “capricorn effect”. Environ. Dev. Sustain. doi:10.1007/s10668-023-03736-6
Hayes, A. F. (2009). Beyond baron and kenny: statistical mediation analysis in the new millennium. Commun. Monogr. 76, 408–420. doi:10.1080/03637750903310360
Jabbour, C. J. C., Jugend, D., Jabbour, A. B. L. de S., Govindan, K., Kannan, D., and Leal Filho, W. (2018). “There is no carnival without samba”: revealing barriers hampering biodiversity-based R&D and eco-design in Brazil. Reveal. barriers hampering biodiversity-based R& D eco-design Braz. 206, 236–245. doi:10.1016/j.jenvman.2017.10.019
Jiang, C., and Ma, X. (2019). The impact of financial development on carbon emissions: a global perspective. Sustainability 11, 5241. doi:10.3390/su11195241
Jiang, L., Niu, H., Ru, Y., Tong, A., and Wang, Y. (2023). Can carbon finance promote high-quality economic development: evidence from China. Heliyon 9, 22698. doi:10.1016/j.heliyon.2023.e22698
Jotzo, F., and Löschel, A. (2014). Emissions trading in China: emerging experiences and international lessons. Energy Policy 75, 3–8. doi:10.1016/j.enpol.2014.09.019
Lee, C. C., and Brahmasrene, T. I. C. T. (2014). ICT, CO2Emissions and economic growth: evidence from a panel of ASEAN. Glob. Econ. Rev. 43, 93–109. doi:10.1080/1226508x.2014.917803
Lee, C. C., and Lee, C. C. (2022). How does green finance affect green total factor productivity? Evidence from China. Energy Econ. 107, 105863. doi:10.1016/j.eneco.2022.105863
Lei, T., Luo, X., Jiang, J., and Zou, K. (2023). Emission reduction effect of digital finance: evidence from China. Environ. Sci. Pollut. Res. 30, 62032–62050. doi:10.1007/s11356-023-26424-4
Li, G., Jia, X., Khan, A. A., Khan, S. U., Ali, M. A. S., and Luo, J. (2023). Does green finance promote agricultural green total factor productivity? Considering green credit, green investment, green securities, and carbon finance in China. Environ. Sci. Pollut. Res. 30 (13), 36663–36679. doi:10.1007/s11356-022-24857-x
Liang, Q., and Li, Y. (2013). Carbon finance and the carbon market in China: progress and challenges. Energy Policy 60, 234–240.
Liddle, B. (2013). Population, affluence, and environmental impact across development: evidence from panel cointegration modeling. Environ. Model. and Softw. 40, 255–266. doi:10.1016/j.envsoft.2012.10.002
Liu, Y., and Bae, J. (2018). Urbanization and industrialization impact of CO2 emissions in China. J. Clean. Prod. 172, 178–186. doi:10.1016/j.jclepro.2017.10.156
Lohmann, L. (2009). Toward a different debate in environmental accounting: the cases of carbon and cost–benefit. Account. Organ. Soc. 34, 499–534. doi:10.1016/j.aos.2008.03.002
Mackinnon, D. P., Lockwood, C. M., Hoffman, J. M., West, S. G., and Sheets, V. (2002). A comparison of methods to test mediation and other intervening variable effects. Psychol. Methods 7, 83–104. doi:10.1037/1082-989x.7.1.83
Maria, A., Gustavo, C., and Canavire Bacarreza, G. (2017). Does Financial development promote innovation in developing economies?An empirical analysis. Rev. Dev. Eco-nomics 21, 475–496. doi:10.1111/rode.12314
Ouyang, X., and Sun, C. (2015). Energy savings potential in China's industrial sector: from the perspectives of factor price distortion and allocative inefficiency. Energy Econ. 48, 117–126. doi:10.1016/j.eneco.2014.11.020
Ren, Y. (2020). Intelligent statistical analysis on the influence of industrial agglomeration on innovation efficiency by spatial econometric model. J. Intelligent and Fuzzy Syst. 39, 4881–4890. doi:10.3233/jifs-179974
Shafik, N. Economic Development and Environmental Quality: An Econometric Analysis (1994). Oxf. Econ. Pap. 46, 757–773.
Shahbaz, M., Solarin, S. A., Mahmood, H., and Arouri, M. (2013). Does financial development reduce CO2 emissions in Malaysian economy? A time series analysis. Econ. Model. 35, 145–152. doi:10.1016/j.econmod.2013.06.037
Sobel, M. E. (1987). Direct and indirect effects in linear structural equation models. Sociol. Methods and Res. 16, 155–176. doi:10.1177/0049124187016001006
Twum, A., ZhongMing, T., Agyemang, A., and Ayamba, E. C. (2019). The impact of internal and external factors of credit risk on businesses: An empirical study of Chinese commercial banks. J. Corp. Account. Finan. 32 (6). doi:10.1002/jcaf.22482
Wang, Q., and Su, M. (2020). The effects of urbanization and industrialization on decoupling economic growth from carbon emission – a case study of China. Sustain. Cities Soc. 51, 101758. doi:10.1016/j.scs.2019.101758
Wang, X., Muhammad, U. J., Sobia, B., Hamna, Y., Jan, A., and A. S, A. (2022). Interplay among institutional actors for sustainable economic development—role of green policies, ecoprenuership, and green technological innovation. Front. Environ. Sci. 10. doi:10.3389/fenvs.2022.956824
Wang, Y., Li, L., Kubota, J., Han, R., and Zhu, X. (2019). Is decoupling economic growth from fuel consumption possible in developing countries? – a comparison of China and India. J. Clean. Prod. 229, 806–817. doi:10.1016/j.jclepro.2019.04.403
Xingjian, Y., and Zhou, L. (2019). Does digital financial inclusion significantly influence household consumption? evidence from household survey data in China. Journal of Financial Research 461 (11), 47–67.
Yang, Y., Chen, W. H., and Qian, H. H. Effects of agricultural technical efficiency on agricultural carbon emission: based on spatial spillover effect and threshold effect analysis. Chin. J. Eco-Agriculture, 2023, 31, 226–240. doi:10.12357/cjea.20220571
Yang, Y., and Hao, F. (2023). Does the carbon emission rights trading pilot policy aggravate local government fiscal pressure? Evidence from China. Environ. Sci. Pollut. Res. 30, 65217–65236. doi:10.1007/s11356-023-26914-5
Zeng, S., Fu, Q., Haleem, F., Shen, Y., and Zhang, J. (2023). Carbon-reduction, green finance, and high-quality economic development: a case of China. Sustainability 15, 13999. doi:10.3390/su151813999
Zhang, M., Li, C., Zhang, J., and Chen, H. (2024). How green finance affects green total factor productivity—evidence from China. Sustainability 16, 270. doi:10.3390/su16010270
Zhang, S., Zhu, D., Shi, Q., and Cheng, M. (2018). Which countries are more ecologically efficient in improving human well-being? An application of the Index of Ecological Well-being Performance. Resour. Conservation Recycl. 129, 112–119. doi:10.1016/j.resconrec.2017.10.015
Zhang, Y. J., and Da, Y. B. (2015). The decomposition of energy-related carbon emission and its decoupling with economic growth in China. Renew. Sustain. Energy Rev. 41, 1255–1266. doi:10.1016/j.rser.2014.09.021
Zhang, Y. J., Peng, Y. L., Ma, C. Q., and Shen, B. (2017). Can environmental innovation facilitate carbon emissions reduction? Evidence from China. Energy Policy 100, 18–28. doi:10.1016/j.enpol.2016.10.005
Zhou, P., Ang, B. W., and Han, J. Y. (2010). Total factor carbon emission performance: a Malmquist index analysis. Energy Econ. 32, 194–201. doi:10.1016/j.eneco.2009.10.003
Zhou, X., Tang, X., and Zhang, R. (2020). Impact of green finance on economic development and environmental quality: a study based on provincial panel data from China. Environ. Sci. Pollut. Res. 27, 19915–19932. doi:10.1007/s11356-020-08383-2
Zhou, Y., Chen, G., Huang, L., Lin, Z., Jin, J., and Lu, F. (2023). Empirical analysis of the impact of green finance and technological progress on total factor productivity in China's industrial sector. Proc. Bus. Econ. Stud. 6, 39–44. doi:10.26689/pbes.v6i6.5732
Keywords: green total factor productivity, spatial durbin model, sustainable economic growth, mediating effects, spatial spillover effects, carbon finance
Citation: Chen Y and Ma G (2024) The characteristics and mechanisms of carbon finance development on green economic efficiency: an empirical analysis based on endogenous economic growth model. Front. Environ. Sci. 12:1420708. doi: 10.3389/fenvs.2024.1420708
Received: 21 April 2024; Accepted: 13 August 2024;
Published: 30 August 2024.
Edited by:
Mobeen Ur Rehman, Keele University, United KingdomReviewed by:
Gui Jin, China University of Geosciences Wuhan, ChinaAiliang Xie, Linyi University, China
Copyright © 2024 Chen and Ma. This is an open-access article distributed under the terms of the Creative Commons Attribution License (CC BY). The use, distribution or reproduction in other forums is permitted, provided the original author(s) and the copyright owner(s) are credited and that the original publication in this journal is cited, in accordance with accepted academic practice. No use, distribution or reproduction is permitted which does not comply with these terms.
*Correspondence: Yiru Chen, eWM1Nm4yM0Bzb3Rvbi5hYy51aw==; Guangcheng Ma, Z3VhbmdjaGVuZzNAc290b24uYWMudWs=