- College of Economics and Management, Nanjing Agricultural University, Nanjing, China
With the increasing demand for crops, the excessive application of fertilizers has gradually become a significant factor affecting water quality. Therefore, studying the relationship between agricultural fertilizer runoff and water quality is crucial for the sustainable development of agricultural production. The present study sets up a new dynamic model of nitrogen fertilizer loss, introducing variables such as precipitation, fertilization application during dry and rainy seasons, and their lagged and interaction terms. The paper analyzed the issue of fertilizer runoff under the complex interactions of various factors, including spatial and temporal scales and climatic conditions, and explored the relationship between agricultural activities and water quality changes in the context of sustainable development. Due to Hainan Island’s independent river system, which is free from transboundary pollution, and its low level of industrial pollution, it provides an excellent sample for assessing the impact of agricultural non-point source pollution on water quality. Based on watershed monitoring data from Hainan Island, this study draws the following conclusions: 1. Precipitation exhibits a pronounced seasonal influence on total nitrogen concentration. 2. Nitrogen fertilizer enters into water bodies through precipitation, resulting in a lag effect on total nitrogen concentration. 3. The influence of grain and tropical crops on nitrogen loss is less significant, while cash crops will trigger the nitrogen overloading of rivers in scenarios where they account for a high proportion of the planting structure of the sown area.
1 Introduction
With the continuous growth of the global population, the demand for food is rising. Fertilizers are widely used as an important tool for improving crop yields. However, the utilization rate of fertilizers is relatively low in many countries due to outdated technologies and inappropriate usage strategies. China, for example, is a major consumer of fertilizers, accounting for 32% of the world’s fertilizer usage (Zhang et al., 2010). However, the utilization rates of nitrogen and phosphorus fertilizers for rice production in China are only between 40% and 65% and 15% and 25%, respectively (Wang et al., 2018). The inefficient long-term utilization of fertilizer leads to the discharge of a large number of nutrients, such as nitrogen and phosphorus, into water bodies through pathways of runoff and leaching. According to data from “The Bulletin of the Second National Pollution Source Census” (Ministry of Ecology and Environment of the People’s Republic of China, 2020), the amount of nitrogen emission by water pollutants in the whole country totaled 3,041,400 tons in 2017, of which emissions from the planting industry accounted for about 23.66%, or 719,500 tons. Over-fertilization has already caused significant harm to the ecological environment and requires more attention.
Water pollution caused by fertilizer application in farmland falls under the category of agricultural non-point source pollution, by which discharged solid or dissolved pollutants, such as nitrogen, phosphorus, pesticides, and organic or inorganic substances from rural areas, enter into water bodies through surface runoff, agricultural drainage and groundwater seepage, resulting in significant pollution. Agricultural non-point source pollution is difficult to predict due to its characteristics of a wide pollution range, highly dispersed sources, lag effects, and randomness of occurrence in both time and space. There is evidence that cultivated land systems has significant influence on surface water quality, and the fertilizer application has become the major source of agricultural non-point source pollution (Zou et al., 2020; Tim and Jolly, 1994).
To analyze the impact of non-point source pollution of agricultural fertilizer on surface water quality, this study selects Hainan Island as the research object. It offers the following unique advantages:
(1) A high proportion of agriculture. Given the relatively slow pace of industrial development in Hainan Province, agriculture plays a significant role. Therefore, it is easier to focus on the influence of agricultural pollution on water pollutants here;
(2) No transit water pollution. Hainan Island, as a closed island, does not encounter transit water pollution, and thus is not subjected to the influence of upstream and downstream transit water pollution, ensuring accuracy when studying the factors influencing agricultural fertilizer loss in this location;
(3) Significant spatiotemporal characteristics. Hainan Island experiences distinct dry and rainy seasons, with overall abundant precipitation. These climatic features result in a relatively lighter carrying effect and obvious spatiotemporal changes in agricultural non-point source pollutants, which is conducive to comparative studies on the effects of precipitation on fertilizer discharge.
There are many methods for studying the agricultural non-point source pollution, which are generally divided into empirical models, such as ECM (Han et al., 2011), IECM (Cheng et al., 2018) and HSM (Zhang et al., 2019), and mechanistic models, such as SWAT (Chen et al., 2019) and AGNPS (Haregeweyn and Yohannes, 2003). Most models perform well in the perspective of simulation results; however, their disadvantages regarding data sensitivity and regional applicability are quite pronounced. On one hand, the estimation accuracy of these models depends on numerous parameters, requiring large amount of input data and extensive available information, making their calibration and validation highly challenging (Xin et al., 2017). On the other hand, although most methods demonstrate good performance in developed countries, they may not be applicable for developing countries due to disparities between actual environmental conditions and experimental settings (de Oliveira et al., 2017). To estimate pollution load from agricultural non-point source, some scholars have adopted inventory analysis methods, utilizing readily accessible input production factors derived from statistical data to estimate pollution loads (Chen et al., 2006; Zou et al., 2020).
Due to the characteristics of agricultural non-point source pollution, it is not appropriate to simply describe the emission quantities of pollutants or their corresponding proportions as contributors to water pollution. Therefore, when conducting a macro-level analysis of the levels and trends of non-point source pollution of agricultural fertilizer, especially in a vast country like China, it is essential to employ a reliable estimation algorithm (model) and a supporting indicator system. Without such a framework, integrating diverse data and materials becomes challenging, making it even more difficult to draw scientific conclusions.
Based on existing research, two key issues related to the effects of pathways of agricultural non-point source pollution on water quality should be considered (Jin and Wu, 2008): 1. The proportions of pollutants emitted from agricultural productive activities that ultimately enter water bodies. 2. The hydrological conditions of water bodies as they determine increases and reductions in pollutant concentrations.
The amount of fertilizer applied provides the material basis of the discharge of pollutants from agricultural non-point sources (Bulut and Aksoy, 2008; Liu and Liang, 2019; Greenwood et al., 1989; Zhang et al., 2004), and the main factor driving fertilizer discharge is precipitation. Unabsorbed and unvolatile fertilizers continuously accumulate before precipitation, and can act as pollutants when they enter water bodies through runoff after precipitation. However, the processes by which pollutants enter water bodies are influenced by various factors, such as land use conditions, topography, hydrological characteristics, climate and weather, all of which leads to great spatiotemporal heterogeneity (Huang et al., 2015; Zhou et al., 2021; Wang et al., 2022; Mihiranga et al., 2021). Moreover, precipitation plays a dual role in the quality of water bodies: it has a carrying effect by bringing fertilizers into water bodies, increasing the total amounts and concentrations of pollutants in the water, as well as a dilution effect by enhancing the flow and velocity of water bodies, increasing the dilution and diffusion capacity of pollutants, facilitating water self-purification, and reducing pollutant concentration (De Girolamo et al., 2020).
To analyze the impact of agricultural fertilizer runoff on surface water quality, this study establishes a dynamic model that incorporates lag terms and interaction terms based on the mediating effect of precipitation (including carrying and dilution effects). The model is designed to explore the relationship between agricultural fertilization practices and surface water quality while identifying the impact of agricultural non-point source pollutants on water quality. The paper provides a reliable analytical method to promote sustainable agricultural development.
2 Study area
2.1 Regional profile
The present study focuses on the major rivers on Hainan Island, which is located in the northwest area of the South China Sea. Hainan Island is separated from Guangdong Province by the Qiongzhou Strait to the north and from Guangxi Province and Vietnam by the Beibu Gulf to the west (18°10′N∼20°10′N, 108°37′E∼111°03′E).
Hainan Island is located at the northern edge of the tropics and experiences a warm and hot climate throughout the year. The rainfall on Hainan Island is abundant, with distinct rainy and dry seasons. The dry season spans from November to April, while the rainy season extends from May to October, with frequent typhoons. The rainy season contributes 80%–90% of the annual rainfall, and rainfall during the typhoon season in August, September and October accounts for about 70% of the annual rainfall. Hainan Island presents a terrain of a central highland surrounded by lower areas. With Wuzhi Mountain as its highest point, located at its center, the elevation gradually decreases outward, forming an annular and multi-level layered structure. Due to the geomorphological characteristics of Hainan Island, rivers flow radially from the central mountainous or hilly areas towards the periphery, forming a radially patterned water system. The rivers are numerous, with steep gradients, short lengths and relatively low flow.
In terms of pollutant emissions, according to the “Second National Pollution Source Census Bulletin of Hainan Province” released in 2021 (Department of Ecology and Environment of Hainan Province, 2021). Hainan Province discharged a total of 41,789 tons of nitrogen in 2017, of which the agricultural sector accounted for 39.17%, or 16,369.16 tons. The total nitrogen discharge of the whole country in the corresponding period was 304.14 million tons, of which the agricultural contribution was only 23.66%, or 719,500 tons.
2.2 Water quality measurement
The water quality data was collected by National Surface Water Quality Stations which were established by Chinese government for the real-time, continuous and remote monitoring of water quality conditions in key sections of major rivers. The water quality data primarily includes parameters such as pH, dissolved oxygen, permanganate index, ammonia nitrogen, total nitrogen, total phosphorus and chlorophyll-α. A total of 27 Water Quality Monitoring Stations have been established at points along 20 rivers on Hainan Island; they are distributed throughout 11 cities and counties, including Haikou, Sanya, Danzhou, Wenchang, Qionghai, Wanning, Chengmai, Lingao, Ledong, Changjiang and Lingshui. Figure 1 illustrates the rivers within Hainan Island and the locations of the Water Quality Monitoring Stations. By virtue of these stations, we have a comprehensive understanding of water quality conditions in the rivers in Hainan Province, providing crucial data for water quality management and environmental protection.
Since agricultural fertilizer pollutants primarily consist of nitrogen and phosphorus, and considering the main pollution characteristics of Hainan Island, this study identifies total nitrogen as the primary focus of the pollution research.
2.3 Characteristics of changes in water quality, fertilizer application and precipitation
Table 1 summarizes the annual average total nitrogen concentration, total nitrogen loss and total fertilizer application in the watersheds of monitoring stations, as well as the proportions of sown areas occupied by cash crops at the 27 water quality monitoring stations in 2021. It can be observed from the table that total nitrogen pollution was relatively severe in the rivers of Hainan Island during this time. The total nitrogen loss at various water quality monitoring stations in 2021 was approximately 371,111 tons. According to the requirements for Class III water quality in the Chinese standard “GB 3838–2002 Environmental Quality Standards for Surface Water,” the total nitrogen concentration in the water should be less than 1.0 mg/L. However, among the 27 monitoring stations, 19 presented an annual average total nitrogen concentration exceeding 1.0 mg/L, while only 8 stations showed below 1.0 mg/L. This indicates that the total nitrogen concentrations at the majority of these stations exceeded the relevant standards.
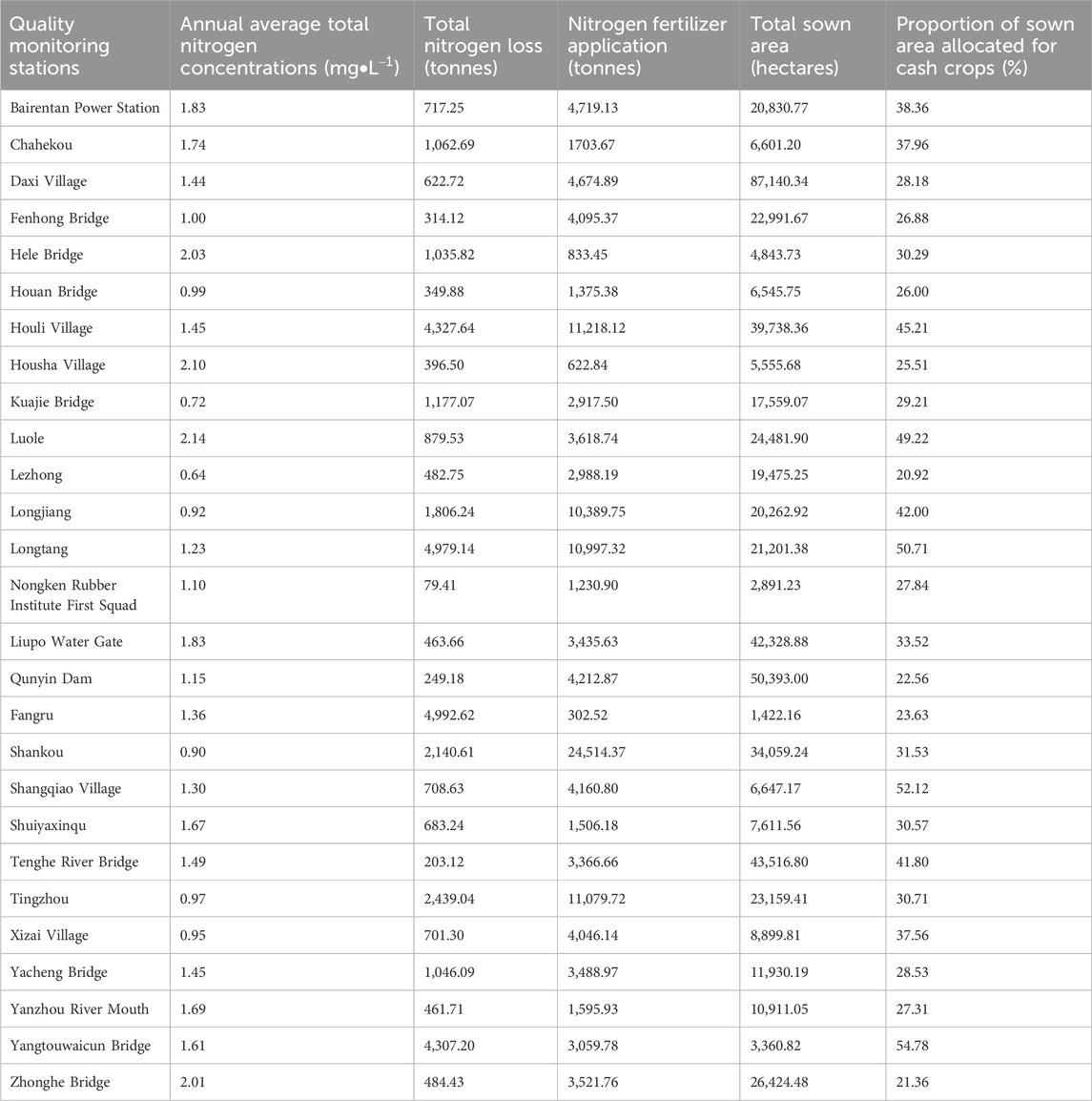
Table 1. Annual average concentrations of total nitrogen, total nitrogen loss and nitrogen fertilizer application, and proportion of sown area allocated for cash crops at the water quality monitoring stations in 2021.
For the fertilizer application, at these stations, the total levels of fertilizer application for agricultural production reached approximately 129,677 tons in 2021, with the proportion of sown area allocated for cash crops ranging from 20.92% to 54.78%.
2.4 Data sources
The present study primarily utilizes weekly measured data of total nitrogen concentration and nitrogen loss at water quality monitoring stations across Hainan Island, as well as data on crop planting area, fertilizer application and precipitation in 2021. The data were obtained through the following sources.
2.4.1 Nitrogen loss and total nitrogen concentration
The water quality data were derived from 27 national surface water monitoring stations on Hainan Island. The data are collected every 4 h automatically. For this study, total nitrogen data from January 1st to December 30th were obtained from 27 monitoring sections for analysis. Weekly average total nitrogen concentration is here calculated based on the measured concentrations, and the total nitrogen loss is calculated using the total nitrogen concentration and flow rate at the monitoring stations.
2.4.2 Sown area, fertilizer applications and fertilization intensities
The data on planting area, total quantity of nitrogen fertilizer applied and nitrogen fertilization intensities were obtained from watersheds whereat the water quality monitoring stations are located. The relevant information has been estimated based on data from the “Hainan Statistical Yearbook” regarding crop sown area and fertilizer application, cultivated land area within the watersheds of the water quality monitoring stations, as well as cultivation technical standards for crops and other relevant information for China and Hainan province.
2.4.3 Precipitation
Precipitation data for watersheds whereat the monitoring stations are located have been derived from the ERA5-Land hourly dataset from 1950 to the present, provided by the European Centre for Medium-Range Weather Forecasts (ECMWF). The weekly precipitation for each city and county has been calculated from the dataset.
3 Theoretical analysis and methods
3.1 Theoretical analysis
3.1.1 Mechanism of nitrogen fertilizer loss in farmland
The mechanism of nitrogen fertilizer loss in farmland is illustrated in Figure 2. According to studies by He et al. (2001) and Huang et al. (2021), the schedules and amounts of fertilizer application in agricultural production are determined by the local cropping system. Following the application of nitrogen fertilizers, nitrogen undergoes several transformations across different phases. Initially, a portion of ammonium (
3.1.2 The “substance-dynamic-factor” conceptual model of nitrogen loss in farmland
Based on the mechanisms of nitrogen fertilizer loss, Hou et al. (2008) proposed the conceptual model of “Substance Dynamic Factor” for nitrogen loss on farmland. In this model, the loss of nitrogen is primarily determined by the substance (total amount of fertilizer applied), the driving force (precipitation) and the regional environmental conditions (such as topography, soil properties and vegetation cover). The three factors interact with each other and ultimately affect water quality. Therefore, based on the three factors, a comprehensive analysis of the effects of agricultural fertilizer non-point source pollution on water quality can be conducted. The analytical model can be expressed as follows:
In Equation 1,
For regional analyses, A and U can be ignored by virtue of the relatively minor variations in the combinations of influential factors, such as topography and geomorphology, soil type, vegetation cover, and the utilization pattern of regional land (Hou et al., 2009). In this case, the model is expressed as:
Equation 2, is a linear function model, which can be utilized to describe the statistical relationship between nitrogen loss, fertilizer application and precipitation across a large regional scale. In general, the relationship can be described by a univariate function:
In Equation 3,
3.2 Model setup
3.2.1 Analysis model of the influence of nitrogen fertilizer loss in farmland on water quality
Jin studied (2014) the influence of precipitation on ammonia nitrogen concentration, taking into account time lag in the process from nitrogen runoff to entry into the water. Lagged terms for precipitation and fertilizer application are incorporated into the “Substance Dynamics Factors” conceptual model of nitrogen loss on farmland. Furthermore, this model can be extended into a dynamic panel model with regional fixed effects to analyze the regional-scale influences.
Based on the above-mentioned research, a model for analyzing the influence of nitrogen fertilizer loss on farmland on water quality can be established as follows:
In Equation 4, subscript i represents the monitoring station; subscript t represents time (weeks);
After nitrogen fertilizer applied, the remaining nitrogen, following crops uptake, will be lost through various pathways including leaching, volatilization and runoff. Since this study primarily focuses on runoff loss, it requires the loss coefficient
The utilization efficiency of nitrogen fertilizer ranges approximately from 30% to 45% on farmland (Quan et al., 2021; Lin et al., 2007), which implies that the amount of nitrogen fertilizer loss accounts for 55%–70% of the total amount of nitrogen fertilizer application. According to Chen’s research (2014), the proportions of nitrogen loss with runoff is approximately 23%.
Loss coefficient
As the result, the loss coefficient (
According to “Bulletin of the Second National Pollution Census of Hainan Province” released by the government of Hainan Province, the total nitrogen fertilizer loss with runoff from agriculture in Hainan Province was 16,369.16 tons in 2017, while the total nitrogen fertilizer application amounted to 155,700 tons. Therefore, the loss of nitrogen with runoff from farmland accounts for approximately 10.5%. This loss coefficient (
Pollutants carried by precipitation into rivers represent a primary mode of non-point source pollution; therefore, the precipitation level significantly affects water quality. According to Equation 4, when precipitation exhibits statistical significance and the estimated coefficient is positive, it indicates a carrying effect of the precipitation, and this leads to an increase in total nitrogen concentration. Conversely, when the precipitation exhibits statistical significance and the estimated coefficient is negative, it suggests a dilution effect, and this leads to a reduction in nitrogen concentration.
The statistical significance and positive coefficient for nitrogen fertilizer application, as a source of pollutants, in Equation 4 illustrates that an increase in fertilizer application will have a positive influence on water quality. Since fertilizers need to be carried into the river by precipitation, an interaction term between fertilizer application and precipitation is introduced to verify their combined effect.
Considering the possible time required for nitrogen fertilizer to enter rivers through precipitation, lagged terms for fertilizer application, precipitation and their interaction are added into Equation 4 in order to investigate the influence of lagged precipitation and fertilization on water quality in the subsequent period.
Because of the distinct dry and rainy seasons on Hainan Island, fertilizer application and precipitation during different seasons exert different effects on nitrogen runoff. The accumulation of fertilizers differs between the dry and rainy seasons due to the lower precipitation and longer intervals in the dry season, which results in divergence in the effects of fertilizer application and precipitation on water quality between the dry and rainy seasons. To analyze the influences of fertilizer application and precipitation on total nitrogen concentration during the dry and rainy seasons, DS (dummy variable for dry season) and RS (dummy variable for rainy season) are introduced. By incorporating these dummy variables into the explanatory variables of Equation 4 and forming new interaction terms, nitrogen runoff models for the dry season Equation 7 and the rainy season Equation 8 can be obtained:
In this model, all variables in the equations have been logarithmically transformed, which allows for a clearer expression of the influence of the change rate of fertilizer application and precipitation on the change rate of total nitrogen concentration.
Table 2 shows the definitions and descriptive statistics of each variable used in the model.
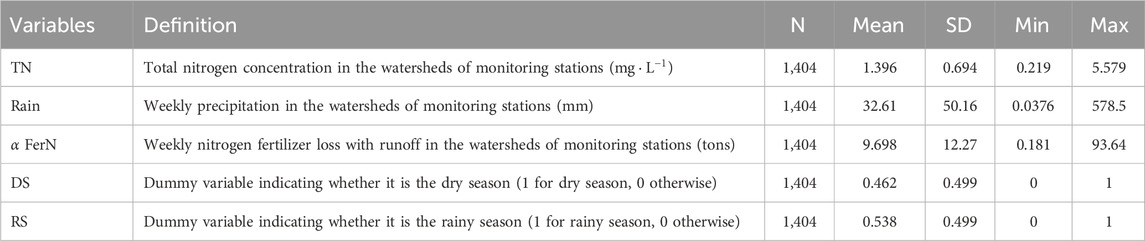
Table 2. Definitions and descriptive statistics of model variables related to the influence of nitrogen fertilizer loss in farmland on water quality.
3.2.2 Model of the influence of planting structure on nitrogen fertilizer loss
The material basis for the loss of nitrogen fertilizer in farmland is the regional planting structure. The total amount of fertilizer applied varies across different regions due to the influence of planting structure, and this subsequently affects the quantity of pollutant loss. On the basis of studies by Liang et al. (2013) and Min et al. (2020) regarding the influence of planting structure on fertilizer application and loss, the following model is established to analyze the influence of planting structure on nitrogen loss:
In Equation 9, subscript i represents the monitoring station; subscript t represents time (weeks); the dependent variable
In the model, all variables in the equations have been logarithmically transformed, which allows for a clearer expression of the influence of the change rate of each variable on the change rate of total nitrogen loss. Table 3 shows the definitions and descriptive statistics of each variable in the model.
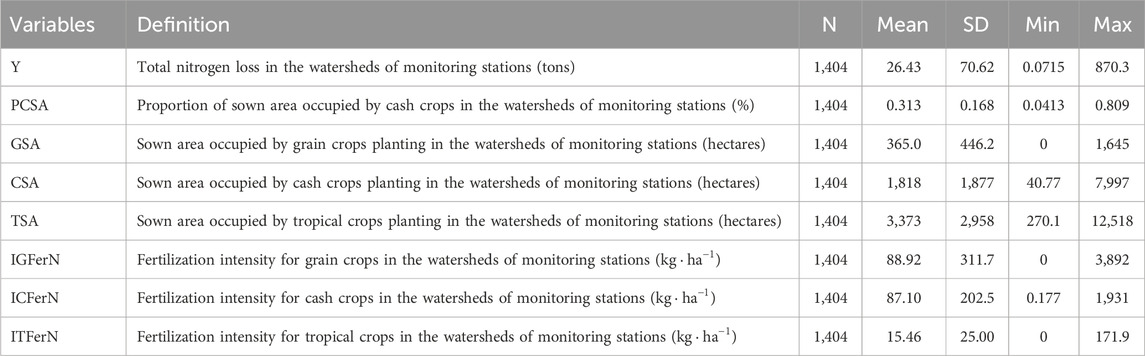
Table 3. Definitions and descriptive statistics of model variables related to the influence of planting structure on nitrogen loss.
3.3 Estimation method
A lagged term of total nitrogen concentration has been incorporated into the model assessing the influence of nitrogen fertilizer runoff from farmland on water quality, which means that the explanatory variables in the model now include lagged terms of the dependent variables, thus introducing a correlation between the dependent variables and the random error terms. Meanwhile, for a long dynamic panel dataset where the number of time series (52 weeks in total) exceeds the number of monitoring points (27 in total), using Generalized Method of Moments (GMM) for estimation might result in significant biases.
Kivet et al. (1999), through Monte Carlo simulation analysis, found that for long panels, the estimates yielded by the Bias-Corrected Least Square Dummy Variable Model (LSDVC) were significantly superior to those from the Difference GMM and System GMM. The LSDVC model was capable of correcting over 90% of the biases. Therefore, this study utilizes the LSDVC model for its estimations based on the Difference GMM estimator proposed by Arellano-Bond. Simultaneously, a comparative analysis is conducted using OLS regression and a Fixed Effects model. Although OLS regression and the Fixed Effects model may present biases in estimating dynamic panel data, their results respectively indicate the upper and lower bounds of true estimates for the dependent variables’ lagged terms. Thus, the present paper presents the estimations produced by OLS regression and the Fixed Effects model for comparison.
The model regarding the influence of planting structure on nitrogen loss is based on panel data. Therefore, the Fixed Effects model is utilized to estimate the coefficients of explanatory variables.
4 Results
4.1 Influence of agricultural nitrogen fertilizer loss on water quality
In this section, we analyze the influence of nitrogen fertilizer application and precipitation on the total nitrogen concentration in rivers across Hainan Island. Furthermore, we estimate whether there are variations between the dry season and the rainy season. The results are presented in Tables 4, 5.
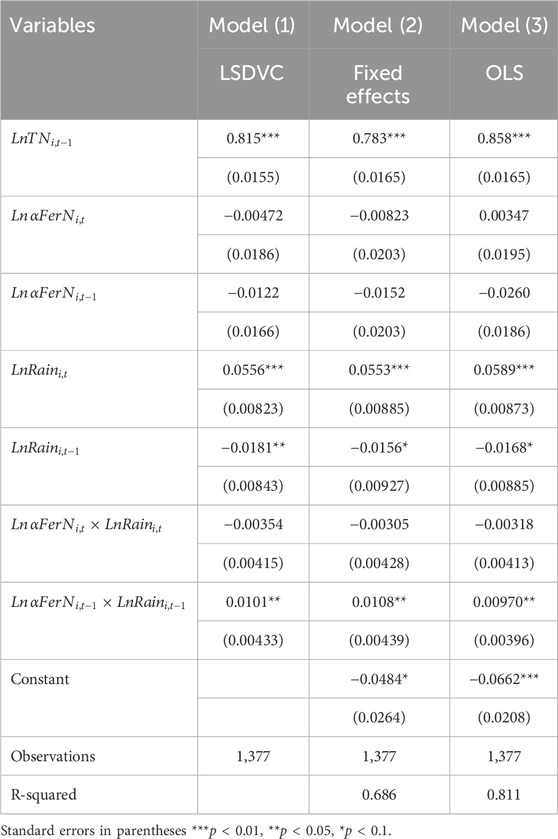
Table 4. Regression results of the influence of agricultural nitrogen fertilizer loss on water quality Models.
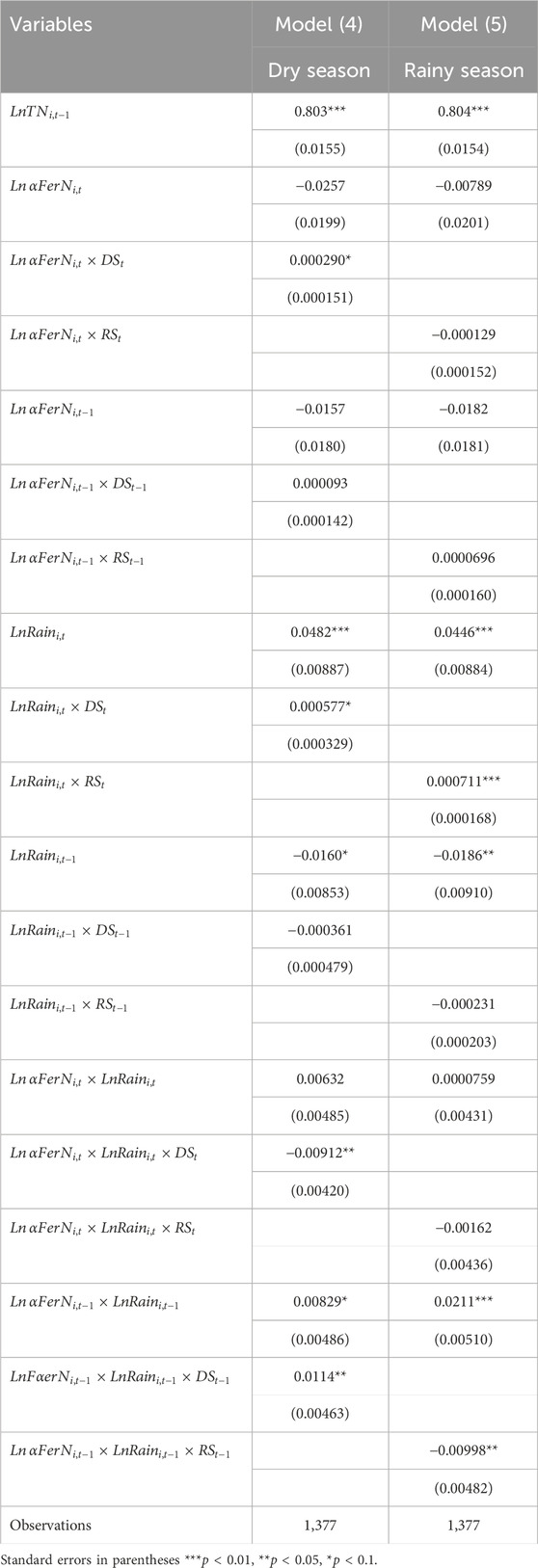
Table 5. LSDVC regression results of the influence of agricultural nitrogen fertilizer loss on water quality models in dry and rainy season.
Table 4 shows the regression results of the model for the influence of agricultural nitrogen fertilizer loss on water quality. In Model (1), the lagged total nitrogen concentration is statistically significant at the 1% level, indicating the cumulative nature of pollutants. An increase in the total nitrogen concentration in the previous period significantly raises the concentration of total nitrogen in the current period. The influence of current precipitation on total nitrogen concentration is statistically significant at the 1% level, with an estimated coefficient of 0.0556. The lagged precipitation is statistically significant at the 5% level, with an estimated coefficient of −0.0181. Neither current nor lagged fertilizer application are statistically significant. However, the interaction term between lagged precipitation and lagged nitrogen fertilizer application is statistically significant at the 5% level, with an estimated coefficient of 0.0101.
The regression results elucidate the static relationship between the effects of precipitation and fertilizer application on total nitrogen concentration.
Firstly, precipitation on Hainan Island plays a dual role in influencing water quality by both carrying effect and diluting effect. In most simulated rainfall experiments, rainfall intensity has been identified as a significant factor influencing the losses of nitrogen and phosphorus (Song et al., 2017). The elevation of rainfall intensity in these experiments is associated with overall increases in total nitrogen losses (Wu et al., 2018). In this study, through the analysis of current precipitation on Hainan Island, it has been found that current precipitation has a carrying effect on nitrogen loss. Specifically, for every 1% increase in current precipitation, the total nitrogen concentration in rivers increases by 0.0542%. In addition, some studies have also shown that as runoff flow increases, the dilution effect tends to reduce nitrogen concentration. In Yang et al. (2009) study on the influence of precipitation on nitrogen and phosphorus loss in the Sichuan Basin, it was found that precipitation has a dilution effect on the concentration of NO₃-N in the flow, and the concentration decreases with the increase flow before increasing with the decrease in flow. Girolamo et al. (2020) simulation results for the southern watershed of Sardinia Island also show that the concentration of nitrogen decreased in accordance with the flow, and increased with a decrease in flow. In the present study, the lagged precipitation on Hainan Island exhibits a diluting effect on water quality. For each 1% increase in the lagged precipitation, there is a decrease of 0.014% in the total nitrogen concentration in rivers. The above findings indicate that precipitation on Hainan Island plays a dual role in the quality of water bodies.
Secondly, the fertilization practices of farmers on Hainan Island significantly impact water quality; however, this influence exhibits a lag effect. Some studies indicate that within 8 days after the application of nitrogen fertilizer, initial surface runoff events significantly increase the nitrogen concentration (Cui et al., 2020), and nitrogen concentration reaches higher levels within 7–10 days after fertilization, and gradually decreases thereafter (Xue et al., 2014). Based on the results of this study, it can be observed that the influence of nitrogen fertilizer application on water quality also demonstrates a lagged effect. For the nitrogen fertilizer loss induced by precipitation, each 1% increase in the lagged application of nitrogen fertilizer leads to a 0.0101% increase in total nitrogen concentration.
To validate the credibility of the LSDVC regression results, the estimated coefficients of the LSDVC method for the lagged terms of the dependent variable should lie between those of the fixed effects model and OLS regression. Model (2) and Model (3) correspond to the estimation results of the fixed effects model and OLS regression, respectively. The results from both models are statistically significant at the 1% level, and the estimated coefficients are positive. For the lagged term of total nitrogen concentration, the estimated coefficient in the fixed effects model is 0.783, representing the lower limit. In the OLS regression model, the estimated coefficient is 0.858, representing the upper limit. The LSDVC method’s estimated coefficient for the lagged total nitrogen concentration is statistically significant at the 1% level, with the value of 0.815. It falls within the credible interval between the upper and lower limits, indicating that the LSDVC method provides reliable estimates for total nitrogen concentration.
Table 5 presents the regression results of the influence models for nitrogen fertilizer loss in farmland on water quality during the dry season (Model 4) and the rainy season (Model 5). In the model of the dry season (Model 4), the interaction term between current precipitation and the dummy variable for the dry season is statistically significant at the 10% level, with an estimated coefficient of 0.000577. Moreover, the interaction terms between lagged nitrogen fertilizer application, lagged precipitation and the dummy variable for the dry season are statistically significant at the 5% level, with coefficients of 0.0114.
On the other hand, in the model of the rainy season (Model 5), the interaction term between current precipitation and the dummy variable for the rainy season is statistically significant at the 1% level, with an estimated coefficient of 0.000711. Meanwhile, the estimates for the interaction terms between lagged nitrogen fertilizer application, lagged precipitation and the dummy variable for the rainy season are statistically significant at the 10% level, with coefficients of −0.00998.
Figure 3 presents the weekly average total nitrogen concentration, precipitation and nitrogen fertilizer application data for all monitoring stations. It can be observed that there are noticeable seasonal variations on the island, with pronounced differences in precipitation between the dry season and the rainy season, the total nitrogen concentration in the rivers exhibits a V-shaped variation trend throughout the year and The variations in nitrogen fertilizer application are correlated with the planting season, and show two distinct peaks.
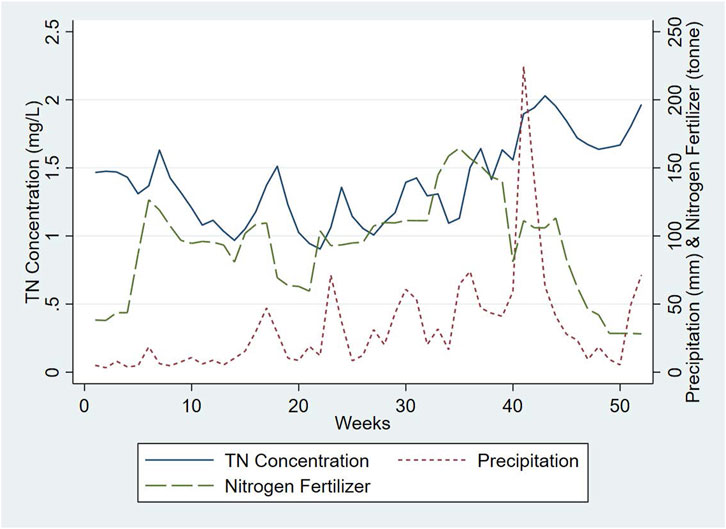
Figure 3. Weekly average precipitation, fertilizer application and total nitrogen concentration in Hainan Island in 2021.
As shown in the figure, the influence of precipitation and fertilization on water quality in Hainan Island exhibit dynamic correlations. The estimation results of the dry season model (Model 4) and the wet season model (Model 5) further illustrate the dynamic relationship between these factors. It can be observed that the influence of precipitation and fertilization on total nitrogen concentration in rivers on Hainan Island exhibit significant differences between the dry and rain seasons. During the dry season, the longer intervals between precipitation allow for greater accumulation of fertilizer in agricultural fields compared to the rainy season. Consequently, with the same amount of precipitation, the carrying effect of precipitation is stronger in the dry season, resulting in greater nitrogen runoff into rivers and a greater impact on total nitrogen concentration. However, since the amount and frequency of precipitation are lower in the dry season compared to the rainy season, the dilution effect of lagged precipitation on total nitrogen concentration is relatively weaker.
4.2 Influence of planting structure on nitrogen loss
The regression results of the model assessing the influences of nitrogen fertilizer loss on water quality reveal significant effects of agricultural fertilization activities on total nitrogen pollution. Considering the potential influence of changes in planting structure on the total amount of fertilization, this section focuses on estimating the influence of planting structure on nitrogen loss, with nitrogen loss as the dependent variable and the proportion of sown area allocated for cash crops as the core explanatory variable. The estimated results are presented in Table 6.
From the estimation results, the R-squared value of 0.535 shown in Table 6 indicates that the model can account for 53.5% of the variance in total nitrogen loss. The proportion of sown area allocated for cash crops is statistically significant at the 1% level, with an estimated coefficient of 3.595, indicating that planting structure is one of the main factors affecting nitrogen loss. When the proportion of sown area allocated for cash crops with high fertilizer application but low utilization efficiency increases, the amount of nitrogen loss in the watersheds will also rises. Specifically, for each 1% increase in the proportion of sown area allocated for cash crops, the nitrogen loss increases by 3.595%.
The results indicate that the planting structure on Hainan Island significantly affects the nitrogen loss. As the proportion of sown area allocated for cash crops increases, which are characterized by high fertilizer application and low utilization efficiency, nitrogen loss in the watersheds also rises. Specifically, for each 1% increase in the sown area allocated for cash crops, nitrogen loss increases by 3.595%. Additionally, the estimated results show that a 1% increase in the fertilization intensity of cash crops leads to a 0.326% increase in total nitrogen loss in the watersheds. In contrast, the production activities of grain crops and tropical crops have a relatively smaller influence on nitrogen runoff.
Based on existing research, from the perspective of land use, indicated that land use and land cover can affect the phosphorus output (Recanatesi et al., 2013) and there is significant variation in the total nitrogen output per unit area of farmlands allocated for crops such as sugarcane, rice and rubber (Yang et al., 2011). From the perspective of fertilizer utilization efficiency in crops, the demands for nitrogen fertilizer and its utilization efficiency vary among different crop type, resulting in differences in nitrogen runoff among different crops on Hainan Island. Therefore, for agricultural production activities on Hainan Island, the cultivation of cash crops significantly impacts nitrogen runoff, while the influence of grain crops and tropical crops is relatively smaller. Consequently, the total nitrogen runoff on Hainan Island is expected to increase with a higher proportion of cash crop cultivation and greater fertilizer application intensity.
5 Conclusion and policy implications
5.1 Conclusion
This study investigated the effects of fertilization and precipitation on the total nitrogen concentration in rivers on Hainan Island, and extends the total nitrogen loss model by introducing lag terms, dummy variables and interaction terms for the dry and rainy seasons. The influences of fertilization and precipitation on river nitrogen concentration at different time periods (current and lagged) as well as during different seasons (dry and rainy) are analyzed, and the dynamic correlation between agricultural production activities and non-point source pollution is explored. Additionally, the relationship between planting structure and non-point source pollutant loss is investigated, highlighting the significant differences between the effects of various crop types on pollutant loss. The results indicate that the proportion of planting area allocated for economic crops and fertilizer intensity significantly influences pollutant losses, while the influences of food crops and tropical crops are less pronounced.
Compared to previous literature, this study utilizes weekly data from 27 monitoring stations on Hainan Island in 2021, providing accurate and detailed information on composition of water pollution. This dataset addresses the scarcity of direct observational data on agricultural non-point source pollution. Methodologically, this study incorporates precipitation as an exogenous factor, considering its mediating effect in the entry of non-point source pollutants into water bodies. Additionally, it includes lagged and interaction terms to examine the effects of precipitation and fertilization on water quality. This method addresses the shortcomings of insufficient detailed regional data on agricultural non-point source pollutants and transforms the static relationship of pollution influence into a dynamic one, resulting in more accurate results. It helps in analyzing the contribution of agricultural non-point source pollution to water pollution and provides reliable references for sustainable agricultural development.
5.2 Policy implications
The results of this study offer significant policy guidance for the prevention and control of agricultural non-point source pollution. The recommendations are as follows:
(1) Adopt advanced technologies for reducing fertilizer application, such as soil testing, slow-release fertilizers, precision fertilization techniques and the utilization of organic fertilizers. Implement scientific and rational fertilization management, improve nutrient utilization efficiency and minimize negative externalities associated with excessive fertilizer application on the environment.
(2) Strengthen collaboration between agricultural regulatory authorities and meteorological departments in Hainan Province to develop scientific and rational guidelines for the planting periods and structures of cash crops. Such guidelines should be tailored based on local rainfall and temperature conditions in order to be better adapted to climate changes and enhance the efficiency of agricultural production. Agricultural regulatory authorities can also access real-time meteorological data to assist farmers in formulating more precise fertilization plans, reducing fertilization application before precipitation, and minimizing nutrient loss during rainy periods.
(3) It is crucial to reinforce water resource management policies, such as by establishing effective isolation facilities between rivers and irrigation channels, including diversion embankments, barriers or other physical obstacles, to control the amount of irrigation or precipitation drainage from farmland. Such measures would not only reduce the likelihood of nitrogen elements flowing into rivers, but would also facilitate the reuse of nitrogen-containing water resources, thereby improving nitrogen fertilizer utilization rates and minimizing the adverse environmental impacts of nitrogen loss.
Data availability statement
The original contributions presented in the study are included in the article/supplementary material, further inquiries can be directed to the corresponding author.
Author contributions
SW: Conceptualization, Data curation, Formal Analysis, Investigation, Methodology, Resources, Software, Validation, Visualization, Writing–original draft, Writing–review and editing. SZ: Conceptualization, Investigation, Methodology, Resources, Validation, Writing–review and editing.
Funding
The author(s) declare that no financial support was received for the research, authorship, and/or publication of this article.
Conflict of interest
The authors declare that the research was conducted in the absence of any commercial or financial relationships that could be construed as a potential conflict of interest.
Publisher’s note
All claims expressed in this article are solely those of the authors and do not necessarily represent those of their affiliated organizations, or those of the publisher, the editors and the reviewers. Any product that may be evaluated in this article, or claim that may be made by its manufacturer, is not guaranteed or endorsed by the publisher.
References
Bulut, E., and Aksoy, A. (2008). Impact of fertilizer usage on phosphorus loads to Lake Uluabat. Desalination 226 (1–3), 289–297. doi:10.1016/j.desal.2007.02.112
Chen, L., Chen, S., Li, S., and Shen, Z. (2019). Temporal and spatial scaling effects of parameter sensitivity in relation to non-point source pollution simulation. J. Hydrology 571, 36–49. doi:10.1016/j.jhydrol.2019.01.045
Chen, M., Chen, J., and Du, P. (2006). An inventory analysis of rural pollution loads in China. Water Sci. Technol. 54 (11-12), 65–74. doi:10.2166/wst.2006.831
Chen, X., Cui, Z., Fan, M., Vitousek, P., Zhao, M., Ma, W., et al. (2014). Producing more grain with lower environmental costs. Nature 514, 486–489. doi:10.1038/nature13609
Cheng, X., Chen, L., Sun, R., and Jing, Y. (2018). An improved export coefficient model to estimate non-point source phosphorus pollution risks under complex precipitation and terrain conditions. Environ. Sci. Pollut. Res. 25, 20946–20955. doi:10.1007/s11356-018-2191-z
Cui, N., Cai, M., Zhang, X., Abdelhafez, A. A., Zhou, L., Sun, H., et al. (2020). Runoff loss of nitrogen and phosphorus from a rice paddy field in the east of China: effects of long-term chemical n fertilizer and organic manure applications. Glob. Ecol. Conserv. 22, e01011. doi:10.1016/j.gecco.2020.e01011
De Girolamo, A. M., and Lo Porto, A. (2020). Source apportionment of nutrient loads to a mediterranean river and potential mitigation measures. Water 12 (2), 577. doi:10.3390/w12020577
de Oliveira, L. M., Maillard, P., and de Andrade Pinto, E. J. (2017). Application of a land cover pollution index to model non-point pollution sources in a Brazilian watershed. Catena 150, 124–132. doi:10.1016/j.catena.2016.11.015
Department of Ecology and Environment of Hainan Province (2021). Bulletin of the second national pollution census of hainan province. Available at: https://hnsthb.hainan.gov.cn/xxgk/0200/0202/zwgk/zfwj/202101/t20210106_2914986.html (Accessed December 18, 2023).
Greenwood, D. J., Kubo, K., Burns, I. G., and Draycott, A. (1989). Apparent recovery of fertilizer n by vegetable crops. Soil Sci. Plant Nutr. 35 (3), 367–381. doi:10.1080/00380768.1989.10434770
Han, L. X., Huo, F., and Sun, J. (2011). Method for calculating non-point source pollution distribution in plain rivers. Water Sci. Eng. 4 (1), 83–91. doi:10.3882/j.issn.1674-2370.2011.01.008
Haregeweyn, N., and Yohannes, F. (2003). Testing and evaluation of the agricultural non-point source pollution model (AGNPS) on Augucho catchment, western Hararghe, Ethiopia. Agric. Ecosyst. and Environ. 99 (1-3), 201–212. doi:10.1016/s0167-8809(02)00120-2
He, B., Zhou, N., and Hu, X. (2001). On farmland precipitation runoff non-point pollution model — farmland nitrogen pollution model in Shanghai suburbs. Resour. Environ. Yangtze Basin 02, 159–165.
Hou, Y., Li, H., Zhou, Y., and Zhao, H. (2008). Nitrogen non-point field pollution in China: II establishment of index system for evaluation of pollution degree. J. Agro-Environment Sci. 27 (4), 1277–1282.
Hou, Y., Li, H., Zhou, Y., and Zhao, H. (2009). Nitrogen non-point field pollution in China: III demonstration of the model. J. Agro-Environment Sci. 28 (7), 1337–1340.
Huang, J. J., Lin, X., Wang, J., and Wang, H. (2015). The precipitation driven correlation-based mapping method (pcm) for identifying the critical source areas of non-point source pollution. J. Hydrol. 524, 100–110. doi:10.1016/j.jhydrol.2015.02.011
Huang, W., Zhou, F., Liang, H., and Chen, L. (2021). Research progress on nitrogen and phosphorus runoff loss models for rice paddy. J. Lake Sci. 33 (2), 336–348. doi:10.18307/2021.0202
Jin, S., and Wu, Y. (2008). Is agricultural non-point source the primary cause of water pollution — proof based on data from the Huaihe River Basin. Chin. Rural. Econ. 09, 71–81.
Kiviet, J. F., Phillips, G. D. A., and Schipp, B. (1999). Alternative bias approximations in first-order dynamic reduced form models. J. Econ. Dyn. Control 23, 909–928. doi:10.1016/s0165-1889(98)00055-4
Liang, J., Qiu, H., Jiang, Y., Liao, S., and Han, W. (2013). Decomposition of factors contributed to the increase of China’s chemical fertilizer use and projections for future fertilizer use in China. J. Nat. Resour. 28 (11), 1869–1878. doi:10.11849/zrzyxb.2013.11.004
Lin, D. X., Fan, X. H., Hu, F., Zhao, H. T., and Luo, J. F. (2007). Ammonia volatilization and nitrogen utilization efficiency in response to urea application in rice fields of the Taihu Lake Region, China. Pedosphere 17 (5), 639–645. doi:10.1016/S1002-0160(07)60076-9
Liu, J., and Liang, Y. (2019). Effect of planting structure adjustment on chemical fertilizer application in China. J. Agro-Environment Sci. 38 (11), 2544–2552. doi:10.11654/jaes.2019-0477
Mihiranga, H. K. M., Jiang, Y., Li, X., Wang, W., De Silva, K., Kumwimba, M. N., et al. (2021). Nitrogen/phosphorus behavior traits and implications during storm events in a semi-arid mountainous watershed. Sci. Total Environ. 791, 148382. doi:10.1016/j.scitotenv.2021.148382
Min, J., Ji, R., Wang, X., Chen, K., Xu, J., Pan, Y., et al. (2020). Changes in planting structure and nitrogen and phosphorus loss loads of farmland in Taihu like Region. Chin. J. Eco-Agriculture 28 (08), 1230–1238. doi:10.13930/j.cnki.cjea.200152
Ministry of Ecology and Environment of the People’s Republic of China (2020). The bulletin of the second national pollution source census. Available at: https://www.mee.gov.cn/xxgk2018/xxgk/xxgk01/202006/t20200610_783547.html (Accessed December 15, 2023).
Quan, Z., Zhang, X., Fang, Y., and Davidson, E. A. (2021). Different quantification approaches for nitrogen use efficiency lead to divergent estimates with varying advantages. Nat. Food 2, 241–245. doi:10.1038/s43016-021-00263-3
Recanatesi, F., Ripa, M. N., Leone, A., Luigi, P., and Luca, S. (2013). Land use, climate and transport of nutrients: evidence emerging from the Lake Vicocase study. Environ. Manage. 52 (2), 503–513. doi:10.1007/s00267-013-0060-6
Song, X., Gao, Y., Green, S. M., Dungait, J. A. J., Peng, T., Quine, T. A., et al. (2017). Nitrogen loss from karst area in China in recent 50 years: an in-situ simulated rainfall experiment’s assessment. Ecol. Evol. 7 (23), 10131–10142. doi:10.1002/ece3.3502
Tim, U. S., and Jolly, R. (1994). Evaluating agricultural nonpoint-source pollution using integrated geographic information systems and hydrologic/water quality model. J. Environ. Qual. 23 (1), 25–35. doi:10.2134/jeq1994.00472425002300010006x
Wang, D., Xu, C., Ye, C., Chen, S., Chu, G., and Zhang, X. (2018). Low recovery efficiency of basal fertilizer-N in plants does not indicate high basal fertilizer-N loss from split-applied N in transplanted rice. Field Crops Res. 229, 8–16. doi:10.1016/j.fcr.2018.09.008
Wang, S., Peng, H., Hu, Q., and Jiang, M. (2022). Analysis of runoff generation driving factors based on hydrological model and interpretable machine learning method. J. Hydrol. Reg. Stud. 42, 101139. doi:10.1016/j.ejrh.2022.101139
Wu, L., Peng, M., Qiao, S., and Ma, X. (2018). Assessing impacts of rainfall intensity and slope on dissolved and adsorbed nitrogen loss under bare loessial soil by simulated rainfalls. Catena 170, 51–63. doi:10.1016/j.catena.2018.06.007
Xin, X. K., Yin, W., and Li, K. F. (2017). Estimation of non-point source pollution loads with flux method in Danjiangkou Reservoir area, China. Water Sci. Eng. 10 (2), 134–142. doi:10.1016/j.wse.2017.05.001
Xue, L., Yu, Y., and Yang, L. (2014). Maintaining yields and reducing nitrogen loss in rice–wheat rotation system in Taihu Lake region with proper fertilizer management. Environ. Res. Lett. 9 (11), 115010. doi:10.1088/1748-9326/9/11/115010
Yang, J. L., Zhang, G. L., Shi, X. Z., Wang, H. J., Cao, Z. H., and Ritsema, C. J. (2009). Dynamic changes of nitrogen and phosphorus losses in ephemeral runoff processes by typical storm events in Sichuan Basin, Southwest China. Soil Tillage Res. 105 (2), 292–299. doi:10.1016/j.still.2009.04.003
Yang, S., Dong, G., Zheng, D., Xiao, H., Gao, Y., and Lang, Y. (2011). Coupling Xinanjiang model and SWAT to simulate agricultural non-point source pollution in Songtao watershed of Hainan, China. Ecol. Model. 222 (20), 3701–3717. doi:10.1016/j.ecolmodel.2011.09.004
Zhang, Q. L., Chen, Y. X., Jilani, G., Shamsi, I. H., and Yu, Q. G. (2010). Model AVSWAT apropos of simulating non-point source pollution in taihu lake basin. J. Hazard. Mat. 174 (1), 824–830. doi:10.1016/j.jhazmat.2009.09.127
Zhang, T., Yang, Y., Ni, J., and Xie, D. (2019). Adoption behavior of cleaner production techniques to control agricultural non-point source pollution: a case study in the Three Gorges Reservoir Area. J. Clean. Prod. 223, 897–906. doi:10.1016/j.jclepro.2019.03.194
Zhang, W., Wu, S., Ji, H., and Kolbe, H. (2004). Estimation of agricultural non-point source pollution in China and the alleviation strategies i. estimation of agricultural non-point source pollution in China in early 21 century. Sci. Agric. Sin. 37 (7), 1008–1017.
Zhou, W., Zhu, Z., Xie, Y., and Cai, Y. (2021). Impacts of rainfall spatial and temporal variabilities on runoff quality and quantity at the watershed scale. J. Hydrol. 603, 127057. doi:10.1016/j.jhydrol.2021.127057
Keywords: agricultural non-point source pollution, fertilizer, precipitation, LSDVC, planting structure
Citation: Wang S and Zhou S (2024) The dynamic relation between fertilization, precipitation and the diffusion of agricultural non-point pollution in Hainan Island. Front. Environ. Sci. 12:1419912. doi: 10.3389/fenvs.2024.1419912
Received: 19 April 2024; Accepted: 18 October 2024;
Published: 29 October 2024.
Edited by:
David William O’Connell, Trinity College Dublin, IrelandReviewed by:
Jianrong Ma, Chinese Academy of Sciences (CAS), ChinaFrancisco José De Paula Filho, Universidade Federal do Cariri, Brazil
Copyright © 2024 Wang and Zhou. This is an open-access article distributed under the terms of the Creative Commons Attribution License (CC BY). The use, distribution or reproduction in other forums is permitted, provided the original author(s) and the copyright owner(s) are credited and that the original publication in this journal is cited, in accordance with accepted academic practice. No use, distribution or reproduction is permitted which does not comply with these terms.
*Correspondence: Shudong Zhou, sdzhou@njau.edu.cn