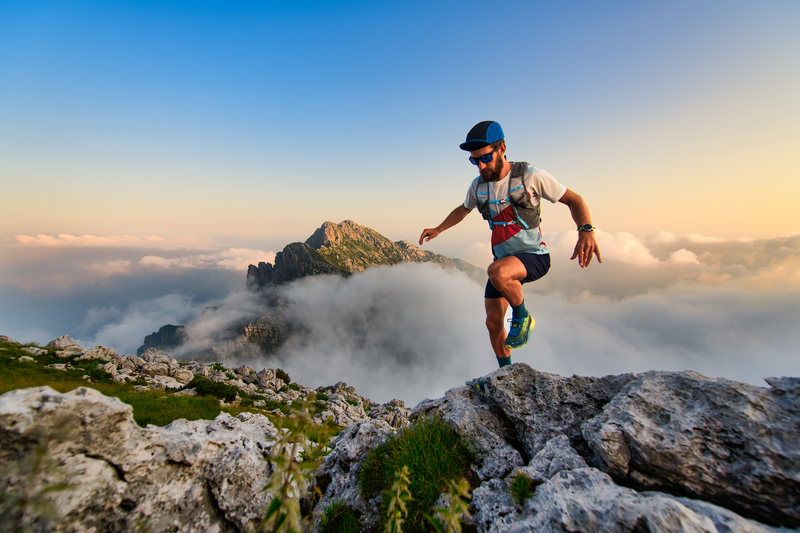
95% of researchers rate our articles as excellent or good
Learn more about the work of our research integrity team to safeguard the quality of each article we publish.
Find out more
ORIGINAL RESEARCH article
Front. Environ. Sci. , 26 July 2024
Sec. Environmental Economics and Management
Volume 12 - 2024 | https://doi.org/10.3389/fenvs.2024.1414365
This article is part of the Research Topic Green Finance & Carbon Neutrality: Strategies and Policies for a Sustainable Future View all 36 articles
Amidst global sustainability challenges, green finance emerges as a crucial instrument for advancing sustainable development, garnering increasing attention for its pivotal role in fostering high-quality economic development (HQED), particularly within the dynamic economic landscape of China. This study delves into the nexus between green finance and HQED across 30 Chinese provinces from 2012 to 2021. Employing the entropy method, indices for green finance and HQED index system are calculated, and their interaction is analyzed through a panel data model, incorporating tests for moderating effects of FinTech and green technological innovation, as well as assessing the heterogeneity across diverse regions. The findings highlight green finance’s significant role in enhancing HQED, with notable regional disparities. Specifically, the eastern region shows the strongest impact, followed by the central region, while the western and northeastern regions exhibit weaker influences. The study also identifies FinTech and green technological innovation as pivotal moderators, amplifying green finance’s positive effect on HQED. These insights underscore green finance’s importance in driving sustainable economic growth and highlight the necessity for region-specific strategies to optimize its impact. Policy recommendations based on these findings include prioritizing the development of green finance, formulating region-specific strategies, and leveraging the catalytic roles of FinTech and green technological innovation to enhance the efficacy of green finance in achieving HQED.
In recent decades, the pursuit of economic growth worldwide has often come at a significant environmental cost, including rampant natural resource consumption, energy overuse, and escalating carbon emissions. These practices have led to environmental degradation, climate change, and increased economic vulnerability, posing formidable challenges to sustainable development (Tol, 2009; Hafeez et al., 2019; Islam et al., 2022). Recognizing these challenges, the United Nations introduced the “2030 Agenda for Sustainable Development”, setting a global direction towards reconciling economic growth with environmental conservation. Amidst this global context, as one of the largest developing countries in the world, China’s journey of remarkable economic expansion has unfolded, marked by a GDP increase from 367.87 billion yuan in 1978 to 121,020.72 billion yuan in20221. However, this growth has been accompanied by substantial environmental and social costs, including excessive resource consumption, severe pollution, and widening inequalities (Gao et al., 2022; Wang et al., 2023).
In addressing these challenges, China’s economic development strategy is undergoing a fundamental shift. The previous model, focused solely on GDP growth, is gradually transitioning to a balanced and sustainable approach, emphasizing the quality of economic development (Yang, 2023). This strategic pivot underscores the essential link between China’s development path and broader sustainability challenges, highlighting the country’s commitment to playing a responsible role in global environmental governance.
To achieve a balanced and sustainable model of economic development, the Chinese government has prioritized high-quality economic development (HQED) as the paramount endeavor in building a comprehensively modernized nation. This strategy emphasizes a novel development concept, asserting that success should not be measured solely by GDP growth rates. High-quality development must be driven by innovation as the primary force, characterized by coordination as an intrinsic feature, manifested through a green and sustainable approach, pursued through openness as an essential pathway, and aimed at achieving shared prosperity as the fundamental goal.
Against this backdrop, green finance, as a crucial component supporting green development, integrates environmental protection into financial activities, utilizing financial tools and policies to facilitate the transformation and enhancement of businesses (Khan et al., 2022a). Green finance occupies a central role in transitioning economic development towards pathways that are green, low-carbon, and circular (Fu et al., 2023; Kumar et al., 2023), positioning as an indispensable driver of HQED.
However, green finance may also pose numerous challenges to socioeconomic development. Firstly, the redirection of funds from traditional industries to more environmentally friendly projects through green finance increases the financial burden on polluting industries. This necessitates greater expenditure by these industries to adopt cleaner technologies or even exit the market (Xiong et al., 2023). Secondly, the advancement of green finance within China is currently hindered by issues such as a monolithic structure, incomplete information disclosure, low returns, long investment horizons, and significant risks. These factors render current green finance initiatives less conducive to long-term sustainable development (Chen et al., 2023). Thirdly, the transition of developing economies to green finance faces high financial challenges, implying that green finance also introduces transitional risks (OECD, 2016). Therefore, researching the factors and relationships impacting green finance and HQED, and promoting their coordinated advancement, is a topic of widespread interest and urgent inquiry among academics and governments at all levels. This research direction holds significant theoretical and practical implications for guiding policy formulation in China, optimizing economic structures, and advancing local sustainable development.
Furthermore, Financial technology (FinTech), emerging from the profound integration of modern information technology and traditional financial services, started relatively late in China but has undergone rapid development (Wu, 2018), serving as a pivotal catalyst for economic growth. FinTech effectively tackles challenges in customer acquisition and risk management by leveraging advanced technologies such as blockchain, big data, cloud computing, and artificial intelligence. This advancement significantly expands the scale and efficiency of financial supply, thereby laying a solid foundation for HQED. Simultaneously, green technological innovation focuses on mitigating pollution, enhancing the efficiency of technological progress, and promoting the concurrent advancement of ecological environmental protection and socioeconomic development (Lv et al., 2021). It reduces high consumption and pollution through green technologies, alleviating resource pressure, improving the ecological environment, and enhancing corporate competitiveness. This, in turn, increases the efficiency of economic operations and elevates HQED.
Academics have undertaken extensive investigations into the factors influencing HQED from various perspectives, including green finance, FinTech and green technological innovation, which provides a solid reference base for this research. Nonetheless, there remains a noticeable void in the literature regarding a comprehensive framework that integrates these elements. To bridge this gap, this paper employs panel data from 30 Chinese provinces covering the period from 2012 to 2021. Under the new development concept, this study constructs a HQED index system incorporating five dimensions: innovation, coordination, green, openness, and sharing. Additionally, it establishes a green finance index system comprising green credit, green securities, green investment, green insurance, and carbon finance. By utilizing panel data models, the study empirically analyzes the impact relationship between green finance and HQED across Chinese regions from the perspectives of FinTech and green technological innovation and conducts an analysis of regional heterogeneity. The aim is to provide region-specific strategies for local government and market practices, contribute to the realization of a sustainable development model for China, and thus promote economic development towards a more green, efficient, and balanced direction.
The remainder of this study is organized in the following manner: the second section provides a review of the extant literature; the third section articulates the theoretical framework and posits research hypotheses; the fourth part discusses the model construction and variable measurement. The fifth section presents an analysis of the empirical findings, including tests of mechanisms, robustness checks, and examinations of heterogeneity. The concluding section outlines the study’s findings, offers policy implications, acknowledges the research’s limitations, and suggests avenues for future inquiry.
Green finance, as a novel financial paradigm, sets itself apart from traditional finance by leveraging diverse financial instruments and products to address environmental pollution risks, aiming for green development (Khan et al., 2022b). Unlike conventional finance, green finance is committed to promoting environmental betterment and achieving economic sustainability (Yin and Xu, 2022). Early research in the field of green finance predominantly focused on micro-level analyses, such as investigating the roles that financial institutions play in environmental preservation and the promotion of sustainable economic growth (White, 1996; Jeucken and Bouma, 2017). Recently, the academic community has shifted its focus towards the interplay between green finance and corporate performance, with many scholars advocating a positive correlation between the two. Xu et al. (2020), using a meta-analytic approach, discovered a substantial positive link between green finance and corporate green performance. Lu et al. (2022), based on publicly listed companies in the A-share market and utilizing the Difference-in-Differences (DID) method, concluded that green finance significantly bolsters firms’ capacity for green technological innovation by reallocating internal and external financing constraints. Du et al. (2022), also utilizing the DID approach, examined the motivational effects of the Green Credit Guidelines (GCG) on the technological innovation and financial performance of China’s listed green companies, finding that green finance actively stimulates technological innovation and financial performance in these enterprises. Wang et al. (2022) using data from listed companies in China, assessed the influence of green finance on corporate ESG performance, observing an overall enhancement in corporate ESG due to green finance policies, with the effects varying across different governance structures. Yu et al. (2023) have developed a regional green finance development index in order to evaluate the influence of green finance on the financial performance of green enterprises within the context of China. Their findings indicate that green finance effectively enhances the financial performance of these enterprises, primarily through the mechanisms of capital concentration and information dissemination. Sun et al. (2023) applied the DID model to examine the effects of green finance policies on firms’ ESG performance, showing a positive effect on ESG outcomes. Berikhanovna et al. (2023) utilized a panel regression model to investigate the Green Credit Policy (GCP)’s effect on firms’ green innovation, transformation, and upgrading. Their findings indicate a positive and statistically significant effect, particularly within the context of large firms.
The concept of high-quality development is characterized by efficient, equitable, and green sustainable growth, aimed at fulfilling the increasingly sophisticated aspirations of the populace for a better life. It involves the harmonious progress of five key areas: economic development, political stability, cultural enrichment, social progress, and ecological civilization (Zhang et al., 2019). In the study of HQED, the academic community commonly employs Green Total Factor Productivity (GTFP) as a metric to assess the quality of economic development. Most studies use Data Envelopment Analysis (DEA) to calculate GTFP, explaining the level of HQED, as DEA circumvents the need for a specified production function and accommodates multiple inputs and outputs, making the measurement of HQED more convenient and comprehensive (Yu et al., 2019). Mei and Chen (2016) integrated DEA with the Directional Distance Function, utilizing the BML index to gauge China’s TFP under constraints of carbon emissions and energy input, thus depicting the economic growth quality. Yu et al. (2019) calculated the GTFP of 230 cities as a vital gauge of high-quality development using the SBM model that includes undesirable outputs, examining the spatial-temporal dynamics of China’s economic transition from a phase of rapid growth to one characterized by high-quality development, spanning the period from 2003 to 2016. Li, (2023) employed the U-SE-SBM-DEA model, along with the global reference ML index to assess the inclusive GTFP. They posited that inclusive green TFP can embody a new development pattern centered on the domestic grand cycle, scientifically encompassing innovation, coordination, green, and sharing concepts, effectively reflecting the level of HQED in China. Zhang et al. (2021) identified green productivity as an indicator of HQED, observing that green finance boosts green productivity by reducing pollution emissions and enhancing clean production, thus advancing HQED. Liu et al. (2023) argued that the quality in HQED not only pertains to utility but also to cost-effectiveness, quality expectations, and competitiveness. Consequently, a steady increase in TFP is considered a key indicator of HQED. Dongming et al. (2023) used the Global Malmquist-Luenberger (GML) productivity index, with inputs including capital stock, labor, and energy consumption, desired output as GDP, and undesirable outputs as emissions of sulfur dioxide, wastewater, and smoke dust, to measure HQED. However, traditional TFP, focusing solely on input constraints of production factors like labor and capital, fails to account for environmental pollution and resource impacts, thus inadequately reflecting the economic effects and resource allocation of production factors, and cannot accurately measure the economic development levels (Hua et al., 2021).
In the realm of green finance and its relationship with sustainable economic development, early theories on green finance predominantly emphasized the critical role of banks and other financial entities in environmental conservation and the promotion of economic health (Jeucken, 2010; Scholtens, 2017; Eremia and Stancu, 2006). Additionally, scholars have also explored the impact of green finance on both the economy and the environment from a macroeconomic perspective. For instance, Salazar (1998) argued that green finance effectively bridges the environment with the economy, enabling economic growth while considering environmental protection. Climent and Soriano (2011) discovered that the innovation and deployment of green financial tools, including green funds, green credits, green bonds, and green insurance, can significantly stimulate economic development and enhance its vitality. Recent studies have leaned more towards examining the relationship between green finance and sustainable economic growth. Pradhan et al. (2018), after analyzing the interconnections between energy consumption, financial development, and economic growth in Financial Action Task Force (FATF) countries, suggested that governments should endorse investments in green finance, particularly within clean energy sectors like natural gas, which not only aid in environmental protection but also foster economic growth. Sachs et al. (2019) noted that innovative financial instruments and policy measures, including green bonds, green banks, carbon market tools, fiscal policies, and green central banking, have opened new avenues for green projects, aiding in achieving sustainable development goals. He et al. (2019) observed that investments in renewable resources have a dual threshold effect on green economic development, indicating that, over the long haul, green investments in renewable energy are capable of driving green economic growth effectively. Moreover, Wang et al. (2022) evaluated the causal dynamics between green finance and sustainable development on a global level using the bootstrap rolling-window Granger causality test. Their empirical findings indicate that green finance has positive effects on sustainable development across various subperiods. Mohsin et al. (2023), through empirical research, also identified green finance as a key element in green and sustainable development. Boubaker and Le (2024) noted that green finance is crucial in fostering sustainable development by steering financial resources towards environmentally sustainable projects. In summary, the aforementioned studies primarily investigate from the perspective of green or sustainable economic development, overlooking a more comprehensive assessment of the quality of economic development.
However, current research on the relationship between green finance and HQED predominantly focuses on the measurement of development levels, with most analyses grounded in theoretical exploration and less in empirical studies. A review of existing literature reveals that numerous scholars have investigated the role of green finance and its impact on HQED, with the majority indicating that green finance can foster such development. Despite rich theoretical analyses enhancing our understanding of this field, the lack of sufficient empirical testing means these conclusions require further validation. To address this gap, this paper employs a combined theoretical and empirical approach, aiming to delve deeper into the exact impact of green finance on HQED, thereby enhancing the credibility of the research.
Compared to existing research, this paper potentially offers innovations in the following three aspects: Firstly, it constructs a multidimensional perspective for measuring HQED. Most scholars, while studying the nexus between green finance and economic growth, have used GDP as the economic growth indicator, this approach fails to fully capture how green finance influences the quality of economic growth. This paper, however, develops HQED indicators from five aspects: innovation, coordination, green, openness, and sharing. It focuses not only on the speed of economic growth but also emphasizes its sustainability, balance, and comprehensiveness, aligning with the call of the national development policy in the new era. Secondly, the paper introduces the mechanistic roles of FinTech and green technological innovation. These two mechanism variables provide a new perspective to understand the pathways of green finance’s impact, aiding in the exploration of factors influencing HQED through green finance. Thirdly, the paper’s heterogeneity analysis involves a division into four regions. Following the economic regional classification method released by China’s National Bureau of Statistics in 2011, which categorizes China into four main regions. This classification, based on socioeconomic characteristics including levels of economic development, industrial structure, and resource endowment, is more suitable for our research subject. This assists in revealing the heterogeneity in green finance development and HQED across different regions, providing a basis for formulating more targeted regional policies.
Building on these innovations, this study firstly examines the impact relationship between green finance and HQED, aiming to enrich the body of research on the determinants of HQED. Secondly, it investigates the moderating effect of green finance on HQED from the perspectives of FinTech and green technological innovation, thereby broadening the scope of external mechanism research between green finance and HQED. Finally, drawing on the insights garnered from this study, the paper offers policy recommendations for governments to enhance the green finance policy system and achieve HQED.
China’s economy has undergone a pivotal transformation, shifting from a period characterized by swift expansion to a stage of high-quality development. In this new phase, green development has become crucial. It is not only integral to HQED but also a vital pathway for transitioning from traditional economic growth models to high-quality development. Green finance, through activities such as green credits, green securities, green investments, and green insurance, directs social capital into environmentally friendly industries, energy-saving industries, renewable energy, and clean transportation. At the same time, it reduces financial support for high pollution levels, excessive energy consumption, and significant emissions sectors. This approach lowers the investment and financing costs for green sectors, reshapes industrial and energy structures, and further propels HQED. Green finance not only alters corporate production methods but also significantly impacts consumer behavior and habits. It guides consumers towards more environmentally-friendly consumption patterns, fostering the rise of green consumption. Particularly in the area of green credits, methods like lowering loan interest rates and increasing credit limits can incentivize the acquisition of eco-friendly products, like new energy vehicles, thereby reducing pollution emissions and promoting the consumption of energy-saving and environmentally friendly products. This consumer behavior stimulates demand for environmentally friendly and green products, prompting the production side to increase the supply of these products and create more employment opportunities. Concurrently, as production scales up, it reduces the prices of consumer goods, further encouraging consumption and creating a virtuous cycle that robustly drives HQED. In light of the foregoing discussion, this paper introduces Research Hypothesis 1:
H1. Green finance significantly promotes HQED.
Technological innovation, particularly green technological innovation, serves as a vital driver of economic growth and a crucial factor in achieving HQED. The process of technological innovation requires substantial investment in research and development (R&D), especially for small and medium-sized enterprises (SMEs), for whom acquiring market financing is a key channel for technological innovation. Green finance facilitates this by providing credit support to green enterprises and issuing green bonds, thereby alleviating the financial burden of R&D and spurring technological innovation, which in turn fosters green and innovative development in the economy. For enterprises characterized by high pollution levels, excessive energy consumption, and significant emissions, green finance promotes technological innovation by guiding capital outflows and restricting credit through financial measures. This compels these enterprises to innovate, aiming to achieve energy efficiency, emission reduction, and HQED. The higher the level of investment in technological R&D, the more green finance can stimulate innovative output, enhancing its role in promoting HQED. In view of the analysis presented above, this paper posits Research Hypothesis 2:
H2. Green technological innovation positively moderates the effect of green finance on HQED.
Financial technology (FinTech), as a product of the deep integration between modern information technology and traditional financial services, started relatively late in China but has shown rapid development (Wu, 2018). It has demonstrated tremendous potential in enhancing the efficiency of financial services and propelling the real economy forward. On one hand, FinTech facilitates faster and more accurate information transmission among various parties in financial activities, significantly reducing information asymmetry. On the other hand, the efficiency of FinTech enables better fulfillment of enterprises’ innovative financing needs, creating a favorable financial ecosystem2 for sustainable corporate development. The objective of green finance is to foster the advancement of green industries, thereby aiding HQED. Given the characteristics of green industries, where green innovation projects typically involve high initial investment and significant uncertainty, and considering that China’s economy is transitioning from rapid expansion to high-quality advancement, replacing old drivers with new ones, the development of green industries has yet to reach maturity. FinTech can aid financial institutions in better identifying green innovation enterprises with potential and strength by reducing information asymmetry. It can provide these projects and businesses with more transparent, friendly, green, efficient, and automated financial support. As increasing financial support and resources flow towards sustainable green fields, green industries will gradually achieve scale effects. In this context, FinTech plays a crucial role in bridging this financial gap, ultimately facilitating the efficient transformation of China’s economic development and promoting high-quality growth. Accordingly, the paper proposes Research Hypothesis 3:
H3. FinTech positively moderates the effect of green finance on HQED.
In summary, the mechanism of green finance’s effect on HQED is shown in Figure 1.
This paper seeks to investigate the nexus between green finance and HQED across 30 provinces in China (Tibet, Hong Kong, Macau, and Taiwan excluded) over the period from 2012 to 2021. The data sources include various annual statistical year-books such as the “China Statistical Yearbook,” “China Science and Technology Statis-tical Yearbook,” “China Environment Statistical Yearbook,” “China Energy Statistical Yearbook,” “China Industrial Statistics Yearbook,” “China Insurance Statistical Year-book,” “China Economic Census Yearbook,” and “China Energy Statistics Yearbook.” In addition, provincial statistical yearbooks and a series of databases like the economy prediction system (EPS), CSMAR (China Stock Market & Accounting Research Database), Wind, and environmental bulletins are also utilized. For the few instances of missing data, interpolation methods were applied to estimate missing values.
HQED research is primarily categorized into two types: the first utilizes Total Factor Productivity (TFP) as a metric to evaluate economic growth quality (Mei and Chen, 2016; Zhang et al., 2023); the other, deriving from the new development concept, devises a comprehensive evaluation system with multiple indicators for HQED (Zhou et al., 2022). Conventional TFP measures focus solely on input limitations of production factors such as labor and capital, neglecting the impact of environmental pollution and resource use, thus failing to comprehensively reflect the economic effects and resource allocation status of production factors, thereby inaccurately measuring the level of economic development (Hua et al., 2021). This study, predicated on an in-depth analysis of the connotations of HQED, in conjunction with the availability of data and referencing the research methodology of Sun et al. (2020), Chen and Huo (2022) and Mao et al. (2023), constructs an index system under the new development concept. This system encompasses five dimensions—innovation, coordination, green, openness, and sharing, comprising five secondary indicators and 26 tertiary indicators. The entropy method is employed to calculate the HQED level across Chinese provinces, with the specific indicators enumerated in Table 1.
Based on the aforementioned methodologies, we computed the HQED indices for the 30 provinces in China in 2012, 2017, and 2021. It is evident from Figure 2 that China’s HQED level displays significant regional heterogeneity. Beijing, Tianjin, and Shanghai consistently exhibit markedly higher HQED levels compared to other provinces throughout the entire period. This phenomenon can be attributed to the policy support enjoyed by municipalities directly under the central government, affording them the ability to consolidate various advantageous resources and thereby gain an absolute advantage in HQED. Conversely, western regions, notably the northwest, consistently manifest lower levels of HQED relative to their counterparts, which can be ascribed to factors such as restricted resource accessibility, underdeveloped infrastructure, and a less conducive policy environment compared to the eastern coastal provinces. Specifically, in 2012, coastal provinces in the eastern region generally outpaced inland provinces in terms of HQED. However, by 2017, after 5 years of development, central regions, particularly provinces surrounding Hubei province, witnessed significant improvements in HQED. In 2021, there was a notable further enhancement in HQED observed in both central and western regions of China, albeit the enduring pattern of higher HQED levels in the east and lower levels in the west persisted. These regional disparities in HQED can be attributed to various factors, including disparities in economic structure, resource endowment, level of industrialization, infrastructure development, and policy support.
Green finance in China is a multifaceted concept, and this study aims to develop a comprehensive green finance index by considering its main components. Leveraging available data and inspired by the research methodologies of He et al. (2019) and Xu et al. (2023), the green finance index is developed encompassing five principal aspects: green credit, green securities, green investment, green insurance, and carbon finance. Firstly, due to the absence of provincial-level statistics on China’s green credit balance, we employ the interest expense ratio of enterprises in the six major high-energy-consuming industries to their total expenses and the Credit Proportion of Environmental Protection Projects as proxies for assessing green credit; Secondly, green securities demonstrate the financial endorsement of the capital market towards energy-saving and environmental protection enterprises. Owing to the late start of green securities and the difficulty in obtaining provincial-level data, this dimension is evaluated based on the market value ratio between listed companies operating in environmental protection sectors and those engaged in the six major high-energy-consuming industries; Thirdly, internationally recognized green insurance primarily encompasses environmental pollution liability insurance and catastrophic disaster insurance. In China, the implementation of compulsory environmental pollution liability insurance only commenced in 2013, resulting in a limited time frame and lacking systematic statistical data. Thus, the development of agricultural insurance, which is significantly affected by natural conditions, is selected as a measure; Fourthly, green investment is represented by the proportion of investment allocated to environmental pollution control and public expenditure on energy-saving and environmental protection; Lastly, carbon finance is denoted by the ratio of domestic and foreign currency loans to carbon emissions. The specific indicators are detailed in Table 2.
The development status of FinTech can be indirectly gauged through the search indices of related keywords. This study utilized the Baidu search index to gather data on the number of searches for FinTech-related keywords in various Chinese provinces from 2012 to 2021, subsequently constructing a FinTech development index. This index reflects the prevalence of FinTech across different regions and time points, meeting the provincial panel data requirements of this research. In determining the keywords, this study initially referred to existing academic research (Yue and Pin, 2015; Sheng and Fan, 2020). Considering the data availability from Baidu search index, a list of keywords related to FinTech was selected for the study. These keywords reflect the diverse applications of FinTech from different perspectives: firstly, from the basic technology perspective, including “big data,” “cloud computing,” “artificial intelligence,” “blockchain,” “biometrics,”; secondly, from the funds payment perspective, covering “online payment,” “mobile payment,” “third-party payment,”; thirdly, FinTech intermediary service models, involving “online lending,” “online financing,” “internet financing,” “internet micro-loans,” “internet loans,” “internet banking,” “electronic banking,” “online banking,” “open banking,” “internet banks,” “direct banks,”; and fourthly, direct terms related to FinTech, such as “internet finance,” “FinTech.” In terms of data processing, the study employed the entropy method to determine the weights of the aforementioned keywords in each dimension, thereby integrating multiple in-dices into a single comprehensive index. This method not only ensures the scientific and objective nature of the composite index but also effectively reveals the comprehensive development level of FinTech in various aspects. The specific indicators are detailed in Table 3.
Green technological innovation is crucial in driving economic growth and sustainable development. Patents are recognized as a standard measure of a company’s technological innovation capacity by the academic community and reflect an enterprise’s capability to innovate in new processes, materials, and technologies (Semenova et al., 2023). This paper, following the “International Patent Green Classification List” promulgated by the World Intellectual Property Organization (WIPO) in 2010, matches the number of green patents applied for and granted at the provincial level annually. Considering that patent grants require time and may impact the economy and society during the granting process, patent applications, as opposed to granted patents, are more timely and reliable. Therefore, drawing on the research of Wurlod and Noailly (2018) and Deng et al. (2021), this study adopts the tally of green invention patent applications as a measurement for green technological innovation.
HQED is influenced not only by the advancement of green finance but also by other macroeconomic variables. Building upon the research of previous scholars and considering the current economic development landscape in China, this study controls a series of variables that affect HQED to mitigate biases caused by omitted variables. Specifically, it identifies a selection of macroeconomic variables as control factors: level of openness to the outside world (OPEN), human capital level (HUMAN), energy structure (ES), labor level (LABOUR), research and development intensity (R&D), and level of educational support (EDU).
The level OPEN reflects the degree of China’s engagement with international markets, measured by the ratio of total imports and exports to the GDP. HUMAN is gauged by the proportion of higher education students to the total population, representing the country’s educational level and talent resources. ES is indicated by the percentage of electricity consumption in total energy consumption, showcasing the structural characteristics of the nation in energy utilization. LABOUR is represented by the natural logarithm of the number of employed individuals, reflecting the scale and state of the labor market. R&D is evaluated by the ratio of R&D internal expenditures to GDP, assessing the nation’s investment in scientific research and innovation. Lastly, EDU is denoted by the ratio of local fiscal education expenditure to general budget expenditure, reflecting the government’s commitment to the education sector. By incorporating these variables, the study aims to comprehensively assess the influence of various macroeconomic factors on HQED. This approach enables a more nuanced understanding of how different economic elements interact and contribute to sustainable economic growth.
This paper, referencing the research methodology of Zhang et al. (2023), employs the entropy method to measure the levels of green finance and HQED. This method uses the degree of variation among the values of various evaluation indicators to depict the importance of each indicator, thereby reducing biases caused by human factors to some extent. Before calculating the entropy value, to avoid subjective biases and address issues such as inconsistency in the types and dimensions of evaluation indicators, we first use the normalization method to dimensionlessly process the original indicators.
Data standardization process:
Eq. 1 standardizes positive indicators, while Eq. 2 standardizes negative indicators. Additionally, to eliminate the impact of zero and negative values, a minimum unit value
Calculation of indicator weights: The proportion of the
Calculation of indicator entropy: Using the results of Eq. 3 to calculate the information entropy
Determination of indicator entropy redundancy: Employing the entropy value
Calculation of weight results: Utilizing the results of Equation 5 to calculate the weight
Comprehensive development level index measurement: The comprehensive evaluation value is the sum of the products of each indicator’s score and its weight, as shown in Equation 7:
In pursuit of exploring the effects of green finance on indicators of HQED, we referenced the research methodology of Balestra and Nerlove (1966). The ensuing regression model was formulated as follows:
in Eq. 8
In the process where green finance facilitates HQED, FinTech plays a pivotal moderating role. Consequently, this paper delves further into whether green finance promotes HQED through FinTech. Drawing on the methodologies of Frazier et al. (2004), an interaction term between green finance and FinTech is incorporated into the regression equation, leading to the construction of the following panel data model,
in Eq. 9
Additionally, this paper will further examine whether green technology innovation amplifies the effect of green finance on HQED. To investigate this, the following panel data model is constructed,
in Eq. 10
To further elucidate the moderating effects of FinTech and green technological innovation more comprehensively, and following the research methods of McCabe et al. (2018) and Zhang and Liu (2015), the marginal effects of green finance on HQED at different percentiles of both FinTech and green technological innovation are computed. The calculation process for the marginal effect of green finance on HQED at a given level of the moderating variable is as follows:
For FinTech:
For green technological innovation:
Eqs 11, 12 indicate how the marginal effect of green finance on HQED varies with the levels of FinTech and green technological innovation.
The descriptive statistics for the variables selected in this research are presented in Table 4. Analysis of the results from Table 4 reveals significant variations within the sample for both the HQED index and the green finance index. The minimum value of the HQED index is 0.107, and its maximum is 0.651, reflecting a progressive increase over time in the quality of economic development. Similarly, the green finance index exhibits a minimum value of 0.078 and a maximum of 0.633, signifying a growing trend in green finance over time. The descriptive statistical outcomes for the other variables are within reasonable bounds and are not elaborated further here.
Prior to estimating the described models empirically, an assessment of multicollinearity was conducted to examine the extent of variance inflation factor (VIF) among the variables, with detailed findings presented in Supplementary Appendix SA. Subsequently, due to the utilization of panel data, a Hausman test was performed on the benchmark regression model (1). The results indicate the rejection of the null hypothesis favoring the random effects model, indicating a preference for adopting a fixed effects model for the benchmark regression analysis. The direct impact of green finance development on the HQED of China is presented in the regression results, as depicted in Table 5.
The findings presented in Table 5 indicate that the regression coefficients for the primary explanatory variables are significantly positive when analyzed using both individual fixed effects and individual time two-way fixed effects models. Specifically, in columns (1) and (2), the impact of green finance on HQED is positive, with the regression coefficient in column (2) being 0.1511, higher than 0.0972 in column (1), both reaching the significant level of 1%. The coefficient of 0.1511 in column (2) implies that a one-unit increase in green finance development corresponds to a 0.1511 unit increase in HQED. This supports the theoretical analysis that green finance fosters HQED and also validates the proposed hypothesis H1 of this study. This conclusion aligns with the perspective that green finance is instrumental in promoting HQED, as echoed in prior research by Yang et al. (2021) and Chen et al. (2023).
Delving deeper, accounting for the time-lag effect of green finance development on HQED, the core explanatory variable lagged by one period was incorporated into columns (3) and (4) for regression analysis. Column (3) uses an individual time two-way fixed effects model, while column (4) uses an individual fixed effects model. The results demonstrate that the coefficients for green finance lagged by one period are significantly positive in both models, being 0.1016 and 0.1683 respectively, and both achieving the significant 1% level. Compared to the non-lagged regression outcomes, these figures reveal higher coefficients and levels of significance, suggesting that green finance with a one-period lag exerts a more potent influence on HQED. This indicates a temporal delay in the impact of green finance development on HQED, meaning that the current level of HQED in a region is also affected by the green finance development of the previous period. This result aligns with the findings of a similar study conducted by Han et al. (2023). This delay can be attributed to the time required for the implementation and realization of benefits from certain green financial instruments.
In terms of control variables, the level of openness to foreign markets, human capital, and research and development intensity all showed a positive correlation with HQED. The significance of these factors highlights the crucial role of an open economy, a high-quality workforce, and sustained technological innovation in propelling HQED.
However, the negative correlation between labor force levels and high-quality development may suggest that merely increasing labor input is not effective in enhancing economic quality. This also implies that future economic growth is likely to rely more on improving labor productivity rather than on increasing the quantity of laborers. The negative coefficients of educational support, significant in columns (2) and (4), may indicate the delayed effects of education investment, which may not yield immediate results in terms of HQED. Alternatively, this could be attributed to the increased education spending potentially crowding out investments in areas that have a more direct impact on HQED, such as technological innovation and environmental protection.
By incorporating a dynamic panel model to analyze the influence of green finance on HQED, and employing the System Generalized Method of Moments (System GMM) to estimate the dynamic panel within the panel, this study selects the first-order lag of the explained variable as an exogenous variable. This approach aims to address the issues of endogeneity and estimation bias present in static panel models. Specifically, in column (2), green finance lagged by one period is included as an independent variable for regression. The estimations derived from the System GMM model regarding the impact of green finance on HQED are illustrated in Table 6. Reflecting the dynamic panel model’s nuances, the endogenous explanatory variables encompass green finance, level of openness to foreign markets, human capital, energy structure, labor level, and research and development intensity. To ensure the regression outcomes’ integrity, evaluations for the model’s validity and the instrumental variables’ efficacy were conducted. The findings, as delineated in Table 6, reveal the error term’s difference showcasing first-order autocorrelation without evidence of second-order autocorrelation, thus accepting the null hypothesis of no autocorrelation in the error term. The p-values for the Hansen test fall between 0.1 and 0.25, indicating that the regression results do not suffer from over-identification and that the number of instrumental variables is reasonable. As indicated in Table 6, green finance lagged by one period is significantly positive at the 5% level, demonstrating a positive effect of the previous period green finance on ensuing HQED, thereby verifying the temporal delay impact of green finance., thus confirming the time-lag effect of green finance. The influence coefficient of green finance on HQED is significantly positive at the 5% level, suggesting that green finance plays a positive guiding and promotional role in HQED. This research finding is consistent with the conclusion of the study by Li (2023), which used the GMM model to discover the promoting effect of green finance on HQED.
Building upon the examination of green finance’s impact on HQED, this study further incorporates variables of FinTech (FT) and green technological innovation (GTI) and constructs interaction terms to scrutinize the moderating effects of FinTech and green technology on green finance’s role in enhancing HQED. Within the framework of the fixed effects model, a multivariate regression analysis is conducted using panel data. To empirically test the moderation effect model and address the issue of multicollinearity, the interaction terms in models (2) and (3) are decentered, with the regression results presented in Table 7. Column (1) demonstrates that green finance (GF) has a significant positive impact on HQED. Column (2) adds FinTech (FT) and the interaction term of green finance and FinTech (GF*FT) to column (1). It reveals that the interaction term’s coefficient is significantly positive at the 1% level, underscoring that FinTech significantly enhances the positive effect of green finance on HQED, thus confirming the proposed hypothesis H3. This result aligns with the findings of a similar study conducted by Yang et al. (2021). It also further demonstrates that FinTech plays a moderating role in the impact of green finance on HQED. This may be attributed to FinTech improving the efficiency of financial services, thereby strengthening the role of green finance in resource allocation.
Column (3) examines the role of green technology innovation (GTI), with results indicating that the independent variable of green technology innovation has no significant impact on HQED. Column (4) incorporates the interaction term of green finance and green technology (GF*GTI), with its coefficient also being significantly positive at the 1% level. This suggests that with the support of green finance, the application of green technology significantly positively contributes to HQED, confirming hypothesis H2. This conclusion is in harmony with the perspective that green technology serves as a moderator in the nexus between green finance and HQED, as suggested by Li (2023). These estimated outcomes posit that GTI constitutes a crucial conduit by which green finance bolsters HQED, indeed playing a positive moderating role. The rationale is that green finance can provide substantial funding for the development of GTI. With robust financial support, GTI more effectively serves industrial transformation, green development, innovative development, open development, inclusive development, thereby driving HQED.
To further elucidate the moderating effects of FinTech and GTI, we calculated the marginal effects. According to Table 7, the coefficient for the interaction term between green finance and FinTech is 0.2622, and the coefficient for green finance is 0.0743. Given the median (P50) value for FinTech is 0.179 (Table 4), using our formula (11), the marginal effect of green finance at P50 for FinTech is calculated as 0.0743 + 0.2622 × 0.179. Thus, at the 50th percentile of FinTech, the marginal effect of green finance on HQED is 0.1212. Similarly, for GTI, the coefficient for the interaction term between green finance and GTI is 0.0718, and the coefficient for green finance is 0.0800. Given the 50th percentile (P50) value for GTI is 0.1994, according to formula (12), the marginal effect of green finance at P50 for GTI is calculated as 0.0800 + 0.0718 × 0.1994. Thus, at the 50th percentile of GTI, the marginal effect of green finance on HQED is 0.0943.
Furthermore, we calculated the marginal effects at the 0th, 10th, 20th, ., and 100th percentiles of both FinTech and GTI, as displayed in Supplementary Appendix SB1. Specifically, at the 0th percentile of FinTech (i.e., the minimum value), the marginal effect of green finance on HQED is 0.0757. As FinTech advances to the 10th percentile, this marginal effect increases to 0.0870, demonstrating the enhancing role of FinTech in magnifying the impact of green finance. This trend continues, with the marginal effect reaching 0.2951 at the 100th percentile (the maximum value), which is approximately four times the initial value at the 0th percentile (the minimum value). GTI also shows similar results in magnifying the impact of green finance.
This reveals that both FinTech and GTI consistently enhance the positive impact of green finance on HQED across their respective percentiles. As the levels of FinTech and GTI increase, the marginal effects of green finance on HQED become significantly stronger, confirming the hypothesized moderating roles of these variables. This incremental pattern underscores the importance of integrating advanced FinTech and GTI to maximize the efficacy of green finance in promoting HQED.
To ascertain the empirical findings’ robustness, this paper employs four distinct methods for robustness testing. First, the explanatory variable is substituted. Green finance encompasses tools such as green credit, green securities, green insurance, green investment, and carbon finance. Green credit is a pivotal instrument utilized by the government to incentivize financial institutions to channel resources and steer capital towards areas of environmentally friendly production. According to the research by Zhang et al. (2021), this study opts to use green credit as a substitute for green finance as the explanatory variable. Second, the sample interval is replaced. Considering the policy specificity of the municipalities, and referring to the approach of and Xu et al. (2020), the sample is re-estimated after excluding the four municipalities of Beijing, Tianjin, Shanghai, and Chongqing. Third, additional control variables are included. There are many objective factors affecting HQED, and to reduce the bias in estimation results due to omitted variable bias, this paper, following Zhang et al. (2023), further controls for the level of urbanization. Fourth, data from the year 2012 is excluded. Since the China Banking Regulatory Commission issued the “Green Credit Guidelines” in 2012, prompting financial institutions in the banking sector to develop green credit, and since green credit is a major component and an important influencing factor of green finance, the data on green finance post-2012 is more effective, thus excluding the data for 2012. Columns (1), (2), and (3) show the estimation results with green credit as the substituted explanatory variable; Column (4) presents the results after redefining the sample interval; Column (5) shows the regression results after excluding the 2012 sample data, and column (6) reports the results after adding new control variables. According to the results in Table 8, whether substituting the explanatory variable, changing the sample interval, adding control variables, or excluding specific year data, the coefficient of green finance remains significantly positive, further affirming the robust and reliable role of green finance in promoting HQED.
Considering the vast geographical expanse of China and the varying socioeconomic development across different regions, this study, based on the economic regional division method published by the National Bureau of Statistics of China in 2011, categorizes China into Eastern, Central, Western, and Northeastern regions3. This classification, based on socioeconomic characteristics including levels of economic advancement levels, industrial composition, and resource endowment, is more suitable for our research subject. Notably, variations in the levels of green finance exist among these regions, potentially influencing the quality of economic development differently. This study delves into the regional heterogeneity of this influence, with the results detailed in Table 9. The results demonstrate a significant positive correlation between green finance and HQED in the eastern region, marked by a coefficient of 0.1271, statistically significant at the 1% level. This implies that a 1% increment in green finance in the eastern region could enhance HQED by 0.1271%. This may be ascribed to the region’s more mature financial markets and a richer array of green financial tools and services, effectively promoting green technologies and industries. Although the central region also shows a positive impact of green finance on HQED, denoted by a coefficient of 0.0635, it is significant only at the 5% level, suggesting a weaker influence compared to the eastern region. Nonetheless, it reaffirms the positive role of green finance in the central region’s HQED. In contrast, the influence of green finance on HQED in the western region is not significant, with a coefficient of 0.0555, indicating that green finance has not yet become a significant driver of HQED in this region. Possible reasons include inadequate coverage of basic financial services, underdeveloped green finance products and mechanisms, and a weaker foundation for green industries. The northeastern region presents a unique case, with a coefficient of −0.0238 for green finance’s impact on HQED. Although not significant, the negative coefficient may indicate that the relationship between green finance and HQED is not consistently positive in this region.
It is noteworthy to discuss that some control variables exhibit negative significance. Firstly, the impact of the energy structure in the Western region on HQED is negatively significant. The energy structure is reflected through the proportion of electricity consumption in total energy consumption. The negative coefficient might stem from the Western region’s reliance on traditional energy sources like coal (Mi and Sun, 2021; Hongjun et al., 2023). Additionally, a high ratio of electricity consumption may indicate a higher level of industrialization, which could entail environmental pollution and excessive resource extraction, contradicting the principles of HQED that emphasize sustainability and eco-friendliness. Secondly, in the Eastern and Central regions, the level of the workforce (measured by the number of employed individuals) negatively influences HQED. This could be attributed to the lack of corresponding increases in labor productivity despite the rising labor force numbers in China, a consequence of demographic changes in the working population (Wu et al., 2023). This means that the increase in labor has not translated into more efficient production and services. Furthermore, the Eastern and Central regions may face issues of labor surplus, leading to inefficient employment and overcapacity, which are detrimental to HQED. Thirdly, financial support for education in the Eastern region has a negative impact on HQED. This could be due to the delayed effects of education investment, which typically takes a long period to manifest in economic development and may not yield immediate visible outcomes (Hanushek and Woessmann, 2010). Alternatively, it could be because an increase in education spending might crowd out investments in other areas more directly impacting HQED, such as technological innovation and environmental protection.
Overall, the impact of green finance on the HQED of the eastern, central, western, and northeastern regions exhibits significant regional disparities. The eastern region benefits the most conspicuously, succeeded by the central region, while the influence of green finance is comparatively weaker in the western and northeastern regions. This conclusion, that the promotion effect of green finance on high-quality economic development is more significant in the eastern and central regions of China compared to other regions, corresponds with the findings of Li (2023). However, it differs slightly from the findings of Yang (2023), who also acknowledged the heterogeneity of the impact of green finance on HQED but believed that the influence was greater in the eastern and western regions than in the central region. This contrast highlights the complexities and varying perspectives in current research, further demonstrating the spatial heterogeneity of the impact of green finance on HQED. This disparity likely reflects the differences in economic development levels, financial market maturity, and green finance policies and practices among these regions. This underscores the need for not only strengthened policy coordination between regions in advancing green finance but also for taking into consideration the unique characteristics of each region to maximize the benefits of green finance. This disparity likely reflects the differences in economic development levels, financial market maturity, and green finance policies and practices among these regions. This underscores the need for not only strengthened policy coordination between regions in advancing green finance but also for taking into consideration the unique characteristics of each region to maximize the benefits of green finance.
After analyzing regional heterogeneity, we further examined the moderating effects of FinTech and GTI on the impact of green finance across different regions. The results indicate that, although the interaction terms between green finance and both FinTech and GTI are significantly positive in the eastern region, the core coefficient of green finance becomes insignificant after including the moderating terms. This suggests that while FinTech and GTI exhibit significant moderating effects in these regions, they may diffuse the direct impact of green finance, rendering its independent effect insignificant. In contrast, in the central, western, and northeastern regions, such moderating effects are not significant. This may be due to the lower development levels of FinTech and GTI in these areas, as well as the inadequate infrastructure for financial services and technological support, which fails to significantly enhance the efficacy of green finance. Detailed results are presented in Supplementary Appendix SB2.
In the context of the global environmental challenges and economic development needs of the 21st century, green finance, as a vital instrument for supporting sustainable development, has increasingly attracted attention for its role in promoting HQED. This study analyzes panel data from 30 provinces in China spanning from 2012 to 2021, examining the relationship between green finance and HQED across Chinese regions from the perspectives of FinTech and green technological innovation (GTI) and conducting an analysis of regional heterogeneity. The outcomes reveal that: Firstly, the enhancement of green finance significantly fosters HQED, exerting a notable promotional effect on it. Secondly, the mechanism test further validates that FinTech and GTI are crucial pathways through which green finance promotes HQED, indeed playing a positive moderating role in the relationship between green finance and HQED. Finally, The impact of green finance on HQED is characterized by marked regional disparities. The eastern region benefits the most, succeeded by the central region, while the influence of green finance is relatively weaker in the western and northeastern regions. Lastly, the moderating effects of FinTech and GTI on the impact of green finance on HQED also exhibit regional heterogeneity.
Firstly, fostering green finance development with a long-term vision requires coordinated efforts from policymakers and financial institutions. Government agencies should prioritize the development of green finance by creating favorable regulatory frameworks and offering incentives for green financial products and services, including green credit, green securities, green investments, and green insurance. Financial institutions should intensify the promotion of these products to facilitate broader market adoption. Given the lag effect of green finance on HQED, it is advised to consider long-term benefits in policy formulation and project evaluation rather than relying solely on short-term economic indicators. Additionally, establishing a comprehensive tracking and evaluation mechanism is recommended to continually monitor and assess the long-term impacts of green finance projects.
Secondly, maximizing the catalytic role of GTI and FinTech in bolstering green finance for HQED requires active support from both the government and private sector. Government agencies should provide grants and subsidies to encourage corporate investment in GTI and FinTech. Companies should leverage technologies like big data, cloud computing, blockchain, and artificial intelligence to enhance the transparency and tracking capabilities of green finance projects, thereby improving the efficiency and coverage of green financial products and services. This approach will better leverage the potential of GTI and FinTech in facilitating the role of green finance in HQED.
Thirdly, formulating differentiated strategies based on regional heterogeneity. Given the observed regional disparities in green finance’s impact across the Eastern, Central, Western, and Northeastern regions in China, it is imperative for policymakers and financial bodies to implement more precise regional strategies. For the Eastern and Central regions, government agencies should provide further support for green finance initiatives through subsidies, tax incentives, and public-private partnerships to consolidate and expand its role in HQED. For the Western and Northeastern regions, despite the less significant promotional effects observed, this does not diminish the importance of green finance in economic development. It is recommended to analyze the reasons behind the insufficient impact of green finance in these regions such as lack of funds, technological backwardness, immature markets, etc., and provide tailored policy support and financial services to improve green finance and the capacity for HQED in these areas.
This study primarily employed empirical methods and variable data to explore green finance, facing limitations due to a scarcity of relevant data and a narrow geographic focus on China. This approach restricted the depth and breadth of our empirical analysis, offering a limited perspective on a subject with global implications. Future research should aim for a more comprehensive understanding by utilizing a wider array of data and expanding the geographical scope, thereby enabling a deeper investigation into the nuances and complexities of how green finance contributes to HQED.
The original contributions presented in the study are included in the article/Supplementary Material, further inquiries can be directed to the corresponding author.
YX: Conceptualization, Data curation, Formal Analysis, Methodology, Software, Validation, Writing–original draft, Writing–review and editing. ZD: Conceptualization, Formal Analysis, Funding acquisition, Methodology, Supervision, Writing–review and editing.
The author(s) declare that financial support was received for the research, authorship, and/or publication of this article. This research was supported by the National Social Science Foundation of China (21CGL026).
The authors declare that the research was conducted in the absence of any commercial or financial relationships that could be construed as a potential conflict of interest.
All claims expressed in this article are solely those of the authors and do not necessarily represent those of their affiliated organizations, or those of the publisher, the editors and the reviewers. Any product that may be evaluated in this article, or claim that may be made by its manufacturer, is not guaranteed or endorsed by the publisher.
The Supplementary Material for this article can be found online at: https://www.frontiersin.org/articles/10.3389/fenvs.2024.1414365/full#supplementary-material
1Data source: “China Statistical Yearbook”.
2Financial ecosystem refers to the infrastructure, fundamental institutions, and external economic environment on which financial operations rely (Xu, 2005).
3To accurately reflect the socioeconomic development status of different regions in China, the National Bureau of Statistics of China released the “Method for Dividing Eastern, Central, Western, and Northeastern Regions” in 2011. This method divides China’s economic regions into four major areas: Eastern, Central, Western, and Northeastern.
Aiken, L. S., and West, S. G. (1991). Multiple regression: testing and interpreting interactions. Thousand Oaks, CA: Sage google Sch. 2, 103–135.
Balestra, P., and Nerlove, M. (1966). Econometric analysis of panel data. Econ. Theory 10 (1), 1–29.
Berikhanovna, C. M., Bauirzhanovna, B. A., Kudaibergenovna, N. G., Gulbagda, B., and Serikovna, Y. G. (2023). The influence of green credit policy on green innovation and transformation and upgradation as a function of corporate diversification: the case of Kazakhstan. Economies 11 (8), 210. doi:10.3390/economies11080210
Boubaker, S., and Le, T. H. (2024). Green finance and sustainable development goals. World Sci. Eur., 420. doi:10.1142/q0427
Chen, J., Li, L., Yang, D., and Wang, Z. (2023). The dynamic impact of green finance and renewable energy on sustainable development in China. Front. Environ. Sci. 10, 1097181. doi:10.3389/fenvs.2022.1097181
Chen, L., and Huo, C. (2022). The measurement and influencing factors of high-quality economic development in China. Sustainability 14 (15), 9293. doi:10.3390/su14159293
Climent, F., and Soriano, P. (2011). Green and good? The investment performance of US environmental mutual funds. J. Bus. Ethics 103, 275–287. doi:10.1007/s10551-011-0865-2
Deng, Y. P., Wang, L., and Zhou, W. J. (2021). Does environmental regulation promote green innovation capability. Evid. China. Stat. Res 38, 76–86. doi:10.19343/j.cnki.11-1302/c.2021.07.006
Dongming, W., Yang, X., and Naihua, G. (2023). Digital economy promote the high-quality economic development. Sci. Res. Manag. 44 (9), 10.
Du, M., Zhang, R., Chai, S., Li, Q., Sun, R., and Chu, W. (2022). Can green finance policies stimulate technological innovation and financial performance? Evidence from Chinese listed green enterprises. Sustainability 14 (15), 9287. doi:10.3390/su14159287
Eremia, A., and Stancu, I. (2006). Banking activity for sustainable development. Theor. Appl. Econ. 6 (501), 23–32.
Frazier, P. A., Tix, A. P., and Barron, K. E. (2004). Testing moderator and mediator effects in counseling psychology research. J. Couns. Psychol. 51 (1), 115–134. doi:10.1037/0022-0167.51.1.115
Fu, C., Lu, L., and Pirabi, M. (2023). Advancing green finance: a review of sustainable development. Digital Econ. Sustain. Dev. 1 (1), 20. doi:10.1007/s44265-023-00020-3
Gao, B. Y., Huang, Z. J., Zhang, T. T., Sun, X. Y., and Song, M. Y. (2022). Exploring the impact of industrial land price distortion on carbon emission intensity: evidence from China. Land 12 (1), 92. doi:10.3390/land12010092
Hafeez, M., Yuan, C., Yuan, Q., Zhuo, Z., Stromaier, D., and Sultan Musaad O, A. (2019). A global prospective of environmental degradations: economy and finance. Environ. Sci. Pollut. Res. 26, 25898–25915. doi:10.1007/s11356-019-05853-0
Han, J., Zheng, Q., **e, D., Muhammad, A., and Isik, C. (2023). The construction of green finance and high-quality economic development under China’s SDGs target. Environ. Sci. Pollut. Res. 30 (52), 111891–111902. doi:10.1007/s11356-023-28977-w
He, L., Liu, R., Zhong, Z., Wang, D., and Xia, Y. (2019). Can green financial development promote renewable energy investment efficiency? A consideration of bank credit. Renew. Energy 143, 974–984. doi:10.1016/j.renene.2019.05.059
Hongjun, G., Liye, D., and Aiwu, Z. (2023). Energy structure dividend, factor allocation efficiency and regional productivity growth--An empirical examination of energy restructuring in China. Energy Policy 172, 113307. doi:10.1016/j.enpol.2022.113307
Hua, X., Lv, H., and Jin, X. (2021). Research on high-quality development efficiency and total factor productivity of regional economies in China. Sustainability 13 (15), 8287. doi:10.3390/su13158287
Islam, M. M., Chowdhury, M. A. M., Begum, R. A., and Amir, A. A. (2022). A bibliometric analysis on the research trends of climate change effects on economic vulnerability. Environ. Sci. Pollut. Res. 29 (39), 59300–59315. doi:10.1007/s11356-022-20028-0
Jeucken, M. (2010). Sustainable finance and banking: the financial sector and the future of the planet. China: Routledge.
Jeucken, M., and Bouma, J. J. (2017). “The changing environment of banks,” in Sustainable banking (China: Routledge), 24–38.
Khan, M. A., Riaz, H., Ahmed, M., and Saeed, A. (2022a). Does green finance really deliver what is expected? An empirical perspective. Borsa Istanb. Rev. 22 (3), 586–593. doi:10.1016/j.bir.2021.07.006
Khan, S., Akbar, A., Nasim, I., Hedvičáková, M., and Bashir, F. (2022b). Green finance development and environmental sustainability: a panel data analysis. Front. Environ. Sci. 10, 2134. doi:10.3389/fenvs.2022.1039705
Kumar, B., Kumar, L., Kumar, A., Kumari, R., Tagar, U., and Sassanelli, C. (2023). Green finance in circular economy: a literature review. Environ. Dev. Sustain. 26, 16419–16459. doi:10.1007/s10668-023-03361-3
Li, C. G. (2023). The impact of green finance on high-quality economic development. J. Zhongnan Univ. Econ. Law (02), 65–77. doi:10.19639/j.cnki.issn1003-5230.2023.0014
Liu, L., Si, S., and Li, J. (2023). Research on the effect of regional talent allocation on high-quality economic development—based on the perspective of innovation-driven growth. Sustainability 15 (7), 6315. doi:10.3390/su15076315
Lu, N., Wu, J., and Liu, Z. (2022). How does green finance reform affect enterprise green technology innovation? Evidence from China. Sustainability 14 (16), 9865. doi:10.3390/su14169865
Lv, C., Shao, C., and Lee, C. C. (2021). Green technology innovation and financial development: do environmental regulation and innovation output matter? Energy Econ. 98, 105237. doi:10.1016/j.eneco.2021.105237
Mao, J., Wang, Z., and Ma, T. (2023). Dynamic evolution of high-quality economic development levels: regional differences and distribution in west China. Land 12 (11), 1975. doi:10.3390/land12111975
McCabe, C. J., Kim, D. S., and King, K. M. (2018). Improving present practices in the visual display of interactions. Adv. Methods Pract. Psychol. Sci. 1 (2), 147–165. doi:10.1177/2515245917746792
Mei, L., and Chen, Z. (2016). The convergence analysis of regional growth differences in China: the perspective of the quality of economic growth. J. Serv. Sci. Manag. 9 (6), 453–476. doi:10.4236/jssm.2016.96049
Mi, Z., and Sun, X. (2021). Provinces with transitions in industrial structure and energy mix performed best in climate change mitigation in China. Commun. Earth Environ. 2 (1), 182. doi:10.1038/s43247-021-00258-9
Mohsin, M., Iqbal, N., and Iram, R. (2023). The nexus between green finance and sustainable green economic growth. Energy Res. Lett. 4. doi:10.46557/001c.78117
OECD (2016). Green financing: challenges and opportunities in the transition to a clean and climate-resilient economy. OECD J. Financial Mark. Trends 2016 (2), 63–78. doi:10.1787/fmt-2016-5jg0097l3qhl
Pradhan, R. P., Arvin, M. B., Nair, M., Bennett, S. E., and Hall, J. H. (2018). The dynamics between energy consumption patterns, financial sector development and economic growth in Financial Action Task Force (FATF) countries. Energy 159, 42–53. doi:10.1016/j.energy.2018.06.094
Sachs, J., Woo, W. T., and Taghizadeh-Hesary, F. (2019). Handbook of green finance: energy security and sustainable development. (No Title).
Salazar, J. (1998). “Environmental finance: linking two worlds,” in A workshop on financial innovations for biodiversity (Bratislava: Virginia Tech), 1–18.
Scholtens, B. (2017). Why finance should care about ecology. Trends Ecol. Evol. 32 (7), 500–505. doi:10.1016/j.tree.2017.03.013
Semenova, A., Semenov, K., and Storchevoy, M. (2023). One, two, three: how many green patents start bringing financial benefits for small, medium and large firms? Economies 11 (5), 137. doi:10.3390/economies11050137
Sheng, T. X., and Fan, C. L. (2020). Fintech, optimal banking market structure, and credit supply for SMEs. J. Financial Res. 480 (6), 114–132.
Sun, H., Gui, H. Q., and Yang, D. (2020). Measurement and evaluation of the high-quality of China provincial economic development. Zhejiang Soc. Sci. 8, 4–14. doi:10.14167/j.zjss.2020.08.001
Sun, X., Zhou, C., and Gan, Z. (2023). Green finance policy and ESG performance: evidence from Chinese manufacturing firms. Sustainability 15 (8), 6781. doi:10.3390/su15086781
Tol, R. S. J. (2009). The economic effects of climate change. J. Econ. Perspect. 23 (2), 29–51. doi:10.1257/jep.23.2.29
Wang, X., Elahi, E., and Khalid, Z. (2022). Do green finance policies foster environmental, social, and governance performance of corporate? Int. J. Environ. Res. Public Health 19 (22), 14920. doi:10.3390/ijerph192214920
Wang, Z., Teng, Y. P., Wu, S., and Chen, H. (2023). Does green finance expand China’s green development space? Evidence from the ecological environment improvement perspective. Systems 11 (7), 369. doi:10.3390/systems11070369
White, M. A. (1996). Environmental finance: value and risk in an age of ecology. Bus. strategy Environ. 5 (3), 198–206. doi:10.1002/(sici)1099-0836(199609)5:3<198::aid-bse66>3.3.co;2-w
Wu, Y., Su, B., and Li, J. (2023). The impact of low fertility rates on labor demand and socioeconomic development in China. China CDC Wkly. 5 (27), 599–604. doi:10.46234/ccdcw2023.115
Wu, Y. N. (2018). Legal challenges and regulations for the application of frontiers of financial technology—a perspective of block chain and regulatory technology. J. Dalian Univ. Technol. Soc. Sci. 39 (03), 78–86. doi:10.19525/j.issn1008-407x.2018.03.011
Wurlod, J. D., and Noailly, J. (2018). The impact of green innovation on energy intensity: an empirical analysis for 14 industrial sectors in OECD countries. Energy Econ. 71, 47–61. doi:10.1016/j.eneco.2017.12.012
Xiong, X., Wang, Y., Liu, B., He, W., and Yu, X. (2023). The impact of green finance on the optimization of industrial structure: evidence from China. PloS One 18 (8), e0289844. doi:10.1371/journal.pone.0289844
Xu, H., Mei, Q., Shahzad, F., Liu, S., Long, X., and Zhang, J. (2020). Untangling the impact of green finance on the enterprise green performance: a meta-analytic approach. Sustainability 12 (21), 9085. doi:10.3390/su12219085
Xu, J., Wang, J., Li, R., and Gu, M. (2023). Is green finance fostering high-quality energy development in China? A spatial spillover perspective. Energy Strategy Rev. 50, 101201. doi:10.1016/j.esr.2023.101201
Xu, X. L. (2005). The assessment of regional financial ecology environment. Financial Res. (11), 39–45.
Yang, Y., Su, X., and Yao, S. (2021). Nexus between green finance, fintech, and high-quality economic development: empirical evidence from China. Resour. Policy 74, 102445. doi:10.1016/j.resourpol.2021.102445
Yang, Z. (2023). The impact of green finance on high-quality economic development in China: vertical fiscal imbalance as the moderating effect. Sustainability 15 (12), 9350. doi:10.3390/su15129350
Yin, X., and Xu, Z. (2022). An empirical analysis of the coupling and coordinative development of China’s green finance and economic growth. Resour. Policy 75, 102476. doi:10.1016/j.resourpol.2021.102476
Yu, B., Liu, L., and Chen, H. (2023). Can green finance improve the financial performance of green enterprises in China? Int. Rev. Econ. Finance 88, 1287–1300. doi:10.1016/j.iref.2023.07.060
Yu, Y. Z., Yang, X. Z., and Zhang, S. H. (2019). Research on the characteristics of time and space conversion of China’s economy from high-speed growth to high-quality development. J. Quant. Tech. Econ. 36, 3–21. doi:10.13653/j.cnki.jqte.2019.06.001
Yue, S., and Pin, G. (2015). Internet finance, technology spillover and commercial banks TFP. J. Financ. Res. 3, 160–174.
Zhang, C., and Liu, G. (2015). Evolving marginal effects of financial structure on economic growth. Econ. Res. J. 50, 84–99.
Zhang, D., Jiao, F., Zheng, X., and Pang, J. (2023). Analysis of the influence mechanism of new urbanization on high-quality economic development in northeast China. Sustainability 15 (10), 7992. doi:10.3390/su15107992
Zhang, J. K., Hou, Y. Z., Liu, P. L., He, J. W., and Zhuo, X. (2019). The goals and strategy path of high-quality development. Manag. World 35 (7), 1–7. doi:10.19744/j.cnki.11-1235/f.20190711.001
Zhang, S., Liang, B. B., Xu, S. Z., and Hou, J. L. (2023). Empirical analysis of green finance and high-quality economic development in the Yangtze River Delta based on VAR and coupling coordination model. Front. Environ. Sci. 11, 1211174. doi:10.3389/fenvs.2023.1211174
Zhang, S., Wu, Z., Wang, Y., and Hao, Y. (2021). Fostering green development with green finance: an empirical study on the environmental effect of green credit policy in China. J. Environ. Manag. 296, 113159. doi:10.1016/j.jenvman.2021.113159
Keywords: green finance, high-quality economic development (HQED), FinTech, green technological innovation, regional disparities
Citation: Xu Y and Ding Z (2024) Sustainable growth unveiled: exploring the nexus of green finance and high-quality economic development in China. Front. Environ. Sci. 12:1414365. doi: 10.3389/fenvs.2024.1414365
Received: 08 April 2024; Accepted: 11 July 2024;
Published: 26 July 2024.
Edited by:
Wei Zhang, China University of Geosciences Wuhan, ChinaReviewed by:
Diby Francois Kassi, Henan University, ChinaCopyright © 2024 Xu and Ding. This is an open-access article distributed under the terms of the Creative Commons Attribution License (CC BY). The use, distribution or reproduction in other forums is permitted, provided the original author(s) and the copyright owner(s) are credited and that the original publication in this journal is cited, in accordance with accepted academic practice. No use, distribution or reproduction is permitted which does not comply with these terms.
*Correspondence: Yige Xu, MjAyMTAzMzA1QHN0dS5zaWNhdS5lZHUuY24=; Zhao Ding, emRpbmdAc2ljYXUuZWR1LmNu
Disclaimer: All claims expressed in this article are solely those of the authors and do not necessarily represent those of their affiliated organizations, or those of the publisher, the editors and the reviewers. Any product that may be evaluated in this article or claim that may be made by its manufacturer is not guaranteed or endorsed by the publisher.
Research integrity at Frontiers
Learn more about the work of our research integrity team to safeguard the quality of each article we publish.