- 1College of Resources and Environmental Sciences, Henan Agricultural University, Zhengzhou, China
- 2College of Public Administration, Central China Normal University, Wuhan, Hubei, China
The study of agricultural non-point source pollution (ANNSP) patterns and driving mechanisms is crucial for regional ecological management. This paper evaluates the sources of ANNSP in the Huang-Huai-Hai Plain for the years 2000, 2010, and 2020 using the source list method. Additionally, nitrogen source data for the InVEST model were supplemented and adjusted, and the nitrogen output load and proportions derived in farmland from both methods were compared. The temporal and spatial distribution of nitrogen emission intensity was analyzed based on InVEST model outputs. Key source areas were identified through hotspot analysis, and spatial driving factors were examined using geodetector. Key findings reveal: 1) While the source list method estimated a higher nitrogen output load, both methods concurred on the proportion of agricultural nitrogen. The main sources of nitrogen input were overuse of fertilizer and livestock breeding. 2) Over this period, nitrogen emission intensity declined, exhibiting a distinct spatial pattern of “northwest low, southeast high.” 3) The extent of critical source areas underwent a dynamic shift, initially contracting before expanding. 4) Rainfall emerged as the primary driver of spatial variability in agricultural TN emission intensity. These insights offer critical reference points for achieving efficient ANNSP management in the Huang-Huai-Hai Plain.
1 Introduction
In the crucible of China’s economic metamorphosis, agriculture stands resolute as an enduring pillar, achieving commendable milestones and profoundly influencing global food security (Zhang et al., 2023). Despite these strides, the relentless march of urbanization has constricted arable lands, amplifying the demand for agricultural and livestock products. The pursuit of enhanced yields has strained the natural environment’s capacity, resulting in soil and water pollution and the emergence of agricultural non-point source pollution challenges (Lu et al., 2023). This pollution not only imperils water quality (Wang et al., 2023c), but also stands as a chief instigator of eutrophication. The “Second National Census of Pollution Sources Bulletin” in 2020 unveiled that agricultural sources alone contribute 46.52% of total nitrogen (TN) emissions, surpassing industrial and domestic counterparts. The genesis of agricultural non-point source pollution is intricately woven with climatic nuances, topographical peculiarities, land-use dynamics, and vegetative cover, rendering the monitoring, simulation, and mitigation of its impact a daunting task (Luo et al., 2023; Jolly et al., 2023; Wang et al., 2023a). The Huang-Huai-Hai Plain, as one of China’s nine major agricultural regions (Shirazi et al., 2022), shoulders the crucial task of supplying grains. However, over the past two decades, this region has faced severe threats from agricultural non-point source pollution, elevating the risk of water eutrophication (Chang and Zhang, 2023; Yue et al., 2022). Consequently, effectively and economically addressing agricultural non-point source pollution in the Huang-Huai-Hai Plain has emerged as a focal and formidable challenge in current research.
In the realm of addressing agricultural non-point source pollution, the accurate estimation of pollutant loads stands as a pivotal undertaking. Within this context, non-point source pollution models emerge as indispensable tools, categorically classified into two primary domains: empirical models and mechanistic models. Empirical models, exemplified by the output coefficient approach, leverage a broad spectrum of empirical data, but can fall short in portraying the intricate migration pathways of pollutants. Limitations stem from the inherent constraints imposed by regional boundaries, hindering a holistic understanding of the environmental phenomena (Li et al., 2021b). In contrast, mechanistic models engage in a more comprehensive examination, integrating diverse factors such as hydrology, meteorology, and geography to delve into the intricate spatial and temporal variations of non-point source pollution (Ji et al., 2023). These models not only facilitate the identification of pollutant sources and the analysis of their concentrations but also compute pollutant output loads. Consequently, they furnish robust support for the formulation of agricultural non-point source pollution mitigation strategies and the assessment of preventative measures (Zuo et al., 2023). Amid the plethora of non-point source pollution models, distributed models have gained widespread popularity due to their proficiency in simulating and predicting the migration and transformation of pollutants within river basins. This category encompasses models such as the Soil and Water Assessment Tool (SWAT) (Zhu et al., 2023; Ding et al., 2023b), the Annualized Agricultural Non-point Source (AnnAGNPS) (Chao et al., 2023; Liu et al., 2023), the Hydrological Simulation Program-Fortran (HSPF) (Lee et al., 2023), and the Integrative Valuation of Ecosystem Services and Tradeoffs Tool (InVEST) model (Ding et al., 2023a). In the practical application of these models, the judicious selection of a model must account for various factors, including the unique characteristics of the study area, simulation scale, and data availability.
The InVEST model distinguishes itself from other models through its straightforward parameterization and transparent mechanisms for nutrient flow and transformation within water systems. This simplicity and clarity make it particularly well-suited for large-scale simulations of non-point source pollution (Li et al., 2021a; Scordo et al., 2018). The Nutrient Delivery Ratio (NDR) module, integral to the InVEST model, provides a scientific basis for decision makers by modelling and estimating the contribution of vegetation and soil to water purification, particularly in controlling nutrient pollutants in runoff. Globally, researchers utilize NDR modules to explore non-point source pollution within watersheds. For instance, in the White River Basin of the Midwest United States, NDR modules have effectively delineated areas with elevated nitrogen and phosphorus loads across varying land use scenarios (Han et al., 2021). Similarly, studies in the United Kingdom have underscored the utility of NDR modules in evaluating the relative contributions of nitrogen and phosphorus outputs (Redhead et al., 2018). In China, Yan et al. (2018) evaluated the impact of constructed wetlands on total nitrogen removal in the Jiulong River basin, while NDR modules were used to estimate nitrogen and phosphorus pollutants in the mountainous terrain of Baoxing County, Sichuan Province (Liu et al., 2019). Zhang et al. (2021a) investigated non-point source distributions in the farmland of the Haihe River Basin, identifying critical pollution sources. These examples provided exemplify the robustness and dependability of the NDR module in studying non-point source pollution.
Variations in nutrient levels within a river basin can be evident across diverse regions. Within these areas, the preponderance of pollutant outputs from a single area may constitute the principal fraction of the overall pollution load within the entire basin, exerting a decisive influence on water quality. These localized domains, denominated as critical source areas (CSAs) (Ghebremichael et al., 2013; Hoang et al., 2019), assume paramount importance in the context of water quality management. Effectively identifying and managing these CSAs is imperative for the control of non-point source pollution. Although the task of non-point source pollution control is acknowledged to be formidable and resource-intensive (Chen et al., 2023a; Hou et al., 2022), achieving the objectives of water quality management can be done with greater efficacy and precision. Consequently, research endeavors revolving around CSAs have increasingly gained prominence. The strategic identification of CSAs through distributed models enables the prioritization of limited resources towards targeted remediation efforts, thereby markedly enhancing intervention efficiency (Giri et al., 2012). Such an approach not only holds promise for bolstering the efficacy of pollution control measures but also underscores a paradigm shift towards more discerning and resource-efficient environmental management strategies.
Currently, significant progress has been achieved in estimating non-point source pollution loads and identifying CSAs (Zuo et al., 2022). However, there remains a gap in understanding the mechanisms driving pollution emissions (Duan and Li, 2023). Using the InVEST model, this study conducted simulations of Agricultural nitrogen non-point source pollution (ANNSP) across the Huang-Huai-Hai Plain for the years 2000, 2010, and 2020. The aim of this study was to analyze the spatio-temporal patterns of total nitrogen (TN) emission intensity, pinpoint critical source areas, and quantify the influence of various factors. The primary objective of this research is to offer scientific support for decision-making regarding the control of Agricultural nitrogen non-point source pollution in the Huang-Huai-Hai Plain and advance the sustainable development of agriculture and contribute to rural ecological revitalization.
2 Materials and methods
2.1 Study area
The Huang-Huai-Hai Plain, located in the north of China, resides within a continental monsoon climate characterized by warm temperate sub-humid conditions, fostering unique opportunities for agricultural advancement. With ample rainfall and abundant light and heat resources, this region offers an optimal natural milieu for crop cultivation (Zhao et al., 2022). Boasting a deep and fertile soil layer, the Huang-Huai-Hai Plain primarily engages in dry farming practices. Notably, it serves as a pivotal hub for the cultivation of staple grain crops like wheat and corn, cementing its status as a significant contributor to China’s grain, cotton, and oil production (Liu et al., 2022).
This study specifically targets the Huang-Huai-Hai Plain, recognized among the nine principal agricultural regions designated by the Chinese Academy of Sciences. Encompassing Beijing, Tianjin, Shandong, Hebei, and Henan provinces, the area under investigation comprises 47 prefectures and cities. The study area encompasses a total expanse of 542, 30, 000 ha, experiencing an average rainfall of 715 mm over the span of 2000–2020 years. Within this domain, considerable regional disparities exist in both agricultural production and non-point source pollution.
2.2 Data sources
This study utilized the InVEST model to simulate the spatial and temporal dynamics of ANNSP across the Huang-Huai-Hai Plain. The model execution necessitated a range of data inputs, including Digital Elevation Model (DEM), land use and land cover (LULC), meteorological data, agricultural area data, administrative boundaries, and statistical data. The detailed specifics of the data employed in this investigation, alongside their respective sources, are comprehensively documented in Table 1.
2.3 Methods
2.3.1 InVEST model
The InVEST model leverages the robust capabilities of ArcGIS software and establishes a sophisticated framework for assessing ecosystem functions (Redhead et al., 2018). By integrating diverse natural data inputs, the model dynamically analyzes ecosystem service volumes, offering regional decision makers an intuitive, quantitative perspective on how ecosystem changes impact human wellbeing consequences. The InVEST model seeks to reconcile economic development with socio-ecological expectations, aiming to optimize sustainable resource allocation by promoting economic growth while safeguarding ecosystem health and resilience. Applied and validated in fields such as water conservation (Li et al., 2021a), habitat service quality assessment (Wu et al., 2021), and carbon storage estimation (Zhao et al., 2019), the model has garnered recognition for its accuracy and practical utility. Compared to similar models, InVEST stands out due to its straightforward parameters, clear mechanisms, and significant advantages in spatial information processing, database technology, mathematical calculations, and visual representation (Wei et al., 2022).
2.3.1.1 NDR module
The NDR module, integral to the InVEST model, is designed to simulate and assess nutrient transport within ecosystems. Built upon a hydrological framework, it replicates water volume, fluid dynamics, and flow paths, integrating crucial hydrological factors such as rainfall, evaporation, and runoff to precisely evaluate nutrient transport via runoff (Small et al., 2023). In this study, the NDR module adjusts total nitrogen (TN) input loads based on the watershed’s Runoff Potential Index (RPI) grid to compute the nitrogen transport rate at each pixel, as outlined in Equations 1–5. This analysis focuses on surface nutrient transport dynamics.
Where loadmod (x,i) is the nutrient load for each raster pixel i corrected. RPIi is the runoff potential index. RPi is the runoff agent on raster pixel i. RPav is the average proxy parameter on the grid. NDRsurf,i is the surface nitrogen transmission rate, NDRo,i is the nitrogen transmission rate retained by downstream pixels, ICj is the topographic index, ICo and k are calibration parameters, loadsurf,i is the nitrogen load of surface pixel i corrected, Xexp,i is the corrected nitrogen export load for each raster pixel i, Xexp,tot is the corrected total nitrogen export load of all grids.
This study focused on surface nitrogen nutrients in farmland and simplified the parameters in the biophysical table (Table 2). Set the cumulative flow threshold to 2000 m3/s, set the calibration parameter K to 2, and determine the RPI based on the annual rainfall.
2.3.2 Source list method
The source inventory method, widely recognized for its efficacy in identifying and quantifying pollution sources, particularly in estimating nitrogen contributions (Han et al., 2023a; Zhang et al., 2021a). In the context of China’s agricultural and rural activities, significant non-point sources include fertilizer application, straw return to fields, livestock breeding, and rural life. The InVEST model leverages detailed pollution load data from the source inventory method to conduct comprehensive analyses of various pollution sources’ impacts on ecosystem health. This process involves complex model operations addressing spatial dispersal, environmental transformation, and pollutant bioaccumulation. Moreover, Ding et al. (2023a) research underscores that statistical analysis of nitrogen output loads across farmland type further validates the InVEST model’s predictions, enhancing its scientific accuracy and reliability. Crucially, the source inventory method not only quantifies the specific contributions of each pollution source to nitrogen pollution (Fu et al., 2023; Wang et al., 2023a) but also accurately identifies principal sources, providing a data foundation for targeted pollution management strategies.
2.3.2.1 Fertilizer application
The quantification of nitrogen within applied fertilizers was determined by transforming the pure nitrogen content of both nitrogen fertilizers and nitrogen-containing compound fertilizers into statistical data. For nitrogen-containing compound fertilizers, a standardized assumption was made regarding the ratios of nitrogen to phosphorus to potassium, establishing a uniform ratio of 1:1:1, as detailed by Li et al. (2023a). The calculation formula is expressed as Equation 6:
Where Nfer is the input quantity of nitrogen-containing fertilizers, Nf is the amount of nitrogen fertilizer applied, Ncf is the amount of nitrogen-containing compound fertilizer applied.
2.3.2.2 Straw return to fields
Within this investigation, the TN content arising from the practice of returning to cropped fields was assessed across seven distinct crop types. The computation of the nitrogen surface source pollution load induced by straw returning to fields necessitates a comprehensive consideration of factors, including straw type, yield, crop-to-straw ratio, nitrogen content, the proportion of straw returned to fields, and product coefficient, as elucidated by Liu et al. (2021). The numerical values for each parameter (Table3) are extracted from pertinent literature sources (Yin et al., 2018; Zhang et al., 2021b; Wang et al., 2023b). The calculation formula is expressed as Equation 7:
Where Nstr is the TN input into farmland resulting from the practice of returning crop straw to fields, Yi is the yield of crop i, Ri is the ratio of crop i to straw, Ci is the nitrogen content in crop i, Fi is the proportion of crop i's straw returned to fields, Pi is the pollution production coefficient of crop i.
2.3.2.3 Livestock breeding
The ANNSP loss load stemming from livestock breeding is computed by utilizing metrics such as breeding volume alongside coefficients for manure and urine pollution generation. As reported in statistical yearbooks, the spectrum of livestock and poultry farming primarily encompasses large animals (cows, horses, donkeys, mules), pigs, sheep, and poultry. The coefficients for manure and urine excretion stand at 61.1, 4.51, 2.28, and 0.275 kg・head/piece−1・a−1, respectively, as documented by Chen et al. (2023b) and Qiu et al. (2021). The calculation formula is expressed as Equation 8:
Where Nlp is the TN input into agriculture stemming from animal husbandry, Qi is the quantity of livestock i at the end of the year, Ei is the coefficient of fecal excretion for livestock i.
2.3.2.4 Rural life
Within the purview of this inquiry, the TN load ascribed to rural settings emanates solely from domestic sewage, solid waste, and fecal waste generated by the rural populace (Zou et al., 2020). The calculation formula is expressed as Equation 9:
Where Nrl is the TN input into agriculture originating from rural life, Pi is the number of individuals in the rural community, Wi is the pollution coefficient attributed to each rural resident, calculated based on the standard of 1.58 kg per person per year.
2.3.2.5 Loss pattern
According to the manual Agricultural Pollution Source Fertilizer Loss Coefficient determine the pollution emission coefficient of different land loss patterns. The formula for calculating nitrogen is based on the pollution loss load of the source list method as shown in Equation 10:
Where N is the pollution TN discharge, Ej is the TN loss coefficient of non-point source pollution pattern j (Table 4), Qj is the basic loss, Ui is the pollution emission factor for different sources.
2.3.3 Hotspot analysis
Utilizing the Getis-Ord
Where
2.3.4 Geodetector
The Geodetector emerges as a potent instrument, endowed with the capacity for both factor detection and interactive detection, predicated on the resemblance between the contribution of driving factors and their spatial dispersion (Duan and Li, 2023). A meticulous examination involved statistical partitioning of TN emission intensity (Y). The subsequent phase involved the discretization of multiple independent variables, encompassing DEM (X1), slope (X2), annual rainfall (X3), proportion of farmland in each city (X4), TN input intensity (X5), Nfer (X6), Nstr (X7), Nlp (X8), and Nrl (X9). This study elucidates the impact of driving factors on spatial variations in nitrogen emission intensity, facilitating the identification of key individual factors through the q value measure developed by Li et al. (2022) and Shi et al. (2023). The calculation formula is expressed as Equation 14:
Where q represents the explanatory degree of X on Y, h is to the stratification of variable Y or factor X, Nh is the number of units in layer h, N represents the entire region,
3 Results and analysis
3.1 Comparison of InVEST model and source list method
The source list method offers a more comprehensive assessment of nitrogen pollution loads by recording and analyzing various nitrogen input sources. In contrast, the InVEST model may be constrained by its structure and parameter settings, limiting its ability to fully capture all significant nitrogen sources. Therefore, the source list method serves as a crucial supplement to the InVEST model, enhancing the accuracy of nitrogen input information. This study integrates the source list method with the InVEST model to provide a quantitative evaluation of nitrogen point source pollution. Focusing on farmland, a pivotal land use category, we calculated the agricultural surface nitrogen non-point source pollution load for each city and assessed its contribution to the overall load (Table 5), thereby illuminating the potential environmental consequences of agricultural activities. Notably, the source list method consistently yielded higher nitrogen output estimates than the InVEST model across the three temporal snapshots of 2000, 2010, and 2020. Nitrogen pollution is a dynamic process influenced by various factors, including climate change, land use changes, and agricultural management practices. The source list method is limited in its ability to detect small-scale variations in nitrogen pollution and cannot accurately simulate the loss and transformation of nitrogen fertilizers in agricultural settings. In contrast, the InVEST model incorporates a wider range of influential factors, offering a more comprehensive analysis of nitrogen dynamics.
Further scrutiny of farmland’s nitrogen output contribution to the total nitrogen non-point source pollution load reveals that the source tabulation method consistently yielded percentages of 67.24%, 64.93%, and 65.37% across the analyzed periods, underscoring the persistence of farmland as the primary source of nitrogen pollution load. The InVEST model estimates, albeit slightly varying at 64.32%, 62.7%, and 62.43%, agree on the pivotal role of farmland nitrogen management in regional pollution control. This congruence resonates with the recent findings of Ding et al. (2023a), reinforcing the InVEST model’s applicability and credibility in assessing agricultural nitrogen non-point source pollution. The similar nitrogen output ratios across farmland primarily stem from the consistent role of farmland as a major source of nitrogen pollution and the uniformity of agricultural management practices.
On the basis of detailed analysis of the input ratio of each nitrogen source (Table 6), this study clearly pointed out that fertilizer application and livestock breeding were the main pollution sources of agricultural TN load. Specifically, from 2000 to 2020, nitrogen fertilizer application and livestock farming accounted for a significant share of total TN output load, with the former contributing 54.66%–66.09%, and the latter contributing 24.56%–30.71%. This data distribution not only revealed the main drivers of agricultural nitrogen pollution, but also mapped the profound changes in the structure and mode of agricultural production. Looking at the dynamics over this time period, we notice a striking trend: with the acceleration of urbanization, the expansion of urban areas inevitably leads to the reduction of arable land, and while the absolute demand for agricultural output may fluctuate due to a variety of factors, agricultural activity itself shows a relatively concentrated growth trend. In this context, agricultural producers tend to increase the application amount of nitrogen fertilizer in order to maintain or increase production. Meanwhile, the large-scale development of animal husbandry has further aggravated the burden of nitrogen emissions.
3.2 Analysis of TN emission intensity
In this survey, the TN output load per hectare of farmland served as a quantitative indicator for assessing agricultural non-point source pollution (ANNSP) emission intensity across various cities, with classifications derived using the natural breaks method (Figure 2). Analysis of data from 2000, 2010, and 2020 reveals average TN output loads of 8.43 kg/ha, 4.08 kg/ha, and 3.66 kg/ha, respectively.
Temporally, a noteworthy decreasing trend in TN emission intensity was evident across all cities. Spatially, there existed significant heterogeneity in TN emission intensity, with an overarching pattern of “northwest low, southeast high,” aligning with the distribution of TN output load. This implies that farmland exerts minimal influence on the spatial distribution of TN emission intensity. It is pertinent to highlight that throughout all study years, Xinyang exhibited the highest TN emission intensity, registering values of 27.28 kg/ha, 9.49 kg/ha, and 10.87 kg/ha, respectively. In contrast, Zhangjiakou consistently demonstrated the lowest TN emission intensity, with values of 0.67 kg/ha, 0.41 kg/ha, and 0.26 kg/ha, respectively.
The decline in agricultural TN emission intensity can be primarily attributed to the formulation and execution of pertinent policies. Since 2015, China’s Ministry of Agriculture has spearheaded the implementation of the Action Plan to Achieve Zero Growth in Fertilizer Use by 2020. This strategic initiative aims at progressively curtailing fertilizer application through the adoption of scientific fertilization practices and enhanced fertilization techniques. Concurrently, heightened emphasis from local administrations on agricultural environmental preservation has propelled the widespread promotion of eco-friendly agricultural methodologies and approaches. This concerted effort has incentivized farmers to embrace alternatives such as organic fertilizers, biofertilizers, and other substitutes for chemical fertilizers, thereby curbing fertilizer usage and subsequently mitigating TN emissions. Moreover, governmental oversight of ANNSP has intensified, ushering in measures such as farmland drainage regulation and livestock breeding pollution management. These interventions further contribute to the reduction of TN emission intensity, thus fostering positive strides towards agricultural sustainability and ecological preservation.
3.3 Identification of critical source areas
To delineate the CSAs of ANNSP in the Huang-Huai-Hai Plain, this study employed the ArcGIS hotspot analysis tool. We conducted an extensive examination of data from 2000, 2010, and 2020, focusing on the agricultural TN emission intensity of individual cities. By configuring various levels of confidence, we derived the classification outcomes of CSAs (Figure 3).
In 2000, 2010, and 2020, the cumulative TN loads from CSAs represented 26%, 7%, and 28% of the entire study area, respectively. Temporally, the CSAs displayed a trend of initial contraction followed by expansion. Specifically, the number of cities situated within CSAs decreased from 5 to 2, before rising to 6. Regarding spatial distribution, CSAs progressively extended from the southern to the southeastern areas of the study domain. Initially, in 2000, the CSAs were dispersed across the southeastern region of Henan Province, encompassing Xuchang, Luohe, Zhoukou, Zhumadian, and Xinyang. By 2010, the CSAs were predominantly concentrated in the southern expanse of Henan Province, specifically in Luohe and Zhumadian. By 2020, these regions were primarily situated in the southeastern territories of Henan and Shandong provinces, including Xinyang, Linyi, Qingdao, Rizhao, Weifang, and Zibo.
The CSAs are significantly influenced by factors such as terrain and agricultural practices. These factors contribute to notable variations in soil moisture conditions and erosion resistance compared to other regions. Consequently, nitrogen nutrients are more susceptible to loss through runoff and leaching in these areas. Addressing this challenge necessitates a focus on improving ANNSP management practices and optimizing cropping structures within these CSAs. The integration of 3S (Geographic Information System (GIS), Global Positioning (GPS) and Remote Sensing (RS)) technologies and mechanistic models facilitates a more precise identification of CSAs for ANNSP. By simulating pollutant migration paths, transformation processes, and output continuity, these technologies aid in pinpointing the most polluted areas and locations within the study region. In CSAs such as Henan and southeastern Shandong, GIS and RS technologies were leveraged to monitor soil moisture and rainfall, thereby informing agricultural production practices. Initiatives included the advancement of water-saving irrigation technologies to mitigate water and nitrogen loss, as well as the promotion of crop varieties tailored to local climatic and soil conditions to bolster resilience against erosion.
3.4 Spatial evolutionary drivers analysis
In this investigation, by employing Geodetector, we scrutinized the discrimination and identification of factors influencing agricultural TN emission intensity across various cities within the Huang-Huai-Hai Plain, aiming to elucidate the primary drivers impacting nitrogen emissions in the region (Figure 4). Our findings revealed temporal variations in the main influencing factors. Specifically, in 2000, key drivers included annual rainfall (X3), TN input intensity (X5), and DEM (X1) (a). Conversely, by 2010, the primary factors shifted to encompass annual rainfall (X3), Nrl (X9), and the proportion of farmland in each city (X4) (b). This transition can be attributed to extensive rural-to-urban migration between 2000 and 2010, alongside rapid declines in farmland concurrent with ongoing urbanization. Come 2020, the principal drivers comprised annual rainfall (X3), DEM (X1), and slope (X2) (c). Notably, the enduring stability of annual rainfall (X3) as a driving factor across time scales warrants attention. Furthermore, significance level analyses underscored the pivotal roles of rainfall and terrain in shaping agricultural TN emission intensity. Nitrogen pollutants are predominantly influenced by rainfall-runoff and erosion processes, leading to their ingress into water bodies, concomitant with changes in terrain elevation, ultimately polluting receiving waters.
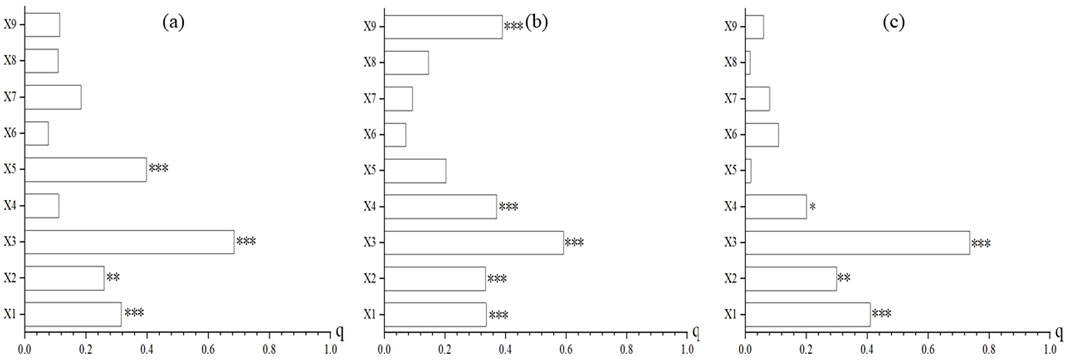
Figure 4. The contribution of driving factors. Note: (A–C) for the years 2000, 2010 and 2020, respectively. ***, ** and * are significant at 1%, 5% and 10% levels respectively.
Rainfall exerts a profound impact on pollution discharge, primarily influencing surface runoff and soil erosion, which can enhance soil nitrogen dissolution and facilitate nitrogen emissions. Additionally, rainfall interacts with topographic features, such as elevation and slope, exacerbating runoff and erosion in sloped areas, thereby intensifying nitrogen loss and acting as a crucial regulatory factor in agricultural nitrogen emissions. However, the spatio-temporal variability of rainfall impacts, constrained by regional and seasonal differences, complicates nitrogen pollution prevention and control. Consequently, effective ANNSP management strategies must account for the interplay between rainfall and terrain, implementing targeted measures to mitigate their adverse environmental effects.
4 Discussion
4.1 Comparison between source list method and InVEST model
This study employed the source list method to quantify primary nitrogen input sources in the Huang-Huai-Hai Plain and simulated the dynamic effects of agricultural nitrogen pollution on river nitrogen flux for the years 2000, 2010, and 2020 using an optimized NDR module. By comparing the total agricultural nitrogen inflow estimated through the source list method with outputs from the InVEST model, we rigorously assessed the consistency and complementary strengths of these approaches in evaluating the contribution of farmland nitrogen pollution. The theoretical framework provided by Ding et al. (2023a) underpins this analysis. The findings indicate that fertilizer overapplication and inadequate livestock management are major drivers of agricultural nitrogen pollution in the region, aligning with the prevailing consensus (Zhou et al., 2023) and underscoring the pivotal role of agricultural practices in the regional nitrogen cycle. Notably, the source listing method offers comprehensive nitrogen input data and helps identify key sources potentially overlooked by the InVEST model, thereby enhancing the accuracy of nitrogen pollution assessments. Meanwhile, the InVEST model excels in simulating nitrogen dynamics under complex environmental conditions, particularly when considering multiple factors such as climate change, land use change, and agricultural practices. Although the two methods differ in total agricultural nitrogen emissions, this discrepancy is attributed to their varying sensitivities to complex environmental factors, rather than any fundamental shortcomings in model suitability.
4.2 Effects of simulation and attribution analysis of ANNSP
The ANNSP emissions within the Huang-Huai-Hai Plain manifest distinct spatial disparities, presenting formidable challenges for pollution management. To more efficiently address these concerns, each region must comprehensively assess its agricultural production status and objectives. Tailored agricultural non-point source pollution control measures should be devised, taking into account the unique characteristics of pollution sources, economic development levels, and environmental conservation imperatives. For instance, implementing and enhancing a contemporary fertilization technology infrastructure, advocating for environmentally friendly, scientifically guided, and precise fertilization practices (Tian et al., 2023), pioneering innovative approaches for crop residue utilization, and augmenting utilization efficiencies. Furthermore, there is a pressing need to delve deeper into the origins, systems, and holistic treatment of ANNSP (Wang et al., 2023a).
In the face of increasingly severe ANNSP issues, strengthening research and control efforts is particularly urgent. Considering economic costs and implementation difficulties, focusing governance on CSAs is a wise choice (Tao et al., 2023). By prioritizing strengthening management measures and reasonably arranging the layout of governance projects, investment efficiency can be significantly improved, land resources can be saved, pollution control difficulties can be reduced, and better achievement of expected governance goals can be achieved, coordinating the relationship between agricultural production and environmental protection (Han et al., 2023b). In CSAs like Henan and southeastern Shandong, 3S technology was employed to steer agricultural production. Advocate improving the comprehensive utilization efficiency of straw and water-saving irrigation technology to reduce the loss of water and nitrogen (Yin et al., 2018).
4.3 The improvement direction of research
In forthcoming applications, the integration of the InVEST model with the PLUS model (Zhu et al., 2024) and prospective climate scenario (Li et al., 2023b) data holds promise for advancing non-point source pollution prediction and analysis. Additionally, to achieve more effective ANNSP control, factors other than CSAs identification and management need to be considered. For example, the promotion and application of agricultural technology, the formulation and implementation of policies and regulations, farmers’ environmental awareness and participation. These factors have important impacts on ANNSP control and management and need to be considered in future studies.
5 Conclusion
Based on the results of InVEST model, this study delved into the pivotal agricultural nitrogen non-point source pollution hotspots in the Huang-Huai-hai Plain and their tailored mitigation strategies. Key findings reveal:
(1) Although the source listi method yielded a higher load estimate than the InVEST model simulation, both concurred on the consistent proportion of output nitrogen from farmland. Over-fertilization and livestock breeding emerged as the primary contributors to TN loads within the agricultural landscape of the Huang-Huai-Hai Plain.
(2) Time-series analysis unveiled a pronounced decrease in nitrogen emission intensity, accompanied by a spatial pattern characterized by a gradual increase from northwest to southeast.
(3) Throughout the study period, the CSAs experienced a dynamic shift, initially narrowing and subsequently expanding. Notably, Xinyang City remained consistently situated within the CSAs.
(4) Factor detection analysis highlighted annual rainfall (X3) as possessing the highest q-value in 2000, 2010, and 2020, underscoring rainfall as the primary driving factor shaping spatial variations in agricultural TN emission intensities across cities in the Huang-Huai-Hai Plain.
Data availability statement
The original contributions presented in the study are included in the article/supplementary material, further inquiries can be directed to the corresponding author.
Author contributions
YY: Conceptualization, Methodology, Writing–original draft. WC: Conceptualization, Funding acquisition, Writing–review and editing. GJ: Data curation, Writing–review and editing. YG: Resources, Writing–review and editing. YC: Methodology, Writing–review and editing. SS: Writing–review and editing. YD: Formal Analysis, Writing–review and editing. QW: Supervision, Writing–review and editing.
Funding
The author(s) declare that financial support was received for the research, authorship, and/or publication of this article. This research was funded by the National Key Research and Development Project (2021YFD1700900).
Conflict of interest
The authors declare that the research was conducted in the absence of any commercial or financial relationships that could be construed as a potential conflict of interest.
Publisher’s note
All claims expressed in this article are solely those of the authors and do not necessarily represent those of their affiliated organizations, or those of the publisher, the editors and the reviewers. Any product that may be evaluated in this article, or claim that may be made by its manufacturer, is not guaranteed or endorsed by the publisher.
References
Chang, D., and Zhang, Y. X. (2023). Farmland nutrient pollution and its evolutionary relationship with plantation economic development in China. J. Environ. Manag. 325, 116589. doi:10.1016/j.jenvman.2022.116589
Chao, X. B., Witthaus, L., Bingner, R., Jia, Y., Locke, M., and Lizotte, R. (2023). An integrated watershed and water quality modeling system to study lake water quality responses to agricultural management practices. Environ. Model. Softw. 164, 105691. doi:10.1016/j.envsoft.2023.105691
Chen, M. J., Janssen, A. B. G., de Klein, J. J. M., Du, X., Lei, Q., Li, Y., et al. (2023a). Comparing critical source areas for the sediment and nutrients of calibrated and uncalibrated models in a plateau watershed in southwest China. J. Environ. Manag. 326, 116712. doi:10.1016/j.jenvman.2022.116712
Chen, T., Lu, J. Y., Lu, T., Yang, X., Zhong, Z., Feng, H., et al. (2023b). Agricultural non-point source pollution and rural transformation in a plain river network: insights from Jiaxing city, China. Environ. Pollut. 333, 121953. doi:10.1016/j.envpol.2023.121953
Ding, L., Qi, C. C., and Zhang, W. Q. (2023a). Distribution characteristics of non-point source pollution of TP and identification of key source areas in Nanyi Lake (China) Basin: based on InVEST model and source list method. Environ. Sci. Pollut. Res. Int 30, 117464–117484. doi:10.1007/s11356-023-30405-y
Ding, W. L., Xia, J., She, D. X., Zhang, X., Chen, T., Huang, S., et al. (2023b). Assessing multivariate effect of best management practices on non-point source pollution management using the coupled Copula-SWAT model. Ecol. Indic. 153, 110393. doi:10.1016/j.ecolind.2023.110393
Duan, T. T., and Li, Y. X. (2023). A multiscale analysis of the spatially heterogeneous relationships between non-point source pollution -related processes and their main drivers in Chaohu Lake watershed, China. Environ. Sci. Pollut. Res. Int 30, 86940–86956. doi:10.1007/s11356-023-28233-1
Fu, D., Gong, Y., Chen, C. T., Gui, X., Liu, H., Chen, S., et al. (2023). Nitrogen and phosphorus loading characteristics of agricultural non-point sources in the tuojiang River Basin. Water 15, 3503. doi:10.3390/w15193503
Ghebremichael, L. T., Veith, T. L., and Hamlett, J. M. (2013). Integrated watershed- and farm-scale modeling framework for targeting critical source areas while maintaining farm economic viability. J. Environ. Manag. 114, 381–394. doi:10.1016/j.jenvman.2012.10.034
Giri, S., Nejadhashemi, A. P., and Woznicki, S. A. (2012). Evaluation of targeting methods for implementation of best management practices in the Saginaw River Watershed. J. Environ. Manag. 103, 24–40. doi:10.1016/j.jenvman.2012.02.033
Han, A. X., Liu, P. Y., Wang, B. F., and Zhu, A. (2023a). E-commerce development and its contribution to agricultural non-point source pollution control: evidence from 283 cities in China. J. Environ. Manag. 344, 118613. doi:10.1016/j.jenvman.2023.118613
Han, B. S., Reidy, A., and Li, A. H. (2021). Modeling nutrient release with compiled data in a typical Midwest watershed. Ecol. Indic. 121, 107213. doi:10.1016/j.ecolind.2020.107213
Han, Y., Liu, Z., Chen, Y. F., Liu, H., Song, L., et al. (2023b). Assessing non-point source pollution in an apple-dominant basin and associated best fertilizer management based on SWAT modeling. Int. Soil Water Conservation Res. 11, 353–364. doi:10.1016/j.iswcr.2022.10.002
Hoang, L., Mukundan, R., Moore, K. E. B., Owens, E. M., and Steenhuis, T. S. (2019). Phosphorus reduction in the New York City water supply system: a water-quality success story confirmed with data and modeling. Ecol. Eng. 135, 75–88. doi:10.1016/j.ecoleng.2019.04.029
Hou, L., Zhou, Z. Y., Wang, R. Y., Li, J., Dong, F., and Liu, J. (2022). Research on the non-point source pollution characteristics of important drinking water sources. Water 14, 211. doi:10.3390/w14020211
Ji, Y., Gao, D. M., Liu, Q. L., Su, J., Liu, Y., Zhao, J., et al. (2023). An integrated framework for agricultural non-point source pollution control technology evaluation: application of an improved multiple attribute decision making method. Expert Syst. Appl. 229, 120319. doi:10.1016/j.eswa.2023.120319
Jolly, Y. N., Rakib, M. R. J., Kumar, R., Sultana, S., Rahman, S. M. M., Kabir, J., et al. (2023). Evaluation of surface water quality near pollution sources in Buriganga River and deciphering their probable emergence, ecological, and health risk aspects. Regional Stud. Mar. Sci. 63, 102988. doi:10.1016/j.rsma.2023.102988
Lee, H., Kim, H., Kim, J., Jun, S. M., Hwang, S., Song, J. H., et al. (2023). Analysis of the effects of low impact development practices on hydrological components using HSPF. J. Hydro-Environment Res. 46, 72–85. doi:10.1016/j.jher.2023.01.001
Li, B. Z., Guo, B., Zhu, Q. B., and Zhuo, N. (2023a). Impact of technical training and personalized information support on farmers' fertilization behavior: evidence from China. Sustainability 15, 8925. doi:10.3390/su15118925
Li, M. Y., Liang, D., Xia, J., Song, J., Cheng, D., Wu, J., et al. (2021a). Evaluation of water conservation function of danjiang River Basin in qinling mountains, China based on InVEST model. J. Environ. Manag. 286, 112212. doi:10.1016/j.jenvman.2021.112212
Li, Q., Yang, Z. H., Yue, Y., and Zhong, H. (2021b). Variation of total phosphorus concentration and loads in the upper Yangtze River and contribution of non-point sources. Water Supply 21, 1687–1700. doi:10.2166/ws.2021.015
Li, Q. S., Zhang, J. C., Zhang, J. W., Gao, H., Chen, W., Huang, J., et al. (2023b). Spatial and temporal distribution characteristics and prediction analysis of nitrogen and phosphorus surface source pollution in Shandong Province under the climate and land use changes. Front. Ecol. Evol. 11. doi:10.3389/fevo.2023.1231394
Li, X. C., Niu, Y., He, Q. N., and Wang, H. (2022). Identifying driving factors of the runoff coefficient based on the geographic detector model in the upper reaches of Huaihe River Basin. Open Geosci. 14, 1421–1433. doi:10.1515/geo-2022-0438
Liu, B. Y., Lin, B. J., Li, X. X., Virk, A. L., N'dri Yves, B., Zhao, X., et al. (2021). Appropriate farming practices of summer maize in the North China Plain: reducing nitrogen use to promote sustainable agricultural development. Resour. Conservation Recycl. 175, 105889. doi:10.1016/j.resconrec.2021.105889
Liu, C., Qu, L. Q., Clausen, J., Lei, T., and Yang, X. (2023). Impact of riparian buffer zone design on surface water quality at the watershed scale, a case study in the jinghe watershed in China. Water 15, 2696. doi:10.3390/w15152696
Liu, J., Fu, B., Zhang, C. H., and Wang, Y. k. (2019). Modelling spatial variation in the treatment costs of non-point source pollution in mountainous regions of southwest China. J. Mt. Sci. 16 (8), 1901–1912. doi:10.1007/s11629-018-5051-x
Liu, J., Sun, B., Shen, H. L., Ding, P., Ning, D., Zhang, J., et al. (2022). Crop water requirement and utilization efficiency-based planting structure optimization in the southern Huang-Huai-Hai Plain. Agronomy-Basel 12, 2219. doi:10.3390/agronomy12092219
Lu, Y. R., Wang, C., Yang, R. J., Sun, M., Zhang, L., Zhang, Y., et al. (2023). Research on the progress of agricultural non-point source pollution management in China: a review. Sustainability 15, 13308. doi:10.3390/su151813308
Luo, M., Liu, X. X., Legesse, N., Liu, Y., Wu, S., Han, F. X., et al. (2023). Evaluation of agricultural non-point source pollution: a review. Water Air Soil Pollut. 234, 657. doi:10.1007/s11270-023-06686-x
Qiu, W. W., Zhong, Z. B., and Li, Z. L. (2021). Agricultural non-point source pollution in China: evaluation, convergence characteristics and spatial effects. Chin. Geogr. Sci. 31, 571–584. doi:10.1007/s11769-021-1200-1
Redhead, J. W., May, L., Oliver, T. H., Hamel, P., Sharp, R., and Bullock, J. M. (2018). National scale evaluation of the InVEST nutrient retention model in the United Kingdom. Sci. Total Environ. 610, 666–677. doi:10.1016/j.scitotenv.2017.08.092
Scordo, F., Lavender, T. M., Seitz, C., Perillo, V. L., Rusak, J. A., Piccolo, M. C., et al. (2018). Modeling water yield: assessing the role of site and region-specific attributes in determining model performance of the InVEST seasonal water yield model. Water 10, 1496. doi:10.3390/w10111496
Shi, S. H., Wang, X. L., Hu, Z. R., Zhao, X., Zhang, S., Hou, M., et al. (2023). Geographic detector -based quantitative assessment enhances attribution analysis of climate and topography factors to vegetation variation for spatial heterogeneity and coupling. Glob. Ecol. Conservation 42, e02398. doi:10.1016/j.gecco.2023.e02398
Shirazi, S. Z., Mei, X. R., Liu, B. C., and Liu, Y. (2022). Estimating potential yield and change in water budget for wheat and maize across Huang-Huai-Hai Plain in the future. Agric. Water Manag. 260, 107282. doi:10.1016/j.agwat.2021.107282
Small, G. E., Martensson, N., Janke, B. D., and Metson, G. S. (2023). Potential for high contribution of urban gardens to nutrient export in urban watersheds. Landsc. Urban Plan. 229, 104602. doi:10.1016/j.landurbplan.2022.104602
Tao, W., Wei, J., and Wang, N. L. (2023). The characteristics of non-point source nitrogen pollution in the lower reaches of huangshui basin in western China based on SWAT model. Water Air Soil Pollut. 234, 491. doi:10.1007/s11270-023-06480-9
Tian, M. L., Liu, R. F., Wang, J., Liang, J., Nian, Y., and Ma, H. (2023). Impact of environmental values and information awareness on the adoption of soil testing and formula fertilization technology by farmers-A case study considering social networks. Agricilture-Basel 13, 2008. doi:10.3390/agriculture13102008
Tran, D. X., Pearson, D., Palmer, A., Gray, D., Lowry, J., and Dominati, E. J. (2022). A comprehensive spatially-explicit analysis of agricultural landscape multifunctionality using a New Zealand hill country farm case study. Agric. Syst. 203, 103494. doi:10.1016/j.agsy.2022.103494
Wang, J. L., Fu, Z. S., Qiao, H. X., Bi, Y., and Liu, F. (2023a). Identifying the spatial risk patterns of agricultural non-point source pollution in a Basin of the upper yangtze river. Agronomy-Basel 13, 2776. doi:10.3390/agronomy13112776
Wang, L., Wang, E. L., Qian, X., Gao, Y., Zhang, H., Li, Z., et al. (2023b). Optimal straw management co-benefits crop yield and soil carbon sequestration of intensive farming systems. Land Degrad. and Dev. 34, 2322–2333. doi:10.1002/ldr.4610
Wang, M. M., Jiang, T. H., Mao, Y. B., Wang, F., Yu, J., and Zhu, C. (2023c). Current situation of agricultural non-point source pollution and its control. Water Air Soil Pollut. 234, 471. doi:10.1007/s11270-023-06462-x
Wei, Q. Q., Abudureheman, M., Halike, A., Yao, K., Yao, L., Tang, H., et al. (2022). Temporal and spatial variation analysis of habitat quality on the PLUS-InVEST model for Ebinur Lake Basin, China. Ecol. Indic. 145, 109632. doi:10.1016/j.ecolind.2022.109632
Wu, L. L., Sun, C. G., Fan, F., et al. (2021). Estimating the characteristic spatiotemporal variation in habitat quality using the InVEST model-a case study from Guangdong-Hong Kong-Macao Greater Bay area. Remote Sens. 13, 1008. doi:10.3390/rs13051008
Yan, Y. Y., Guan, Q. S., Wang, M., Su, X., Wu, G., Chiang, P., et al. (2018). Assessment of nitrogen reduction by constructed wetland based on InVEST: a case study of the Jiulong River Watershed, China. Mar. Pollut. Bull. 133, 349–356. doi:10.1016/j.marpolbul.2018.05.050
Yin, H. J., Zhao, W. Q., Li, T., Cheng, X., and Liu, Q. (2018). Balancing straw returning and chemical fertilizers in China: role of straw nutrient resources. Renewable& Sustain. Energy Rev. 81, 2695–2702. doi:10.1016/j.rser.2017.06.076
Yue, Y. J., Yang, W. Q., and Wang, L. (2022). Assessment of drought risk for winter wheat on the Huanghuaihai Plain under climate change using an EPIC model-based approach. Int. J. Digital Earth 15, 690–711. doi:10.1080/17538947.2022.2055174
Zhang, Q. L., Hu, H. T., Wang, D. Y., et al. (2021a). Spatial distribution of nitrogen and phosphorus in Haihe Basin and their determinants. J. Irrigation Drainage 40 (4), 97–106. doi:10.13522/j.cnki.ggps.2020411
Zhang, S. J., Zhang, G., Wang, D. J., and Liu, Q. (2021b). Long-term straw return with N addition alters reactive nitrogen runoff loss and the bacterial community during rice growth stages. J. Environ. Manag. 292, 112772. doi:10.1016/j.jenvman.2021.112772
Zhang, X., Sun, S. H., and Yao, S. B. (2023). Spatiotemporal distribution and dynamic evolution of grain productivity efficiency in the Yellow River Basin of China. Environ. Dev. Sustain. 26, 12005–12030. doi:10.1007/s10668-023-03619-w
Zhao, M. M., He, Z. B., Du, J., Chen, L., Lin, P., and Fang, S. (2019). Assessing the effects of ecological engineering on carbon storage by linking the CA-Markov and InVEST models. Ecol. Indic. 98, 29–38. doi:10.1016/j.ecolind.2018.10.052
Zhao, Y. X., Xiao, D. P., Bai, H. Z., Liu, D. L., Tang, J., Qi, Y., et al. (2022). Climate change impact on yield and water use of rice-wheat rotation system in the Huang-Huai-Hai Plain, China. Biology-Basel 11, 1265. doi:10.3390/biology11091265
Zhou, J. H., Liu, X. H., Liu, X. J., Wang, W., and Wang, L. (2023). Assessing agricultural non-point source pollution loads in typical basins of upper Yellow River by incorporating critical impacting factors. Process Saf. Environ. Prot. 177, 17–28. doi:10.1016/j.psep.2023.07.003
Zhu, K. W., Zhang, Y., Tian, X. S., Guan, D., Zhang, S., He, Y., et al. (2024). Evolutionary trend analysis of agricultural non-point source pollution load in chongqing based on land use simulation. Agronomy 14, 737. doi:10.3390/agronomy14040737
Zhu, Z. C., Fu, C. S., Wu, H. W., Zhang, H., Cao, Y., et al. (2023). What influences does grazing bring about to stream nutrient fluxes in alpine meadows? Agric. Water Manag. 289, 108502. doi:10.1016/j.agwat.2023.108502
Zou, L. L., Liu, Y. S., Wang, Y. S., and Hu, X. (2020). Assessment and analysis of agricultural non-point source pollution loads in China: 1978-2017. J. Environ. Manag. 263, 110400. doi:10.1016/j.jenvman.2020.110400
Zuo, D. P., Bi, Y. Q., Song, Y. H., Xu, Z., Wang, G., Ma, G., et al. (2023). The response of non-point source pollution to land use change and risk assessment based on model simulation and grey water footprint theory in an agricultural river basin of Yangtze River, China. Ecol. Indic. 154, 110581. doi:10.1016/j.ecolind.2023.110581
Keywords: agricultural nitrogen non-point source pollution, InVEST model, hotspot analysis, geodetector, Huang-Huai-Hai Plain
Citation: Yu Y, Chen W, Ji G, Guo Y, Chen Y, Sheng S, Deng Y and Weng Q (2024) Simulation and attribution analysis of agricultural nitrogen non-point source pollution in Huang-Huai-Hai Plain based on the InVEST model. Front. Environ. Sci. 12:1415215. doi: 10.3389/fenvs.2024.1415215
Received: 12 April 2024; Accepted: 07 November 2024;
Published: 20 November 2024.
Edited by:
Keith Schilling, The University of Iowa, United StatesReviewed by:
Wei Shui, Fuzhou University, ChinaNing Niu, Henan University of Economic and Law, China
Copyright © 2024 Yu, Chen, Ji, Guo, Chen, Sheng, Deng and Weng. This is an open-access article distributed under the terms of the Creative Commons Attribution License (CC BY). The use, distribution or reproduction in other forums is permitted, provided the original author(s) and the copyright owner(s) are credited and that the original publication in this journal is cited, in accordance with accepted academic practice. No use, distribution or reproduction is permitted which does not comply with these terms.
*Correspondence: Weiqiang Chen, Y2h3cWdpc0BoZW5hdS5lZHUuY24=