- 1Department of Environmental Science, Indira Gandhi National Tribal University, Amarkantak, Madhya Pradesh, India
- 2College of Agriculture, Indira Gandhi Agricultural University, Bilaspur, Chhattisgarh, India
- 3Department of Environmental Science, Sant Gahira Guru Vishwavidyalaya, Ambikapur, Chhattisgarh, India
- 4Krishi Vigyan Kendra, Indira Gandhi National Tribal University, Amarkantak, Madhya Pradesh, India
- 5Guru Ghasidas University, Bilaspur, Chhattisgarh, India
- 6College of Agriculture, Central Agricultural University, Imphal, Manipur, India
- 7School of Hydrology and Water Resources, Nanjing University of Information Science and Technology, Nanjing, China
- 8Department of Zoology, College of Science, King Saud University, Riyadh, Saudi Arabia
- 9Jindal Global Business School (JGBS), O. P. Jindal Global University, Sonipat, Haryana, India
Introduction: Anthropogenic disturbances resulting from extensive mining activities in tropical regions pose significant threats to native land use, leading to deforestation, biodiversity loss, climate change impacts, environmental degradation, health risks, landscape fragmentation, compromised ecological security, and societal wellbeing. Monitoring Land Use and Land Cover Change (LULCC) becomes imperative for evaluating the extent and nature of land degradation in mined areas.
Methods: This study examined and compared land cover change patterns across three coalmined sites: Sohagpur (Site-I), Jamuna & Kotma (Site-II), Bishrampur (Site-III) in Central India over 3 decades using Landsat satellite imagery from 1994, 2007, and 2022. The Maximum Likelihood (ML) algorithm, within a supervised classification framework, was applied to discern mining impacts on decadal land use shifts.
Results and discussion: The analysis revealed that 7.32%–17.61% of forest cover, 5.0%–10% of water bodies, and 3%–5% of agricultural lands were lost due to mining activities, with Site three and Site two experiencing greater losses compared to Site 1. Overall, native land cover diminished by 35% between 1994 and 2022. Indices including Soil Index, Climate Index, Terrain Index, Land Utilization Index, and Vegetation Index were derived to assess land degradation patterns. These indices were integrated using a weighted index model in ArcGIS to generate the Land Degradation Vulnerability Index (LDVI). Vulnerability notably escalated with mining expansion, particularly pronounced at Site 3 (Bishrampur) and lower at Site 1 (Sohagpur). The “extremely vulnerable” class encompassed a substantial area (25%–40%), while the “low vulnerable” class was less than 5% across all sites. This study’s comprehensive analysis aids policymakers, planners, and managers in prioritizing targeted interventions and implementing sustainable land management 175 practices for ecorestoration, aligning with the goal of Zero Net Land Degradation 176 (ZNLD) in coal-mined landscapes.
1 Introduction
Globalization has had tremendous impact on our lives. It has opened new avenues of technological advancement, increased communication, scientific developments with smarter innovation (Xia, et al., 2022; Zhao, et al., 2022; Swamy and Darro, 2023; Fang et al., 2024). On the other hand, the impact of globalization on environment cannot be undermined (Song, et al., 2020; Xiao et al., 2023). Coal mining has emerged as a major energy generating industrial activity in thermal power dependant countries such as India to address the emerging challenges of growing energy needs with burgeoning population explosion (Oskarsson et al., 2024). The humongous expansion of mining has impacted land use, polluted the natural environment, and jeopardized the ecological security and wellbeing of the people living in the vicinity of mined landscapes and directly compromised the local ecosystems with irreversible transformations (Saini, et al., 2016; Bandhophadyay et al., 2020; Aihrwal et al., 2021; Mishra et al., 2022; Sethi et al., 2024). Numerous studies have clearly demonstrated that land-use change is a critical parameter, that needs to be monitored at regular intervals to understand the alterations in bio-geochemical cycles, surface energy balance, structure and diversity of ecosystems (Song, et al., 2018; Swamy and Darro, 2023; Thakur et al., 2024; Zhang et al., 2024). The mining sector undoubtedly boosts the economic prosperity, albeit at the expense of environmental degradation (Thakur, et al., 2019; Kumari, et al., 2020). Deforestation in mined landscapes disrupts local forest-dependent communities and contributes around 10% of anthropogenic greenhouse gas emissions, impacting future climate (Scholes et al., 2018). India has experienced significant deforestation, stabilized since the 1980 ban on clear felling. However, forest degradation persists due to industrial expansion, biotic pressure, fires, and land diversion for mining (Joshi et al., 2006).
Coal mining has accelerated landscape fragmentation and edge effects, converting the dense forests into open forests, open forests to grasslands, grasslands into grazing lands further opening the routes of agricultural encroachments in forested lands (Mishra, et al., 2022). In 2016, a research conducted by Maryland University summarized that over 73 million acres of the global tree cover were lost for mining and the process is consistently undergoing (Bradford, 2018). The indiscriminate destruction of forests and other natural resources compromise the core concept of sustainable development, impeding the achievement of the United Nations’ targeted Sustainable Development Goals (SDGs) for 2030 (Weisse and Goldman, 2017). It becomes imperative to minimize the abrupt land use changes and deforestation in coal mined environs, while simultaneously conserving the remnant forests and implementing sustainable land management practices, which are vital for bringing resilience to the deteriorated landscape. Coal as a pivotal non-renewable source of energy is currently irreplaceable due to paucity of viable alternative renewable energy resources. China, India, United States, Australia and the European Union are major coal producers and consumers, with their consumption predicted to rise by 2.6% by 2020 (Thakur T. K. et al., 2022). India is currently ranked as the third largest producer of coal and has experienced rapid growth over the last few decades increasing from 30 million tonnes (Mt) in 1946 to 729.10 Mt in 2020 (Ministry of Coal, 2021; Thakur et al., 2022b). Coal mining has diverse environmental impacts, including topsoil and vegetation loss from overburden dumps formed during continuous blasting or excavation. Mine tailings, rich in heavy metals and pollutants, accumulate on leased land, posing contamination risks (Anon, 2006; Sahu and Dash, 2011). Open-cast mining, producing 8–10 times more waste than underground mining, exacerbates land degradation and environmental issues (Anon, 2006; Sahu and Dash, 2011). Mine spoils lead to groundwater contamination, mineral leaching, heavy metal discharge, soil pollution, erosion, and effluent discharge in sensitive ecosystems (Sahu and Dash, 2011). Despite increased awareness of coal mining’s environmental impacts, industry expansion plans persist, aiming to double production in the coming decades. These plans involve deeper mining and maintaining coal quality standards, resulting in significant waste generation. For instance, extracting 1 million tonnes of coal could generate 15 million tonnes of waste, intensifying pressure on India’s dwindling land resources (Sahu and Dash, 2011).
The storage and removal of overburden further contribute to the loss of forest cover and fertile soil (Wickham, et al., 2007; Moomen and Dewan, 2016). With increased mining, the issue of Mining Induced Land Degradation (MILD) will accelerate and it is predicted to escalate exponentially in future with growing demands for mineral commodities (Moomen and Dewan, 2016). Vulnerability to degradation varies under different environmental conditions despite similar land use (Van der Werf and Petit, 2002; Darradi et al., 2012). Activities associated with mining stimulate alteration in topography, topsoil loss, vegetation loss and which eventually result in land degradation under different landuse. Various physical, social, and environmental factors such as climate, soil, terrain, and natural features collectively contribute to land degradation vulnerability (Nowak and Schneider, 2017). Land degradation from mining has become a focal point globally, with efforts to achieve Zero Net Land Degradation (ZNLD) by 2030 in developing countries (Hintjens, 2000; EPA, 2003; Ma et al., 2019). Prioritizing the mitigation of land degradation caused by open-cast coal mining is crucial to prevent its conversion into ecologically fragile wastelands (Guan and Yu, 2021; Thakur et al., 2022c; Pandey et al., 2022), which poses significant challenges to sustainable development, impacting the environment, agriculture, food security, and livelihoods (UNCCD, 2015).
LULCC monitoring is vital for resource planning and protection, with a shift towards quantifying impacts on ecological processes (Kumar et al., 2022; Swamy and Darro, 2023). Quantitative studies on vegetation cover disturbances are limited but crucial for sustainable resource management (Moomen and Dewan, 2016; Guan and Yu, 2021). Geospatial techniques, including satellite remote sensing (RS) and geographical information systems (GIS), are pivotal for understanding ecosystem dynamics and developing decision support systems (Brown et al., 2005; Thakur, 2018; Thakur T. K. et al., 2022; Mishra et al., 2022). Despite India’s small land area, it supports a large population, leading to significant land degradation (Sahu and Dash, 2011). Evaluating land degradation trends due to coal mining in peninsular India is essential for targeted reclamation efforts and ecosystem regeneration. This study compares LULCC across three mines in Central India (Sohagpur, Jamuna & Kotma, and Bishrampur) to assess their impact on land degradation vulnerability. Unlike previous studies that focused on single criteria, this research employs multiple indices derived from climatic, topographic, edaphic, and land variables to quantify land degradation patterns. These indices, including the soil index, climate index, terrain index, land utilization index, and natural resource prioritized index, are weighted based on their expected influence and integrated within a GIS framework to calculate the Land Degradation Vulnerability Index (LDVI). The LDVI aids in prioritizing ecorestoration efforts in degraded landscapes, supporting policymakers and planners in sustainable natural resource management in coal mining areas. Therefore, the current work was mainly focused to examine the effect of coal mining on i) Land use and land cover changes, ii) variations in soil index, terrain index, climate index and land utilization index iii) land-degradation vulnerability, and iv) finally, to advocate the implications for ecorestoration strategies of degraded landscapes.
2 Materials and methods
2.1 Study site
The study was conducted in three coal mining regions viz., Sohagpur - Site-I, Jamuna & Kotma - Site-II, Bishrampur -Site-III, in central India. The sites are located in the states of Madhya Pradesh and Chattishgarh, respectively, and are spread from 230 05′to 23° 30′N latitudes and 81° 13′to 82° 12′E longitudes in the Central Indian region covering an area of 14, 660 km2 (Figure 1). The region is characterized by a tropical sub-humid climate, with a year divisible into three distinct seasons. The hot summer extends from March to June, monsoon sets between July to October, and a cold winter start from November and ends by the second week of February. The mean annual temperature usually ranges between 25°C and 31°C. The region receives about 1192.3 mm of rain annually, with the majority falling during the monsoon seasons from July to September, accounting for 80%–90% of the total rainfall during the south-west monsoon period. The terrain is undulating, occasionally with high hills, dissected plateaus, steep slopes, and scarps with three types of soils: Alfisols, Inceptisols, and Vertisols. The area is predominately covered with Sal and Dense Mixed forests occasionally interspersed with bamboo brakes. The primary occupation is agriculture, with rain-fed paddy being monocropped during the rainy season on most of the land. In the winter season, crops such as wheat, Gram, mustard, niger, sunflower, etc., are cultivated in irrigated areas.
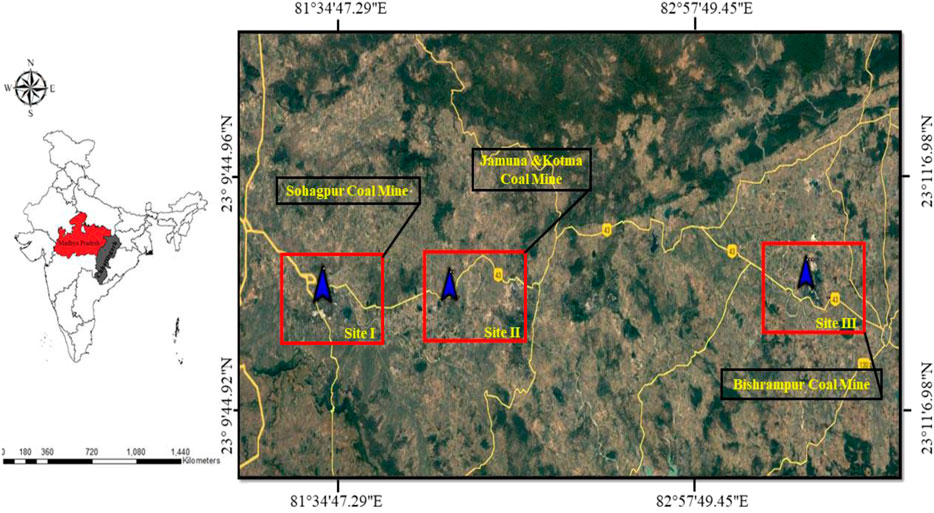
Figure 1. Location map of the study area along with all three selected sites of Sohagpur coal mines (Site I), Jamuna coal mines (Site II) and Bishrampur coal mines (Site III), Central India covering an area of 14, 660 km2.
2.2 Selection of satellite and ancillary data
The datasets utilized in this investigation are listed in Table 1 and comprising satellite data, reference data, digital elevation model (DEM), and Google Earth data. The datasets were archived from Landsat-5 (TM) for the year 1994, and Landsat-7 and Landsat-8 OLI for the years 2007 and 2022, respectively. The datasets were archived from the Landsat-5 (TM) for the year 1994, Landsat-7 and Landsat 8 OLI for the years 2007 and 2022, respectively. The data were collected and sorted from the Earth Explorer (http://earthexplorer.usgs.gov/). The same reference system UTM (zone 44) and WGS 84 datum have been used to project all the data for comparision Table 1 provides entire information regarding the research area, which lies inside the Worldwide Reference System of Landsat, path 142–143 and row 44. In March 2022, ground truth was perfomed using referencing data and Global Positioning System (GPS). The categorization, spatial analysis, and entire precise examination of categorized images all involved ground reference data. Figure 2 illustrates the schematic representation pattern for the analysis of images and generation of thematic maps. Preprocessing of satellite images including geometric, radiometric, atmospheric corrections were conducted before image analysis.
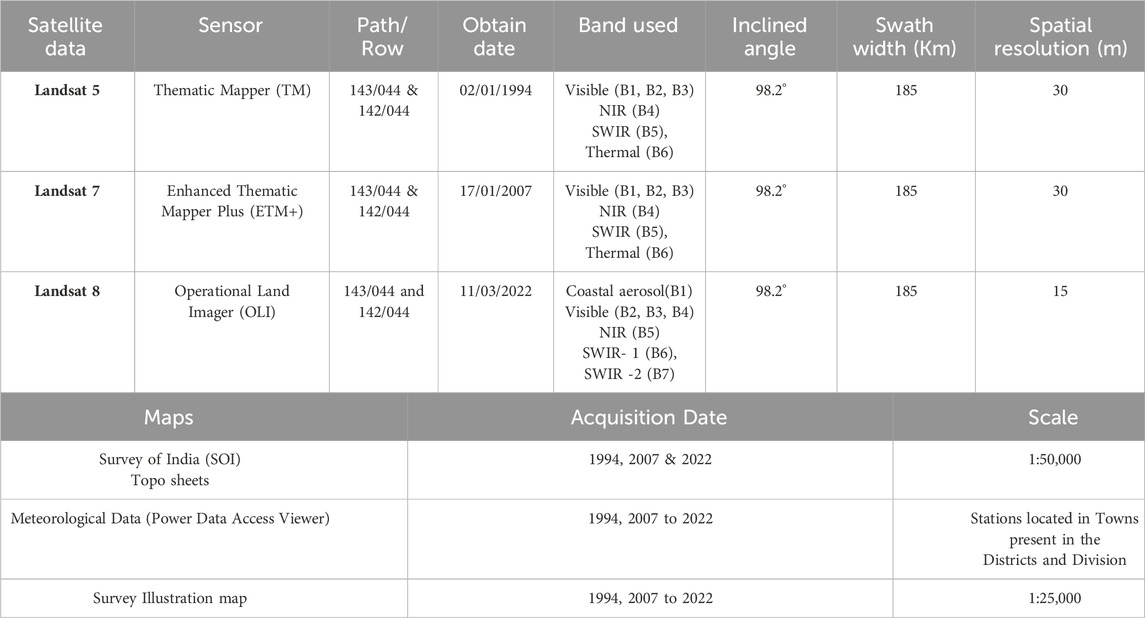
Table 1. Details of the source of data used in the present study. The satellite data used for LULC classification are derivative from Landsat-5 Thematic Mapper for 1994, whereas Landsat-7 & 8, Operational Land Imager (OLI) and Thermal Infrared Sensor (TIRS) data sets are used for the year 2007 & 2022.
2.3 LULC preparation and change detection analysis
Machine Learning Algorithms (MLAs) can automatically classify the land cover (Tan, et al., 2021) with the large set of data often used in land mapping applications. Digital image processing of Landsat satellite images were performed under ERDAS 2014 version and spatial analysis of other variables in ARCGIS. MLA and supervised classification were used to assess the LULC of the study area. The evaluation of land use categories on a spatio-temporal basis was depicted in Figure 3. The pixels have been classified into various land use types using supervised classification employing maximum likelihood algorithm (Ahmad and Quegan, 2013). Ancillary data from SOI (Survey of India) maps and field observations from GPS locations were used as reference data for improving the classification. The study area was classified into eight LULC groups. i.e., classes include forest, plantation, agriculture, wasteland, settlements, coal mines, overburden (OB) dumps, and water bodies.
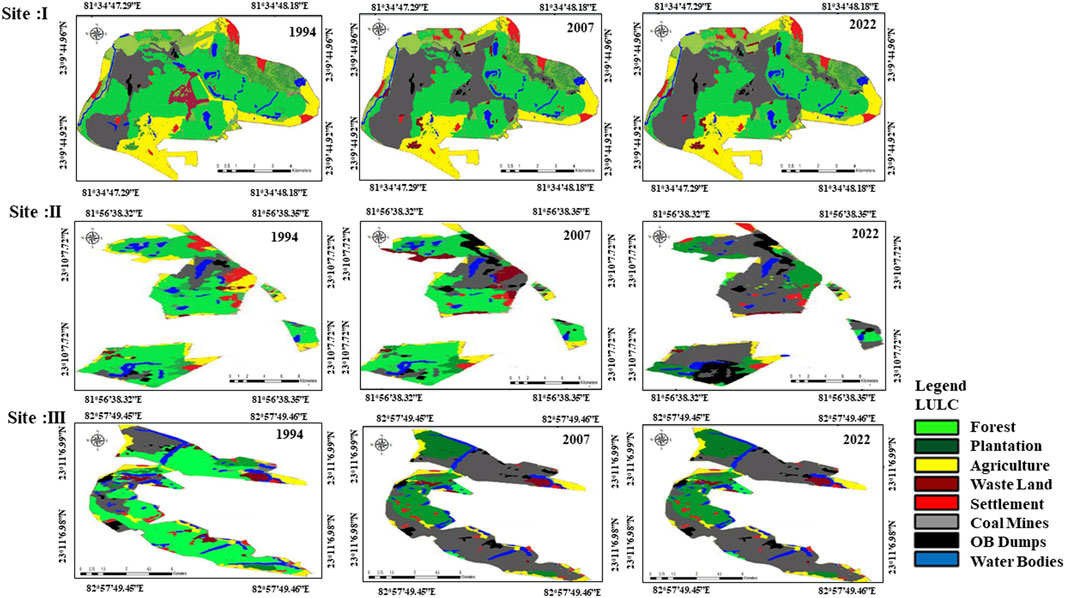
Figure 3. Land Use and land cover (LULC) maps of the study area (Site I, II and III) during 1994, 2007 and 2022 (study period).
For the detection of LULC change pattern during the different periods, the LULC maps corresponding to 1994, 2007 and 2022 has been resampled from the moderate resolution of satellite data (30 m) to high spatial resolution (5.8 m) to facilitate the comparsion of images. To evaluate the pattern of LULC changes between 1994 and 2022, all the categorized image alterations were created and analyzed using ERDAS (Yang and Wen, 2011).
2.4 Drivers of land degradation and land degadation vulnerability
The Land Degradation Vulnerability Index (LDVI) was constructed through a systematic process: 1) Identification of critical factors influencing land degradation, 2) Assessment and scoring of parameters within each variable criterion, 3) Determination of weights for each criterion, 4) Development of five indices (topography, soil, climate, land use, and vegetation), and 5) Integration of these indices to generate the final LDVI map. Various indices were derived using edaphic, climatic, land use, topographic, and vegetation data from relevant sources to formulate the LDVI model. The individual indices were developed by assigning different weights to contributing factors, with each factor considered in relation to all others from available sources (Supplementary Table S1). A thematic map layer of each criterion has been generated using GIS tool. In natural vulnerability all thematic layer give same weight but in environmental vulnerability all thematic layer were given different weight based on their sensitivity or effectiveness in the study area (Grigio et al., 2004). Thereafter, the different layers corresponding to different indices were integrated by assigning weightage score ranging from 0.1 to 1.0 based on literature and suggestions of experts (Table 2), where 0.1 was assigned to the lowest class pertaining to the specific LDVI index, while 1.0 was assigned to the highest triggering class. The model was employed to assess the land degradation vulnerability in three different sites of coal mining. The selection of pertinent indicators, methodologies were used for normalization, weighting strategies, all have a significant impact on the assessment of the susceptibility of a region to land degradation and the procedure adopted for deriving indices under ARC GIS are as follows.
2.5 Soil index (SI)
Depth, erosivity, depth of groundwater, soil drainage, and pH are some of soil characteristics that drive land degradation (Khan and Romshoo, 2008; Kumar, et al., 2022). The following equation was used to generate the index for each soil parameter independently.
Where Wi is weightage for the soil parameter, Ai denotes area of a particular class and Cj is Class code of jth class.
The soil index has been done by association of all the attributes, i.e., Soil Depth, Ground Water Depth, Soil Drainage and Erosivity.
Note:. Link for the above equation is required
where Si denotes Soil index; D is soil depth, GDW is ground water depth; SD is soil drainage and E is erosivity.
In order to assess the Composite Soil Index, the criteria have been classified into four groups, i.e., Very Good, Good, Poor, and Very Poor. The very poor soil index class, assigned higher weitage values, represents extreme vulnerability to soil degradation, whereas the very good soil index class, with lower weitage values, indicates lower susceptibility to vulnerability. Represent less prone to vulnerability degradation.
2.6 Terrain index (TI) and climate index (CI)
According to Freer et al. (2002), topography is a significant physical component that directly affects the process of land degradation. The Terrain Index has been calculated using ASTER Digital Elevation Model (DEM) along with a soil map (Rao, et al., 2016). The slope map was generated, categorizing the terrain into six distinct slope classes: flat plains (≤5%), gentle slopes (5%–10%), moderate slopes (11%–15%), moderately steep slopes (16%–20%), steep slopes (21%–25%), and very steep slopes (>25%). DEM was also utilized to generate the elevation map of land from contours, and drainage networks were extracted using depression-filling method under ArcGIS (Planchon and Darboux, 2002). The variability in climatic conditions, specifically temperature and rainfall (as depicted in SF1and2), significantly influences the land degradation process. The Thiessen polygon method was utilized to analyze Climate Index (CI), creating a climate surface from a time series of precipitation and temperature data spanning 1994 to 2022. CI categorizes the climate into four groups and is generated through the integration of temperature and precipitation layers within GIS.
2.7 Land utilization index (LUI)
LUI is a system for categorizing land, where each land unit is utilized in accordance with its potential for production and environmental deterioration, if the land is not properly used. The following equation was used to integrate multi-temporal LULC, VI, and LCI in the GIS environment to produce the land utilization index (LUI).
Where LUI denotes Land utilization index; VI is Vegetation index and LCI isLand capability index.
2.8 Land degradation vulnerability index (LDVI) maps
In the final step, the LDVI was derived using weighted overlay tool built in spatial analysis in Arc GIS, which integrated deterministic components (criteria-degradation indices). The aggregated index LDVI was calculated in the GIS environment following Eq. 6, adopting the procedure outlined by Mishra et al. (2022). The final output raster model was created by averaging the obtained cell values. Higher vulnerability towards land degradation is shown by greater raster values whilst, lesser vulnerability is indicated by lower values. Five classes (numbered 1–5) were assigned to the vulnerability raster output.
where DF indicates determinant ith factor and criteria classes of land degradation (TI, CI, SI, LUI and VI), Wi is weight of that criteria derived through analysis and n is number of criteria used (criteria = 5).
The LDVI map has been prepared using the range of LDVI values. The five classes of land degradation vulnerability identified were as follows: extremely vulnerable (>4), highly vulnerable (3 to ≤4), moderately vulnerable (2 to ≤3), low vulnerable (1 to ≤2), and very low vulnerable (<1) classes.
2.9 Clarity and accuracy of LDVI classes
The robustness of a model primarily depends on its accuracy, while the models are not precisely useful without validation (Pandey, et al., 2022). The validation of LDVI map was done by randomly taking the five sample points from each vulnerability class and the accuracy of the mapped vulnerability index was checked by Google Earth and ground truthing in the field.
3 Results
LULC maps illustrating changes in studied coal mine landscapes (Site I, Site II, and Site III) from 1994 to 2022 are presented in Figure 3 and Supplementary Table S1. Eight categories of land use, namely, forest, plantation, agriculture, coal mines, overburden (OB) dumps, wastelands, settlements, water bodies, etc., were delineated in three sites. The results indicate a marked decrease in forest area due to the expansion of coal mining at all the sites between 1994 and 2022. Comparatively a higher decrease in forest cover was found in Site III, followed by Site I and Site II. Forest cover was lost by 34%, 22% and 38% at Site I, Site 2 and Site 3, with in a span of 28 years. Nevertheless, the area under man-made plantations has increased with an increase in restoration activities in disturbed landscape especillay on mine over burdens. It increased by 4.30% in site I, 9.67% in site II and 2.03% in site III. The plantations were established in larger areas at site I followed by Site III compared to site I. The overall LULC destruction were higher in Site I and Site III mined landscape but quite less in site II. The waterbodies gradually dried up and disappeared due to the development of a network of new roads and built-up areas as settlements, coupled with the allocation of new coal blocks adjacent to the old ones. The higher losses of waterbodies were found in Site I and Site III, which accounted for 7.11% and 3.53%, respectively, while meagre losses of 1.55% were recorded in Site II. A very small decline in agricultural areas corresponds with an increase in settlement across the mining areas, clearly indicating the predominant loss of forest cover in favor of the expansion of mines in the study area. The natural forests, water bodies, and agricultural lands are remarkbly affected by coal mining and formation of huge overburden dumps. The overall disturbances in land use corresponding for the years 1994, 2007, and 2022 and the land use changes are illustrated and presented in the Supplementary Table S2. The land use/land cover maps were compared with reference data, prepared through the utilization of ground truth data, sample points, and Google Earth, achieving an overall classification accuracy exceeding 90% for all dates.
3.1 Soil and terrain index
The indexing method employed to determine the soil quality is classified into four categories: very poor, poor, good, and very good (Table 3). The mines located in Site I faced rapid land degradation, resulting in the majority of the area converted to very poor-quality soil between 1994 and 2007. The mines located in Site I experienced rapid land degradation, resulting in the majority of the area converted to very poor-soil quality between 1994 and 2007. However, soil amelioration in the later period from 2007 to 2022 led to a reduction in the area under this category, decreasing from 1604 ha to 1336 ha. Consequently, the area under very good quality soil was also enhanced from 1104 ha in 2007 to 1204 ha in 2022. In contrast, the soil quality at Site II mine exhibited continuous deterioration from 1994 to 2022. In 2022, very poor quality soil covers an area of 555 ha, an increase from 325 ha in 1997, while the extent of very good quality soil has decreased from 534 ha in 1994 to 301 ha in 2022. Unlike Site I, the land in Site III mines saw a continuous increase in soil degradation as mining expanded. Area under very good quality soil reduced from 1332 ha in 1994 to 1091 ha in 2022. Similarly, the area under very poor-quality soil also declined. The higher vulnerability of poor-quality soil to degradation is evident, and all three mines have shown an increase in the area where the soil is more susceptible to degradation (Table 3). The terrain index categorizes the study area into four primary slope classes: 0%–3%, 3%–12%, 12%–20%, and >20% slope. At Site I and Site III mined locations, have their maximum area under steep slopes, exceeding 20% slope, while at Site II mines, the maximum area falls within the less slope category (0%–3%). In both Site I and Site III, the land, under varying slopes follows the order: 0%–3% > 3–12% > 12–20%. Meanwhile at Site II, the order is 20% > 3–12% and 12%–30% categories. This value helps to analyze the steepness of the terrain, and it is evident as steepness in terrain is maximum in Site III followed by Site I and Site II.
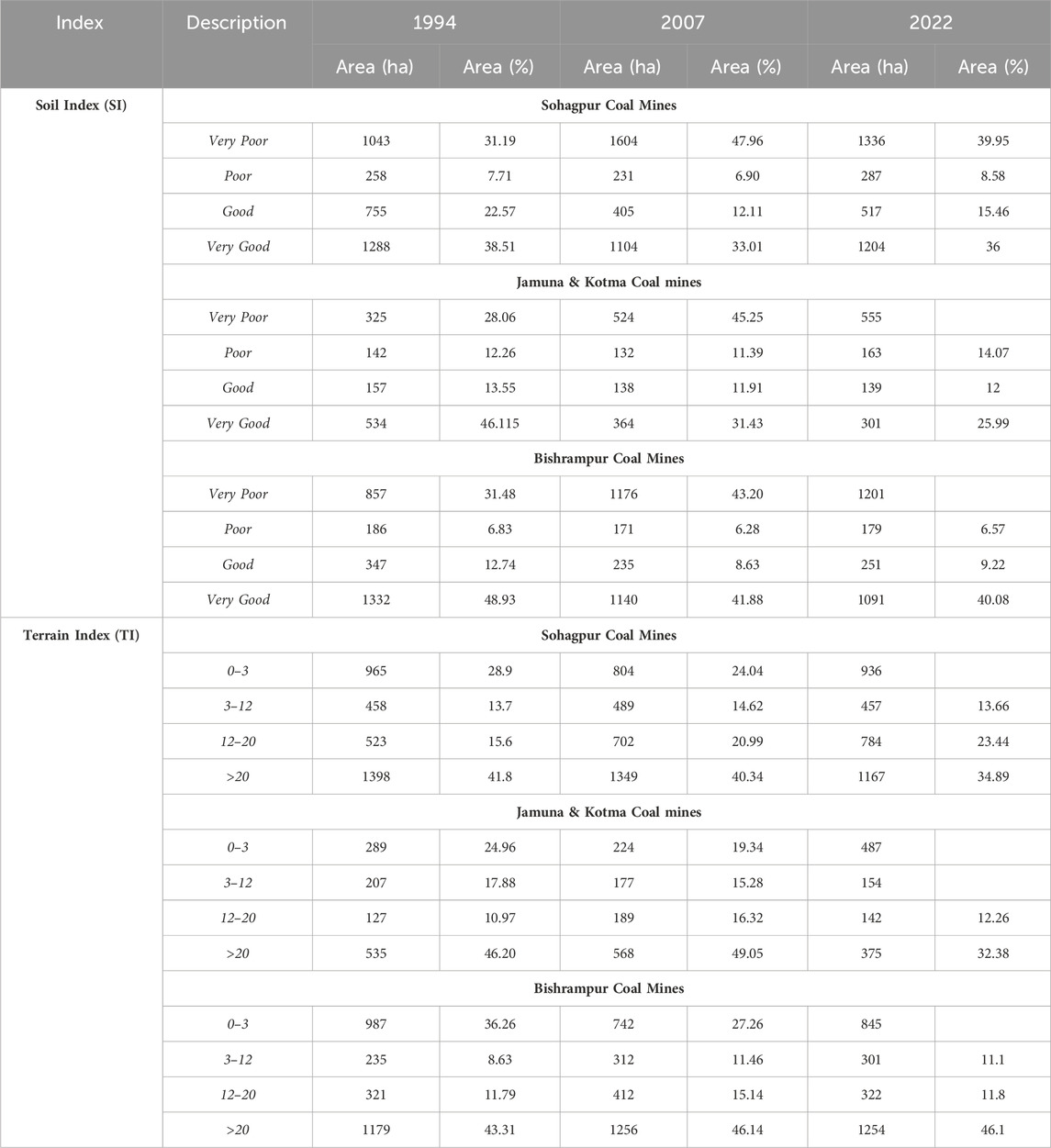
Table 3. Area under Soil Index (SI) classes (very poor, poor, good and very good) & Terrain Index (TI) (0–3, 3–12, 12–20, >20) classes for three coal mines for the year of 1994, 2007 and 2022.
3.2 Climate index (CI) and land utilization index (LUI)
The climate index helps to determine the area’s fragility in response to climatic aberrations. On the basis of climatic sensitivity, the area was classified into four classes: highly fragile, fragile, moderately fragile, and highly stable (Figure 4). In all mined sites, the area under high fragility increased, while the highly stable area decreased with the expansion of mining from 1994 to 2022. On-site, initially, over 63.03% of the area was classified as a fragile zone in 1994, while 46.98% of this classified area was later converted into a highly fragile zone by 2022. In Site II location, the area under climatically highly stable decreased between 1994 and 2022. An extent of the 575-ha area was categorized as a climatically fragile zone, whereas only a 246-ha area was classified under the highly stable category in 2022. The highly stable area has remained almost the same since 1994, but majority of the area classified as ‘fragile’ was changed to the highly climatically fragile class. In Site III, the maximum area of 845 ha was under the highly climatically fragile category, followed by stable category of 824 ha in 2022. Fragile regions are more vulnerable to degradation than non-fragile areas due to climatic aberrations, which are exacerbated by increased mining. This not only intensifies the vulnerability of local communities but also jeopardizes resource availability. The data about the change in land area under various classes are summarized in Table 4.
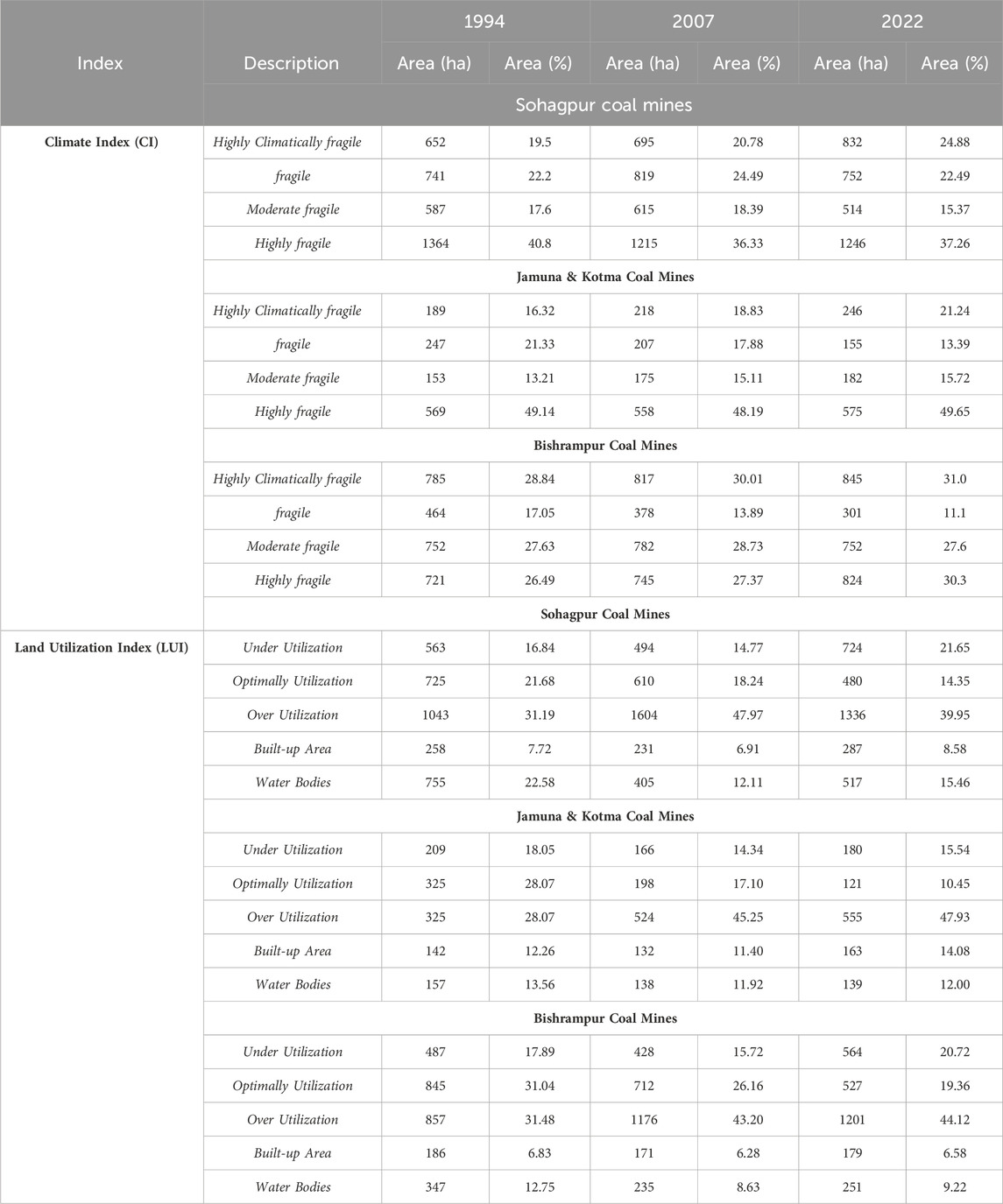
Table 4. Area under Climate Index (CI) categorized (highly climatically fragile, fragile, moderately fragile and highly fragile) and Land Utilization Index (LUI) (under-utilization, optimally-utilization, over-utilization, built-up area and water bodies) for three coal mines for the year of 1994, 2007 and 2022.
The Land Utilization Index (LUI) is useful for assessing land utilization in terms of sustainability, which identified five LUI classes: Underutilized, Optimally utilized, Over-utilized, Built-up area, and Water bodies (Figure 5). Site I experienced a decline in the area under water bodies and a simultaneous increase in built-up areas between 1994 and 2022. Furthermore, the land utilization pattern showed a paradigm shift, with the maximum land being over-utilized, increasing from 1043 ha in 1994 to 1336 ha in 2022. In contrast, at Site II, water bodies increased from 138 ha to 139 ha, and the built-up area expanded from 132 ha to 163 ha from 1994 to 2022. However, the increase in water body size was considerably less compared to the built-up area. The maximum land has been consistently over-utilized, reaching 555 ha by 2022, and this trend has been increasing since 1994. Similarly, at Site III, LUI results revealed an increase in the built-up area from 171 ha to 179 ha and water bodies from 235 ha to 251 ha between 2007 and 2022. The LUI showed that the majority of the study area was overutilized, with the greatest expansion occurring from 2007 to 2022 and resulting in an increase from 1176 ha to 1201 ha. The distribution pattern of areas under different categories of climate index and Land utilization index is presented in Table 4.
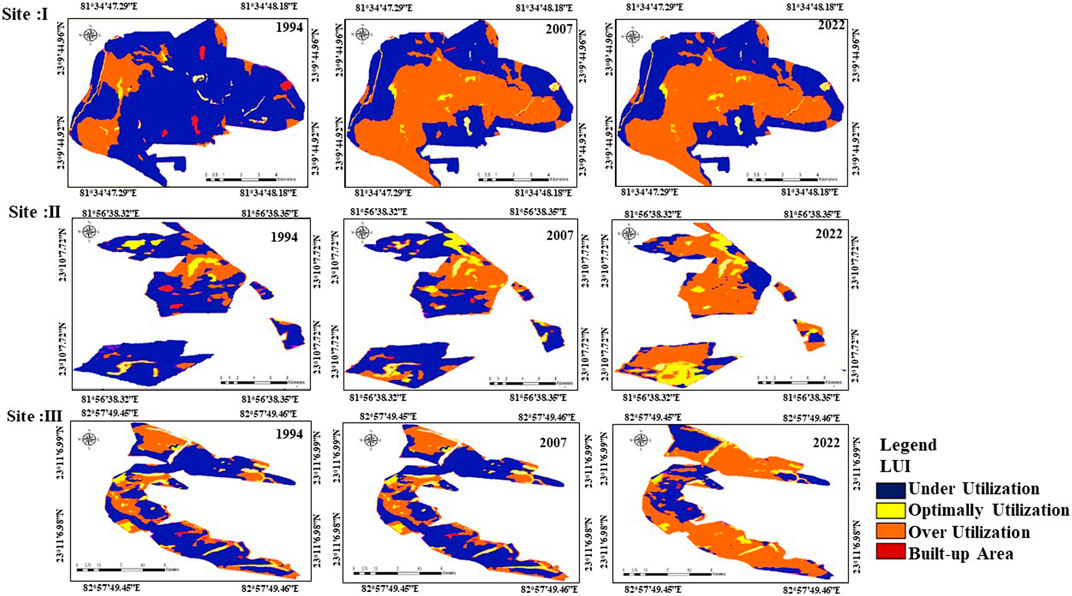
Figure 5. Land Utilization Index (LUI) of the study area (Site I, II and III) during the study period.
3.3 Land degradation vulnerability analysis
The LDVI generated from GIS based modelling was classified into six vulnerability classes: very low vulnerability, low vulnerability, moderate vulnerability, high vulnerability, forest/plantation, and water bodies. In the present study area, this index has been used to classify land in terms of vulnerability (Figure 6). At site I, a larger portion of the land, totaling 733 ha, was identified as high vulnerability, followed by 494 ha under low vulnerability and 385 ha under moderate vulnerability during 2022. At the same period, an extent of 1022 ha was under forest/plantation. Similarly, in Site II, the maximum land, comprising 507 ha, falls under the category of high vulnerability in 2022, while the forest/plantation covers approximately 198 ha. Since 1994, Site III have undergone a continuous increase in land under high vulnerability expanding to 1043 ha by 2022. The spatial distribution shows that highly vulnerable area are concentrated in the invicinty of the mines. In 2022, the forest cover in the study area was only about 81 ha, compared to 799 ha in 2007, as clearly illustrated in Figure 6.
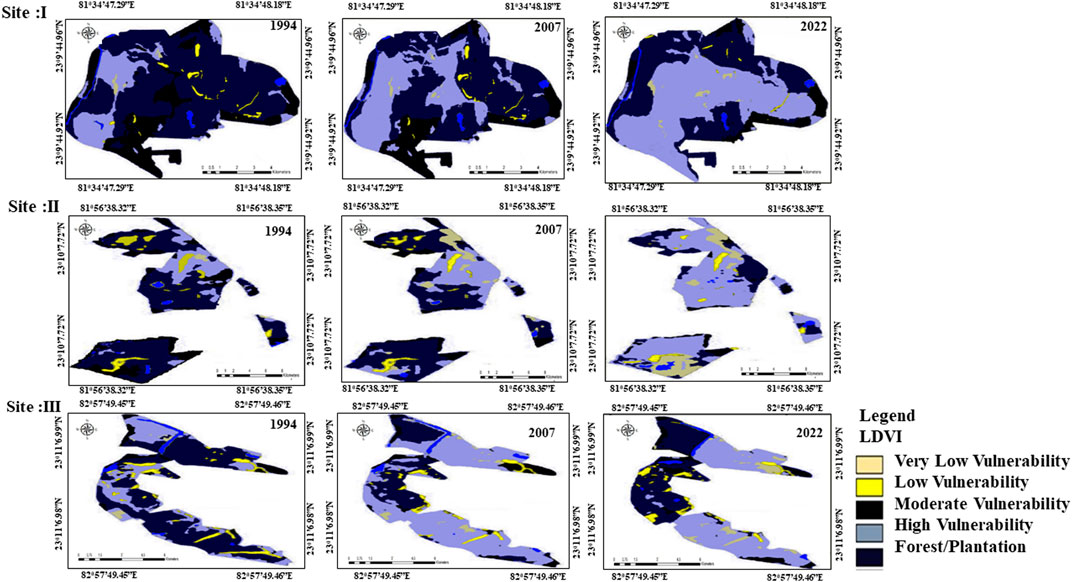
Figure 6. Land Degradation Vulnerability Index (LDVI) of the study area (Site I, II and III) during the study period.
4 Discussion
The results clearly indicate that geospatial analysis using Landsat satellite data, along with ancillary data, has proven to be an effective tool in quantifying land use changes and assessing land degradation in coal-mined landscapes. Studies conducted by Dubovyk (2017) and (Gao, et al., 2020) addressed intricacies involved in understanding the complex land degradation processes by harnessing remote sensing techniques. The potential capabilities of Landsat 4, 5, 7, and 8 in identifying land use dynamics in anthropogenically disturbed coal mine areas and analyzing physiographic, soil, climatic, and socio-economic indicators that influence the land degradation process were widely reported in the past (Pandey, et al., 2022; Thakur et al., 2021; Hong, et al., 2018). The results revealed that mining activities induced spatial and temporal changes in land use and land cover at different sites in the study region between 1994 and 2022. A significant amount (10.62%) of forest area was diverted to mining activities, settlements/bare surface, and plantation. The forest cover was rapidly lost due to intensified mining activities aimed at increasing coal production over a span of 28 years. The observed trends align with findings from other studies conducted in various regions of India (Garai and Narayana, 2018; Ahirwal et al., 2021) and within Central India (Thakur, et al., 2021; Pandey, et al., 2022). Studies consistently show declines in forest cover and waterbodies due to mining expansion for coal-related infrastructure like washeries, storage depots, and roads, leading to significant land and environmental degradation (Guan and Yu, 2021; Ferreira et al., 2022; Wang et al., 2023). Studies indicate that agricultural encroachment is the primary driver of forest degradation, with open-cast coal mining further exacerbating the issue by causing significant land-use changes. This transition often converts dense forests into open forests. In central India, a region abundant in coal, extensive mining activities have led to the widespread loss of natural ecosystems (Thakur et al., 2024).
Anthropogenic activities and socio-ecological factors drive land use change and land degradation in coal-mined landscapes (Romshoo et al., 2020; Abuzaid et al., 2021). This study highlights severe impacts on soil, climate, topography, and land use due to extensive mining over the past 25 years. Coal mining’s expansion, driven by rapid industrialization and energy demands, has negative implications for local ecosystems and communities (Ma et al., 2019; Ahirwal and Maiti, 2021; Rawat et al., 2022; Tariyal et al., 2022). Reinvesting in natural capital regeneration is essential in these mined regions (Hota and Behera, 2016). Mining significantly impacts soil, water, and the biosphere, as evidenced by soil quality degradation and water contamination (Kumar et al., 2022; Mishra et al., 2022; Pandey et al., 2022). Nutrient-rich topsoil removal from forests and agricultural lands deteriorates soil properties (Singh et al., 2010). Acid mine drainage in water bodies and heavy metal pollution are common consequences of mining activities (Equeenuddin et al., 2010). Soil physical and chemical properties are altered, leading to compaction, reduced fertility, and erosion risks (Frouz et al., 2011; Jing et al., 2018; Feng et al., 2019; Thakur et al., 2021). Effective reclamation and soil management strategies are crucial for mitigating land degradation vulnerability in mining areas (Mishra et al., 2022).
The terrain index indicates that natural terrain is being altered by anthropogenic coal mining activities, and the expanding area with moderate and steep slopes are increasing the degree of vulnerability to land degradation (Balakrishnan, 2019; Biswas et al., 2020). Mined over burdens reshapes topography both in terms of slope and elevation stimulate land degradation through increased water and wind erosion (Ahirwal and Maiti, 2021). Xiao, et al. (2020) monitored the changes in topographic features in the coal-mining areas of Fushun, China. They observed a maximum depth increase of 140 m, with an elevation rise of 10 m over the course of 10 years between 1996 and 2006. Dumping overbuden material near stream banks induces positive topographic alterations, signaling detrimental impacts on the hydrological system. Unfavorable relief changes manifest as profound depressions in coal mines resulting from coal extraction. These depressions transform into areas of water accumulation, leading to the wastage of both surface and groundwater resources. Soil degradation is caused by land deformation has also been documented in previous studies in India, Australia, and other countries (Frouz et al., 2011; Moomen and Dewan, 2016; Ahirwal and Maiti, 2021), which has increasingly become a worldwide concern.
The climate index has also shown that area under mining is highly vulnerable to climatic aberrations such as erratic rainfall pattern, high evaporation, high temperature, and low humidity conditions. Climate Change has been an emerging driver of mining policies adopted across the globe (Odell, et al., 2018). The anomalies in climatic variables are attributed to increased rates of albedo and insolation, as well as significantly reduced rates of evapotranspiration compared to unmined portions of the landscape (Wickham et al., 2007). Native plants are removed during mining operations, and since native trees seldom reestablish themselves in the harsh conditions of mines, they are often replaced by grasses and invasive alien species, which offer less protection. Previous studies have emphasized that coal mining activities are hazardous and are the main driving factors for spiking ambient temperature and a decrease in rainfall (Ahirwal and Maiti, 2021; Nie, et al., 2021). This is due to increased fugitive emissions, removal of vegetation, coal gangue fires, increased consumption of petroleum fuels for transportation, and occasional mine fires during blasting, drilling and removal of coal from open pits (Ahirwal and Maiti, 2021; Nie, et al., 2021). High temperatures and unpredictable rainfall pose health risks such as heat stroke, heart attacks, and fatigue, along with increasing the prevalence of epidemic diseases. Land use analysis reveals that extensive mining activities and expansion of coal allocation blocks have surpassed sustainable levels, leading to over-utilization of mineral resources and heightened land degradation concerns (Huang et al., 2018; Thakur T. K. et al., 2022). Global trends show a decline in non-degraded land availability while competing land uses continue to rise rapidly (Scholes et al., 2018). Prioritizing land use based solely on immediate economic gains neglects environmental and social considerations, contributing to widespread unsustainable practices in coal-mined regions. Optimal utilization of underutilized lands presents opportunities for maximizing benefits (Ahirwal and Maiti, 2021; Mishra et al., 2022; Pandey et al., 2022).
Land degradation is a key contributors to acceleration of climate change and has gained global attention for its adverse impacts on ecosystems and societies. Numerous studies have repeatedly warned that climate change can be further exacerbated if land degradation is not addressed at scale within the stipulated time frame (Scholes, et al., 2018; Kumar, et al., 2022; Mishra, et al., 2022; Pandey, et al., 2022). Despite growing evidence of land degradation being the primary cause for triggering socio-economic problems by endangering biological resources, food, nutrition, energy security, etc., still, comprehensive estimates on extent and types, underlying causes, and site-specific technologies are largely lacking for restoration of such landscapes (Xie, et al., 2020; Sreenivas, et al., 2021). Coal mining is an important economic activity that cannot be immediately closed, despite its adverse impacts on deforestation, land degradation, climate change, biodiversity loss, and disruptions in the lifestyles of people in central India, which is a rich repository of coal reserves (Thakur, et al., 2021; Mishra, et al., 2022; Pandey, et al., 2022). Due to deforestation, the global estimate of carbon emissions from forest degradation ranges from 40% to 212% (Gao, et al., 2020). According to Sreenivas et al., 2021, it has been reported that the per capita agricultural land in India is approximately 0.15 ha. It is estimated that this figure might further decline to 0.09 ha by the year 2075, with nearly 30% of the national area potentially suffering from the vagaries of land degradation. It is estimated that India is one of the major coal producers as well as consumer and her consumption pattern was predicted to rise by 2.6% by 2020 (Thakur et al., 2022a; b).
The LDVI, integrating climatic, edaphic, and topographic indices, identifies highly vulnerable areas near mining sites, aligning with reports of land degradation and soil quality deterioration (Martins et al., 2020). Vulnerability to degradation varies even under similar land use, influenced by factors like topography, soils, climate, and geology (Van der Werf and Petit, 2002; Nowak and Schneider, 2017). Tailoring land-use sensitivity, exposure, and capacity to withstand disruptions is crucial for sustainable development goals. As land use landscapes deteriorate internally and bear human disturbances, vulnerability becomes more evident. Site I shows lower vulnerability compared to Sites 2 and 3, where intensified mining has concentrated severely to extremely vulnerable areas. Despite their size and adaptive capacity, all areas face potential degradation. Identifying strategies to address vulnerability across landscapes is crucial to halt further degradation. Ecological restoration and green mining practices are advocated as priorities based on the extent of vulnerability to land degradation.
5 Ecorestoration strategies
The study reveals significant changes in LULC due to intensive mining and associated activities, leading to reductions in water bodies and forests. Land degradation worsens with disturbances in land cover, terrain alterations, and resource exploitation, increasing ecosystem fragility. Although mining authorities implement ad hoc eco-restoration plans, our research recommends prioritizing long-term, result-oriented bio-engineering techniques for the most vulnerable sites. Figure 7 comprehensively illustrates the complex interactions and eco-restoration measures necessary for sustainable development in disturbed landscapes affected by aggressive mining.
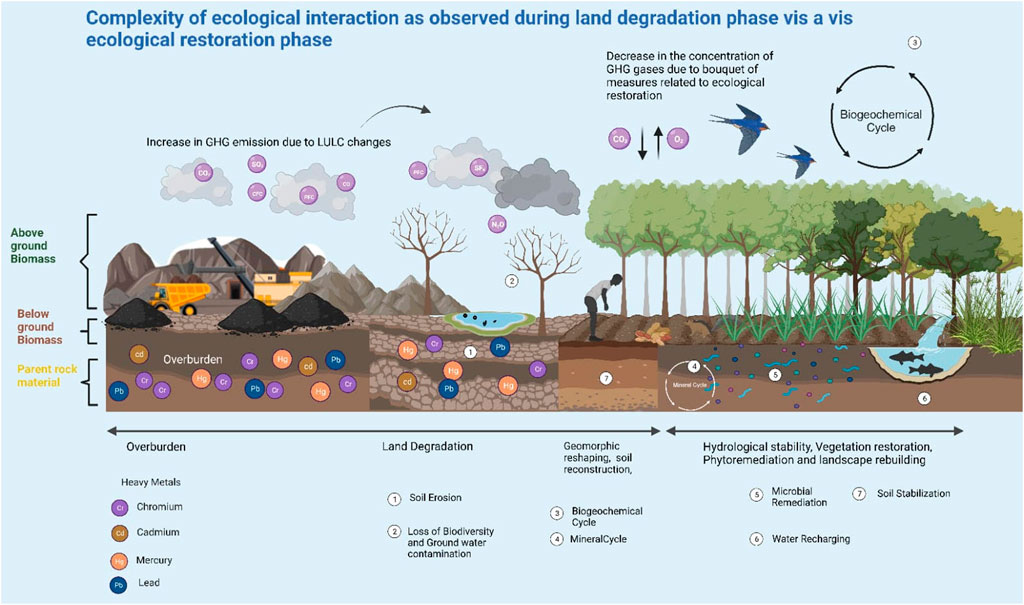
Figure 7. The figure illustrates the complexity of ecological interactions as observed during land degradation phase vis a vis ecological restoration phase in active coal mine sites of the study area. The figure stands on the pillar of UNCCD 2018–2030 Strategic Framework: a comprehensive international commitment to attain Land Degradation Neutrality (LDN) with three core objectives: (i) The restoration of productivity of degraded land, (ii) Enhancing the livelihoods of people dependent on them, (iii) Mitigating the impact of droughts on vulnerable populations.
5.1 Reclamation of vulnerable coal mined sites
The restoration of vulnerable coal mined sites requires a holistic and sequential planning. The core focus is to be given on developing nature-based solutions (Nbs) as proposed by International Union for Conservation of Nature and Natural Resources-Commission on Ecosystem Management (IUCN-CEM) (Fu, 2021; Thakur et al., 2024). The two-pronged strategy of any comprehensive eco-restoration plan includes both ecological integrity and socio-economic development (Gong, et al., 2020). Sustainable Ecosystem Restoration (SER) stands on the pillar of solving a cascade of cross-cutting issues such as regional ecological conflicts, reconstruction of damaged ecosystems, and Zero Ecosystem Degradation (Swamy and Darro. 2023; Suding, 2011). Disturbed landscapes as a result of coal mining and mine spoils demand urgent attention as a top priority site for implementing land reclamation as well as eco-restoration measures due to it is extreme vulnerability to land degradation. The path towards sustainable coal mining and reclamation advocates for a comprehensive Ecosystem Reclamation Approach (ERA) (Feng et al., 2019), which aims to rebuild ecosystem services previously provided by the landscape. The five distinct phases suggested for the reclamation of mine soil are as follows: i) geomorphic reshaping, ii) soil reconstruction, iii) hydrological stability, iv) vegetation restoration, v) phytoremediation, and vi) landscape rebuilding and approach for reclamation of degraded coal mine areas is illustrated in Figure 7.
Geomorphic reshaping is fundamental for achieving effective reclamation, guiding subsequent phases (Macdonald et al., 2015; Bandyopadhyay et al., 2020). At the microsite level, reclamation influences initial topography and contributes to long-term stability through soil texture and arrangement (Macdonald et al., 2015). Soil reconstruction addresses compaction, acidity, and carbon loss using natural and artificial methods (DeFries and Eshleman, 2004; Zipper et al., 2015). Organic additions aid nutrient cycling, while proper placement of salvaged materials restores soil quality (Frouz et al., 2011). Hydrological stability is tied to soil and vegetation restoration (Townsend et al., 2009; Macdonald et al., 2015; Zapico et al., 2018), with loose materials enhancing water flow and recharge (Frouz et al., 2011). Effective landform design manages moisture and water movement, supported by vegetation that mitigates erosion, requiring integrated erosion control approaches (Townsend et al., 2009).
The importance of vegetation in reclamation has been validated by prior studies (Frouz et al., 2011; Macdonald et al., 2015; Zipper et al., 2015; Pandey et al., 2022). Plant communities’ composition is influenced by landform changes, restoration techniques, hydrology, and landscape design, impacting ecosystem services and soil development. Enhanced plant-soil interactions promote nutrient cycling, soil biota development, infiltration, and water retention. Mixed plantations are recommended for resilience against pests and environmental stressors, fostering diverse habitats and reducing competition (Macdonald et al., 2015; Zapico et al., 2018). Despite slower establishment, mixed plantations improve surface conditions through synergistic soil-plant interactions. Landscape reconstruction and geomorphic reshaping occur across scales, affecting topography, hydrology, soil properties, and vegetation establishment (Macdonald et al., 2015). These processes, along with gardening and recreational methods, enhance mine soil properties and provide various ecosystem services.
Coal mining activities inadvertently contaminate the soil by releasing heavy metals such as arsenic (As), cadmium (Cd), chromium (Cr), copper (Cu), mercury (Hg), nickel (Ni), and lead (Pb) etc. Through waste dumping and the leaching of water from mine soils into the surrounding environment (Dutta and Zaman, 2022). These elements are toxic, creating stress conditions by interfering with biochemical or physiological processes obstruct uptake and translocation of nutrients and water, and affecting photosynthesis thus retarding growth and development of plants. Moreover, they are hazardous and even carcinogenic to human beings at mild concentrations can enhance ecological risk. The organic amendments, biofertilizers and mycorrihzal fungi along with suitable plant species are useful in remediation of polluted mine spoils. Phytoremediation is an economically and environmentally sound option, where plants are grown, to remove or stabilize the contaminated site by eliminating toxic heavy metal pollutants mainly by the process of phyto-stabilization phyto extraction and phytovolatilization to mitigate the risks and vulnerability (Figure 8). A proposed novel eco-restorative strategy through wetland plant Typha latifolia and HM phytoremediation using a micro-biotechnological approach for reclamation of degraded coal mine areas is illustrated in Figure 9. Plants employed for phytoremediation also have the ability to tolerate and accumulate multiple metals in their above-ground and belowground tissues (Mukherjee et al., 2017; Barya et al., 2020a; b). Phytoremediation is considered more effective for native microbial communities than human induced bioaugmentation (Bandyopadhyay et al., 2020; Thakur et al., 2023). The different mechanisms of Phytoremediation is depicted in Figure 8.
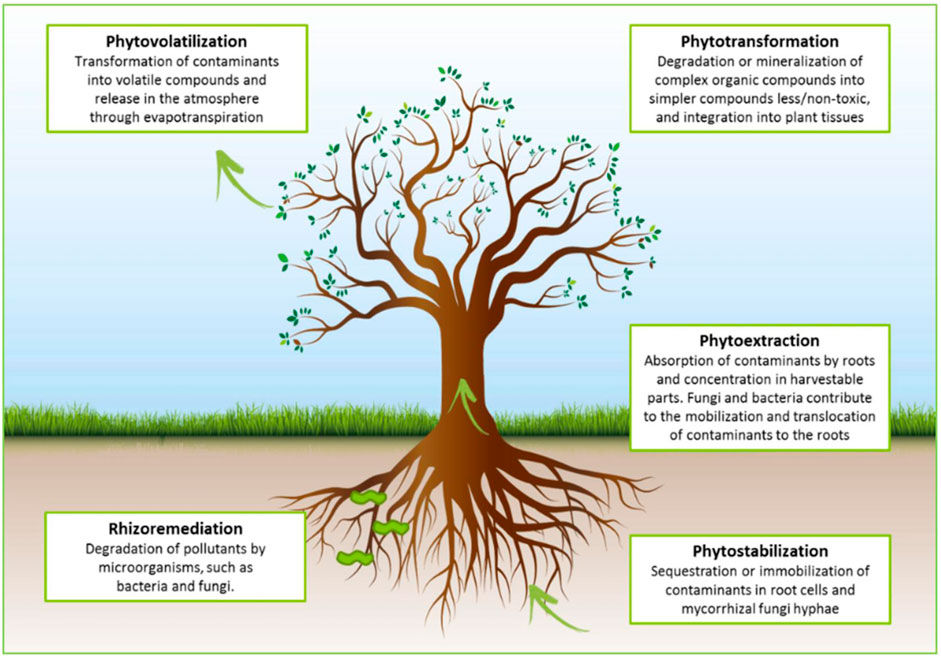
Figure 8. The figure depicts the mechanism of Phytoremediation and its types. (Figure adapted from Rohrbacher et al., 2016 and due permission taken from authors.).
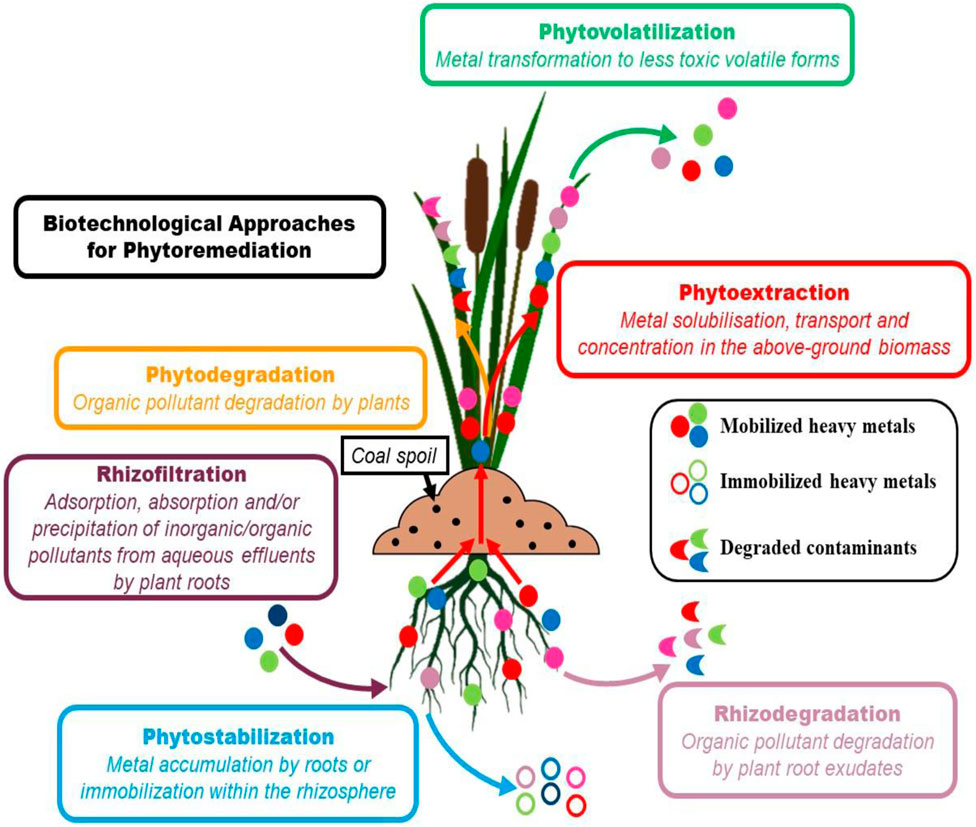
Figure 9. Different approaches of Phytoremediation employing the wetland plants are in practice for land reclamation of coal mine areas. The plant depicted in the figure is Typha latifolia used in phytoremediation across the world.
Phytostabilization involves heavy metal accumulation or valence reduction in the rhizosphere, limiting their mobility and absorption into root tissues or cell walls (Mukherjee et al., 2017; Bandyopadhyay et al., 2020). This process detoxifies soil health by plant defense mechanisms, stabilizing heavy metals and preventing leaching and dispersion (Mukherjee et al., 2017). Unlike phytoextraction, phytostabilization does not require disposing of hazardous biomass (Bandyopadhyay et al., 2020). Phytoextraction, a newer phytoremediation method, involves plants absorbing and storing heavy metals in their above-ground biomass (Ali et al., 2013; Zapico et al., 2018), offering a commercial and permanent decontamination solution by removing heavy metals from soil. Phytovolatilization, another phytoremediation process, transforms heavy metals like Hg, As, and Se into volatile forms within plants, subsequently releasing them into the atmosphere (Tangahu et al., 2011).
The plant species chosen for phytoremediation in coal mine-polluted sites should possess specific traits: fast growth, high biomass production, deep roots, natural hyperaccumulation ability, or genetic engineering (Ali et al., 2013). These plants detoxify heavy metals, improve soil quality, prevent erosion, resist pests, and enhance biodiversity, aiding in ecosystem self-sustainability. The native species of Albizia lebbeck, Albizia procera, Dalbergia sissoo and Dendrocalamus strictus along with local shrubs and grasses Saccharum spontaneum, Cynodon dactylon, Eulaliopsis binata, Croton oblongifolius, Lantana indica were found promising for reducing heavy metal concentrations over exotic Eucalyptus teriticornis and Leucaena leucocephala for phytoremediation in coal mine areas (Thakur et al., 2022c; Pandey et al., 2022), however site specific evaluation of species is necessary as the degree of decontamination depend interaction of site and environment. Further research is needed to understand the effects of heavy metal-enriched biomass from natural or transgenic hyperaccumulating trees on various ecological components. Phytoremediation methods can detoxify mining sites from heavy metals, enabling future agricultural or forestry use, enhancing ecosystem services, and benefiting local communities. Balancing the importance of coal mining for industrial growth in Central India with its significant environmental impacts necessitates a strategic approach to restoration. Effective governance must prioritize restoration efforts, considering the substantial hazards to both environment and human health. Specific mechanisms should be integrated to address the unique challenges posed by coal mining degradation. Comprehensive environmental development measures should be implemented alongside mining activities, requiring collaboration among mine authorities, local entities, environmental organizations, NGOs, and communities to execute targeted eco-restoration plans, particularly in highly vulnerable degraded sites. We propose an integrated approach involving the combinatorial use of grasses, shrubs, and trees, enhanced with biofertilizers and organic soil amendments, for phytobial remediation of toxic pollutants. This method aims to facilitate the reclamation and revegetation of degraded coal landscapes. By increasing the phytocover and improving topsoil quality, this strategy seeks to restore soil fertility and support the long-term ecological rehabilitation of these areas (Barya et al., 2020b; Thakur et al., 2024).
6 Conclusion
The study conducted a comprehensive assessment of coal mining’s impact on decadal land use dynamics and developed a Land Degradation Vulnerability Index (LDVI) to prioritize areas for eco-restoration. It serves as a benchmark for further research on mining-induced land degradation. Over 28 years, significant changes were observed in Land Use and Land Cover (LULC) due to increased mining activities, leading to the loss of natural forest areas and water bodies, and disruptions in agricultural land use. Despite reclamation efforts through tree plantations, the rate of land degradation remains high near mines and overburdens, indicating the need for integrated restoration approaches involving biological, engineering, and chemical methods.
Sustainable land management practices are recommended for moderately to low vulnerable lands, while highly vulnerable areas require intensified restoration efforts. Scaling up restoration operations with stakeholder involvement is essential for holistic development and achieving the Sustainable Development Goals (SDGs), particularly the goal of zero net land degradation by 2030. The study’s findings provide valuable insights for planners and policymakers to develop sustainable land management practices and implement effective reclamation measures for eco-restoration in coal-mined areas, promoting sustainable development.
Data availability statement
The original contributions presented in the study are included in the article/Supplementary Material, further inquiries can be directed to the corresponding author.
Author contributions
TT: Conceptualization, Data curation, Formal Analysis, Methodology, Writing–original draft, Writing–review and editing. SS: Conceptualization, Data curation, Investigation, Resources, Validation, Writing–review and editing. JD: Validation, Writing–review and editing. AT: Validation, Writing–review and editing. AM: Validation, Writing–review and editing. PS: Investigation, Writing–review and editing. AK: Conceptualization, Funding acquisition, Validation, Visualization, Writing–review and editing. BA: Project administration, Writing–review and editing. RK: Data curation, Visualization, Writing–review and editing.
Funding
The author(s) declare that financial support was received for the research, authorship, and/or publication of this article. This reaserch work is partially supporting by project number (RSP2024R414), King Saud University, Riyadh, Saudi Arabia.
Acknowledgments
The authors gratefully acknowledge the contributions of scientific illustrator Digvesh & Soma for their ideas in aesthetic development of the research paper. All the authors thank their respective institutions for encouraging them to do good research for greater human welfare thus consistently adding value behind the core objective of a sustainable tomorrow. TT and JD extends their gratitude to their respective team of research scholars and students who are the main driving force behind any research output from the respective labs. The authors sincerely thank Researchers Supporting Project Number (RSP2024R414), King Saud University, Riyadh, Saudi Arabia.
Conflict of interest
The authors declare that the research was conducted in the absence of any commercial or financial relationships that could be construed as a potential conflict of interest.
The author(s) declared that they were an editorial board member of Frontiers, at the time of submission. This had no impact on the peer review process and the final decision.
Publisher’s note
All claims expressed in this article are solely those of the authors and do not necessarily represent those of their affiliated organizations, or those of the publisher, the editors and the reviewers. Any product that may be evaluated in this article, or claim that may be made by its manufacturer, is not guaranteed or endorsed by the publisher.
Supplementary material
The Supplementary Material for this article can be found online at: https://www.frontiersin.org/articles/10.3389/fenvs.2024.1419041/full#supplementary-material
SUPPLEMENTARY FIGURE S1 | Temperature data of the study area during 1994, 2007 and 2022 for Site I, II and Site III.
SUPPLEMENTARY FIGURE S2 | Rainfall pattern of the study area during 1994, 2007 and 2022 for Site I, II and Site II.
References
Abuzaid, A. S., AbdelRahman, M. A. E., Fadl, M. E., and Scopa, A. (2021). Land degradation vulnerability mapping in a newly-reclaimed desert oasis in a hyper-arid agro-ecosystem using AHP and geospatial techniques. Agronomy 11, 1426. doi:10.3390/agronomy11071426
Ahirwal, J., Kumari, S., Singh, A. K., Kumar, A., and Maiti, S. K. (2021). Changes in soil properties and carbon fluxes following afforestation and agriculture in tropical forest. Ecol. Indic. 123, 107354. doi:10.1016/j.ecolind.2021.107354
Ahirwal, J., and Maiti, S. K. (2021). Restoring coal mine degraded lands in India for achieving the united nations-sustainable development goals. Restor. Ecol. 30. doi:10.1111/rec.13606
Ahmad, A., and Quegan, S. (2013). Comparative analysis of supervised and unsupervised classification on multispectral data. Appl. Math. Sci. 7 (74), 3681–3694. doi:10.12988/ams.2013.34214
Alemu, B. (2015). The effect of land use land cover change on land degradation in the highlands of Ethiopia. J. Environ. Earth Sci. 5 (1), 1–13.
Ali, H., Khan, E., and Sajad, M. A. (2013). Phytoremediation of heavy metals—concepts and applications. Chemosphere 91 (7), 869–881. doi:10.1016/j.chemosphere.2013.01.075
Anon, M. (2006). Dirty metal, mining communities and environment. Washington (DC): Earthworks: Oxfam America, 203–214.
Avtar, R., Komolafe, A. A., Kouser, A., Singh, D., Yunus, A. P., Dou, J., et al. (2020). Assessing sustainable development prospects through remote sensing: a review. Remote Sens. Appl. Soc. Environ. 20, 100402. Article 100402. doi:10.1016/j.rsase.2020.100402
Balakrishnan, S. (2019). Recombinant urbanization: agrarian-urban landed property and uneven development in India. Int. J. Urban Regional Res. 43 (4), 617–632. doi:10.1111/1468-2427.12790
Bandyopadhyay, S., Novo, L. A. B., Pietrzykowski, M., and Maiti, S. K. (2020). Assessment of forest ecosystem development in coal mine degraded land by using integrated mine soil quality index (IMSQI): the evidence from India. Forests 11 (12), 1310. doi:10.3390/f11121310
Barya, M. P., Gupta, D., Shukla, R., Thakur, T. K., and Mishra, V. K. (2020a). Phytoremediation of Heavy metals from mixed domestic sewage through vertical subsurface flow constructed wetlands planted with Acorus calamus and Canna indica. Curr. World Environ. 15 (3), 430–440. doi:10.12944/CWE.15.3.06
Barya, M. P., Gupta, D., Thakur, T. K., Shukla, R., Singh, G., and Mishra, V. K. (2020b). Phytoremediation performance of Acorus calamus and Canna indica for the treatment of primary treated domestic sewage through vertical subsurface flow constructed wetlands: a field-scale study. Water Pract. Technol. 15 (02), 528–539. doi:10.2166/wpt.2020.042
Biswas, S., Mukhopadhyay, B. P., and Bera, A. (2020). Delineating groundwater potential zones of agriculture-dominated landscapes using GIS based AHP techniques: a case study from Uttar Dinajpur district, West Bengal. Environ. Earth Sci. 79 (12), 302. doi:10.1007/s12665-020-09053-9
Bradford, A. (2018). Deforestation: facts, causes and effects. Available at: https://www.livescience.com/27692-deforestation.html.
Brown, D. G., Riolo, R., Robinson, D. T., North, M., and Rand, W. (2005). Spatial process and data models: toward integration of agent-based models and GIS. J. Geogr. Syst. 7, 25–47. doi:10.1007/s10109-005-0148-5
Darradi, Y., Saur, E., Laplana, R., Lescot, J.-M., Kuentz, V., and Meyer, B. C. (2012). Optimizing the environmental performance of agricultural activities: a case study in La Boulouze watershed. Ecol. Indic. 22, 27–37. doi:10.1016/j.ecolind.2011.10.011
Dasgupta, A., Sastry, K. L. N., Dhinwa, P. S., Rathore, V. S., and Nathawat, M. S. (2013). Identifying desertification risk areas using fuzzy membership and geospatial technique –A case study, Kota District, Rajasthan. J. Earth Syst. Sci. 122 (4), 1107–1124. doi:10.1007/s12040-013-0331-x
DeFries, R., and Eshleman, K. N. (2004). Land-use change and hydrologic processes: a major focus for the future. Hydrol. Process. 18, 2183–2186. doi:10.1002/hyp.5584
Dubovyk, O. (2017). The role of Remote Sensing in land degradation assessments: opportunities and challenges. Eur. J. Remote Sens. 50 (1), 601–613. doi:10.1080/22797254.2017.1378926
Dutta, J., Zaman, S., Thakur, T. K., Kaushik, S., Mitra, A., Singh, P., et al. (2022). Assessment of the bioaccumulation pattern of Pb, Cd, Cr and Hg in edible fishes of East Kolkata Wetlands, India. Saudi J. Biol. Sci. 29, 758–766. doi:10.1016/j.sjbs.2021.09.039
EPA (2003). National action plan to combat drought and desertification. Ghana: Environmental Protection Agency.
Equeenuddin, S. K., Tripathy, S., Sahoo, P., and Panigrahi, M. (2010). Hydrogeochemical characteristics of acid mine drainage and water pollution at Makum Coalfield, India. J. Geochem. Explor. 105, 75–82. doi:10.1016/j.gexplo.2010.04.006
Fang, L., Gao, R., Wang, X., Zhang, X., Wang, Y., and Liu, T. (2024). Effects of coal mining and climate-environment factors on the evolution of a typical Eurasian grassland. Environ. Res. 244, 117957. doi:10.1016/j.envres.2023.117957
Feng, Yu, Wang, J., Baia, Z., and Reading, L. (2019). Effects of surface coal mining and land reclamation on soil properties: a review. Earth-Science Rev. 191 (2019), 12–25. doi:10.1016/j.earscirev.2019.02.015
Ferreira, C. S. S., Seifollahi-Aghmiuni, S., Destouni, G., Ghajarnia, N., and Kalantari, Z. (2022). Soil degradation in the European Mediterranean region: processes, status and consequences. Sci. Total Environ. 805, 150106. Article 150106. doi:10.1016/j.scitotenv.2021.150106
Freer, J., McDonnell, J. J., Beven, K. J., Peters, N. E., Burns, D. A., Hooper, R. P., et al. (2002). The role of bedrock topography on subsurface storm flow. Water Resour. Res. 38 (12), 1269. doi:10.1029/2001WR000872
Frouz, J., Kalcik, J., and Velichova, V. (2011). Factors causing spatial heterogeneity in soil properties, plant cover, and soil fauna in a non-reclaimed post-mining site. Ecol. Eng. 37 (11), 1910–1913. doi:10.1016/j.ecoleng.2011.06.039
Fu, B. J. (2021). Several key points in territorial ecological restoration. Bull. Chin. Acad. Sci. 36 (01), 64–69. doi:10.16418/j.issn.1000-3045.20201222001
Gao, Y., Skutsch, M., Paneque-Gálvez, J., and Ghilardi, A. (2020). Remote sensing of forest degradation: a review. Environ. Res. Lett. 15 (10), 103001. doi:10.1088/1748-9326/abaad7
Garai, D., and Narayana, A. C. (2018). Land use/land cover changes in the mining area of Godavari coal fields of southern India. Egypt. J. Remote Sens. Space Sci. 21 (3), 375–381. doi:10.1016/j.ejrs.2018.01.002
Gong, Q. H., Zhang, H. O., Ye, Y. Y., and Yuan, S. X. (2020). Planning strategy of land and space ecological restoration under the framework of man-land system coupling: take the Guangdong-Hong Kong-Macao Greater BayArea as an example. Geogr. Res. 39 (09), 2176–2188. doi:10.11821/dlyj020200413
Grigio, A. M., Castro, A. F.De, Souto, M. V. S., Amaro, V. E., Vital, H., and Diodato, M. A. (2004). Use of remote sensing and geographical information system in the determination of the natural and environmental vulnerability of the Guamaré municipal district – rio Grande do Norte – northeast of Brazil. J. Coast. Res. SI 39, pg – Pg. Itajaí, S. C. – Braz. ISSN 07490208.
Guan, J., and Yu, P. (2021). Does coal mining have effects on land use changes in a coal resource-based city? Evidence from huaibei city on the north China plain. Int. J. Environ. Res. Public Health 18 (21), 11616. doi:10.3390/ijerph182111616
Hintjens, H. (2000). Environmental direct action in Australia: the case of Jabiluka Mine. Commun. Dev. J. 35, 377–390. doi:10.1093/cdj/35.4.377
Hong, S., Hao, J., Ai, D., Yang, L., Zhou, N., and Meng, P. (2013). Land consolidation strategies in Huang-Huai-Hai plain based on man-earth relationship. Trans. Chin. Soc. Agr. Eng. 29 (24), 251–259. doi:10.3969/j.issn.1002-6819.2013.24.033
Hota, P., and Behera, B. (2016). Opencast coal mining and sustainable local livelihoods in Odisha, India. Mineral. Econ. 29 (1), 1–13. doi:10.1007/s13563-016-0082-7
Huang, J., Guo, S., Zeng, G., Li, F., Gu, Y., Shi, Y., et al. (2018). A new exploration of health risk assessment quantification from sources of soil heavy metals under different land use. Environ. Pollut. 243, 49–58. doi:10.1016/j.envpol.2018.08.038
Jing, Z., Wang, J., Zhu, Y., and Feng, Y. (2018). Effects of land subsidence resulted from coal mining on soil nutrient distributions in a loess area of China. J. Clean. Prod. 177, 350–361. doi:10.1016/j.jclepro.2017.12.191
Joshi, P. K., Kumar, M., Midha, N., Yanand, V., and Wal, A. P. (2006). Assessing areas deforested by coal mining activities through satellite remote sensing images and gis in parts of Korba, Chattisgarh. J. Indian Soc. Remote Sens. 34 (4), 415–421. doi:10.1007/bf02990926
Khaledian, Y., Kiani, F., Ebrahimi, S., Brevik, E. C., and Aitkenhead-Peterson, J. (2016). Assessment and monitoring of soil degradation during land use change using multivariate analysis. Land Degrad. Dev. 28 (1), 128–141. doi:10.1002/ldr.2541
Khan, S., and Romshoo, S. A. (2008). Integrated analysis of geomorphic, pedologic and remote sensing data for digital soil mapping. J. Himal. Ecol. Sustain. Dev. 3, 90–94. doi:10.15373/22778160/june2014/31
Kumar, M., Kumar, A., Thakur, T. K., Sahoo, U. K., Konsam, B., Pandey, R., et al. (2022). Soil organic carbon estimation along an altitudinal gradient of chir pine forests in the Garhwal Himalaya, India: a field inventory to remote sensing approach. Land Degrad. Dev. 33, 3387–3400. doi:10.1002/ldr.4393
Kumari, R., Banerjee, A., Kumar, R., Kumar, A., Saikia, P., and Latif Khan, M. (2020). Deforestation in India: consequences and sustainable solutions. IntechOpen. doi:10.5772/intechopen.85804
Ma, K., Zhang, Y., Ruan, M., Guo, J., and Chai, T. (2019). Land subsidence in a coal mining area reduced soil fertility and led to soil degradation in arid and semi-arid regions. Int. J. Environ. Res. Public Health 16 (20), 3929. doi:10.3390/ijerph16203929
Macdonald, S. E., Landhäusser, S. M., Skousen, J., Franklin, J., Frouz, J., Hall, S., et al. (2015). Forest restoration following surface mining disturbance: challenges and solutions. New For. 46, 703–732. doi:10.1007/s11056-015-9506-4
Martins, W. B. R., Lima, M. D. R., Junior, U. D. O. B., Amorim, L. S. V. B., de Assis Oliveira, F., and Schwartz, G. (2020). Ecological methods and indicators for recovering and monitoring ecosystems after mining: a global literature review. Ecol. Eng. 145, 105707. doi:10.1016/j.ecoleng.2019.105707
Ministry of Coal (2021). Government of India. Available at: https://coal.gov.in/en/about-us/history-background (accessed on August 12, 2022).
Mishra, A., Swamy, S. L., Thakur, T. K., Kumar, A., and Pandey, M. (2022). Impact of coal mining on land use changes, deforestation, biomass and C losses in Central India: implications for offsetting CO2 emissions. Land Degrad. Dev. 33, 3731–3747. doi:10.1002/ldr.4419
Moomen, A. W., and Dewan, A. (2016). Assessing the spatial relationships between mining and land degradation: evidence from Ghana. Int. J. Min. Reclam. Environ. 31 (7), 505–518. doi:10.1080/17480930.2016.1188253
Mukherjee, P., Roychowdhury, R., and Roy, M. (2017). Phytoremediation potential of rhizobacterial isolates from Kans grass (Saccharum spontaneum) of fly ash ponds. Clean Technol. Environ. Policy 19 (5), 1373–1385. doi:10.1007/s10098-017-1336-y
Nie, X. R., Hu, Z. Q., Zhu, M. Q., and Ruan, Y. (2021). Research on temporal and spatial resolution and the driving forces of ecological environment quality in coal mining areas considering topographic correction. Remote Sens. 13 (14), 2815. doi:10.3390/rs13142815
Nowak, A., and Schneider, C. (2017). Environmental characteristics, agricultural land use, and vulnerability to degradation in Malopolska Province (Poland). Sci. Total Environ. 590 (591), 620–632. doi:10.1016/j.scitotenv.2017.03.006
Odell, S. D., Bebbington, A., and Frey, K. E. (2018). Mining and climate change: a review and framework for analysis. Extr. industries Soc. 5 (1), 201–214. doi:10.1016/j.exis.2017.12.004
Oskarsson, P., Krishnan, R., and Dutt, K. L. (2024). Living with coal in India: a temporal study of livelihood changes. Extr. Industries Soc. 17, 101437. doi:10.1016/j.exis.2024.101437
Pandey, M., Mishra, A., Swamy, S. L., Thakur, T. K., and Pandey, V. C. (2022). Impact of coal mining on land use dynamics and soil quality: assessment of land degradation vulnerability through conjunctive use of analytical hierarchy process and geospatial techniques. Land Degrad. Dev. 33, 3310–3324. doi:10.1002/ldr.4390
Planchon, O., and Darboux, F. (2002). A fast, simple and versatile algorithm to fill the depressions of digital elevation models. Catena 46 (2), 159–176. doi:10.1016/s0341-8162(01)00164-3
Rao, K. V., Rejani, R., Yogitha, P., Osman, M., Chary, G. R., Reddy, K. S., et al. (2016). Estimation of soil loss under changing climatic scenarios in semi-arid watersheds. Indian J. Dryland Agric. Res. Dev. 31, 89–95. doi:10.5958/2231-6701.2016.00015.4
Rawat, S., Khanduri, V. P., Singh, B., Riyal, M. K., Thakur, T. K., Kumar, M., et al. (2022). Variation in carbon stock and soil properties in different Quercus leucotrichophora forests of Garhwal Himalaya. Catena 213 (1), 106210. doi:10.1016/j.catena.2022.106210
Rohrbacher, F., and St-Arnaud, M. (2016). Root exudation: the ecological driver of hydrocarbon rhizoremediation. Agronomy 6 (1), 19. doi:10.3390/agronomy6010019
Romshoo, S. A., Amin, M., Sastry, K. L. N., and Parmar, M. (2020). Integration of social, economic and environmental factors in GIS for land degradation vulnerability assessment in the Pir Panjal Himalaya, Kashmir, India. Appl. Geogr. 125 (2020), 102307. doi:10.1016/j.apgeog.2020.102307
Sahu, H. B., and Dash, S. (2011) “Land degradation due to mining in India and its mitigation measures,” in Proceedings of second international conference on environmental science and technology. Singapore: Published by IEEE. Available at: http://dspace.nitrkl.ac.in/dspace/handle/2080/1411 (accessed on August 12, 2022).
Saini, V., Gupta, R. P., and Arora, M. K. (2016). Environmental impact studies in coalfields in India: a case study from Jharia coal-field. Renew. Sustain. Energy Rev. 53, 1222–1239. doi:10.1016/j.rser.2015.09.072
Scholes, R., Montanarella, L., Brainich, A., Barger, N., Ten Brink, B., Cantele, M., et al. (2018). The assessment report on Land degradation and restoration. Intergovernmental Science-Policy Platform on Biodiversity and Ecosystem Services. Available at: https://ipbes.net/assessment-reports/ldr (accessed on August 12, 2022).
Sethi, C., Hazra, B., Ostadhassan, M., Motra, H. B., Dutta, A., Pandey, J. K., et al. (2024). Depositional environmental controls on mechanical stratigraphy of barakar shales in rajmahal basin, India. Int. J. Coal Geol. 104477, 104477. doi:10.1016/j.coal.2024.104477
Singh, A. K., Mahato, M. K., Neogi, B., and Singh, K. K. (2010). Quality assessment of mine water in the raniganj coalfield area, India. Mine Water Environ. 29 (2010), 248–262. doi:10.1007/s10230-010-0108-2
Song, M., Zhao, X., and Shang, Y. (2020). The impact of low-carbon city construction on ecological efficiency: empirical evidence from quasi-natural experiments. Resour. Conserv. Recycl. 157, 104777. doi:10.1016/j.resconrec.2020.104777
Song, X. P., Hansen, M. C., Stehman, S. V., Potapov, P. V., Tyukavina, A., Vermote, E. F., et al. (2018). Global land change from 1982 to 2016. Nature 560 (7720), 639–643. doi:10.1038/s41586-018-0411-9
Sreenivas, K., Sujatha, G., Mitran, T., Suresh, K. G., Ravisankar, T., and Rao, P. V. N. (2021). Decadal changes in land degradation status of India. Curr. Sci. 121 (4), 539. doi:10.18520/cs/v121/i4/539-550
Suding, K. N. (2011). Toward an era of restoration in ecology: successes, failures, and opportunities ahead. Annu. Rev. 42 (1), 465–487. doi:10.1146/annurev-ecolsys-102710-145115
Swamy, S. L., Darro, H., Mishra, A., Lal, R., Kumar, A., and Thakur, T. K. (2023). Carbon stock dynamics in a disturbed tropical forest ecosystem of Central India: strategies for achieving carbon neutrality. Ecol. Indic. 154, 110775. doi:10.1016/j.ecolind.2023.110775
Tan, J., Zuo, J., Xie, X., Ding, M., Xu, Z., and Zhou, F. (2021). MLAs land cover mapping performance across varying geomorphology with Landsat OLI-8 and minimum human intervention. Ecol. Inf. 61, 101227. doi:10.1016/j.ecoinf.2021.101227
Tangahu, B. V., Siti, R., Basri, H., Idris, M., Anuar, N., and Muhammad, M. (2011). A review on heavy metals (as, Pb, and Hg) uptake by plants through phytoremediation. Int. J. Chem. Eng. 2011, 31. doi:10.1155/2011/939161
Tariyal, N., Bijalwan, A., Chaudhary, S., Singh, B., Dhanai, C. S., Tewari, S., et al. (2022). Crop production and carbon sequestration potential of grewia oppositifolia-based traditional agroforestry systems in Indian himalayan region. Land 11 (2022), 839. doi:10.3390/land11060839
Thakur, T., Swamy, S. L., Thakur, A., Mishra, A., Bakshi, S., Kumar, A., et al. (2024). Land cover changes and carbon dynamics in Central India's dry tropical forests: a 25-year assessment and nature-based eco-restoration approaches. J. Environ. Manag. 351, 119809. doi:10.1016/j.jenvman.2023.119809
Thakur, T. K. (2018). Diversity, composition and structure of understorey vegetation in the tropical forest of Achanakmaar Amarkantak Biosphere Reserve, India. Environ. Sustain. 1 (2), 279–293. doi:10.1007/s42398-018-00028-y
Thakur, T. K., Barya, M. P., Dutta, J., Mukherjee, P., Thakur, A., Swamy, S. L., et al. (2023). Integrated phytobial remediation of dissolved pollutants from domestic wastewater through constructed wetlands: an interactive macrophyte-microbe-based green and low-cost decontamination technology with prospective resource recovery. Water 15, 3877. doi:10.3390/w15223877
Thakur, T. K., Dutta, J., Bijalwan, A., and Swamy, S. L. (2022b). Evaluation of decadal land degradation dynamics in old coal mine areas of Central India. Land Degrad. Dev. 33, 3209–3230. doi:10.1002/ldr.4383
Thakur, T. K., Dutta, J., Upadhyay, P., Patel, D. K., Thakur, A., Kumar, M., et al. (2022c). Assessment of land degradation and restoration in coal mines of central India: a time series analysis. Ecol. Eng. 175, 106493. doi:10.1016/j.ecoleng.2021.106493
Thakur, T. K., Kripogu, K. K., Thakur, A., Kumar, A., Bakshi, S., Swamy, S. L., et al. (2022a). Disentangling forest dynamics for litter biomass production in biosphere reserve of Central India. Front. Env. Sci., 940614. doi:10.3389/fenvs.2022.940614
Thakur, T. K., Patel, D. K., Thakur, A., Kumar, A., Bijalwan, A., Bhat, J. A., et al. (2021). Biomass production assessment in a protected area of dry tropical forest ecosystem of India: a field to satellite observation approach. Front. Environ. Sci. 9, 757976. doi:10.3389/fenvs.2021.757976
Thakur, T. K., Swamy, S. L., Bijalwan, A., and Dobriyal, M. J. R. (2019). Assessment of biomass and net primary productivity of a dry tropical forest using geospatial technology. J. For. Res. 30 (1), 157–170. doi:10.1007/s11676-018-0607-8
Townsend, P. A., Helmers, D. P., Kingdon, C. C., McNeil, B. E., de Beurs, K. M., and Eshleman, K. N. (2009). Changes in the extent of surface mining and reclamation in the Central Appalachians detected using a 1976–2006 Landsat time series. Remote Sens. Environ. 113, 62–72. doi:10.1016/j.rse.2008.08.012
UNCCD (United Nations Convention to Combat Desertification) (2015). Land matters for climate: reducing the gap and approaching the target. Available at: https://www.unccd.int/resources/publications/land-matters-climate-reducing-gap-and-approaching-target (accessed on August 12, 2022).
Van der Werf, H. M. G., and Petit, J. (2002). Evaluation of the environmental impact of agriculture at the farm level: a comparison and analysis of 12 indicator-based methods. Agric. Ecosyst. Environ. 93 (1–3), 131–145. doi:10.1016/S0167-8809(01)00354-1
Wang, J., Zhen, J., Hu, W., Chen, S., Lizaga, I., Zeraatpisheh, M., et al. (2023). Remote sensing of soil degradation: progress and perspective. Int. Soil Water Conservation Res. 11 (3), 429–454. doi:10.1016/j.iswcr.2023.03.002
Weisse, M., and Goldman, E. D. (2017). Global tree cover loss rose 51 percent in 2016. Available at: https://www.wri.org/insights/global-tree-cover-loss-rose-51-percent-2016 (accessed on August 12, 2022).
Wickham, J. D., Riitters, K. H., Wade, T. G., Coan, M., and Homer, C. (2007). The effect of Appalachian mountaintop mining on interior forest. Landsc. Ecol. 22 (2), 179–187. doi:10.1007/s10980-006-9040-z
Xia, W., Apergis, N., Bashir, M. F., Ghosh, S., Doğan, B., and Shahzad, U. (2022). Investigating the role of globalization, and energy consumption for environmental externalities: empirical evidence from developed and developing economies. Renew. Energy 183, 219–228. doi:10.1016/j.renene.2021.10.084
Xiao, W., Guo, J., He, T., Lei, K., and Deng, X. (2023). Assessing the ecological impacts of opencast coal mining in Qinghai-Tibet Plateau-a case study in Muli coalfield, China. Ecol. Indic. 153 (2023), 110454. 1470-160X. doi:10.1016/j.ecolind.2023.110454
Xiao, W., Lv, X., Zhao, Y., Sun, H., and Li, J. (2020). Ecological resilience assessment of an arid coal mining area using index of entropy and linear weighted analysis: a case study of Shendong Coalfield, China. Ecol. Indic. 109, 105843. doi:10.1016/j.ecolind.2019.105843
Xie, H., Zhang, Y., Wu, Z., and Lv, T. (2020). A bibliometric analysis on land degradation: current status, development, and future directions. Land 9 (1), 28. doi:10.3390/land9010028
Yang, X. F., and Wen, X. P. (2011). Post classification comparison change detection of Guangzhou Metropolis, China. Key Eng. Mater. 467, 19–22. doi:10.4028/www.scientific.net/kem.467-469.19
Zapico, J. F., Martín, D., Bugosh, N., Laronne, J. B., Ortega, A., Molina, A., et al. (2018). Geomorphic reclamation for reestablishment of landform stability at a watershed scale in mined sites: the Alto Tajo Natural Park, Spain. Ecol. Eng. 111, 100–116. doi:10.1016/j.ecoleng.2017.11.011
Zhang, C., Ren, Z., and Wang, F. (2024). Compaction and seepage characteristics of broken coal and rock masses in coal mining: a review in laboratory tests. Rock Mech. Bull. 3 (2), 100102. doi:10.1016/j.rockmb.2024.100102
Zhao, X., Ma, X., Chen, B., Shang, Y., and Song, M. (2022). Challenges toward carbon neutrality in China: strategies and countermeasures. Conserv. Recycl. 176, 105959. doi:10.1016/j.resconrec.2021.105959
Keywords: vulnerability, LDVI, zero net land degradation (ZNLD), soil erosion, sustainable development, geospatial techniques
Citation: Thakur TK, Swamy SL, Dutta J, Thakur A, Mishra A, Sarangi PK, Kumar A, Almutairi BO and Kumar R (2024) Assessment of land use dynamics and vulnerability to land degradation in coal-mined landscapes of central India: implications for ecorestoration strategies. Front. Environ. Sci. 12:1419041. doi: 10.3389/fenvs.2024.1419041
Received: 18 April 2024; Accepted: 19 June 2024;
Published: 11 July 2024.
Edited by:
Merja H. Tölle, University of Kassel, GermanyReviewed by:
Mukhiddin Juliev, Tashkent Institute of Irrigation and Agricultural Mechanization Engineers (TIIAME), UzbekistanSaikat Sinha Ray, SRM University, India
Copyright © 2024 Thakur, Swamy, Dutta, Thakur, Mishra, Sarangi, Kumar, Almutairi and Kumar. This is an open-access article distributed under the terms of the Creative Commons Attribution License (CC BY). The use, distribution or reproduction in other forums is permitted, provided the original author(s) and the copyright owner(s) are credited and that the original publication in this journal is cited, in accordance with accepted academic practice. No use, distribution or reproduction is permitted which does not comply with these terms.
*Correspondence: Amit Kumar, YW1pdGtkYWhAbnVpc3QuZWR1LmNu