- 1School of Economics, Capital University of Economics and Business, Beijing, China
- 2School of Marxism, Central University of Finance and Economics, Beijing, China
- 3School of Economics, Jiangsu University of Technology, Changzhou, China
As an urbanization effort propelled by administrative measures, city-county merger has been particularly prevalent in the administrative district adjustments of prefecture-level cities in China. However, there has been scant research focusing on the policy’s impact on the efficiency of green utilization of urban land. We selected panel data from Chinese prefecture-level cities from 2003 to 2020, employing the slack-based measure (SBM) model that accounts for undesirable outputs, in conjunction with the Malmquist-Luenberger (ML) productivity index, to measure the efficiency of green land use in cities. Building on this, we utilized the staggered difference-in-differences (DID) model to investigate the impact of policies expanding cities through land leasing on the green utilization efficiency of urban land. Our findings indicate that the city-county merger has generally led to a decline in the efficiency of green utilization of urban land. Further mechanism analysis suggests that local governments’ excessive focus on land leasing for economic development, leading to a low-quality development model, is a significant factor contributing to the decline in green utilization efficiency of urban land. Specifically, the city-county merger policy indirectly reduces land green utilization efficiency by increasing industrial land leasing revenue, the number of industrial polluting enterprises, lowering the rationalization level of urban industrial structure, and raising the overachievement of urban economic growth targets. Further research reveals that the policy has heterogeneous impacts on land green utilization efficiency across different regions, city sizes, administrative levels, economic development levels, and urban planning types. The policy has a more significant inhibitory effect on land green utilization efficiency in non-eastern regions, smaller cities, peripheral cities, underdeveloped cities, and resource-based cities. Our study confirms that as a significant urbanization reform initiative, the effectiveness of the city-county merger still relies on the traditional extensive growth model based on land expansion, which is not conducive to enhancing the efficiency of green land use in cities.
1 Introduction
Land, as a finite and precious resource, plays a crucial role in the sustainable development of cities and the quality of life for urban inhabitants (Platt, 1995). Efficient green land use aims to minimize negative ecological impacts while fostering economic and social sustainability, optimizing land resource allocation, and enhancing land use efficiency (Pauleit and Duhme, 2000). It serves as a vital metric for assessing urban development sustainability and is pivotal in promoting cities’ green and low-carbon transformations. With urban populations and economic activities expanding, the continual enlargement of urban administrative districts has become a norm. This transformation not only alters cities’ spatial structures but also significantly impacts urban ecological environments, land resource allocation, and green land use efficiency.
In China, the city-county merger process, converting counties into city-administered districts, is a critical governmental tool to advance urbanization1. Unlike developed countries’ market-driven urban models, China’s urbanization is characterized by extensive, rapid, and government-led initiatives underpinned by public land ownership. The reorganization of administrative divisions has long been a focus of academic inquiry (Alesina et al., 1995; Hanes et al., 2012; Hirota and Yunoue, 2017). Proponents argue that merging administrative units breaks down market barriers, fosters agglomeration effects, and stimulates economic growth (Young, 2000; Tang and Hewings, 2017). Conversely, critics contend that such mergers can impede income growth (Hanes and Wikström, 2008), reduce economic efficiency (Blesse and Baskaran, 2016), inflate administrative costs (Zhang et al., 2023), and hinder economic development quality improvement (Hirota and Yunoue, 2017).
Academic research on land use efficiency has evolved from spatial patterns to decision-making processes and driving mechanisms (Ning et al., 2018; Platt, 1995; Zhou et al., 2020). Methodologically, evaluation indicators have shifted from single economic metrics to comprehensive multi-indicator systems integrating economic outputs and environmental impacts such as carbon emissions and land greening. Non-parametric methods like Data Envelopment Analysis (DEA) are adept at handling “multiple inputs + multiple outputs” scenarios, making them suitable for assessing urban land green utilization efficiency comprehensively (Jiao et al., 2020; Tan et al., 2021). Studies on influencing factors reveal that population size (Braid, 1988), resource endowment (Verburg et al., 2010), infrastructure (Cui et al., 2019), and land finance (Wang et al., 2021) affect land use efficiency. Urbanization is closely linked to land use, yet existing research primarily uses urban population ratios or industrial output proportions to measure urbanization levels, neglecting administratively driven “leapfrog” models like city-county mergers.
Since the 18th National Congress of the Chinese Communist Party, “ecological civilization construction” has been incorporated into the Party Constitution, elevating green development as a national priority. The “14th Five-Year Plan” emphasizes economic and intensive land use, and green urban development transformation. Despite ecological initiatives such as farmland protection and systematic restoration, challenges like high development intensity, soil pollution, and fragile ecosystems persist (Zhang et al., 2022). Research indicates significant room for improving China’s land green utilization efficiency (Li et al., 2022). By 2022, China’s urban area exceeded 64,000
Based on panel data from Chinese prefecture-level cities (2003–2020), this study employs a staggered Difference-in-Differences (DID) model to investigate how city-county mergers influence urban land green utilization efficiency and its underlying mechanisms. We explore heterogeneous effects across cities of varying regions, sizes, administrative levels, economic development stages, and urban planning types. Our findings contribute empirical insights into advancing urbanization, transforming economic models, and achieving sustainable urban land use.
2 Theoretical analysis
2.1 City-county mergers and urban land green utilization efficiency
Theoretically, expanding administrative divisions can break down barriers and market segmentation, aiding the integration of administrative zones and unifying urban planning and land use strategies (Sharifi et al., 2023). However, such expansions also increase urban land area, leading to disordered urban growth and reduced land use efficiency (Duranton and Puga, 2020). According to the “Regulations on the Management of Administrative Divisions” issued by the State Council, changes in administrative boundaries between counties and urban districts are proposed by local governments and approved by provincial authorities (State Council, 1985). Unlike market-driven processes in Western countries, administrative changes in China lack citizen participation. While this government-led urbanization method lowers decision-making costs, city-county mergers in underdeveloped areas often reduce efficiency, exacerbate pseudo-urbanization, and deepen urban-rural polarization. Moreover, local governments at all levels in China are responsible for urban construction and local economic development (Besley et al., 2022). In the GDP-oriented promotion model, local officials may prioritize rapid, unsustainable urban development driven by self-interest. Additionally, under state-owned land policies, local governments often use land leasing to intervene in economic activities and industrial layouts, which, alongside land-based financing and investment, accelerates capital and labor influx during city-county mergers but also results in resource waste and challenges sustainable land development (Gyourko et al., 2022).
Land green utilization efficiency seeks to maximize economic benefits while protecting the environment and optimizing resource allocation (Zhou and Lu, 2023). However, post-merger, accelerated urban expansion and infrastructure development often lead to land overdevelopment and ecological damage (Chung and Lam, 2009). Cities pursuing rapid economic growth tend to prioritize economic indicators through extensive land development and infrastructure, neglecting intensive land use and ecological concerns. This growth model may boost short-term economic metrics but can decrease land green utilization efficiency due to its extensive and unsustainable development practices and irrational spatial planning (Kalnay and Cai, 2003).
Specifically, city-county merger policies may reduce urban land economic efficiency, complicating resource allocation and reducing utilization efficiency (Jones and Hameiri, 2021). Imbalances in public infrastructure investment and research expenditures may further limit developmental potential, affecting overall economic efficiency in land use (Liu et al., 2024; Cai et al., 2022). Additionally, these policies may decentralize spatial development, triggering scattered industrial expansion and new agglomeration centers that weaken central city advantages, fostering irregular urban development (Liao et al., 2023; Rosenthal and Strange, 2020; Anas et al., 1998). Moreover, integrating formerly independent administrative units into municipal administrations increases development pressures on surrounding natural environments, potentially harming land vegetation health (Wellmann et al., 2020; Yu et al., 2018).
Therefore, we propose Hypothesis 1:
H1: City-county merger reforms reduce urban land green utilization efficiency.
2.2 City-county mergers and urban land green utilization efficiency: an analysis of mediating mechanisms
The city-county merger reform alters the authority over state-owned construction land leasing, enhancing the autonomy of prefecture-level governments over land leasing in former county areas, enabling them to generate substantial revenue from leasing residential and commercial land (Li, 2011). Concurrently, with the administrative division adjustments, prefecture-level governments, to promote land development and use within the former county areas, not only need to subsidize industrial land leasing to attract external capital but also need to increase municipal infrastructure investments to create conditions for attracting investments (Tiebout, 1956). This approach often involves large-scale land development and industrial project introduction, which, while boosting fiscal revenue and economic growth in the short term, leads to overdevelopment of land resources and a decline in utilization efficiency (Fritsch and Behm, 2021).
Under the pressures of officials’ accountability to higher authorities and career advancement, coupled with the increasing fiscal pressures, local governments, in pursuit of short-term economic growth goals, tend to attract high-pollution, high-energy-consumption, and high-emission manufacturing industries to the merged counties (Hong et al., 2020). While these enterprises boost industrial output and employment, they also increase regional material and energy consumption, raise pollution emission levels per unit output, and cause severe environmental pollution problems.
Rationalization of the industrial structure refers to the coordinated development and optimal allocation among industries, enhancing productivity and resource allocation efficiency across sectors (Hu et al., 2023). However, after the city-county merger, local governments often prioritize the development of high-yield industries such as industrial and real estate sectors to achieve rapid economic growth, neglecting the development of agriculture and services (Yang and Wu, 2015). This irrational industrial structure hinders coordinated development among sectors, reduces resource allocation efficiency, and thus affects land green utilization efficiency.
Economic growth is the most direct and primary goal of the city-county merger reform. At the beginning of the year, local governments in China typically set economic growth targets (usually the GDP growth rate) in their government work reports. After the merger, local governments often adopt aggressive economic policies and development strategies, including large-scale infrastructure construction and industrial project introduction, to achieve economic growth targets. This excessive pursuit of economic growth, while boosting economic figures in the short term, overlooks sustainable resource utilization and environmental protection (Yu et al., 2023).
Based on the above analysis, we propose Hypothesis 2:
H2: City-county merger reduces land green utilization efficiency indirectly by boosting urban industrial land leasing revenue, increasing the number of industrial polluters, lowering the rationalization level of urban industrial structure, and exacerbating the overachievement of urban economic growth targets.
2.3 City-county mergers and urban land green utilization efficiency: a heterogeneity analysis
The eastern regions of China possess early advantages in economic development, investment attraction, talent acquisition, and infrastructure construction. Consequently, these regions achieve more effective land resource utilization and efficient urbanization processes following city-county mergers (Jiao et al., 2020). In contrast, central and western regions encounter greater challenges in economic development and resource allocation due to weaker geographical and economic foundations post-merger.
Smaller cities, with limited population size, market scale, and resource aggregation capabilities, heavily rely on large-scale land development and industrial projects to attract investment and spur economic growth post-merger. However, inadequate infrastructure and public services hinder effective support for urban expansion and industrial upgrading (Hu and Fan, 2020). Moreover, resource constraints in smaller cities lead to increased conflicts of interest between city and county governments, resulting in higher administrative integration costs post-merger (Chung and Lam, 2009).
Under the current administrative system, cities with higher administrative ranks enjoy advantages in land acquisition, financial resources, and policy support. Peripheral cities (Non-sub-provincial or non-provincial capital cities) face disadvantages in administrative rank and resource acquisition capabilities (Gao et al., 2024). Post-merger, these cities encounter greater constraints and challenges in resource allocation and policy support compared to higher-ranked cities.
Less economically developed cities have limited financial resources and often prioritize projects with immediate economic stimulus over investments in technological innovation and ecological protection post-merger (Li and Lin, 2017). Conversely, economically developed cities emphasize quality economic development and environmental protection, utilizing scientific planning and technological innovation to enhance land green utilization efficiency.
Resource-based cities, reliant on natural resource extraction and processing industries such as minerals and forestry, lack incentives for improving resource utilization efficiency through technological innovation (Ruan et al., 2020). Post-merger, these cities continue to prioritize resource industries in land development and industrial growth, leading to deindustrialization and neglect of manufacturing and high-tech sectors (Yin and Miao, 2024). This results in resource misallocation and reduced land green utilization efficiency due to the entrenchment of production factors within the resource industry system.
Based on the above analysis, we propose Hypothesis 3:
H3: City-county mergers exert a more pronounced inhibitory effect on land green utilization efficiency in non-eastern regions, smaller cities, peripheral cities, underdeveloped cities, and resource-based cities.
Finally, Figure 1 provides a visual summary of the theoretical analysis discussed above.
3 Research design
3.1 Model setting
3.1.1 Data envelopment analysis
Data Envelopment Analysis (DEA) is a non-parametric statistical method used to evaluate the production efficiency of Decision Making Units (DMUs) across multiple inputs and outputs. Tone (2004) developed the Slacks-Based Measure (SBM) model incorporating undesirable outputs to assess economic and environmental efficiency. Building on this, we utilize the Malmquist-Luenberger (ML) productivity index to measure green land use efficiency (Boussemart et al., 2003).
Suppose there are
In Equation 1,
Based on the efficiency values derived from the SBM model incorporating undesirable outputs, the ML productivity index is computed to determine green land use efficiency for each DMU, as shown in Equation 2:
3.1.2 Staggered DID
The city-county merger reform in China presents characteristics akin to a quasi-natural experiment. Therefore, we apply a staggered difference-in-differences (DID) approach, controlling for individual and time fixed effects to mitigate potential sample selection biases. This method ensures the DID model’s objectivity, treating the treatment variable as quasi-random:
Here,
The parallel trends assumption is fundamental to the validity of the Difference-in-Differences (DID) method. It posits that, in the absence of intervention, the outcome variables for both the treatment and control groups would follow the same trend. The validity of this assumption is crucial for attributing the observed effects to the intervention rather than to other factors. Therefore, following the approach of Beck et al. (2010), we expand Equation 3 to conduct the parallel trends test:
In Equation 4,
3.1.3 Mediation effect model
The mediation effect model, often referred to simply as mediation, is utilized in statistical analysis to elucidate the mechanism by which an independent variable affects a dependent variable through one or more intermediary variables known as mediators (Zhao et al., 2010).
Here,
The mediation effect model is tested through the following steps: First, assess the direct impact of the explanatory variable, city-county merger, on the dependent variable, urban land green utilization efficiency. This involves testing the significance of the coefficient
3.2 Variable construction
3.2.1 Dependent variable
Urban Land Green Utilization Efficiency
We measure the urban land green utilization efficiency using the ML index calculated based on the SBM model. The input indicators include land, labor, capital, and energy, while the output indicators are categorized into undesirable and desirable outputs. The specifics of each indicator are detailed in Table 1.
3.2.2 Explanatory variable
City-County Merger Policy
3.2.3 Mechanism variables
In Equation 7,
In Equation 8,
In Equation 9,
3.2.4 Other control variables
Drawing upon existing literature (Zhou et al., 2020; Wang et al., 2021; Cui et al., 2019), we introduce the following variables to control for other factors affecting the efficiency of green land use in cities:
3.3 Data description
To ensure consistency, availability, and robustness of the data, we select panel data of Chinese prefecture-level cities from 2003 to 2020 as our research sample. Due to the nature of the Malmquist-Luenberger (ML) index calculation, which is akin to a “difference” analysis, our regression data start from 2004. To ensure the robustness of the results, the following data processing steps are taken: Firstly. Exclude cities that underwent city-county merger reforms before 2004. Secondly. Exclude the four municipalities of Beijing, Shanghai, Shenzhen, and Chongqing due to their administrative particularities. Thirdly. Given the significant variance in the month of city-county merger reforms and the time needed for position changes and department integration post-reform, we consider the following year of a reform that occurred in the fourth quarter as the actual year of policy implementation. Lastly. Use average growth rates to fill in minor missing data. Ultimately, we have 47 cities that underwent City-County Merger reforms (treatment group) and 102 cities that did not (control group).
Our data primarily comes from the following sources:
Descriptive statistics of the main variables used in this study are presented in Table 2.
4 Empirical results
4.1 Baseline results
Table 3 presents the baseline regression results of the city-county merger policy on the efficiency of urban land green utilization. Columns (1) and (2) display the regression outcomes without individual and time fixed effects, whereas columns (3) and (4) incorporate these effects. The results demonstrate that the city-county merger policy significantly reduces the efficiency of urban land green utilization, regardless of the model specification, thus confirming hypothesis H1. Specifically, as observed in column (4), holding other conditions constant, the efficiency of urban land green utilization in the treatment group cities decreased by 0.008 units compared to the control group cities. This indicates that the city-county merger policy, while expanding the urban land use area, notably diminishes the efficiency of urban land green utilization, suggesting that the urbanization promoted by this policy may compromise the green utilization efficiency of urban land.
4.2 Robustness test
4.2.1 Parallel trends test
The parallel trend hypothesis posits that, in the absence of policy impact on the treatment group (the counterfactual), the trends of outcome variables for both the treatment and control groups should exhibit no systematic differences over time. Figure 4 illustrates that the regression coefficients of
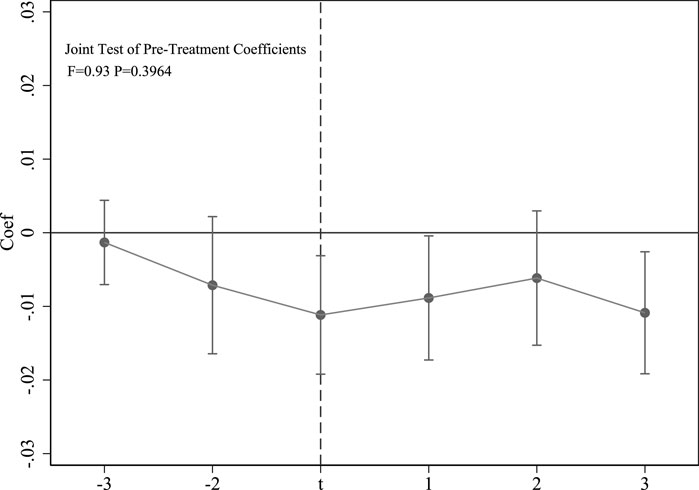
Figure 4. Parallel Trends Test. Note: The vertical axis represents the regression coefficients of
4.2.2 Excluding pandemic impact
The COVID-19 pandemic has significantly impacted economic output, potentially leading local governments to prioritize GDP growth over improvements in LGUE. This economic pressure might have prompted relaxations in regulations on polluting enterprises, thereby increasing undesirable outputs. To eliminate the influence of the pandemic on our regression analysis, we exclude data from the year 2019 onwards and re-run the regression. This approach ensures that our results are not biased by the pandemic’s unprecedented effects, providing a clearer depiction of underlying trends unaffected by this global event. The test results presented in the first column of Table 4 support the conclusions of this paper.
4.2.3 Changing the dependent variable
In our primary analysis, we utilized the Malmquist productivity index to measure Urban Land Green Utilization Efficiency (LGUE), emphasizing its ability to capture both technological progress and efficiency improvements. To validate the robustness of our findings, we also conducted tests using static efficiency scores. The results presented in the second column of Table 4 corroborate the conclusions drawn in this paper. This complementary approach provides a baseline measure of efficiency, strengthening confidence in our findings and ensuring that observed effects are not solely reliant on the chosen methodological framework.
4.2.4 PSM-DID test
In the robustness check section, we employ the PSM-DID (Propensity Score Matching DID) method to validate the causal effects identified in our primary analysis. PSM-DID enhances the credibility of our findings by addressing potential biases and confounders that could affect treatment effect estimations. The results presented in the third column of Table 4 reinforce the core conclusions of this paper. By matching units with similar characteristics, PSM ensures comparability between treated and control groups, thereby mitigating selection bias. Integrating PSM with DID provides a robust basis for causal inference, reaffirming our primary analysis results across different methodological frameworks.
4.3 Further analysis and mechanism examination
The city-county merger, as a policy promoted by local governments to expand administrative areas, has been observed to reduce the efficiency of green land use. However, it is crucial to clarify the underlying reasons for this effect. Investigating this issue not only helps validate the robustness of the aforementioned results but also aids in identifying the mechanisms at play.
4.3.1 Analysis of direct outcomes of city-county mergers
As discussed in the theoretical analysis, city-county mergers alter the structure of urban land use. Under pressure to achieve promotion, local governments may initiate large-scale infrastructure development to rapidly urbanize areas previously managed by counties. This process attracts factors such as labor and capital, stimulating economic growth. However, this factor-driven, extensive economic growth model tends to neglect environmental protection and does not contribute to improving economic growth quality. Therefore, our primary focus is to examine whether the city-county merger policy has fostered a development model that sacrifices the efficiency of green land use.
We investigated this phenomenon from three perspectives: fiscal expenditure, economic growth, and spatial structure.
Using Equation 3, we conducted regressions with urban government expenditure, urban road area, and urban fixed asset investment as dependent variables to explore the direct outcomes of city-county mergers on urban fiscal spending. The regression results are presented in Table 5, indicating a significant increase in urban fiscal expenditure, urban road area, and urban fixed asset investment due to the city-county merger policy. Next, regressions were performed with urban GDP and urban GDP per unit of land area as dependent variables to analyze the direct outcomes of city-county mergers on urban economic growth. Columns (1)–(2) of Table 6 show that while the city-county merger policy significantly boosted urban GDP, it also had a notable inhibitory effect on urban GDP per unit of land area. Finally, regressions with urban personal population density and urban NDVI as dependent variables aimed to explain the direct outcomes of city-county mergers on urban spatial structure. Columns (3)–(4) of Table 6 present empirical evidence indicating that city-county mergers significantly reduce urban personal population density and NDVI.
In summary, our findings demonstrate that the implementation of city-county merger policies boosted the total urban economy by increasing fixed asset investment and large-scale transportation infrastructure construction. However, this growth has been accompanied by a decrease in urban GDP per unit of land area and adverse impacts on population distribution, resource allocation, and urban ecological health. This form of pseudo-urbanization undermines genuine urban development goals and compromises the sustainable capacity of urban land, thereby validating H22.
4.3.2 Mechanism testing of mediation effects
The above analysis indicates that the city-county merger policy has led to a low-quality economic growth model. Furthermore, following Zhao et al. (2010), we employed a mediation effect model to test whether the city-county merger policy inhibits the improvement of urban land green utilization efficiency through increasing industrial land leasing revenue, the number of industrial polluting enterprises, lowering the rationalization level of urban industrial structure, and exacerbating the overachievement of urban economic growth targets.
The results of the mediation effect model are detailed in Table 7. Columns (1) and (2) of Table 7 reveal a significant increase in local industrial land leasing revenue due to the city-county merger policy, which subsequently hampers urban land green utilization efficiency. Thus, confirming the mediation effect of land leasing revenue. Columns (3) and (4) show a notable rise in heavy polluting enterprises following the policy, which significantly undermines urban land green utilization efficiency, confirming the mediation effect of competition in attracting investments. Columns (5) and (6) demonstrate that the policy increases the irrationality of the urban industrial structure, leading to a decrease in urban land green utilization efficiency. Hence, confirming the mediation effect of industrial structure rationalization. Columns (7) and (8) indicate that the policy contributes to the overachievement of urban economic growth targets, subsequently reducing land green utilization efficiency. Thus, confirming the mediation effect of overachieving economic growth targets.
Based on these findings, the mechanism testing of mediation effects reveals that the city-county merger policy indirectly diminishes land green utilization efficiency by enhancing industrial land leasing revenue, increasing the number of industrial polluting enterprises, lowering the rationalization level of urban industrial structure, and exacerbating the overachievement of urban economic growth targets. Therefore, confirming H2.
4.4 Heterogeneity analysis
4.4.1 Regional heterogeneity of cities
Given significant disparities in institutional environments and economic development levels across regions in China, we investigated the impact of the city-county merger policy on cities in Eastern China and those outside the Eastern region. Regression results are presented in columns (1) and (2) of Table 8. These findings indicate that the city-county merger policy significantly reduces land green utilization efficiency in non-Eastern cities, while showing no significant effect on land green utilization efficiency in Eastern cities.
4.4.2 City size heterogeneity
In large cities, the main urban area holds an absolute advantage over counties. In contrast, smaller cities exhibit narrower economic gaps between the main urban area and counties, with county economies sometimes surpassing those of the main urban area. This disparity leads to distinct administrative coordination challenges. Therefore, we examined the heterogeneous impact of the city-county merger policy on cities of varying sizes. Cities were categorized into large and small based on median population size for grouped regression analysis. Results are shown in columns (3) and (4) of Table 8, indicating that the city-county merger policy significantly reduces land green utilization efficiency in small cities, while showing no significant effect on large cities.
4.4.3 Urban administrative level heterogeneity
China’s administrative structure distinguishes between central cities (sub-provincial cities and provincial capital cities) and peripheral cities (ordinary prefecture-level cities), influencing administrative level and resource acquisition. This distinction implies differing impacts of the city-county merger policy on land green utilization efficiency in these two city types. We categorized cities into central and peripheral based on administrative level for grouped regression analysis. Results in columns (5) and (6) of Table 8 reveal that the city-county merger policy significantly reduces land green utilization efficiency in peripheral cities, while showing no significant effect on central cities.
4.4.4 Heterogeneity in urban economic development
Cities at different stages of economic development possess distinct economic foundations and development goals. Thus, we assessed the impact of the city-county merger policy on land green utilization efficiency across cities categorized as developed and underdeveloped based on median per capita GDP. Regression results in columns (7) and (8) of Table 8 indicate a significant inhibitory effect on land green utilization efficiency in cities with lower economic development levels, while showing no significant impact on cities with higher economic development levels.
4.4.5 Heterogeneity in urban planning types
Resource-based cities play a pivotal role in China’s economy but face unique challenges in sustainable development compared to non-resource-based cities. Reflecting on the “National Sustainable Development Plan for Resource-based Cities”, we categorized cities into resource-based and non-resource-based types. Results in columns (9) and (10) of Table 8 demonstrate that the city-county merger policy significantly reduces land green utilization efficiency in resource-based cities, while showing no significant impact on non-resource-based cities.
5 Discussion
5.1 Theoretical contributions
Firstly, this study extends the theoretical perspective of new economic geography on urban scale expansion, emphasizing agglomeration economies and economies of scale to optimize urban land intensive use. Existing research predominantly examines the economic impacts of urban scale expansion from a market perspective, neglecting the influence of local government behaviors (Frick and Rodríguez-Pose, 2018; Lu et al., 2021; Post and Kuipers, 2023). While some studies focus on the effects of city-county mergers in China, they primarily address economic development outcomes with limited attention to land intensive use (Chen et al., 2024). Our research focuses on the administrative expansion of cities through city-county mergers in China, empirically demonstrating that this policy inhibits improvements in land green utilization efficiency. This finding not only expands public policy factors influencing land intensive use during urban expansion but also offers policy recommendations for urban scale expansion from a green development perspective.
Secondly, this study explains why administrative expansion (city-county mergers) reduces urban land green utilization efficiency from the perspective of local government behaviors, enriching theories of fiscal decentralization and officials’ promotion tournaments. Our study moves beyond theories of enterprise heterogeneity (Naito, 2017), path dependence (Giannakis and Bruggeman, 2020), and industrial evolution (Clark and Sudharsan, 2020), expanding new economic geography theory from the viewpoint of local government behavior. Under China’s authoritarian system of fiscal decentralization, local officials prioritize economic scale to meet central government economic targets, often overlooking land intensive use. Moreover, competitive pressures among local governments, driven by promotion tournaments, lead to negative economic consequences that reduce land green utilization efficiency.
Thirdly, situated within the institutional context of a transitioning nation with a strong government, this study explores the heterogeneous impacts of urban scale expansion on land green utilization efficiency, deepening the study of land green utilization efficiency. Unlike research in developed Western countries focusing on voters (Hilber and Robert-Nicoud, 2013; Garnett, 2012), urban morphology (Wang and Debbage, 2021), and regulation (Pendall et al., 2018), our study delves deeper into the performance incentives of local officials regarding the effects of urban scale expansion on land green utilization efficiency. This approach offers insights into the fundamental drivers of land use dynamics and optimization.
5.2 Policy recommendations
Our research findings indicate that while the city-county merger policy has expanded urban development, it has also led to inefficient land green utilization. In 2022, the National Development and Reform Commission (NDRC) emphasized cautious and stringent control over city-county mergers. Based on our findings, the policy implications are as follows:
Streamline intergovernmental relationships: City-county mergers enhance overall economic development but decrease land green utilization efficiency. Policies should promote coordination across government levels and integrate urban planning to align the goals of city, county, and district administrations. By preventing conflicts of interest and administrative redundancies, sustainable land use can be ensured. Adjusting GDP-focused performance criteria is essential to drive governmental function transformation and system reform.
Prioritize long-term planning: Mechanism analysis reveals that city-county mergers often lead to inefficient land green utilization due to expansive, short-term development goals. China should establish a comprehensive evaluation framework emphasizing long-term planning and environmental sustainability. Financial incentives should encourage cities to adopt green infrastructure and renewable energy initiatives. Supporting local green technology startups can foster innovation in sustainable practices and industrial upgrading.
Adapt policies to local contexts: Empirical results highlight varied impacts across regions, with greater inefficiencies observed in non-Eastern, smaller, economically underdeveloped, and resource-based cities. China’s city-county merger policy must account for regional disparities. Tailored policies should balance local contexts with overarching developmental goals, ensuring cautious implementation where conditions are less favorable for mergers.
5.3 Limitations and future directions
Enhancement and comparison of research scope. In 2022, the National Development and Reform Commission (NDRC) introduced stricter controls on city-county mergers to curb rapid and unplanned urban expansion. This policy adjustment has the potential to mitigate some of the observed inefficiencies in land green utilization highlighted in our study. Consequently, these changes could significantly influence our conclusions. Future research should compare the impacts on land green utilization efficiency between cities that underwent city-county mergers before and after 2022. This comparison would facilitate an assessment of how stricter controls affect land use practices, economic growth, and environmental sustainability.
Refinement of research methods. We employed the SBM-ML method to assess land green utilization efficiency and the staggered DID method to examine the effects of city-county mergers. While these methodologies yielded valuable insights, future studies could benefit from real-time and more granular data on land use patterns and urban development, leveraging advancements in 5G, artificial intelligence, big data, and machine learning. These technological advancements offer the potential for more precise evaluations and deeper insights into the impacts of city-county mergers on land green utilization efficiency.
Limited scope of study subjects. Our study concentrated on eligible prefecture-level cities in China, characterized by a distinctive administrative system. While this approach provides valuable insights into city-county mergers in the Chinese context, it limits the generalizability of our findings to countries with different administrative structures and urbanization processes. Future research should incorporate comparative analyses involving countries with both similar and dissimilar administrative frameworks. This broader approach will contribute to a more comprehensive understanding of how diverse administrative systems influence land green utilization efficiency.
6 Conclusion
The city-county merger policy represents a prominent strategy employed by governments in China to foster urbanization through expansive land use. Given the current context where ecological improvements lag behind urban economic development, it is imperative to scrutinize the impact of city-county mergers on land green utilization efficiency within the framework of urban expansion. Using panel data spanning from 2003 to 2020, we assessed urban land green utilization efficiency using the SBM-ML method. Subsequently, we applied a staggered DID approach to analyze the effects and mechanisms of city-county mergers on urban land green utilization efficiency. In summary, our study yields the following key findings:
First, the city-county merger policy significantly inhibits urban land green utilization efficiency. Second, compared to cities without city-county mergers, those implementing this policy have increased fiscal expenditure, road area, fixed asset investment, and GDP, but significantly reduced land GDP, personal population density, and NDVI. Third, mediation effect tests reveal that the city-county merger policy worsens land green utilization efficiency by increasing industrial land leasing revenue, the number of industrial polluting enterprises, lowering the rationalization level of industrial structure, and exacerbating the overachievement of economic growth targets. Fourth, heterogeneity analysis indicates that the detrimental effect of the city-county merger policy on land green utilization efficiency is more pronounced in non-Eastern regions, smaller cities, peripheral cities, economically underdeveloped cities, and resource-based cities.
Data availability statement
The original contributions presented in the study are included in the article/Supplementary Material, further inquiries can be directed to the corresponding author.
Author contributions
MZ: Conceptualization, Formal Analysis, Methodology, Software, Visualization, Writing–original draft, Writing–review and editing. XX: Conceptualization, Funding acquisition, Validation, Writing–review and editing. YL: Conceptualization, Writing–review and editing.
Funding
The author(s) declare that financial support was received for the research, authorship, and/or publication of this article. This study was funded by the Beijing Research Center for Xi Jinping Thought on Socialism with Chinese Characteristics for a New Era: The Historical Experiences of the Communist Party of China Leading the Chinese People to Bring about the Great Rejuvenation of the Chinese Nation (No: 20LLZZB023).
Conflict of interest
The authors declare that the research was conducted in the absence of any commercial or financial relationships that could be construed as a potential conflict of interest.
Publisher’s note
All claims expressed in this article are solely those of the authors and do not necessarily represent those of their affiliated organizations, or those of the publisher, the editors and the reviewers. Any product that may be evaluated in this article, or claim that may be made by its manufacturer, is not guaranteed or endorsed by the publisher.
Supplementary material
The Supplementary Material for this article can be found online at: https://www.frontiersin.org/articles/10.3389/fenvs.2024.1418982/full#supplementary-material
Footnotes
1For detailed policy background, please refer to our Supplementary Material.
2The theoretical rationale posits that city-county merger policies have triggered a low-quality urban development pattern, compromising green land utilization efficiency. These policies drive fiscal expenditures, particularly in infrastructure, fostering economic growth at the expense of environmental quality. This process damages internal agglomeration economies, promotes urban sprawl, and adversely affects urban vegetation health.
References
Alesina, A., Perotti, R., and Spolaore, E. (1995). Togetheror separately? Issues on the costs and benefits of political and fiscal unions. Eur. Econ. Rev. 39, 751–758. doi:10.1016/0014-2921(95)00082-O
Anas, A., Arnott, R., and Small, K. A. (1998). Urban spatial structure. J. Econ. Literature 36, 1426–1464.
Beck, T., Levine, R., and Levkov, A. (2010). Big bad banks? The winners and losers from bank deregulation in the United States. J. Finance 65, 1637–1667. doi:10.1111/j.1540-6261.2010.01589.x
Besley, T., Burgess, R., Khan, A., and Xu, G. (2022). Bureaucracy and development. Annu. Rev. Econ. 14, 397–424. doi:10.1146/annurev-economics-080521-011950
Blesse, S., and Baskaran, T. (2016). Do municipal mergers reduce costs? Evidence from a German federal state. Regional Sci. Urban Econ. 59, 54–74. doi:10.1016/j.regsciurbeco.2016.04.003
Boussemart, J.-P., Briec, W., Kerstens, K., and Poutineau, J.-C. (2003). Luenberger and malmquist productivity indices: theoretical comparisons and empirical illustration. Bull. Econ. Res. 55, 391–405. doi:10.1111/1467-8586.00183
Braid, R. M. (1988). Optimal spatial growth of employment and residences. J. Urban Econ. 24, 227–240. doi:10.1016/0094-1190(88)90040-X
Cai, H., Tong, Z., Xu, S., Chen, S., Zhu, P., and Liu, W. (2022). Fiscal decentralization, government behavior, and environmental pollution: evidence from China. Front. Environ. Sci. 10. doi:10.3389/fenvs.2022.901079
Chen, G., Shan, Y., Hu, Y., Tong, K., Wiedmann, T., Ramaswami, A., et al. (2019). Review on city-level carbon accounting. Environ. Sci. and Technol. 53, 5545–5558. doi:10.1021/acs.est.8b07071
Chen, S., You, C., and Wu, J. (2024). The value of transition periods: do city-county mergers promote economic development? J. Urban Aff., 1–18. doi:10.1080/07352166.2024.2302471
Chung, J. H.,, and Lam, T.-c. (2009). China’s local administration: traditions and changes in the sub-national hierarchy (London: Routledge). doi:10.4324/9780203871065
Clark, J., and Sudharsan, S. (2020). Firm strategies and path dependencies: an emerging economic geography of industrial data. Reg. Stud. 54, 634–646. doi:10.1080/00343404.2019.1619926
Cui, X., Fang, C., Wang, Z., and Bao, C. (2019). Spatial relationship of high-speed transportation construction and land-use efficiency and its mechanism: case study of Shandong Peninsula urban agglomeration. J. Geogr. Sci. 29, 549–562. doi:10.1007/s11442-019-1614-1
Den, X., and Gibson, J. (2020). Sustainable land use management for improving land eco-efficiency: a case study of Hebei, China. Ann. OPERATIONS Res. 290, 265–277. doi:10.1007/s10479-018-2874-3
Di, Z., Fengnian, Z., and Xueqin, W. (2019). Impact of low-carbon pilot policy on the performance of urban carbon emissions and its mechanism. Resour. Sci. 41, 546–556. doi:10.18402/resci.2019.03.12
Dong, Y., Jin, G., and Deng, X. (2020). Dynamic interactive effects of urban land-use efficiency, industrial transformation, and carbon emissions. J. Clean. Prod. 270, 122547. doi:10.1016/j.jclepro.2020.122547
Duranton, G., and Puga, D. (2020). The economics of urban density. J. Econ. Perspect. 34, 3–26. doi:10.1257/jep.34.3.3
Frick, S. A., and Rodríguez-Pose, A. (2018). Big or Small Cities? On city size and economic growth. Growth Change 49, 4–32. doi:10.1111/grow.12232
Fritsch, M., and Behm, S. (2021). Agglomeration and infrastructure effects in land use regression models for air pollution – specification, estimation, and interpretations. Atmos. Environ. 253, 118337. doi:10.1016/j.atmosenv.2021.118337
Gao, M., Cifuentes-Faura, J., Liu, X., and Li, H. (2024). Do high administrative-level cities promote firm technological innovation? A novel analysis based on patent applications. Manag. Decis. Econ. doi:10.1002/mde.4279
Giannakis, E., and Bruggeman, A. (2020). Regional disparities in economic resilience in the European Union across the urban–rural divide. Reg. Stud. 54, 1200–1213. doi:10.1080/00343404.2019.1698720
Gyourko, J., Shen, Y., Wu, J., and Zhang, R. (2022). Land finance in China: analysis and review. China Econ. Rev. 76, 101868. doi:10.1016/j.chieco.2022.101868
Hanes, N., and Wikström, M. (2008). Does the local government structure affect population and income growth? An empirical analysis of the 1952 municipal reform in Sweden. Reg. Stud. 42, 593–604. doi:10.1080/00343400701281311
Hanes, N., Wikström, M., and Wångmar, E. (2012). Municipal preferences for state-imposed amalgamations: an empirical study based on the Swedish municipal reform of 1952. Urban Stud. 49, 2733–2750. doi:10.1177/0042098011429488
Henderson, J. V., Nigmatulina, D., and Kriticos, S. (2021). Measuring urban economic density. J. Urban Econ. 125, 103188. doi:10.1016/j.jue.2019.103188
Hilber, C. A. L., and Robert-Nicoud, F. (2013). On the origins of land use regulations: theory and evidence from US metro areas. J. Urban Econ. 75, 29–43. doi:10.1016/j.jue.2012.10.002
Hirota, H., and Yunoue, H. (2017). Evaluation of the fiscal effect on municipal mergers: quasi-experimental evidence from Japanese municipal data. Regional Sci. Urban Econ. 66, 132–149. doi:10.1016/j.regsciurbeco.2017.05.010
Hong, Y., Lyu, X., Chen, Y., and Li, W. (2020). Industrial agglomeration externalities, local governments’ competition and environmental pollution: evidence from Chinese prefecture-level cities. J. Clean. Prod. 277, 123455. doi:10.1016/j.jclepro.2020.123455
Hu, H., Pan, L., Jing, X., Li, G., Zhuo, Y., Xu, Z., et al. (2022). The spatiotemporal non-stationary effect of industrial agglomeration on urban land use efficiency: a case study of yangtze river delta, China. Land 11, 755. doi:10.3390/land11050755
Hu, L., Yuan, W., Jiang, J., Ma, T., and Zhu, S. (2023). Asymmetric effects of industrial structure rationalization on carbon emissions: evidence from thirty Chinese provinces. J. Clean. Prod. 428, 139347. doi:10.1016/j.jclepro.2023.139347
Hu, W., and Fan, Y. (2020). City size and energy conservation: do large cities in China consume more energy? Energy Econ. 92, 104943. doi:10.1016/j.eneco.2020.104943
Jiao, L., Xu, Z., Xu, G., Zhao, R., Liu, J., and Wang, W. (2020). Assessment of urban land use efficiency in China: a perspective of scaling law. Habitat Int. 99, 102172. doi:10.1016/j.habitatint.2020.102172
Jones, L., and Hameiri, S. (2021). Fractured China: how state transformation is shaping China’s rise. Cambridge: Cambridge University Press. doi:10.1017/9781009047487
Kalnay, E., and Cai, M. (2003). Impact of urbanization and land-use change on climate. Nature 423, 528–531. doi:10.1038/nature01675
Li, K., and Lin, B. (2017). Economic growth model, structural transformation, and green productivity in China. Appl. Energy 187, 489–500. doi:10.1016/j.apenergy.2016.11.075
Li, L. (2011). The incentive role of creating “cities” in China. China Econ. Rev. 22, 172–181. doi:10.1016/j.chieco.2010.12.003
Li, L., Pan, C., Ling, S., and Li, M. (2022). Ecological efficiency of urban industrial land in metropolitan areas: evidence from China. LAND 11, 104. doi:10.3390/land11010104
Liao, X., Fang, C., Shu, T., and Ren, Y. (2023). Spatiotemporal impacts of urban structure upon urban land-use efficiency: evidence from 280 cities in China. Habitat Int. 131, 102727. doi:10.1016/j.habitatint.2022.102727
Liu, X., Cifuentes-Faura, J., Gao, M., and Yang, C. (2024). Financing constraints of firms: is there political favoritism in the economic resources given by the central government?Manag. Decis. Econ. doi:10.1002/mde.4296
Lu, J., Li, B., Li, H., and Al-Barakani, A. (2021). Expansion of city scale, traffic modes, traffic congestion, and air pollution. Cities 108, 102974. doi:10.1016/j.cities.2020.102974
Ma, Y., Zheng, M., Zheng, X., Huang, Y., Xu, F., Wang, X., et al. (2023). Land use efficiency assessment under sustainable development goals: a systematic review. LAND 12, 894. doi:10.3390/land12040894
Naito, T. (2017). Growth and welfare effects of unilateral trade liberalization with heterogeneous firms and asymmetric countries. J. Int. Econ. 109, 167–173. doi:10.1016/j.jinteco.2017.08.011
Ning, J., Liu, J., Kuang, W., Xu, X., Zhang, S., Yan, C., et al. (2018). Spatiotemporal patterns and characteristics of land-use change in China during 2010–2015. J. Geogr. Sci. 28, 547–562. doi:10.1007/s11442-018-1490-0
Niu, S., Luo, X., Yang, T., Lin, G., and Li, C. (2023). Does the low-carbon city pilot policy improve the urban land green use efficiency? investigation based on multi-period difference-in-differences model. Int. J. Environ. Res. Public Health 20, 2704. doi:10.3390/ijerph20032704
Pauleit, S., and Duhme, F. (2000). Assessing the environmental performance of land cover types for urban planning. Landsc. Urban Plan. 52, 1–20. doi:10.1016/S0169-2046(00)00109-2
Pendall, R., Wegmann, J., Martin, J., and Wei, D. (2018). The growth of control? Changes in local land-use regulation in major U.S. Metropolitan areas from 1994 to 2003. Hous. Policy Debate 28, 901–919. doi:10.1080/10511482.2018.1494024
Platt, R. H. (1995). Land use and society: geography, law, and public policy. Washington, D.C: Island Press.
Post, A. E., and Kuipers, N. (2023). City size and public service access: evidence from Brazil and Indonesia. Perspect. Polit. 21, 811–830. doi:10.1017/S1537592722003176
Rosenthal, S. S., and Strange, W. C. (2020). How close is close? The spatial reach of agglomeration economies. J. Econ. Perspect. 34, 27–49. doi:10.1257/jep.34.3.27
Ruan, F., Yan, L., and Wang, D. (2020). The complexity for the resource-based cities in China on creating sustainable development. Cities 97, 102571. doi:10.1016/j.cities.2019.102571
Sharifi, A., Khavarian-Garmsir, A. R., Allam, Z., and Asadzadeh, A. (2023). Progress and prospects in planning: a bibliometric review of literature in urban studies and regional and urban planning, 1956–2022. Prog. Plan. 173, 100740. doi:10.1016/j.progress.2023.100740
Tan, S., Hu, B., Kuang, B., and Zhou, M. (2021). Regional differences and dynamic evolution of urban land green use efficiency within the Yangtze River Delta, China. Land Use Policy 106, 105449. doi:10.1016/j.landusepol.2021.105449
Tang, W., and Hewings, G. J. (2017). Do city–county mergers in China promote local economic development? Econ. Transition 25, 439–469. doi:10.1111/ecot.12118
Tang, Y., Wang, K., Ji, X., Xu, H., and Xiao, Y. (2021). Assessment and spatial-temporal evolution analysis of urban land use efficiency under green development orientation: case of the yangtze river delta urban agglomerations. Land 10, 715. doi:10.3390/land10070715
Tiebout, C. M. (1956). A pure theory of local expenditures. J. Political Econ. 64, 416–424. doi:10.1086/257839
Tone, K. (2004). “Dealing with undesirable outputs in DEA: A Slacks-Based Measure (SBM) approach,” in North American Productivity Workshop 2004 Toronto, Canada, 44–45.
Verburg, P. H., van Berkel, D. B., van Doorn, A. M., van Eupen, M., and van den Heiligenberg, H. A. R. M. (2010). Trajectories of land use change in Europe: a model-based exploration of rural futures. Landsc. Ecol. 25, 217–232. doi:10.1007/s10980-009-9347-7
Wang, M., and Debbage, N. (2021). Urban morphology and traffic congestion: longitudinal evidence from US cities. Comput. Environ. Urban Syst. 89, 101676. doi:10.1016/j.compenvurbsys.2021.101676
Wang, P., Shao, Z., Wang, J., and Wu, Q. (2021). The impact of land finance on urban land use efficiency: a panel threshold model for Chinese provinces. Growth Change 52, 310–331. doi:10.1111/grow.12464
Wellmann, T., Schug, F., Haase, D., Pflugmacher, D., and van der Linden, S. (2020). Green growth? On the relation between population density, land use and vegetation cover fractions in a city using a 30-years Landsat time series. Landsc. Urban Plan. 202, 103857. doi:10.1016/j.landurbplan.2020.103857
Xie, X., Fang, B., Xu, H., He, S., and Li, X. (2021). Study on the coordinated relationship between Urban Land use efficiency and ecosystem health in China. Land Use Policy 102, 105235. doi:10.1016/j.landusepol.2020.105235
Yang, Z., and Wu, A. M. (2015). The dynamics of the city-managing-county model in China: implications for rural–urban interaction. Environ. Urbanization 27, 327–342. doi:10.1177/0956247814566905
Yin, K., and Miao, Q. (2024). Urbanization and low-carbon cities: evidence from city-county merger in China. Econ. Analysis Policy 81, 724–737. doi:10.1016/j.eap.2023.11.019
Young, A. (2000). The razor’s edge: distortions and incremental reform in the people’s Republic of China. Q. J. Econ. 115, 1091–1135. doi:10.1162/003355300555024
Yu, H., Deng, Y., and Xu, S. (2018). Evolutionary pattern and effect of administrative division adjustment during urbanization of China: empirical analysis on multiple scales. Chin. Geogr. Sci. 28, 758–772. doi:10.1007/s11769-018-0990-2
Yu, Y., Li, K., Duan, S., and Song, C. (2023). Economic growth and environmental pollution in China: new evidence from government work reports. Energy Econ. 124, 106803. doi:10.1016/j.eneco.2023.106803
Zhang, L., Wang, S., Liu, Z., Sun, Y., and Zhao, X. (2023). Does urbanization intensify land finance? Evidence from the county-to-district policy in China. Appl. Econ. 55, 2189–2206. doi:10.1080/00036846.2022.2102133
Zhang, L., Yu, Y., and Chen, Y. (2022). The spatial-temporal evolution characteristics and driving factors of the green utilization efficiency of urban land in China. Front. Environ. Sci. 10, 955982. doi:10.3389/fenvs.2022.955982
Zhao, X., Lynch, J. G., and Chen, Q. (2010). Reconsidering baron and kenny: myths and truths about mediation analysis. J. Consumer Res. 37, 197–206. doi:10.1086/651257
Zhou, Y., Li, X., and Liu, Y. (2020). Land use change and driving factors in rural China during the period 1995-2015. Land Use Policy 99, 105048. doi:10.1016/j.landusepol.2020.105048
Keywords: administrative division adjustment, urban land green utilization efficiency, city-county merger, manage land, DID
Citation: Zhao M, Xiao X and Le Y (2024) The impact of city-county mergers on urban land green utilization efficiency: evidence from China. Front. Environ. Sci. 12:1418982. doi: 10.3389/fenvs.2024.1418982
Received: 10 May 2024; Accepted: 19 August 2024;
Published: 30 August 2024.
Edited by:
Merja H. Tölle, University of Kassel, GermanyReviewed by:
Miao He, Hebei University, ChinaYanni Cao, The University of Hong Kong, Hong Kong SAR, China
Zhi Li, Xi’an University of Architecture and Technology, China
Copyright © 2024 Zhao, Xiao and Le. This is an open-access article distributed under the terms of the Creative Commons Attribution License (CC BY). The use, distribution or reproduction in other forums is permitted, provided the original author(s) and the copyright owner(s) are credited and that the original publication in this journal is cited, in accordance with accepted academic practice. No use, distribution or reproduction is permitted which does not comply with these terms.
*Correspondence: Xiang Xiao, 0020120096@cufe.edu.cn