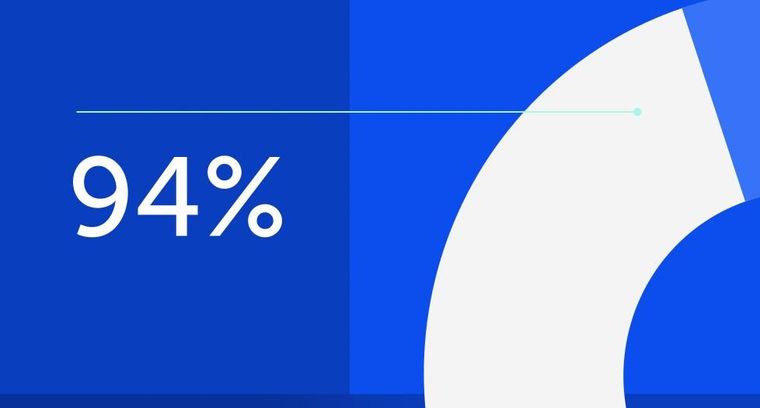
94% of researchers rate our articles as excellent or good
Learn more about the work of our research integrity team to safeguard the quality of each article we publish.
Find out more
ORIGINAL RESEARCH article
Front. Environ. Sci., 18 July 2024
Sec. Environmental Economics and Management
Volume 12 - 2024 | https://doi.org/10.3389/fenvs.2024.1419307
This study explores how foreign direct investment (FDI), material footprint, economic growth, and information and communication technology (ICT) influence the load capacity factor (LCF) in 37 Sub-Saharan African (SSA) countries. The analysis leverages data spanning 1970 to 2019 and employs a range of robust econometric techniques (FM-OLS, D-OLS, DSUR). The findings reveal a surprising yet significant positive association between material footprint and LCF. Conversely, economic growth, FDI, and ICT all exhibit negative relationships with LCF. Interestingly, a causal analysis suggests that these variables–ICT, material footprint, and economic growth–have a two-way influence on LCF, meaning they both affect and are affected by it. These insights highlight the complex interplay between economic development, material footprint, and technological advancement in shaping SSA’s LCF. The study concludes by offering clear policy recommendations for SSA countries aiming to optimize their LCF.
Sub-Saharan Africa (SSA) is now witnessing swift economic expansion, which is partly fuelled by foreign direct investment (FDI), and other topical factors (Adebayo et al., 2024). Nevertheless, this expansion prompts crucial inquiries regarding the enduring viability. Is it possible for the SSA to achieve a harmonious equilibrium between economic expansion and environmental constraints? This study examines the intricate relationship between foreign direct investment (FDI), material footprint, and information and communication technologies (ICT) on the load capacity factor (LCF) in Sub-Saharan African (SSA) nations. The LCF measures the ability of ecosystems in these countries to withstand and support development pressures. The LCF is a vital measure of environmental sustainability since it demonstrates the effectiveness of resource utilization. A high LCF indicates an economy that produces a significant amount of product while causing minimum harm to the environment. On the other hand, a low LCF indicates inefficiency and the risk of depleting resources (Ayad et al., 2023). Gaining insight into the determinants that impact the Load Capacity Factor (LCF) in Sub-Saharan Africa (SSA) is crucial for establishing a viable trajectory towards sustainable development.
At the present time, the preeminent economic and ecological concerns are sustainability and environmental degradation. Specifically, the industrialization and economic development of certain nations have exerted significant strain on the environment. The rapid speed of growth has placed further strain on the environment, beyond its ability to support life (Xu et al., 2022). While global biological capacity has increased by 27% in the last 50 years, the ecological footprint has increased by 190% during the same time frame (WWF, 2018; Marti and Puertas, 2020), resulting in a significant biocapacity deficit. Evidence suggest that more than 80% of the world’s population currently lives in countries where the ecological footprint surpasses the available biological capacity for self-renewal. In 2009, Africa experienced an ecological deficit due to a continuous growth in its ecological footprint over time (Global Footprint Network, 2009). The expanding biocapacity deficit is causing a rise in temperatures and unpredictable climatic conditions, which hinders the ability to absorb carbon emissions, decreases biodiversity, disrupts biological cycles, and intensifies natural catastrophes (Okelele et al., 2022). According to the Global economy’s report in 2021, Africa’s contribution to global carbon dioxide emissions from fossil fuels and industry in 2020 is 3.8%. This exceeds a rate of 2%–3% observed in the past two decades. South Africa has the most emissions among the regions, with a total of 439,640 metric tons. Egypt and Algeria follow with 249,370 and 171,250 metric tons respectively. Nigeria ranks fourth, with a total of 115,280 metric tons of emissions (Belloc and Molina, 2023).
Numerous scholars have thus far examined the factors that influence carbon dioxide emissions in the Sub-Saharan African region (Acheampong et al., 2019; Ayompe et al., 2021; Ganda, 2021). Nevertheless, carbon dioxide which constitutes a substantial proportion of greenhouse gas emissions, may not adequately capture, and evaluate the overall extent of environmental deterioration. Consequently, scholars have initiated an investigation into the factors that influence the ecological footprint, a very comprehensive economic-ecological metric used to assess environmental sustainability (Wang and Dong, 2019). The ecological footprint measures the combined impact of human activities on the environment, including pollution of the air, water, and soil. Ecological footprint accounting consists of two metrics: the demand-side ecological footprint and the supply-side biocapacity (Galli, 2015). The ecological footprint measures the amount of natural resources that are needed in global hectares, whereas biocapacity represents the ability of nature to provide these resources using the same unit of measurement. Multiple recent research have examined the factors that influence the ecological footprint in Sub-Saharan Africa, as shown by Wang and Dong (2019), Espoir et al. (2024), and Zhang et al. (2021).
These studies exclusively concentrate on the ecological footprint, disregarding the supply aspect of the ecosystem. To get precise results regarding the enhancement of environmental quality, it is important to take into account the existing environmental resources. Siche et al. (2010) introduced the load capacity factor as a means to improve the environmental evaluation. The load capacity factor measures the ability of an area or country to sustain its people based on their existing way of life. The load capacity factor is determined by dividing the biocapacity by the ecological footprint. With sustainability limit is set at 1, a load capacity below one signifies an unsustainable environmental situation, whereas a number over one suggests a sustainable system (Siche et al., 2010).
Multiple facets comprise the intricate and complex relationship between economic expansion and the natural environment. Although economic expansion can provide many advantages, such as enhanced living conditions and alleviation of poverty, it can also exert a substantial adverse influence on the environment (Sun et al., 2020). For instance, economic expansion frequently depends on the exploitation and utilization of natural resources, such as fossil fuels, minerals, and lumber (Omisore, 2018). This phenomenon can result in the exhaustion of these resources, giving rise to several adverse outcomes, including elevated pricing, energy vulnerability, and the devastation of habitats (Muheirwe et al., 2022). In addition, economic activity, such as manufacturing and transportation, produces a diverse range of pollutants, including air, water, and soil contamination. The presence of these contaminants can exert detrimental effects on human health, ecosystems, and the climate (Olawuyi, 2018). Similarly, the combustion of fossil fuels significantly contributes to the occurrence of climate change. As economies expand, their energy consumption often rises, resulting in elevated levels of greenhouse gas emissions and exacerbating the issue of climate change (Erdogan, 2024). Moreover, there is compelling data indicating that economic progress frequently results in the proliferation of human settlements and infrastructure, hence causing the degradation and loss of natural ecosystems. This can exert a detrimental influence on biodiversity and the provision of environmental services (Jin et al., 2023). Enhanced economic growth can result in heightened water use, hence exerting pressure on freshwater supplies. This might result in a shortage of water, especially in dry places.
It is essential to note, nevertheless, that economic growth and environmental impact do not invariably exhibit a negative association. Several methods exist in which economic growth can be decoupled from environmental degradation. As an illustration, nations have the option to allocate resources towards clean technologies, such as renewable energy and energy efficiency, in order to diminish their ecological footprint (Teng et al., 2023; Ali et al., 2024). In addition, they have the ability to enact policies aimed at safeguarding the environment, such as legislation to control pollution and laws to promote conservation. The Environmental Kuznets Curve (EKC) posits an inverse U-shaped correlation between economic growth and environmental pollution (Erdogan, 2024). According to the EKC, pollution levels exhibit an initial rise during a country’s development phase, followed by a subsequent fall when the country achieves higher levels of prosperity. There is empirical support for the EKC theory, however it should be acknowledged that it is not a flawless framework (Jin et al., 2023). The link between economic growth and the environment is intricate and contingent on the specific circumstances.
Foreign Direct Investment (FDI) can have both positive and negative consequences. Although foreign investment can offer significant financial resources, advanced technology, and specialized knowledge, it can also result in resource-intensive operations or relaxed environmental restrictions if not properly controlled. As such, FDI refers to the transfer of financial resources from one nation (the investing country) to another (the recipient nation) with the intention of initiating or growing a commercial enterprise (Kansanga et al., 2019; Ohemeng et al., 2023). FDI has the potential to generate economic advantages such as the creation of jobs and developments in technology. However, its effects on the natural environment are intricate and varied, covering both good and negative outcomes (Kansanga et al., 2019). The Pollution Haven Hypothesis posits that firms may transfer their polluting sectors to developing nations with less stringent environmental legislation and enforcement, resulting in heightened environmental deterioration in the recipient country (Adebayo et al., 2024). These might manifest as atmospheric and aquatic pollution resulting from industrial activities, soil pollution caused by mining and waste disposal, and the destruction of forests for the exploitation of resources.
Research also indicates that FDI initiatives in sectors such as extractive mining, logging, and intensive agriculture can result in substantial exhaustion of natural resources, including minerals, timber, and freshwater (Aust et al., 2020; Gabor and Sauvat, 2021). This can result in significant and lasting effects on both the environment and the future economic growth of the recipient country. Insufficient management of FDI projects can lead to the implementation of unsustainable practices such as the adoption of intensive agricultural methods, excessive utilization of pesticides and fertilizers, and poor waste management systems (Aust et al., 2020; Latif and Faridi, 2023). These factors can lead to the deterioration of soil quality, the decline of biodiversity, and the contamination of water sources. Moreover, the establishment of essential infrastructure for foreign direct investment initiatives, including road networks, industrial facilities, and power stations, can result in the fragmentation of habitats, the depletion of forests, and the disturbance of natural corridors (Mugabe, 2005). This can result in adverse effects on animal populations and the provision of ecosystem services.
On the contrary, FDI has the potential to impart knowledge and introduce advanced environmental technologies to the recipient nation. This can result in enhanced waste management, improved environmental contamination, and cleaner production processes (Ning and Wang, 2018). This has the potential to enhance the implementation of a more sustainable development paradigm. FDI can sometimes serve as a spur for the recipient nation to enhance its environmental rules and enforcement procedures. These factors can be influenced by the investing country’s more stringent environmental regulations, customer demand for eco-friendly products, or green international treaties (Yu and Li, 2020). Foreign Direct Investment might additionally facilitate the adoption of sustainable resource management techniques in the nation receiving the investment (Li et al., 2022). This can encompass investments in sustainable energy sources, initiatives for restoring forests, and the use of circular economy ideas to minimize waste and optimize resource utilization.
It is also imperative to note that the expanding population and economic ambitions of the SSA region are expected to lead to a rise in resource consumption, as seen by the material footprint. This can exert pressure on the availability of resources and potentially restrict the long-term viability of production. The material footprint quantifies the overall quantity of raw materials that must be extracted in order to satisfy the consumption needs of a given population (Tiamgne et al., 2022). Initially, this footprint exerts a significant influence on the natural environment through the loss of resources (Baninla et al., 2020). The crux of the issue is in our incessant exploitation of resources. Metals, minerals, fossil fuels, wood, and biomass are being extracted at a concerning pace, frequently beyond sustainable thresholds. The process of depletion can result in several negative consequences. Firstly, it can cause habitat loss, where the clearing of forests for purposes such as mining, agriculture, and infrastructure development destroys crucial ecosystems and displaces wildlife (Olawuyi, 2018; Omisore, 2018; Sun et al., 2020; Osei-Owusu et al., 2022). Secondly, it can lead to biodiversity loss, as resource extraction and habitat destruction pose significant threats to numerous species, pushing them towards the brink of extinction. Lastly, depletion can also result in resource scarcity, as the exhaustion of finite resources may cause their prices to rise and access to become unequal, thereby creating instability and inequity (Olawuyi, 2018; Omisore, 2018; Sun et al., 2020; Osei-Owusu et al., 2022).
Additionally, each phase of the material lifecycle produces emissions and pollution, including extraction, processing, transportation, product use, and disposal (Awosusi et al., 2023; Yang et al., 2023). Various forms of pollution can occur, such as air pollution, which is caused by activities like mining, burning fossil fuels, and industrial processes. These activities release harmful gases and particulate matter, which negatively impact air quality and contribute to respiratory illnesses. Another form of pollution is water pollution, which happens when wastewater from processing plants, agricultural runoff, and improper waste disposal contaminate water bodies. This contamination harms aquatic life and poses risks to human health (Olawuyi, 2018; Omisore, 2018; Sun et al., 2020; Osei-Owusu et al., 2022). Additionally, greenhouse gas emissions are a significant concern. The extraction and use of fossil fuels are major contributors to climate change, resulting in rising temperatures, extreme weather events, and sea level rise. In addition, the disposable culture resulting from human activities produces vast quantities of garbage, which frequently find their way into landfills. Landfills contribute to various environmental issues. Firstly, they occupy valuable land, which is often located in ecosystems that are already compromised. Secondly, pollutants from landfills can seep into the soil and groundwater, leading to leachate contamination. This contamination can have negative impacts on nearby communities and ecosystems. Lastly, the decomposition of organic matter in landfills releases methane, which is a potent greenhouse gas that contributes to climate change. These issues have been documented in studies by Marcelino et al. (2023), Sun et al. (2023), and Vats et al. (2023).
Through facilitating innumerable activities and establishing global connections, information and communication technologies (ICT) have brought about a paradigm shift in our society. Nevertheless, ICT has both positive and negative impacts on the environment. ICT equipment, including sensors, drones, and satellite photos, offer real-time data on deforestation, pollution levels, and wildlife populations, which is valuable for environmental monitoring and control. This data enables us to oversee alterations in the environment, monitor illicit activities, and make well-informed choices for the preservation and sustainable management of resources. Furthermore, ICT plays a pivotal role in enhancing the efficiency of energy systems. Smart grids employ sensors and communication networks to control electricity consumption and include renewable energy sources such as solar and wind power, therefore diminishing dependence on fossil fuels and lowering greenhouse gas emissions (Li X. et al., 2023; Mehmood et al., 2023). In addition, via the utilization of data collected from sensors and artificial intelligence, farmers may enhance the efficiency of water consumption, application of fertilizers, and management of pests, therefore minimizing the environmental impact of agriculture and maximizing crop productivity. Consequently, this can result in an increase in the sustainability of food production and a reduction in the degradation of land. In addition, ICT facilitates teleconferencing, online meetings, and remote work, hence diminishing the necessity for traveling and the resulting transportation emissions (Mapiye et al., 2023). E-commerce has the potential to minimize the environmental consequences of conventional physical retail by streamlining the transportation of goods and decreasing the amount of packaging waste generated (Aydin and Degirmenci, 2023). ICT platforms have the capacity to enhance public knowledge of environmental concerns, facilitate the organization of communities, and establish connections among individuals engaged in the development of sustainable solutions (Ni et al., 2022). This has the potential to generate public backing for environmental policies and initiatives.
Conversely, the swift obsolescence and increasing popularity of electronic gadgets result in a substantial quantity of e-waste, frequently including dangerous substances (Maphosa and Maphosa, 2020). The incorrect disposal of electronic trash contaminates landfills, releases harmful substances into the environment, and presents health hazards. Similarly, data centres, server farms, and the extensive network infrastructure that supports information and communication technology necessitate substantial energy consumption, frequently derived from non-renewable sources such as fossil fuels (Noor et al., 2018). ICT activities result in the release of greenhouse gases and the polluting of the air (Warner and Jones, 2018). In addition, the production of ICT devices such as smartphones and computers involve the extraction of rare earth minerals and other resources, which contributes to environmental deterioration at mining sites and may result in interruptions in the supply chain. Furthermore, spam emails, cryptocurrency mining, and online advertising collectively contribute to the phenomenon known as “digital pollution,” which involves the storage and transfer of data (Oo et al., 2023). This, in turn, results in heightened energy consumption and the subsequent negative environmental consequences.
Drawing from the preceding discourse, the objective of this study is to examine the ways in which FDI, economic growth, material footprint, and ICT impact the load capacity factor. The study’s unique methodology yields significant advancements in the field of sustainable development. Firstly, this paper present concrete proof regarding the influence of FDI, economic growth, material footprint, and ICT on environmental sustainability. Therefore, this study takes into account both the demand for resources (ecological footprint) and the available supply (biocapacity) to offer a more comprehensive understanding, surpassing the use of either metric in isolation. The load capacity factor is the statistic used to evaluate environmental sustainability in the economies of Sub-Saharan Africa. Second, as far as we are aware, this study is the initial investigation into the associations and causation connections among the variables in these particular nations. The matter is of utmost significance due to the fact that a significant number of emerging nations are in dire need of sustainable economic growth, which is widely thought to foster the establishment of an environmentally friendly economy. Thirdly, the focus on Sub-Saharan African nations is justified due to the scarcity of research on the influence of these variables on load capacity factor, especially in developing and rising economies. Given the recent prominence of load capacity factor in Sub-Saharan Africa, the results of this study have great importance in influencing environmental policy in nations within the region.
Fourthly, this study will assist in identifying the factors that contribute to the preservation of natural environments in Sub-Saharan African countries, despite the region’s developmental challenges. This is crucial because these countries bear a significant burden in terms of the costs associated with natural resource exploitation, environmental degradation, and climate change. By understanding these elements, policymakers can develop effective strategies to sustain these environments and prevent further deterioration. Fifth, this study encompassed a total of 37 nations located in the Sub-Saharan African region, spanning from 1970 to 2019. The anticipated outcomes of this longitudinal study have the potential to offer evidence-based guidance for addressing the challenges faced by Sub-Saharan Africa in relation to contaminated foreign direct investment, disparities in wealth distribution, inefficiencies in resource management, and issues with information and communication technology, with the ultimate goal of achieving the sustainable development goals. If practical policy measures are not implemented to restrict the over exploitation of the natural environment in Sub-Saharan Africa, it will hinder its economic progress. Sixth, the study has the potential to offer significant data and perspectives that may be used for comparison analysis with other emerging regions. This might enhance our comprehension of the worldwide problems and prospects for attaining environmental sustainability in various circumstances.
The rest of this study provides a thorough literature review for the subsequent section. The third portion of the study consists of a comprehensive explanation of the data and methodology employed, which is then followed by the presentation of the findings. Thus, the empirical data are analysed in section four, while the conclusions, together with specific policy implications, are presented in the concluding section.
This section examines the existing research on the load capacity factor and its influencing parameters, including material footprint, foreign direct investment, economic growth, and ICT. This discussion focuses on four main relationships: the link between foreign direct investment and load capacity factor, the association involving material footprint and LCF, the connection between economic development and load capacity factor, and the relationship between ICT and load capacity factor.
The initial body of literature centres on research that investigates the relationship between foreign direct investment and load capacity factor. The study conducted by Yıldırım et al. (2024) examines the impact of FDI on the load capacity factor in BRICS states from 1992 to 2020. The findings reveal that FDI leads to a decline in the load capacity factor. The study conducted by Ayad et al. (2023) found no evidence of an N-shaped association between foreign direct investment and load capacity factor in 16 Middle East and North African states from 1990 to 2018. Adebayo et al. (2024) has confirmed that in Thailand, there is a one-way causal relationship from foreign direct investment to LCF across all quantiles analysed from 1975 to 2018. Furthermore, the effect of FDI in decreasing LCF provided evidence in favour of the pollution haven hypothesis (PHH). Xu et al. (2022) emphasize that financial globalization positively impacts Brazil’s load capacity factor. Therefore, policymakers should capitalize on this opportunity by formulating policies that promote the openness of the economy to international investors. Nevertheless, Wang et al. (2023) emphasize that FDI exhibits substantial adverse associations with LCF, aligning with the ongoing progress in the surveyed 96 developing countries. These nations are presently undergoing economic expansion and require industrial development and fuel consumption to drive their economic growth. Consequently, this situation leads to detrimental effects on environmental conditions. According to Latif and Faridi (2023), foreign direct investment has led to a decline in the environmental load capacity factor in 48 Asian nations between 1996 and 2020.
The second branch of literature is on research that investigate the relationship between material footprint and load capacity factor. Pata et al. (2023) employed the Fourier ARDL model to examine the impact of biomass energy on the LCF in the United States from 1965 to 2018. The findings of their study indicate that biomass energy has a positive effect on LCF. In addition, Fareed et al. (2021) argues that export diversification in Indonesia enhances the load capacity factor, hence promoting environmental quality. According to Awosusi et al. (2023), the utilization of renewable energy has a beneficial impact on the LCF in Japan. Yang et al. (2023) conducted a survey on the BRICS nations from 1990 to 2018 and found that natural resources contribute to a decrease in the load capacity factor. Awosusi et al. (2023) utilized a dataset spanning from 1980 to 2017 to examine the situation of South Africa. Their findings indicate that non-renewable energy sources have a detrimental and statistically significant impact on load capacity parameters, both in the short and long term. Hence, the utilization of finite energy sources is the predominant catalyst for environmental degradation in South Africa. However, Butt et al. (2023) adds that a shadow economy considerably reduces environmental pollution.
The third strand of literature pertains to studies that analyse the relationship between economic growth and load capacity factor. Djedaiet et al. (2024) argue that there is a U-shaped link between GDP and LCF in the seven African oil-producing OPEC nations from 1990 to 2018 thereby offering empirical evidence to support the LCC hypothesis in African nations that produce oil. This finding indicates that in the initial phases of development, economic expansion has a detrimental impact on the environment. However, the impact turns favourable after it surpasses a threshold of 8806 or 6674.17 USD per capita. According to Erdogan (2024), there is a relationship between economic growth and LCF that follows an inverted-U shape in the case of 13 African nations from 1992 to 2020, thereby displaying evidence of an inverted load capacity curve. Ali et al. (2024) use the G20 nations panel from 1994 to 2018 to demonstrate that there is a negative correlation between economic growth and LCF across all quantiles, with the most significant impact shown at the 90th quantile. Therefore, in these nations, the expansion of the economy leads to environmental deterioration due to a decrease in the level of natural capital. Similarly, Teng et al. (2023) argue that economic expansion has a detrimental impact on the dynamics of load capacity, leading to environmental deterioration in significant nuclear power nations including France, the USA, Canada, China, and Russia between 1990 and 2021. Similarly, Jin et al. (2023) demonstrates that there is a statistically negative link between economic development and LCF in the long run. This reveals that the economic expansion in Germany presents ecological issues, resulting in detrimental ecological effects. The study utilizes data from 1974 to 2018.
The last branch of literature is on the investigations that explore the connection between ICT and load capacity factor. Dogan and Pata (2022) provide evidence that from 1986 to 2017, ICT has a positive influence on LCF for the G7 nations. In a similar vein, Li X. et al. (2023) report that the long-run results demonstrate that LCF was strengthened by the reliance on digitization in certain Next Eleven economies between 1990 and 2018. According to Li L. et al. (2023), ICT has a positive impact on the connection with LCF in both the short-term and long-term for the G-20 nations between 1995 and 2018. Aydin and Degirmenci (2023) reveal that the long-term outcomes at the country level indicate ICT has a substantial and positive coefficient impact in Portugal and Spain, leading to a rise in LCF in these countries. The analysis is based on data from 1990 to 2018, focusing on sampled European Union nations. The study conducted by Mehmood et al. (2023) examines data from 1990 to 2018 on the economies of the G8 countries and demonstrates that ICT has positive effects on the natural environment’s health. This is because the load capacity factor helps in monitoring environmental health by clearly showing the difference between ecological footprint and biocapacity. Ni et al. (2022) also agree that in high-resource-consuming economies between 1996 and 2019, the long-term findings indicate that dependence on digitalization enhanced LCF. Ramzan et al. (2024) posit that adoption of biomass and hydro energy significantly reduce emissions and play a crucial role in achieving carbon neutrality. Thus, integration of green energy sources could improve ICT.
This research addresses a number of potential voids in the literature review of empirical peer-reviewed studies, as is evident from the studies examined. Although the dependent variable, load capacity factor, has been extensively examined in industrialized economies, its application to Sub-Saharan Africa has received less attention. This study aims to address a significant knowledge vacuum by investigating the precise elements that influence LCF in this distinct setting. It will specifically analyse issues distinctive to the region, where matters such as poverty, informality, and governance challenges are also inherently prevalent. Secondly, current studies on FDI and its effects on the environment mostly concentrate on advanced economies or particular industries, resulting in a lack of comprehensive knowledge on FDI’s environmental consequences in Sub-Saharan Africa within the framework of LCF. This study aims to enhance comprehension of how FDI influences environmental sustainability in Sub-Saharan Africa. It acknowledges that FDI may have both favourable and unfavourable consequences, contingent upon its characteristics and governance. Thirdly, the significance of information and communication technology in environmental management and resource efficiency is increasingly being recognized. However, its connection with LCF in Sub-Saharan Africa has not been well investigated. This study aims to investigate the potential impact of ICT adoption on enhancing environmental monitoring, data analysis, and sustainable resource management methods in the region.
Fourthly, an integrated analysis of foreign direct investment, material footprint, economic growth, and ICT in Sub-Saharan Africa is lacking. Prior research tends to examine these aspects individually. This study aims to provide a comprehensive examination of the interplay between FDI, material footprint, economic growth, and ICT within the framework of the LCF. By doing so, it seeks to enhance our knowledge of how these factors collectively affect environmental sustainability. Finally, although there are many studies on environmental concerns in Sub-Saharan Africa, there is a lack of policy-oriented research that effectively transforms information into practical suggestions. This study aims to address this gap by identifying crucial policy mechanisms and interventions through the examination of FDI, material footprint, economic development, and ICT within the context of the LCF. By rectifying these deficiencies, the research has the potential to make a substantial contribution to the current pool of information and provide valuable insights for future studies, policy-making, and sustainable development initiatives in Sub-Saharan Africa.
Presently under analysis are the material footprint, foreign direct investment, economic growth, and information and communication technology (ICT) as they relate to environmental quality as measured by load capacity factor in 37 Sub-Saharan African nations. The selection of the nations included in the analysis was based on the convenience of data accessibility. A comprehensive dataset covering the period from 1970 to 2019 was used to evaluate these connections. The load capacity factor is the dependent variable, whereas material footprint, economic growth, ICT, and foreign direct investment are the independent factors. To mitigate the issues of serial correlation and heteroskedasticity, the study transformed all the variables into their natural logarithm forms. Table 1 displays the characteristics and origins of the variables included in the study.
This study examines the effects of Foreign Direct Investment, Material Footprint, and Information and Communication Technology on the Load Capacity Factor in Sub-Saharan African nations. The data used in this research is sourced from globally recognized public domain sources, as shown in Table 1. Given the specific focus on Sub-Saharan African nations, this study utilized a purposive sample approach to include all countries within that region. It is crucial to note that the unique exclusion criterion selected for this study is restricted data availability for some nations. This means that not all countries in this region are included in the study.
It is imperative to also note that there exist possible biases and restrictions. First and foremost, there are apprehensions over the accessibility of data. These public databases contain restrictions on the extent of information offered for each indication. For example, the WDI database may not have FDI statistics categorized by certain sectors, which might affect this paper ability to analyze the precise impact of FDI in certain businesses. Moreover, this paper addresses concerns around data harmonization. Therefore, although these databases are considered reliable, there may be discrepancies in the methods of data collection and reporting across various countries.
Therefore, in order to guarantee the quality and dependability of this data, this study executed the further measures. Firstly, the study conducted a comparison of data from several sources to ensure consistency and detect and rectify any outliers within the data sets. The data is free from missing numbers or errors as it only includes nations with consistently available data. In addition, this article included extensive descriptive statistics for every variable utilized in this analysis, as seen in Table 2. This aids in evaluating the scope, average value, and spread of the data, perhaps uncovering any irregularities.
In formulating the econometric methodology, this analysis incorporates sophisticated econometric techniques. This process consists of many sequential phases. The cross-sectional dependence test is employed to initiate the assessment of the common stock impacts in the study (Pesaran, 2004). Furthermore, the CIPS and CADF unit root technique, as proposed by Pesaran and Yamagata (2008), is utilized to examine the stationary uniqueness of every parameter. Moreover, the cointegration connection between the variables is evaluated using two panel tests conducted by Kao and Pedroni (Kao, 1999; Pedroni, 1999). As well, the study employed D-OLS and FM-OLS methodologies to examine the enduring associations between the variables under investigation. In addition, the study employed the second generation Dynamic Seemingly Unrelated Regression (DSUR) as a means of ensuring the reliability and accuracy of the results (Mark et al., 2005). Additionally, the study utilized the Dumitrescu and Hurlin causality test (Dumitrescu and Hurlin, 2012) to determine the causal link between the variables examined in the study Eq. 1. The subsequent equation elucidates the conceptual framework of the investigation: Following Fareed et al. (2021), Ganda (2021), Latif and Faridi (2023), and Mehmood et al. (2023) the subsequent equation elucidates the conceptual framework of the investigation
In the regression [1],
In the beginning of this analysis, the presence of cross-sectional dependence (CD) on the variables estimated was ascertained using the cross-sectional dependence (CD) test. At the start of panel data analysis, a thorough and rigorous assessment of the CD is conducted. In order to obtain the most reliable and suitable information, the present evaluation utilizes the cross-section independence test introduced (Pesaran, 2004) Eq. 2. Therefore, it is frequently presumed that the error terms incorporated in cross-nation panels lack cross-sectional dependence. Nevertheless, there is potential evidence indicating the existence of cross-sectional dependence (CSD) in the panel data model. Failure to include cross-sectional dependence (CSD) during estimating might result in inconsistent errors in the estimations (Andrews, 2005). Hence, when calculating the correlation coefficient, it subtracts the mean values. The null hypothesis of the CD test posits that there is no presence of CD in the cross sections. The equation for the CD may be arranged in the following manner:
Thus, model [2] suggests that the error term for individual, i, is influenced by the error term of another individual, j, capturing the cross-sectional dependence. The test statistic follows a two-tailed standard normal distribution, N (0, 1), when the sample size (N) approaches infinity and T is adequately big. Due to the increasing economic interconnectedness of nations, there is a high likelihood of significant interdependencies among cross countries, particularly when the cross-section (N)- in this study 37 or time (T) dimension-in this study 50, is considerable (De Hoyos and Sarafidis, 2006).
The cointegration trajectories of the considered parameters are examined as the subsequent step following the inspection of the CDs. The findings of the first-generation unit root test may be skewed if the correctional units are not interdependent. In this study, the researchers utilized the Dickey-Fuller cross-sectionally augmented Dickey-Fuller (CADF) and Im-Pesaran-Shin cross-sectionally augmented CIPS tests (Pesaran, 2007) Eq. 3. The CADF test statistics, which assesses the stationarity of the panel series, is derived as follows:
Thus, model [3] show the CADF test statistic which is a value computed to ascertain if a time series parameter is stationary or non-stationary across multiple cross-sections (individuals). The CADF denotes a classification of IPS unit root tests that establishes the presence of the CSD. The overall statistics of this test are calculated by averaging the unit-root statistics for each nation or cross-section in the CADF and CIPS tests. The null hypothesis (H0) suggests that the panel possesses a unit root, whereas the alternative hypothesis (H1) suggests that the panel is stationary Eqs 3–6.
The study also employed the CIPS (Cross-Sectionally Augmented IPS) tests. CIPS possesses the capacity to handle the CSD, therefore delivering outcomes that are more dependable and precise. The CIPS test equation is organised as:
Hence, model [4], involves estimating individual-specific Augmented Dickey-Fuller (ADF) regressions for each cross-sectional unit by including the lagged cross-sectional averages of the dependent variable to account for cross-sectional dependence. It follows that
Thus, model [5] shows the CIPS test statistic which is the average of the ADF t-statistics for the coefficients across all individuals. The existence of a long-run or multiple long-run relationship between two or more non-stationary time series variables constitutes cointegration. Researchers conduct these tests to determine whether two or more non-stationary time series exhibit a stable, long-term equilibrium connection. In order to determine the long-term relationship between the variables in the panel series, this study utilized the panel co-integration tests developed by Pedroni (1999) and Kao (1999). Both tests posit the null hypothesis of no co-integration among the variables. In order to examine the long-term relationship, Pedroni (1999) analysed the co-integrating equation shown below:
In the above regression, the variables T range from one to T and N range from one to N. In this context, T represents the total number of observations, whereas N is the total number of countries in the panel. The
The panel co-integration test has been conducted using seven test statistics to examine the null hypothesis of no co-integration. Out of these test statistics, four (v-statistic, rho-statistic, PP-statistic, and ADF-statistic) rely on ‘within-dimension’ statistics. The next three test statistics (group ρ-statistic, group pp statistics, and group ADF-statistic) rely on ‘between-dimension (group)' statistics. The complete tests are conducted on calculated residuals acquired from the aforementioned co-integrating equation. Hence, the presence of cointegration is founded on residuals test given as
In addition to the seven tests proposed by Pedroni, we will also use the Kao (1999) test, which assumes homogeneity throughout the sample, to check the robustness of our findings. The cointegration test proposed by Eq. 7 Kao (1999) may be expressed as
Therefore, model [7] is created to ascertain if a group of non-stationary time series factors share a long-run equilibrium association within the SSA panel data scenario. In this situation,
The investigation utilized the D-OLS and FM-OLS methodologies to assess the long-term interrelation among the variables under study. The primary rationale for selecting these models is to address the endogeneity concerns and effectively manage the presence of serial correlation within their error terms Eq. 8. The D-OLS and FM-OLS equations can be precisely specified in the following manner: Following Hansen and Phillips (1990), the D-OLS and FM-OLS equations can be precisely specified in the following manner
Thus, model [8], estimate long-run relationships between non-stationary variables in the context of cointegration. Both D-OLS and FM-OLS address the issues of endogeneity and serial correlation that often arise in cointegration analysis. In this case,
In this context, the long-run stationarities matrix is denoted by
Therefore, the drawback of D-OLS and FM-OLS methods is that they fail to account for the correlation between panel sections, which may result in estimation outcomes that are not accurate. In order to overcome this limitation, we employed the dynamic seemingly unrelated regression (DSUR) technique proposed by Mark et al. (2005). This methodology addresses both the presence of diverse characteristics and the interdependence among different sections of data. It is particularly well-suited and efficient for situations when the number of observations (N) is fewer than the duration of the study (T). We utilized the following estimating regression for DSUR estimator, as described by Eq. 9 Mark et al. (2005):
Thus, model [9], describes the DSUR estimator that is obtained by jointly estimating all equations simultaneously, taking into account the potential correlations between the error terms. This is normally conducted by employing maximum likelihood or generalized least squares approaches. The variable
The article’s use of D-OLS, FM-OLS, and DSUR models exemplifies a comprehensive methodology for examining the effects of FDI, Material Footprint, and ICT on the Load Capacity Factor (LCF) in Sub-Saharan African nations. The justification for employing Dynamic Ordinary Least Squares (D-OLS) is its applicability in analysing time series data, which is the type of data utilized in this work (specifically, data spanning from 1970 to 2019). It accounts for possible autocorrelation, which is the association between error terms over time, that can provide misleading regressions with erroneously significant findings. Therefore, D-OLS offers a robust basis for this study.
The reason for using the Fully Modified Ordinary Least Squares (FM-OLS) is that it is a reliable alternative to D-OLS, especially when dealing with possibly endogenous variables (variables that are impacted by the dependent variable, LCF, in this case). It adjusts for the possibility of endogeneity bias, resulting in more dependable estimates. FM-OLS enhances this approach by effectively dealing with problems related to endogeneity.
The justification for employing Dynamic Seemingly Unrelated Regressions (DSUR) is in its capacity to effectively analyze numerous equations concurrently, particularly when confronted with possibly interrelated error terms across equations. These factors may be interconnected if the effects of FDI, Material Footprint, and ICT on LCF may mutually influence one another. Therefore, DSUR offers a thorough representation by taking into account any interconnections among the variables.
To sum-up, the D-OLS method enhances the FM-OLS and DSUR approaches by specifically dealing with the issue of autocorrelation. Although FM-OLS and DSUR are generally resilient, D-OLS particularly addresses the issue of linked error terms over time, which can potentially result in misleading findings. Furthermore, the FM-OLS method enhances the D-OLS and DSUR approaches by effectively dealing with endogeneity. The D-OLS and DSUR models may not adequately consider cases in which independent factors, such as Foreign Direct Investment, might be impacted by the dependent variable, namely, LCF. FM-OLS explicitly addresses the problem of endogeneity, resulting in more accurate estimations of the actual influence of each element. Furthermore, the DSUR enhances the D-OLS and FM-OLS methodologies by taking into account interdependencies. While D-OLS and FM-OLS concentrate on examining individual connections, DSUR enables the simultaneous analysis of a system of equations. For instance, it is essential to consider potential spillover effects between the independent variables, such as FDI impacting ICT, which in turn affects LCF.
While offering long-run coefficients, the D-OLS, FM-OLS, and DSUR models fail to quantify the directional relationship between variables. Therefore, in order to address this problem, the research employed the panel causality analysis introduced by Eq. 10 Dumitrescu and Hurlin (2012). The null hypothesis of the causality model posited that there is no statistically significant causal relationship between the variables under examination. The equations for the Dumitrescu and Hurlin causality test are organised in the manner:
Hence, model [10], outlines the underlying equation for the Dumitrescu and Hurlin (2012) test which is similar to the standard Granger causality regression for each individual panel unit. The sign
From Table 2, LogFDI has the lowest observed minimum value amongst the variables which is −341.82% significantly lower than its mean, suggesting a large variation in FDI levels. LogGDP has the highest observed maximum value amongst the parameters which is about 454% higher than its mean, reflecting a wide range of economic output levels. LogFDI have the highest standard deviation value, suggesting more dispersed distributions, whereas LogMFC has the lowest standard deviation value, indicating a more concentrated distribution. LogGDP show the highest mean value which reflects a high average level of economic output, indicating a productive Sub-Sharan African economy overall. LogICT possess the least mean value which illustrates that the average level of information and communication technology is below its midpoint (max + min value/2), suggesting potential for further development or adoption of ICT in the Sub-Saharan African region. It is noted that all the variables have kurtosis is greater than 3, it indicates a distribution with heavier tails than a normal distribution. More specifically, LogLCF, LogMFC and LogICT have mesokurtic distributions while LogGDP and LogFDI demonstrates extremely leptokurtic distributions. This means that more values of all variables are concentrated around the mean (the central peak of the distribution) compared to a normal distribution. Thus, there is a higher likelihood of extreme values (outliers) in the tails of the distribution.
For panel datasets, the order at which load capacity factor, material footprint, economic growth, FDI, and ICT become stationary is determined utilizing the Augmented Dickey–Fuller–Fisher (ADF–Fisher) and Im–Pesaran–Shin (IPS) stationary tests. The outcomes of the two examinations documented in Table 3 exhibit resemblance. Both the IPS and ADF-Fisher tests indicate that all the variables exhibit first-order integration. Therefore, although variables LogMFC and LogFDI may have integration at a level I (0), this results in all variables having integration at a level I (1) in both tests.
After establishing the order of stationarity, the study proceeds to explore the Cross-Sectional Dependence (CD) test. The verified outcomes of the CD test are presented in Table 4.
The statistical results of the cross-sectional dependence test conducted by Pesaran (2004) indicate that all variables are significant in Table 4. This rejects the null hypothesis at a significance level of 1% and provides evidence of dependence among the economies that were selected from Sub-Saharan Africa (SSA). In summary, we have identified the presence of CD in the SSA nations for the variables that were analysed. Thus, it is important to emphasize that this form of dependency arises when the error terms of distinct units are not mutually independent, as shown by their correlation. This implies that they vary together in a manner that may not be accurately reflected by the estimated model.
Following this, the homogeneity of the slope of parameters is examined using a test proposed by Pesaran and Yamagata (2008). The null hypothesis of the test supports the idea that the slope parameters are the same for all cases, whereas the alternative hypothesis proposes that the slope parameters vary between cases. The results presented in Table 4 indicate that the p-values are statistically significant, which means that we may reject the null hypothesis with a 1% level of significance for values of delta and modified delta. Furthermore, these results demonstrate that the slopes of the parameters are heterogenous. In the next step, this article presents the results of the second-generation tests.
The second-generation tests are vital since a common assumption in panel data models is that the error components are independent across different groups of data. The empirical findings shown in Table 4 indicate that, with a significance level of 1%, we should reject the null hypothesis of cross-sectional independence in our panel for our series. In order to remove this form of dependence, the conventional DF (or ADF) regressions are enhanced by using the cross-section averages of previous levels and first differences of the individual series (known as CADF statistics). Furthermore, this article incorporates the CIPS tests developed by Pesaran to improve and/or support robustness of the study outcomes.
For the critical second-generation panel unit root approaches, we employed CIPS and CADF unit root assessments. The findings of the CIPS and CADF evaluations are presented in Table 5, indicating that the variables examined in this study are stationary after being first differenced. In this regard, the CIPS unit root tests indicate that all variables, except for LogLCF and LogMFC, are non-stationary at the level. However, when taking the first difference, all variables become stationary at a 1% significance level. Similarly, the CADF test statistics show that most variables are nonstationary at level (except for LogLCF, LogFDI, and LogMFC). However, when we take the first difference of all the variables, they become stationary, refuting the hypothesis that there is a unit root. Both of these tests demonstrate that all of the variables analysed for the thirty-seven SSA nations are part of category I (1). The next section assesses if the variables have a long-run association.
Table 6 contains the outcomes of the panel cointegration test. In order to examine the long-run association between material footprint, foreign direct investment, economic growth, information and communication technology, and load capacity factor, all of these factors were incorporated into the panel cointegration test. The outcomes of both the Pedroni cointegration test and the Kao cointegration test were congruent. More precisely, Kao (1999) specifically examines residual cointegration under the null hypothesis that there is no cointegration. The results in Table 6 indicate that there is a significant cointegration among the variables utilized in this analysis for the SSA nations investigated, at a 1% level of significance. These findings indicate the presence of long-run connections among the variables. In addition, we also do the Pedroni panel cointegration tests (Pedroni, 1999, Pedroni, 2004) as an alternative method. The Pedroni panel cointegration test utilizes several test statistics to examine the null hypothesis of the absence of cointegration. The results in Table 6 indicate that eight out of eleven test statistics for the SSA are statistically significant, while only three test statistics demonstrate lower levels of significance. The Pedroni panel cointegration tests strongly indicate the existence of a cointegration connection. Overall, the results of the two cointegration tests indicate the presence of a long-term link between the variables for the 37 economies in Sub-Saharan Africa from 1990 to 2015. In order to conduct a more in-depth analysis of this enduring and consistent association, we employed FM-OLS, D-OLS, and DSUR estimates in the subsequent phase.
Table 7 shows that the coefficient estimates for material footprint, foreign direct investment, economic growth, information and communication technology, in relation to load capacity factor were extracted using the FM-OLS, D-OLS, and DSUR techniques. Thus, the Pedroni and Kao tests only confirmed cointegration and did not provide any indication regarding these coefficients. Concerning material footprint this paper results demonstrates that the variable develops a significantly positive association with load capacity factor in all the three models for the studied SSA economies. Following this, a high load capacity factor indicates, in the context of sustainability, that a nation’s resource consumption and waste production are in close proximity to its natural regenerative capacity. This is typically unfavourable since it signifies ecological strain and perhaps unsustainable behaviours. Hence, the outcome described can be elucidated by several factors inside the SSA. The primary factor contributing to resource strain in SSA is the escalating consumption of raw materials, also known as the material footprint. This is because the increased usage of resources directly corresponds to a rise in demand for these resources (Baninla et al., 2020). This can exert stress on the environment and ecosystems, perhaps beyond their capability to regenerate (carrying capacity).
Alternatively, the positive correlation may be attributed to the influence of economic growth and the extraction of resources in Sub-Saharan Africa. Constructing roads, dams, and other infrastructure in the SSA region generally necessitates substantial material resources, hence contributing to the total material footprint (Tiamgne et al., 2022). Nevertheless, these initiatives can also enhance the country’s potential to efficiently utilize its resources, resulting in an increased load capacity factor. Furthermore, trade patterns and reliance on resources may also have an impact on the connection. Sub-Saharan African nations significantly depend on the exportation of raw resources, resulting in a potentially greater material footprint as a result of extraction and processing operations (Osei-Owusu et al., 2022). This might also enhance the load capacity factor, as the country’s economy is strongly linked to the usage of its natural resources.
This paper’s findings also illustrate that economic growth depicts a significant and negative relationship with load capacity factor in SSA economies. This was confirmed in all the three econometric methods. Hence, according to this interpretation, as Sub-Saharan African economies undergo economic expansion, they place greater emphasis on short-term wealth-generating endeavours, irrespective of the potential negative impact on environmental sustainability (Sun et al., 2020). This may result in the over utilization of natural resources and a reduction in carrying capacity, so diminishing the environment’s capability to regenerate and sustain its present level of output. The negative association may also indicate inefficient or unsustainable resource management techniques in SSA (Omisore, 2018). Therefore, economic progress may not be translating into increased usage of resources, leading in waste and environmental deterioration, eventually reducing the load capacity factor.
Moreover, environmental regulations and enforcement are reportedly lax in SSA economies; consequently, economic actors prioritize profit over environmentally responsible practices (Muheirwe et al., 2022). This may result in unregulated exploitation of resources and contamination, hence exacerbating the degradation of the environment’s carrying capacity. Likewise, the economic expansion in countries of Sub-Saharan Africa (SSA) may be focused on companies that significantly depend on extracting resources or engage in activities that have significant environmental consequences, such as deforestation and fossil fuel extraction (Olawuyi, 2018). This dependence can result in excessive exploitation and diminished load capacity.
This research also confirms that foreign direct investment is significantly negative associated to load capacity factor for the SSA countries from at least two of the models. This research confirms that certain foreign investors in Sub-Saharan African nations may put immediate financial gain ahead of environmental sustainability (Aust et al., 2020). This may entail the utilization of natural resources in an unsustainable manner, resulting in environmental deterioration and a decrease in the total load capacity. These activities may involve the clearing of forests for farming or extracting resources without sufficient measures to protect the environment. Similarly, FDI has the potential to replace current, environmentally friendly practices in nearby areas (Gabor and Sauvat, 2021). For instance, several economies in Sub-Saharan Africa might implement large-scale farming operations that utilize resources intensively, therefore replacing traditional farming systems that are more effective in conserving the environment (Kansanga et al., 2019). This transition may lead to a long-term decline in the land’s capacity to sustain economic activity.
Furthermore, empirical data suggests that host nations in Sub-Saharan Africa do not possess comprehensive environmental regulations and effective enforcement systems, which in turn encourage foreign direct investment that places a higher value on financial gain than environmental stewardship (Gossel, 2018; Ohemeng et al., 2023). The absence of supervision might lead to unsustainable activities and ultimately decrease the load capacity factor. In a related context, FDI in SSA is primarily focused on sectors such as extractive industries and heavy manufacturing, which are inherently resource-intensive and can lead to environmental damage (Mugabe, 2005). The emphasis on certain sectors may account for the inverse correlation with load capacity factor.
This study also adds that ICT has a significantly negative link with load capacity factor for the studied SSA economies. Under these conditions, although ICT may result in resource optimization and efficiency gains, these advantages may not be uniformly disseminated among all sectors and populations in the economies of Sub-Saharan Africa (Mapiye et al., 2023). For instance, the adoption of ICT in specific industries and urban regions in SSA could lead to enhanced resource efficiency. However, rural areas and traditional sectors may not reap the full advantages of this adoption (Sennuga et al., 2020). Consequently, this discrepancy may put a strain on existing resources and surpass the environment’s capacity to sustain these activities, thereby reducing the load capacity factor. It is also important to recognize that the construction and upkeep of ICT infrastructure, such as block chains, data centres, and communications networks, necessitates substantial energy resources (Noor et al., 2018).
Nevertheless, the energy sources powering this infrastructure in SSA nations are neither renewable nor sustainable (Warner and Jones, 2018). Therefore, the growing energy demand resulting from the use of ICT might contribute to environmental deterioration and decrease the total load capacity factor. It is also crucial to highlight that the fast obsolescence of electronic gadgets and the increasing amount of electronic waste linked to ICT might present substantial environmental difficulties if not handled in a sustainable manner in Sub-Saharan Africa (Maphosa and Maphosa, 2020). Hence, incorrect disposal of electronic trash can contaminate ecosystems and exhaust resources, hence exacerbating the decline in the environment’s ability to sustain life.
This study utilizes various estimate approaches (FM-OLS, D-OLS, and DSUR) that have different strengths and drawbacks. This approach offered a more thorough understanding of the connection between the variables. The results show consistency across these three methodologies, hence bolstering the trust in the conclusions of this research and diminishing the probability of erroneous results. FM-OLS improved the correction for heteroskedasticity and autocorrelation, which guaranteed accurate standard errors and valid inference. This further substantiated the argument against fortuitous outcomes. The D-OLS technique facilitated the consideration of endogeneity, therefore preventing the formation of erroneous conclusions on causal links. The consistent outcomes obtained by D-OLS indicated that the conclusions of this article are not mainly influenced by reverse causality or omitted factors. Ultimately, the use of the DSUR allowed for accurate estimation of several equations while accounting for cross-sectional dependency, hence enhancing the credibility of the findings in this work, particularly when the objectives of our study comprised interconnected statements.
Finally, the results of the DH Granger causality test are displayed in Table 8. The data indicate that there is a two-way causal relationship between material footprint, GDP, ICT, and load capacity factor in the chosen economies of Sub-Saharan Africa. The data limitations surrounding the causality method prevent the availability of findings for FDI. Therefore, every decision-maker strategy that earmark to control these factors affects the load capacity factor and vice versa.
The results of the Granger causality analysis, which are displayed in Table 8, indicate that material footprint, economic growth, and ICT have the potential to predict load capacity factor and were substantial determinants of load capacity factor in the economies of Sub-Saharan Africa during the specified time period. The results align with the conclusions derived from the FM-OLS, D-OLS, and DSUR investigations. Furthermore, our research findings provide evidence for a reciprocal influence in the indicated associations. This result underscores the evident and well-supported connections between these factors and stresses the necessity of taking their relationship into account when developing strategies that address load capacity factor challenges in the economies of Sub-Saharan Africa.
At this critical juncture in its development trajectory, Sub-Saharan Africa finds itself. The task of reconciling economic expansion with environmental preservation is an urgent and intricate undertaking that requires a sophisticated comprehension of the intricate interplay between economic endeavours and their ecological impacts. This study examines the effects of FDI, material footprint, economic growth and ICT on the load capacity factor in nations in SSA. The results of this study have important consequences for decision-makers, investors, and stakeholders who are seeking to steer the course towards sustainable development in the region.
Firstly, this paper demonstrates that material footprint relationship with load capacity factor for the SSA economies is significantly positive. In addition, a bi-directional causality link between material footprint and load capacity factor is established. The adoption of renewable energy sources, such as solar, wind, and geothermal, can thus substantially diminish the environmental impact and material footprint associated with extraction and production processes. The SSA region has immense capacity for the implementation of green energy sources, providing a significant chance for the promotion of sustainable development. Furthermore, by advocating for resource efficiency in several areas such as energy, building, and manufacturing, it is possible to reduce the overall amount of materials used. Implementing circular economy concepts, which involve the reuse and recycling of resources, can lead to additional reductions in resource use. Similarly, the implementation of efficient environmental legislation and policies that encourage sustainable activities, discourage unsustainable resource exploitation, and foster resource efficiency can play a crucial role in bringing about significant positive ecological changes. In addition, increasing public consciousness regarding the environmental consequences of material consumption and advocating for responsible consumption choices might facilitate wider social transitions towards sustainable practices.
This research also illustrates that economic growth develops a significantly negative relationship with load capacity factor. Additionally, economic development shows a two-way casual association with load capacity factor in the SSA economies. For this reason, it is interesting yet worrisome that economic growth and load capacity factor in SSA economies have a strongly negative connection. It indicates that as these economies expand, they concurrently experience a decrease in their capacity to manage the environmental stresses caused by their actions. Therefore, it is necessary for the SSA strategy to transition its focus from solely GDP-driven growth to a comprehensive and quality-oriented approach that gives priority to wellbeing, ecological sustainability, and fair distribution of benefits. This may entail the use of measurements such as the green GDP and genuine progress indicator. The economies of Sub-Saharan Africa should also enhance their investments in natural capital, including ecosystem restoration, protected areas, and sustainable land management techniques. This can bolster the environment’s ability to withstand and mitigate the effects of economic activity.
Furthermore, it is crucial for SSA countries to adopt resource pricing mechanisms, including eco-labelling, pay-for-ecosystem services schemes, and environmental levies, in order to accurately assess the expenses associated with resource utilization and to assign value to environmental products and services. This can deter excessive consumption and motivate the adoption of sustainable habits. The SSA should moreover bolster the fortification of environmental legislation, establish efficient monitoring methods, and guarantee rigorous enforcement to avert unsustainable resource extraction and pollution. This should also encompass the expansion of research efforts on cleaner technologies, resource efficiency techniques, and sustainable development models that are applicable to situations in Sub-Saharan Africa. This has the potential to provide valuable insights for policymaking and uncover novel green approaches. Similarly, it is crucial to promote the sharing of information and collaboration between nations in Sub-Saharan Africa and other areas that are encountering comparable difficulties. This can facilitate knowledge exchange and expedite the implementation of effective environmental methods.
Evidence in this paper also suggests that FDI illustrates a significantly negative connection to load capacity factor in the SSA countries. This result indicates that although FDI may give rise to economic advantages, it might also exacerbate environmental stress and diminish the capacity of the SSA region to support such activities. Practitioners in the SSA nations should detect and eliminate foreign investments in sectors that exploit resources, engage in polluting manufacturing, or undertake unsustainable infrastructure projects, since they might lead to environmental deterioration and raise the load capacity factor. Moreover, it is crucial to enhance local expertise and infrastructure concerning environmental management in host nations in order to minimize obstacles that impede their capacity to alleviate the environmental consequences of FDI initiatives.
Similarly, the SSA should adopt measures and incentives that entice international investments in line with sustainable development principles. It should also guarantee that FDI initiatives comply with rigorous environmental regulations and contribute to enhancing the load capacity factor. Policymakers in Sub-Saharan Africa should prioritize the implementation of transparent decision-making procedures when it comes to FDI projects. This includes promoting open discourse and active involvement with local people and organizations that may be affected by these projects. This may effectively resolve any concerns, guarantee that the project is in line with local requirements, and minimize any potential negative effects on the environment.
This article also posits that ICT develops a negative and significant association with load capacity factor in the SSA countries. In addition, a bi-directional casuality link is apparent involving ICT and load capacity factor. Hence, although ICT is frequently lauded as a catalyst for sustainable development, the inverse connection indicates unanticipated environmental strains associated with its implementation in the SSA countries. While the growing dependence on ICT infrastructure, such as data centres, telecommunication networks, and personal gadgets, might lead to higher energy consumption, it is crucial to prioritize the use of renewable energy sources over fossil fuels. This effort has the potential to lead to a decrease in environmental deterioration and a reduction in greenhouse gas emissions. Furthermore, the fast progress in technology results in frequent replacement of devices, so creating substantial quantities of electronic waste. Therefore, it is crucial to implement effective e-waste management in order to mitigate pollution, prevent resource exhaustion, and minimize health hazards.
SSA economies should also actively address the digital gap and strive to achieve fairness. This activity is crucial because providing equal access to ICT reduces socio-economic disparities. This can result in less strain on natural resources in rural regions or marginalized people, since they are provided with broadened alternative methods of communication and access to information. Furthermore, nations within the SSA region have the potential to improve their sustainable sourcing methods, namely, by promoting the use of environmentally friendly sources for minerals and materials utilized in ICT hardware. This includes the promotion of traceability, the implementation of fair-trade standards, and the mitigation of environmental problems resulting from industrial activity.
This study provides significant insights into the determinants of Sub-Saharan Africa’s (SSA) Load Capacity Factor (LCF). Nevertheless, it is crucial to recognize some constraints pertaining to the accessibility of data. Initially, this research focuses exclusively on 37 SSA nations because the data for the selected period (1970–2019) is only available for these countries. Expanding the inclusion of new nations, along with enhanced data gathering efforts, has the potential to offer a more comprehensive depiction. Furthermore, this approach specifically examines foreign direct investment (FDI), material footprint, economic growth, and information and communication technology (ICT). Nevertheless, there may be more variables that are not included in this study that might impact LCF. Additional variables such as governance, environmental restrictions, and social development, amongst others should be investigated in further research. Furthermore, the study indicates that there is a reciprocal link between ICT, material footprint, economic growth, and LCF. However, it is difficult to establish conclusive causal correlations when analysing observational data. Subsequent investigations utilizing experimental or quasi-experimental techniques might yield more insights into causal conclusions. Although there are certain limitations, this analysis offers a strong basis for comprehending the intricate relationship between economic development, resource consumption, and technical improvement in influencing SSA’s LCF. This research promotes the need for more investigation and improvement of knowledge in this crucial field by recognizing these constraints.
Conclusively, analysing the effects of foreign direct investment, information and communication technology, and material footprint on the load capacity factor hypothesis in Sub-Saharan African nations provides fertile ground for future academic inquiry in a number of intriguing avenues. Therefore, it is crucial to evaluate the environmental impacts of various patterns of FDI, changes in material footprint, and adoption of ICT on the load capacity factor, taking into account issues such as greenhouse gas emissions and resource depletion. Furthermore, it is crucial to broaden the research by investigating the impact of governance quality, institutional frameworks, and policies on the correlations between these factors and load capacity factor. This may also be expanded by examining the social ramifications of these elements, encompassing effects on employment, disparities in income, and the availability of energy for various demographic groups. Additional approaches could also create novel data sources by deploying satellite imaging, machine learning techniques, and big data analysis to get more extensive and detailed data on these factors, as well as other important indicators.
The original contributions presented in the study are included in the article/Supplementary Material, further inquiries can be directed to the corresponding author.
FG: Conceptualization, Investigation, Resources, Methodology, Data Analysis, Writing–original draft, Writing–review and editing.
The author(s) declare that no financial support was received for the research, authorship, and/or publication of this article. This research did not receive any specific grant from funding agencies in the public, commercial, or not-for-profit sectors.
The author declares that the research was conducted in the absence of any commercial or financial relationships that could be construed as a potential conflict of interest.
All claims expressed in this article are solely those of the authors and do not necessarily represent those of their affiliated organizations, or those of the publisher, the editors and the reviewers. Any product that may be evaluated in this article, or claim that may be made by its manufacturer, is not guaranteed or endorsed by the publisher.
The Supplementary Material for this article can be found online at: https://www.frontiersin.org/articles/10.3389/fenvs.2024.1419307/full#supplementary-material
Acheampong, A. O., Adams, S., and Boateng, E. (2019). Do globalization and renewable energy contribute to carbon emissions mitigation in Sub-Saharan Africa? Sci. Total Environ. 677, 436–446. doi:10.1016/j.scitotenv.2019.04.353
Adebayo, T. S., Pata, U. K., and Akadiri, S. S. (2024). A comparison of CO2 emissions load capacity factor and ecological footprint for Thailand’s environmental sustainability. Environ. Dev. Sustain 26 (1), 2203–2223. doi:10.1007/s10668-022-02810-9
Ali, E. B., Shayanmehr, S., Radmehr, R., Bayitse, R., and Agbozo, E. (2024). Investigating environmental quality among G20 nations: the impacts of environmental goods and low-carbon technologies in mitigating environmental degradation. Geosci. Front. 15 (1), 101695. doi:10.1016/j.gsf.2023.101695
Aust, V., Morais, A. I., and Pinto, I. (2020). How does foreign direct investment contribute to Sustainable Development Goals? Evidence from African countries. J. Clean. Prod. 245, 118823. doi:10.1016/j.jclepro.2019.118823
Awosusi, A. A., Adebayo, T. S., Kirikkaleli, D., Rjoub, H., and Altuntaş, M. (2023). “Evaluating the determinants of load capacity factor in Japan: the impact of economic complexity and trade globalization,” in Nat resour forum (Oxford UK: Blackwell Publishing Ltd).
Ayad, H., Lefilef, A., Jahanger, A., and Balsalobre-Lorente, D. (2023). “Reinvestigate the effect of foreign direct investment on environmental quality in the MENA region: is there any difference using the load capacity factor?”. J. Knowl. Econ. doi:10.1007/s13132-023-01629-7
Aydin, M., and Degirmenci, T. (2023). The impact of clean energy consumption green innovation and technological diffusion on environmental sustainability: new evidence from load capacity curve hypothesis for 10 European Union countries. Sustain Dev. 32, 2358–2370. doi:10.1002/sd.2794
Ayompe, L. M., Davis, S. J., and Egoh, B. N. (2021). Trends and drivers of African fossil fuel CO2 emissions 1990–2017. Environl Res. Lett. 15 (12), 124039. doi:10.1088/1748-9326/abc64f
Baninla, Y., Lu, Y., Zhang, Q., Omotehinse, A. O., Zheng, X., Zhang, M., et al. (2020). Material use and resource efficiency of African sub-regions. J. Clean. Prod. 247, 119092. doi:10.1016/j.jclepro.2019.119092
Belloc, I., and Molina, J. A. (2023). The ecological footprint in Africa: identifying convergence clubs from 1970 to 2018. App Econ. Lett. 30 (19), 2808–2813. doi:10.1080/13504851.2022.2107984
Butt, S., Faisal, F., Chohan, M. A., Ali, A., and Ramakrishnan, S. (2023). Do shadow economy and institutions lessen the environmental pollution? Evidence from Panel of ASEAN-9 Economies. J. Knowl. Econ., 1–29. doi:10.1007/s13132-023-01217-9
De Hoyos, R. E., and Sarafidis, V. (2006). Testing for cross-sectional dependence in panel-data models. Stata J. 6 (4), 482–496. doi:10.1177/1536867x0600600403
Djedaiet, A., Ayad, H., and Ben-Salha, O. (2024). Oil prices and the load capacity factor in African oil-producing OPEC members: modeling the symmetric and asymmetric effects. Res. Policy 89, 104598. doi:10.1016/j.resourpol.2023.104598
Dogan, A., and Pata, U. K. (2022). The role of ICT R&D spending and renewable energy consumption on environmental quality: testing the LCC hypothesis for G7 countries. J. Clean. Prod. 380, 135038. doi:10.1016/j.jclepro.2022.135038
Dumitrescu, E. I., and Hurlin, C. (2012). Testing for Granger non-causality in heterogeneous panels. Econ. Model. 29 (4), 1450–1460. doi:10.1016/j.econmod.2012.02.014
Erdogan, S. (2024). On the impact of natural resources on environmental sustainability in African countries: a comparative approach based on the EKC and LCC hypotheses. Res. Policy 88, 104492. doi:10.1016/j.resourpol.2023.104492
Espoir, D. K., Sunge, R., Nchofoung, T., and Alola, A. A. (2024). Analysing the drivers of ecological footprint in Africa with machine learning algorithm. Environ. Impact Assess. Rev. 104, 107332. doi:10.1016/j.eiar.2023.107332
Fareed, Z., Salem, S., Adebayo, T. S., Pata, U. K., and Shahzad, F. (2021). Role of export diversification and renewable energy on the load capacity factor in Indonesia: a Fourier quantile causality approach. Front. Environ. Sci. 9, 770152. doi:10.3389/fenvs.2021.770152
Gabor, E., and Sauvat, K. P. (2021). Facilitating sustainable FDI for sustainable development in a WTO Investment Facilitation Framework: four concrete proposals. J. World Trade 55 (2), 261–286. doi:10.54648/trad2021010
Galli, A. (2015). On the rationale and policy usefulness of Ecological Footprint Accounting: the case of Morocco. Environ. Sci. Policy 48, 210–224. doi:10.1016/j.envsci.2015.01.008
Ganda, F. (2021). The influence of growth determinants on environmental quality in Sub-Saharan Africa states. Environ. Dev. Sustain 23 (5), 7117–7139. doi:10.1007/s10668-020-00907-7
Global Footprint Network (2009). Footprint f actbook 2009. Available at: https://wwwfootprintnetworkorg/content/uploads/2020/08/AfricaFactbook_2009pdf.
Gossel, S. J. (2018). FDI democracy and corruption in Sub-Saharan Africa. J. Policy Model. 40 (4), 647–662. doi:10.1016/j.jpolmod.2018.04.001
Gutierrez, L. (2003). On the power of panel cointegration tests: a Monte Carlo comparison. Econ. Lett. 80 (1), 105–111. doi:10.1016/s0165-1765(03)00066-1
Jin, X., Ahmed, Z., Pata, U. K., Kartal, M. T., and Erdogan, S. (2023). Do investments in green energy energy efficiency and nuclear energy R&D improve the load capacity factor? An augmented ARDL approach. Geosci. Front. 15, 101646. doi:10.1016/j.gsf.2023.101646
Kansanga, M. P., Andersen, D., Kpienbaareh, S., Mason-Renton, K., Atuoye, Y., Sano, R., et al. (2019). Traditional agriculture in transition: examining the impacts of agricultural modernization on smallholder farming in Ghana under the new Green Revolution. Int. J. Sustain Dev. World Ecol. 26 (1), 11–24. doi:10.1080/13504509.2018.1491429
Kao, C. (1999). Spurious regression and residual-based tests for cointegration in panel data. J. Econom. 90 (1), 1–44. doi:10.1016/s0304-4076(98)00023-2
Latif, N., and Faridi, M. Z. (2023). Examining the impact of financial development on load capacity factor (LCF): system GMM analysis for Asian economies. Front. Energy Res. 10, 1063212. doi:10.3389/fenrg.2022.1063212
Li, F., Zhang, J., and Li, X. (2022). Research on supporting developing countries to achieve green development transition: based on the perspective of renewable energy and foreign direct investment. J. Clean. Prod. 372, 133726. doi:10.1016/j.jclepro.2022.133726
Li, L., Chen, Q., and Mehmood, U. (2023b). Analyzing the validity of load capability curve: how economic complexity renewable energy R&D and communication technologies take their part in G-20 countries. Environ. Sci. Pollut. Res. 30 (40), 92068–92083. doi:10.1007/s11356-023-28436-6
Li, X., Sun, Y., Dai, J., and Mehmood, U. (2023a). How do natural resources and economic growth impact load capacity factor in selected Next-11 countries? Assessing the role of digitalization and government stability. Environ. Sci. Pollut. Res. 30 (36), 85670–85684. doi:10.1007/s11356-023-28414-y
Maphosa, V., and Maphosa, M. (2020). E-waste management in Sub-Saharan Africa: a systematic literature review. Cogent Bus. Manag. 7 (1), 1814503. doi:10.1080/23311975.2020.1814503
Mapiye, O., Makombe, G., Molotsi, A., Dzama, K., and Mapiye, C. (2023). Information and communication technologies (ICTs): the potential for enhancing the dissemination of agricultural information and services to smallholder farmers in sub-Saharan Africa. Afr. Infor Dev. 39 (3), 638–658. doi:10.1177/02666669211064847
Marcelino, J., Franco, A. M. A., Acácio, M., Soriano-Redondo, A., Moreira, F., and Catry, I. (2023). Anthropogenic food subsidies reshape the migratory behaviour of a long-distance migrant. Sci. Total Environ. 858, 159992. doi:10.1016/j.scitotenv.2022.159992
Mark, N. C., Ogaki, M., and Sul, D. (2005). Dynamic seemingly unrelated cointegrating regressions. Rev. Econ. Stud. 72 (3), 797–820. doi:10.1111/j.1467-937x.2005.00352.x
Marti, L., and Puertas, R. (2020). Analysis of the efficiency of african countries through their ecological footprint and biocapacity. Sci. Total Environ. 722, 137504. doi:10.1016/j.scitotenv.2020.137504
Mehmood, U., Tariq, S., Aslam, M. U., Agyekum, E. B., Uhunamure, S. E., Shale, K., et al. (2023). Evaluating the impact of digitalization renewable energy use and technological innovation on load capacity factor in G8 nations. Sci. Rep. 13 (1), 9131. doi:10.1038/s41598-023-36373-0
Mugabe, J. (2005). “Governing foreign direct investment in Sub-Saharan Africa: policies and practices reconsidered,” in International investment for sustainable development: balancing rights and rewards. Editors L. Zarsky (London: Earthscan), 74–96.
Muheirwe, F., Kombe, W., and Kihila, J. M. (2022). The paradox of solid waste management: a regulatory discourse from Sub-Saharan Africa. Habitat Int. 119, 102491. doi:10.1016/j.habitatint.2021.102491
Ni, Z., Yang, J., and Razzaq, A. (2022). How do natural resources digitalization and institutional governance contribute to ecological sustainability through load capacity factors in highly resource-consuming economies? Res. Policy 79, 103068. doi:10.1016/j.resourpol.2022.103068
Ning, L., and Wang, F. (2018). Does FDI bring environmental knowledge spillovers to developing countries? The role of the local industrial structure. Environ. Res. Eco 71 (2), 381–405. doi:10.1007/s10640-017-0159-y
Noor, S., Yang, W., Guo, M., van Dam, K. H., and Wang, X. (2018). Energy demand side management within micro-grid networks enhanced by blockchain. App Energy 228, 1385–1398. doi:10.1016/j.apenergy.2018.07.012
Ohemeng, W., Ofori-Boateng, K., Kwame Agyapong, E., and Darmoe, J. (2023). Environmental risk and growth in foreign direct investment: is the composition of FDI in sub-Saharan Africa a speculative type? Cogent Econ. Finan 11 (2), 2243695. doi:10.1080/23322039.2023.2243695
Okelele Do Lokina, R., and Ruhinduka, R. D. (2022). Effect of trade openness on ecological footprint in sub-Saharan Africa. Afr. J. Econ. Rev. 10 (1), 209–233. https://www.ajol.info/index.php/ajer/article/view/219359.
Omisore, A. G. (2018). Attaining Sustainable Development Goals in sub-Saharan Africa; the need to address environmental challenges. Environ. Dev. 25, 138–145. doi:10.1016/j.envdev.2017.09.002
Oo, K. T., Jonah, K., and Thin, M. M. Z. (2023). A systematic review of the pros and cons of digital pollution and its impact on the environment. J. Sustain Environ. Manag. 2 (1), 61–73. doi:10.3126/josem.v2i1.53118
Osei-Owusu, A. K., Danquah, M., and Towa, E. (2022). Unravelling Africa's raw material footprints and their drivers. WIDER Working Paper, No. 2022/115. Helsinki: The United Nations University World Institute for Development Economics Research (UNU-WIDER). doi:10.35188/UNU-WIDER/2022/249-2
Pata, U. K., Kartal, M. T., Adebayo, T. S., and Ullah, S. (2023). Enhancing environmental quality in the United States by linking biomass energy consumption and load capacity factor. Geosci. Front. 14 (3), 101531. doi:10.1016/j.gsf.2022.101531
Pedroni, P. (1999). Critical values for cointegration tests in heterogeneous panels with multiple regressors. Oxf Bull. Econ. Stat. 61 (S1), 653–670. doi:10.1111/1468-0084.61.s1.14
Pesaran, M. H. (2004). “General diagnostic tests for cross-sectional dependence in panels,” in Working Paper no 1229 Category 10: empirical and theoretical methods.
Pesaran, M. H. (2007). A simple panel unit root test in the presence of cross-section dependence. J. App Econom. 22 (2), 265–312. doi:10.1002/jae.951
Pesaran, M. H., and Yamagata, T. (2008). Testing slope homogeneity in large panels. J. Econom. 142 (1), 50–93. doi:10.1016/j.jeconom.2007.05.010
Ramzan, M., Razi, U., Kanwal, A., and Adebayo, T. S. (2024). An analytical link of disaggregated green energy sources in achieving carbon neutrality in China: a policy based novel wavelet local multiple correlation analysis. Prog. Nucl. Energy 167, 104986. doi:10.1016/j.pnucene.2023.104986
Sennuga, S. O., Conway, J. S., and Mabayoje, A. S. (2020). Impact of information and communication technologies (ICTS) on agricultural productivity among smallholder farmers: evidence from Sub-Saharan African communities. Int. J. Agric. Ext. Rural. Dev. Stud. 7 (1), 27–43.
Siche, R., Pereira, L., Agostinho, F., and Ortega, E. (2010). Convergence of ecological footprint and emergy analysis as a sustainability indicator of countries: Peru as case study. Commun. Nonlinear Sci. Numer. Simul. 15 (10), 3182–3192. doi:10.1016/j.cnsns.2009.10.027
Sun, H., Pofoura, A. K., Mensah, I. A., Li, L., and Mohsin, M. (2020). The role of environmental entrepreneurship for sustainable development: evidence from 35 countries in Sub-Saharan Africa. Sci. Total Environ. 741, 140132. doi:10.1016/j.scitotenv.2020.140132
Sun, W., Sun, Y., Hong, X., Zhang, Y., and Liu, C. (2023). Research on biomass waste utilization based on pollution reduction and carbon sequestration. Sustain 15 (5), 4535. doi:10.3390/su15054535
Teng, W., Islam, M. M., Vasa, L., Abbas, S., and Shahzad, U. (2023). Impacts of nuclear energy greener energy and economic progress on the load capacity factor: what we learn from the leading nuclear power economies? Geosci. Front. 15, 101739. doi:10.1016/j.gsf.2023.101739
Tiamgne, X. T., Kalaba, F. K., and Nyirenda, V. R. (2022). Mining and socio-ecological systems: a systematic review of Sub-Saharan Africa. Res. Policy 78, 102947. doi:10.1016/j.resourpol.2022.102947
Vats, P., Hiranmai, R. Y., and Neeraj, A. (2023). Bioconversion of organic waste for solid waste management and sustainable agriculture—emphasized impact of bioelectromagnetic energy. Sustain Clean. Tech. Environ. Remed Ave. Nano Biotech. Available at: https://link.springer.com/chapter/10.1007/978-3-031-29597-3_16.
Wang, J., and Dong, K. (2019). What drives environmental degradation? Evidence from 14 Sub-Saharan African countries. Sci. Total Environ. 656, 165–173. doi:10.1016/j.scitotenv.2018.11.354
Wang, Q., Sun, J., Li, R., and Pata, U. K. (2023). Linking trade openness to load capacity factor: the threshold effects of natural resource rent and corruption control. Gondwana Res. 129, 371–380. doi:10.1016/j.gr.2023.05.016
Warner, K. J., and Jones, G. A. (2018). Energy and population in Sub-Saharan Africa: energy for four billion? Environ 5 (10), 107. doi:10.3390/environments5100107
WWF (2018). “Living planet report - 2018,” in WWF gland Switzerland. Editors M. Aiming Higher Grooten, and A. REA Available at: https://c402277sslcf1rackcdncom/publications/1187/files/original/LPR2018_Full_Report_Spreadspdf.
Xu, D., Salem, S., Awosusi, A. A., Abdurakhmanova, G., Altuntaş, M., Oluwajana, D., et al. (2022). Load capacity factor and financial globalization in Brazil: the role of renewable energy and urbanization. Front. Environ. Sci. 9, 823185. doi:10.3389/fenvs.2021.823185
Yang, M., She, D., Abbas, S., Ai, F., and Adebayo, T. S. (2023). Environmental cost of financial development within the framework of the load capacity curve hypothesis in the BRICS economies: do renewable energy consumption and natural resources mitigate some burden? Geo J. 58 (10), 3915–3927. doi:10.1002/gj.4817
Yıldırım, M., Destek, M. A., and Manga, M. (2024). Foreign investments and load capacity factor in BRICS: the moderating role of environmental policy stringency. Environ. Sci. Poll. Res. 31, 11228–11242. doi:10.1007/s11356-023-31814-9
Yu, X., and Li, Y. (2020). Effect of environmental regulation policy tools on the quality of foreign direct investment: an empirical study of China. J. Clean. Prod. 270, 122346. doi:10.1016/j.jclepro.2020.122346
Keywords: load capacity factor, material footprint, economic growth, foreign direct investment (FDI), information and communication technology (ICT)
Citation: Ganda F (2024) Analysing the impacts of FDI, material footprint and ICT on the load capacity factor in sub-saharan African countries. Front. Environ. Sci. 12:1419307. doi: 10.3389/fenvs.2024.1419307
Received: 18 April 2024; Accepted: 19 June 2024;
Published: 18 July 2024.
Edited by:
Muhammad Ramzan, Lebanese American University, LebanonReviewed by:
Asma Kanwal, Sichuan University, ChinaCopyright © 2024 Ganda. This is an open-access article distributed under the terms of the Creative Commons Attribution License (CC BY). The use, distribution or reproduction in other forums is permitted, provided the original author(s) and the copyright owner(s) are credited and that the original publication in this journal is cited, in accordance with accepted academic practice. No use, distribution or reproduction is permitted which does not comply with these terms.
*Correspondence: Fortune Ganda, Zm9jaGk1NTVAeWFob28uY29t
Disclaimer: All claims expressed in this article are solely those of the authors and do not necessarily represent those of their affiliated organizations, or those of the publisher, the editors and the reviewers. Any product that may be evaluated in this article or claim that may be made by its manufacturer is not guaranteed or endorsed by the publisher.
Research integrity at Frontiers
Learn more about the work of our research integrity team to safeguard the quality of each article we publish.