- School of Environment and Resource, Southwest University of Science and Technology, Mianyang, Sichuan, China
The spatial distribution and source analysis of heavy metals in soil are of great significance for the risk assessment and control of heavy metal pollution. Herein, a total of 87 topsoil samples (0–30 cm) were collected in the areas adjacent to a non-ferrous metal slag field of Gejiu City, Yunnan Province. The typical heavy metal concentrations, available phosphorus, available potassium, ammonium nitrogen, nitrate nitrogen, and pH in each soil sample were determined. Based on the above basic data, distribution and origins of heavy metals was analyzed. The results indicated that the mean contents of Pb, As, Cu, Zn, Ni and Cr in the topsoil of the study site were 247.82, 204.36, 104.83, 411.38, 29.73 and 67.59 mg kg-1, respectively. Exception of the Ni element, the contents of the remaining five heavy metals surpassed their respective background levels in study site. Especially, As element (with the highest accumulation effect) is 11.1l times of the background level, and the remaining four elements are 1.04–6.10 times of the background level in Yunnan. Single factor pollution index showed that the pollution degree of Pb, Cu and Zn was light, and the accumulation of As was a great threat to soil quality. Furthermore, the percentages of sites at mild pollution, moderate pollution and heavy pollution levels were 16.09%, 26.09% and 63.22%, respectively. Additionally, The order of potential ecological hazard degree of soil in the survey area was As > Pb > Cu = Ni > Zn = Cr. The spatial dispersion of heavy metals within the study region is impacted by both natural phenomena and human activities. The areas with severe pollution were the mining area in the northwest and planting sites in the east, middle and south of the study area. The main contribution sources of Ni were the mixed sources of natural parent material and industrial activities. The heavy metal element largely contributed by industrial activities and transportation is Pb. The main sources of metals As, Cu and Zn are the integrated sources of agricultural and industrial activities. The main source of Cr is natural source. Therefore, these useful results can guide the heavy metal pollution control and restoration of study site and this research work can also be used to other similar metal slag field and its affected area.
1 Introduction
Southwest China, a typical karst region, where mining activities are intense, has left behind a large number of slag fields. These slag fields, whose soil are rich in toxic substances, such as heavy metals, pose a huge threat to the ecological environment. Because the heavy metals with long-lasting bioavailability could directly harm human health on the one hand (Burges et al., 2015; Mohamed et al., 2024), and also lead to the degradation of the soil function of the cultivated land around the slag field on the other hand. Additionally, previous studies have indicated that carbonate rocks were the predominant rock type of karst regions, which results the higher levels of heavy metal elements in carbonate weathered soils compared to non-karst areas (Liu et al., 2023; Sowers et al., 2024). Furthermore, heavy metal contamination in soils within karst regions also has the characteristics of small holding capacity, strong mobility, and high potential of ecological hazards (Hosseinniaee et al., 2023; Proshad and Idris, 2023). Therefore, studying the spatial distribution and source apportionment of soil heavy metals is of great significance for understanding the current status of heavy metal pollution in soils of karst areas.
As for spatial distribution, it was found that the spatial variability of soil heavy metal content in typical karst areas might be attributed to the distinctive landform and soil parent material characteristics of karst environments, based on classical statistics and ordinary kriging method (Yang et al., 2024). Because the accumulation of heavy metals in the soil adjoining the slag field was notably pronounced and reached moderate-strong pollution level (Zhang et al., 2021; Hasan et al., 2024). Meanwhile, a variety of evaluation methods including enrichment coefficient method (Wang G. et al., 2019), single factor pollution index method (Wang Z. H. et al., 2019), Nemerow comprehensive index method (Zhang et al., 2018), and potential ecological hazard index method (Nambiar et al., 2020) have been proposed for the evaluation of the environmental pollution of soil. In addition, with the research progress in space technology, multivariate statistics and geostatistics (e.g., inverse distance weighting) are also widely used in the study of spatial distribution patterns of soil heavy metals and comprehensive evaluation of environmental pollution (Hossain et al., 2015; Zhao et al., 2019; Siddiqui et al., 2020). As for source analysis, Positive matrix factorization (PMF) model, Absolute Principal Component Score-Multiple Linear Regression (APCS-MLR), and Chemical Mass Balance (CMB) (Ren et al., 2021; Zhang et al., 2021) were always used to ascertain the origin of heavy metals and quantitatively analyze the contribution of different sources in non-karst areas (Wang Z. H. et al., 2019). Source apportionment can further identify the main sources of soil heavy metals (Pathak et al., 2015; Li et al., 2017; Cao et al., 2020).
However, systematic investigations of spatial distribution and source apportionment of soil heavy metals in karst areas are relatively limited, especially for the slag-field influenced soil, and most of the studies are only based on local sampling points or single heavy metal indicator in soil (Wang et al., 2020; Huang et al., 2021). In these studies, experimental data were not combined with multivariate, spatial, and geostatistical analysis, and thus, the interactions between heavy metal distribution and the driving factors were unknown. Furthermore, heavy metal spatial distribution and proximity effect have not been elucidated (Yan et al., 2023).
Therefore, this study proposed integrated research framework for heavy metal pollution risk assessment and pollution source analysis of metal slag field and its affected area located in the karst mountain region. Gejiu City, a typical karst region of Yunnan province of China, owned various non-ferrous metal slag field, thus the area around the slag field was likely to face huge heavy metal pollution problems. However, there are still gaps in the research on the spatial distribution and source analysis of heavy metals pollution in this area. This study focused on the spatial distribution, environmental risk assessment and pollution sources analysis in the soil of area influenced by non-ferrous metal slag field at Gejiu City. The results have the potential to provide scientific basis for the control and management of heavy metals pollution on soil in the affected area of slag yard at karst region.
2 Materials and methods
2.1 Study area
The area is a characteristic non-ferrous metal slag field and its affected area in Gejiu City, Yunnan Province. It is located in the karst mountain slope and valley area, which belongs to the landform of low and middle mountain karst slope. The field is bare or semi-exposed karst slope. This area has a typical karst background: fragile ecological environment, low vegetation coverage, complex terrain, large undulations, barren soil layers, and high degree of rock exposure (Figure 1). The study area was mainly forestland, grassland, and cultivated land with the main crops of corn, tomato, and rice. The area falls within the classification of a subtropical mountainous humid monsoon climate. The area exhibits a mean annual temperature of 15.9°C and an average annual precipitation of 1080.3 mm. The dry and wet seasons in the study area are distinct. May to October of every year is the rainy season, November to April is the dry season. The slag field belongs to the Nanpan River water system, and its secondary tributary (the Shadian River) flowing from southeast to northwest of the northeast side of the slag field (Song et al., 2015; Yu et al., 2020).
2.2 Collection and treatment of samples
87 topsoil samples at the depth of 0–30 cm were obtained around the slag field, mainly based different use type of soil, such as forest, grass land and cultivated land. Among these three types of soil, the sampling points of cultivated soil have been encrypted with the consideration for the importance of cultivated soil to human health (Figure 1). For each sampling point, five samples with a spacing of no more than 1 m were collected and mixed to obtain the final soil sample. After the soil samples were collected, the following steps was used to preparation: air-drying, grinding in a mortar, sieving through a 200-mesh nylon sieve, and ensuring thorough homogenization (Villarruel et al., 2024). Then the prepared samples were carefully transferred into polyethylene bags and stored at room temperature for subsequent physicochemical analysis. As for the determination of heavy metals, 1.00 g of each soil sample was putted into a digestion tube and then subjected to digestion in a graphite digester using nitric acid (5 ml HNO3), hydrofluoric acid (2 mL HF), and perchloric acid (1 mL HClO4) at 180°C. After dilution with 5% nitric acid, the concentrations of Pb, As, Cu, Zn, Ni, and Cr in the soil were determined using inductively coupled plasma optical emission spectrometer (ICP-OES, PerkinElmer Optima 3,300) (Song et al., 2024). The pH of soil samples was measured using a calibrated multiparameter water quality analyzer (Sartorius pH-Meter PB-10) with solid-to-water ratio of 1:5 (w/v) (Chen et al., 2019). The levels of available phosphorus (AP), available potassium (AK), ammonium nitrogen (NH4+-N), nitrate nitrogen (NO3−-N) of soil sample was determined by high precision soil nutrient detector (HM-TYD, Shandong Hengmei Electronic Technology Co., Ltd., Linyi, China), according to the user manual (Meng et al., 2022).
2.3 Soil heavy metal pollution evaluation method
The single-factor pollution index (Bouida et al., 2024), Nemerow comprehensive index (Enjavinejad et al., 2024), and potential ecological hazard index (Bouida et al., 2024) were employed to assess the level of heavy metal pollution using the following mathematical formulas (Zhou et al., 2024).
In this formula,
In the formula,
In the formula,
2.4 Statistical method
2.4.1 Inverse distance weighted (IDW)
The methods described above was used to measure pollution of heavy metal at each individual site. However, this method does not reflect the spatial distribution characteristics and spatial proximity effect. Therefore, in this report, we adopted IDW interpolation algorithm to reflect the degree of pollution of heavy metals, and aims to establish a theoretical foundation for the spatial classification and categorization of heavy metal pollution.
2.4.2 Correlation analysis
The correlation between heavy metal contents in the topsoil and the physicochemical properties of soil was analyzed by Pearson correlation analysis. Statistical Package for the Social Sciences (SPSS) version 25.0 was employed to realize this analysis (Kou et al., 2022).
2.5 Positive matrix factorization (PMF)
US-EPA PMF 5.0 program was used for PMF model. The original matrix Eik was decomposed into matrices Aij, Bjk and a residual matrix Cik with the following formula (Zhang et al., 2024):
In the formula 5, Eik represents the ith heavy mental measured in the kth sample, Aik represents the ith heavy mental from the kth source, Bjk is the concentration of heavy mental from the kth source that contributes to the jth sample, and Cik is the random error. The original matrix was decomposed by PMF.
In the formula 6, σik indicates that the uncertainty of Eik. The uncertainty was calculated with formula 7 when the content of heavy metals was smaller than or equal to the corresponding detection limit (MDL) of the merhod or formula 8 when the content of heavy metals was higher than the corresponding MDL.
In the formula 7, 8, σ symbolizes the relative standard deviation, and c symbolizes the content of elements.
3 Results and discussion
3.1 Physicochemical properties and content of soil heavy metals
The descriptive statistics of the basic parameters in the soils and heavy metal concentrations were presented in Table 1. The results demonstrated that the concentrations of NH4+-N, NO3−-N, AP and AK within the surface soil of the study area were 7.68–289.90 mg kg-1, 4.50–298.50 mg kg-1, 4.37–405.40 mg kg-1, and 58.90–1148.00 mg kg-1, respectively. In addition, the pH ranged from 5.17 to 8.69. The average contents of Pb, As, Cu, Zn, Ni and Cr were 247.82, 204.36, 104.83, 411.38, 29.73 and 67.53 mg kg-1, respectively. These mean contents of heavy metals were higher than the soil background values of Yunnan (Xu et al., 2014; Hu et al., 2020). With the exception of the Ni element, the concentrations of the remaining five heavy metals surpassed their respective background levels in study site. Especially, As element (with the highest accumulation effect) is 11.1l times of the background level, and the remaining four elements are 1.04–6.10 times of the background level in Yunnan. Meanwhile, the coefficient of variation (CV, the ratio of standard deviation to average) of these heavy metals were very high, especially Pb, Ni and Cr exceeding 100%, which indicated that these heavy metals might be influenced by human activities (Wang S. et al., 2019). Furthermore, the type of land use also has an important influence on heavy metal contents and basic physicochemical properties. It was found that the average contents of Cu, Zn, Ni and Cr were always higher at forest than grassland and cultivated land, with 155.62, 490.96, 76.16 and 107.18 mg kg-1, respectively, which may be due to the lower pH of forest soil.
3.2 Spatial distribution of heavy metals
To further analyze the spatial distribution in the whole area, spatial superposition and IDW were used to perform comprehensive pollution evaluation and spatial interpolation analysis on the soil heavy metal levels, and the results were shown in Figure 2. It demonstrated that the contents of all heavy metals were relatively high in the northwest near the slag field and the cultivated area (southeast), while its content is relatively low in the rest areas. The areas with high content of Pb, As, Cu, Zn, Ni, and Cr were all close to the slag field and were greatly influenced by human activities. For Pb, As, Cu, and Zn elements far exceeding the soil background value (Table 1), the regions with elevated concentration of heavy metals were located in the central west, southwest and northwest of the slag field, and the areas with low concentration were mainly in the northeast and southeast of slag field. As for Ni and Cr, their concentration was relatively low, except for some individual points. Meanwhile, the southeast (high content of Pb), the southwest (high content of As), the south (high content of Cu), the northeast (high content of Zn), the southwest and east-central (high content of Cr) areas were all cultivated land with relatively high pollution. The areas with low content of soil heavy metals were close to woodland and grassland with relatively low pollution. In general, the contents of heavy metals are more likely influenced by human activities and natural factors, when the vegetation coverage in the soil is low (Kabas et al., 2012). This finding indicated the lowest heavy metal concentrations in woodland soils, suggesting that trees may impede the migration and deposition of heavy metals (Li et al., 2017).
3.3 Environmental risk assessment of heavy metals
As shown in Figure 3, the proportion of heavy metal pollution levels was first determined based on the single-factor pollution index (Pi) and Nemerow comprehensive index (PN). The Pi results demonstrated that most sites were contaminated by Pb, As, Cu and Zn, except for the safe sites of Ni and Cr accounted for 98.85%. The sites of Pb, Cu and Zn with mild pollution was dominated, accounting for 42.53%, 51.72%, and 63.22% of the total sampling sites. However, sites with severe pollution of As accounted for 70.11% of the total sampling sites. These results indicated that the pollution of Pb, Cu and Zn is relatively light, and the accumulation of As is a great threat to soil quality. The order of potential ecological hazard level of individual heavy metal was As > Pb > Cu=Ni > Zn=Cr based on their corresponding occupied percentages of different risk levels for all samples. The PN results showed that the sites in the heavy pollution level account for 63.22%, those in the moderate pollution level account for 26.09%, those in the mild pollution level accounted for 16.09%, indicating that most of the soil in the entire study area was still relatively unsafe.
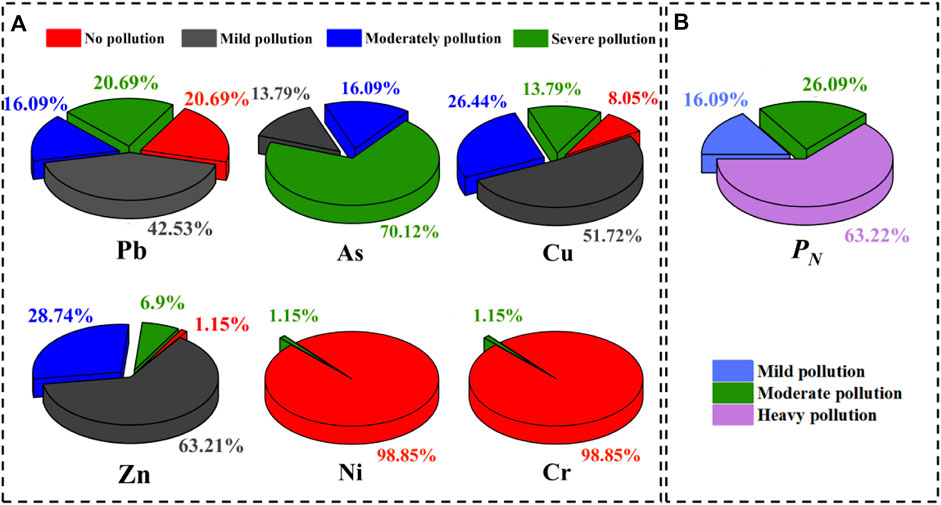
Figure 3. Percentages of sites at different pollution levels based on the (A) single-factor pollution index (Pi) and (B) Nemerow comprehensive index (PN).
To further evaluate the ecological risk of heavy metals in the study area, the index of
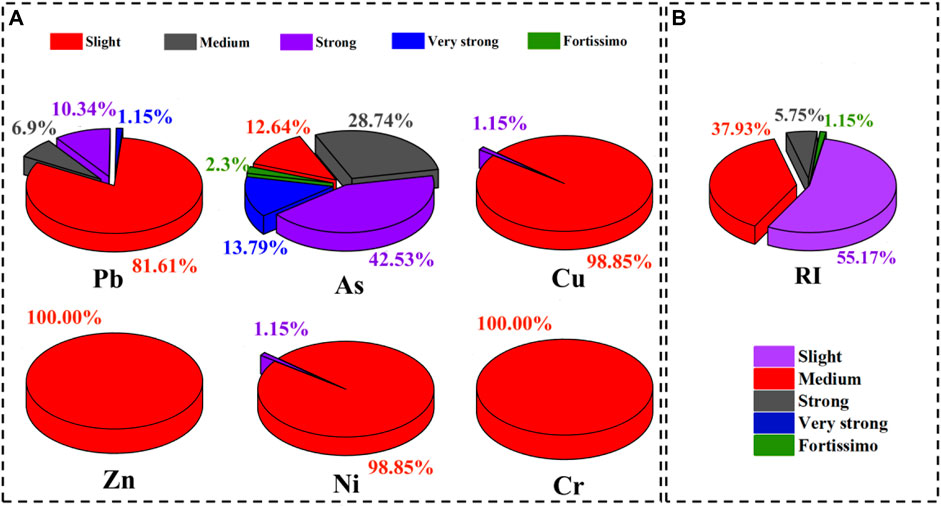
Figure 4. Percentages of sites at different potential ecological risks based on (A)
3.4 Source and pollution degree of heavy metals in soil
3.4.1 Pearson correlation analysis
The interrelationship among heavy metal levels within 87 topsoil samples obtained from the designated study area was analyzed. The obtained findings demonstrated a significant correlation among the concentrations of diverse heavy metals, as illustrated in Figure 5. Among the three soil utilization types, the strong correlations among Pb-As-Cu-Zn-Ni-Cr were observed in grassland soil. Especially, the Pearson correlation coefficient for Pb-As in grassland soil was greater than 0.5, indicating a significant correlation. In cultivated soil, the Pearson correlation coefficients for As-Cu-Zn and Pb-Cr were greater than 0.5, indicating a significant correlation. In the grassland soil, a significant positive correlation between As and NH4+-N was observed. A significant positive correlations between Cu and NH4+-N, and between Zn and AP were also observed. In cultivated soil, a significant positive correlation between As and AP was found. The element copper (Cu) exhibits a notable positive correlation with ammonium nitrogen (NH4+-N), whereas nickel (Ni) demonstrates a significant negative correlation with nitrate nitrogen (NO3−) and available potassium (AK).
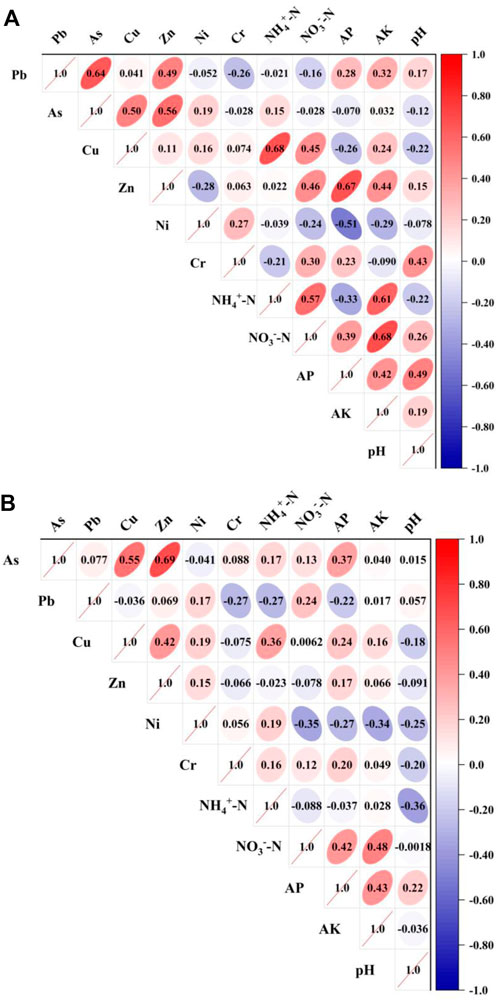
Figure 5. Correlation between physical and chemical indexes of (A) grassland and (B) cultivated soil and heavy metals.
3.4.2 Source analysis in PMF model
The contribution rates of the six soil heavy metals from the four sources within the study area were analyzed using the PMF model (Figure 6). The findings indicated that the Q value reached its minimum, and the residuals ranged from −3 to three when utilizing four factors. The correlation between the measured and predicted values was satisfactory. As shown in Figure 6, Ni accounted for the highest proportion in factor 1 (Ni dominated heavy metal pollution source which is simultaneously impacted by natural processes and human activities), with a contribution rate of 85.6%. Ni is an iron-philic element in the supergenous geochemical process. The average Ni concentration within the study area falls below the background level. Ni content in the soil is most likely dominated by the soil-forming process. However, the CV of Ni is greater than 1, which is highly variable, indicating that the accumulation of Ni in soil is also affected by human activities in addition to natural factors (Kandalai and Patel, 2024). Studies have shown that point source pollution, e.g., soil parent material and small manufacturers are the cause of soil Ni accumulation, and the impact of point sources on Ni is higher than that of soil parent material (Xu et al., 2014). Therefore, factor 1 can be treated as a mixture of natural sources and industrial activities.
Pb, Zn, and As are the main loading elements of factor 2 (Pb-Zn-As mixed heavy metal pollution source which is mainly impacted by human activities), and the contribution rates are 75.8%, 9.8%, and 9.4%, respectively. As shown in Table 1, CV of Pb is greater than 1, indicating a strong variability and that its source is mainly influenced by human activities. The concentrations of Pb, Zn, and As were higher in the areas closer to the non-ferrous metal slag field (Figure 2). The transportation in the study area is well developed, and the scattering of traffic pollutants into the soil can increase the content of Pb, Zn, and As. Previous study has demonstrated that the dust on both sides of dense roads usually contains high concentrations of Zn, which then enter the soil through atmospheric deposition (Yang et al., 2019). In addition, the exhaust emissions and tire wear and tear in transportation activities also aggravate the enrichment of soil Pb, Zn, and As (Tshisikhawe and Ngole-Jeme, 2024). Therefore, the source factor 2 is predicted to be a mixed source of transportation and industrial activities.
The primary loading constituents of factor 3 were identified as arsenic (As), zinc (Zn), and copper (Cu) (As-Zn-Cu mixed heavy metal pollution source which is mainly impacted by human activities), exhibiting contribution rates of 70.9%, 48.6%, and 46.8%, correspondingly. As was mainly present in the slag affected area and cultivated land (Figure 2), and a significant positive correlation between As and AP was found (Figure 5). It has already demonstrated that phosphate fertilizer contains a large amount of As, and the application of fertilizer and pesticide increased the As content in the soil (Jiao et al., 2012). High Cu concentrations were predominantly observed in close proximity to both the slag field and the planting area, with sites near the mining area exhibiting higher Cu levels compared to other locations. The field survey revealed the cultivation of fruit trees such as pomegranate and dragon fruit, along with crops including corn, tomato, and rice, in the study area. Local farmers use herbicides and pesticides to increase the production and avoid pests. The production of pesticides necessitates the extensive utilization of copper sulfate (CuSO4) as an insecticidal agent for the protection of fruit trees and crops, subsequently leading to the accumulation of copper (Cu) in the topsoil within agricultural regions. Cu and Zn are often added to livestock feed as trace element additives to prevent disease and promote growth. However, 95% of Cu and Zn are excreted from the body through feces, increasing the contents of Cu and Zn in soil. In addition, the notable coefficient of variation (CV) values observed for arsenic (As), copper (Cu), and zinc (Zn) (Table 1) suggest the potential existence of point source pollution, wherein residues of As, Cu, and Zn elements have the capability to accumulate on the surface during mining operations. The long-term wind erosion and atmospheric deposition in the slag field led to the As, Cu, and Zn pollution in the study area, which are diffused to the surrounding areas under the action of rainwater leaching, and then migrated to the soil, resulting in the increase of As, Cu, and Zn content in the surrounding soil of the mining area (Castillo et al., 2013; Gutiérrez et al., 2016). Therefore, factor 3 can be regarded as a source of mixed agricultural and industrial activities.
Cr element occupies a large proportion in factor 4 (Cr dominated heavy metal pollution source which is mainly impacted by natural processes), and its contribution rate is 80.8%. The average content of Cr was roughly equal to the background concentration (Table 1). The contribution rate of Zn, CU, Pb and Ni was 20.3%, 14.5%, 13.7%, and 13.0%, respectively, which can be defined as natural sources, e.g., parent material and soil-forming process. Previous study has demonstrated that soil Cr content mainly depends on soil parent material and is less influenced by human activities (Jiang et al., 2017). In the multivariate statistical analysis of soil heavy metal sources, other heavy metals in the factor grouping of Cr element are considered to be affected by natural factors. Because of the typical karst landforms in the study, the accumulation and pollution of soil heavy metal elements, e.g., Cr, Zn, and Cu, are more easily affected by natural conditions including topography (elevation and slope), lithology, geological background, and pedogenesis (Jiang et al., 2020; Zhao et al., 2021). Previous investigations have substantiated substantial elevations in chromium (Cr) and nickel (Ni) concentrations within rocks located in karst regions when compared to other rock formations. Additionally, it has been observed that the contents of Cr, Cu, and Ni within soil exhibit a discernible correlation with the parent rock associated with soil formation. (Facchinelli et al., 2001; Mico et al., 2006). Therefore, the content of Cr, Cu, and Ni in soil is closely related to the soil-forming parent material and the process of soil formation.
4 Conclusion
This study proposed integrated research framework for heavy metal pollution risk assessment and pollution source analysis, which was applied to non-ferrous metal slag field and its affected area located in the karst mountain region. It pointed out that the mean contents of Pb, As, Cu, Zn, Ni and Cr in the topsoil of the study site were 247.82, 204.36, 104.83, 411.38, 29.73 and 67.59 mg kg-1, respectively. With the exception of the Ni element, the concentrations of the remaining five heavy metals surpassed their respective background levels in study site. Especially, As element (with the highest accumulation effect) is 11.1l times of the background level, and the remaining four elements are 1.04–6.10 times of the background level in Yunnan.
The single factor pollution index showed that the pollution degree of Pb, Cu and Zn was light, and the accumulation of As was a great threat to soil quality. Furthermore, there were almost no clean and slight pollution samples in the study area, and the percentages of sites at mild pollution, moderate pollution and heavy pollution levels were 16.09%, 26.09% and 63.22%, respectively. Additionally, the potential ecological hazard degree of single element heavy metals was analyzed. The order of potential ecological hazard degree of soil in the survey area was As > Pb > Cu = Ni > Zn = Cr.
The spatial dispersion of heavy metals within the study region is impacted by both natural phenomena and human activities. The areas with severe pollution were the mining area in the northwest and planting sites in the east, middle and south of the study area. The main contribution sources of Ni were the mixed sources of natural parent material and industrial activities. The heavy metal element largely contributed by industrial activities and transportation is Pb. The main sources of metals As, Cu and Zn are the integrated sources of agricultural and industrial activities. The main source of Cr is natural source. Therefore, these useful results can guide the heavy metal pollution control and restoration of study site and this research work can also be used to other similar metal slag field and its affected area.
Data availability statement
The original contributions presented in the study are included in the article/Supplementary Material, further inquiries can be directed to the corresponding author.
Author contributions
LJ: Data curation, Methodology, Visualization, Writing–original draft, Writing–review and editing. HL: Data curation, Methodology, Writing–review and editing. MF: Data curation, Methodology, Writing–review and editing. SG: Data curation, Methodology, Writing–review and editing. TY: Data curation, Methodology, Writing–review and editing. MW: Writing–review and editing. MS: Writing–review and editing. SC: Supervision, Writing–original draft, Writing–review and editing. ZW: Writing–review and editing. KF: Writing–review and editing.
Funding
The author(s) declare that financial support was received for the research, authorship, and/or publication of this article. The Sichuan Science and Technology Program (No. 2023YFS0493) funding was obtained for this study.
Conflict of interest
The authors declare that the research was conducted in the absence of any commercial or financial relationships that could be construed as a potential conflict of interest.
Publisher’s note
All claims expressed in this article are solely those of the authors and do not necessarily represent those of their affiliated organizations, or those of the publisher, the editors and the reviewers. Any product that may be evaluated in this article, or claim that may be made by its manufacturer, is not guaranteed or endorsed by the publisher.
References
Bouida, L., Ya’acob, F., Jaafar, M. H., Abdullah, H., and Fadhullah, W. (2024). Human and ecological risk assessment of soil and plants metal contamination in a tropical municipal solid waste dumping site. Soil Sediment. Contam., 1–37. doi:10.1080/15320383.2024.2329951
Burges, A., Epelde, L., and Garbisu, C. (2015). Impact of repeated single-metal and multi-metal pollution events on soil quality. Chemosphere 120, 8–15. doi:10.1016/j.chemosphere.2014.05.037
Cao, J., Li, C., Zhang, L., Wu, Q., and Lv, J. (2020). Source apportionment of potentially toxic elements in soils using APCS/MLR, PMF and geostatistics in a typical industrial and mining city in Eastern China. PLoS One 15 (9), e0238513. doi:10.1371/journal.pone.0238513
Castillo, S., De La Rosa, J. D., De La Campa, A. M. S., González-Castanedo, Y., Fernández-Caliani, J. C., Gonzalez, I., et al. (2013). Contribution of mine wastes to atmospheric metal deposition in the surrounding area of an abandoned heavily polluted mining district (Rio Tinto mines, Spain). Sci. Total Environ. 449, 363–372. doi:10.1016/j.scitotenv.2013.01.076
Chen, J., Wang, P., Wang, C., Wang, X., Miao, L., Liu, S., et al. (2019). Dam construction alters function and community composition of diazotrophs in riparian soils across an environmental gradient. Soil Biol. biochem. 132, 14–23. doi:10.1016/j.soilbio.2019.01.020
Enjavinejad, S. M., Zahedifar, M., Moosavi, A. A., and Khosravani, P. (2024). Integrated application of multiple indicators and geographic information system-based approaches for comprehensive assessment of environmental impacts of toxic metals-contaminated agricultural soils and vegetables. Sci. Total Environ. 926, 171747–747. doi:10.1016/j.scitotenv.2024.171747
Facchinelli, A., Sacchi, E., and Mallen, L. (2001). Multivariate statistical and GIS-based approach to identify heavy metal sources in soils. Environ. Pollut. 114 (3), 313–324. doi:10.1016/S0269-7491(00)00243-8
Gutiérrez, M., Mickus, K., and Camacho, L. M. (2016). Abandoned Pb-Zn mining wastes and their mobility as proxy to toxicity: a review. Sci. Total Environ. 565, 392–400. doi:10.1016/j.scitotenv.2016.04.143
Hasan, A. B., Reza, A. H. M. S., Siddique, M. A., Akbor, M. A., Nahar, A., Hasan, M., et al. (2024). Origin, spatial distribution, sediment contamination, ecological and health risk evaluation of trace metals in sediments of ship breaking area of Bangladesh. J. Hazard. Mater. 465, 133214. doi:10.1016/j.jhazmat.2023.133214
Hossain, M. A., Ali, N. M., Islam, M. S., and Hossain, H. Z. (2015). Spatial distribution and source apportionment of heavy metals in soils of Gebeng industrial city, Malaysia. Environ. Earth Sci. 73, 115–126. doi:10.1007/s12665-014-3398-z
Hosseinniaee, S., Jafari, M., Tavili, A., Zare, S., and Cappai, G. (2023). Investigating metal pollution in the food chain surrounding a lead-zinc mine (Northwestern Iran); an evaluation of health risks to humans and animals. Environ. Monit. Assess. 195 (8), 946. doi:10.1007/s10661-023-11551-9
Hu, B. F., Shao, S., Ni, H., Fu, Z. Y., Hu, L. S., Zhou, Y., et al. (2020). Current status, spatial features, health risks, and potential driving factors of soil heavy metal pollution in China at province level. Environ. Pollut. 266, 114961. doi:10.1016/j.envpol.2020.114961
Huang, F., Wei, X. M., Zhu, T. B., Luo, Z. a. X., and Cao, J. H. (2021). Insights into distribution of soil available heavy metals in karst area and its influencing factors in guilin, southwest China. Forests 12, 609. doi:10.3390/f12050609
Jiang, C. F., Gao, X. B., Hou, B. J., Zhang, S. T., Zhang, J. Y., Li, C. C., et al. (2020). Occurrence and environmental impact of coal mine goaf water in karst areas in China. J. Clean. Prod. 275, 123813. doi:10.1016/j.jclepro.2020.123813
Jiang, Y. X., Chao, S. H., Liu, J. W., Yang, Y., Chen, Y. J., Zhang, A. C., et al. (2017). Source apportionment and health risk assessment of heavy metals in soil for a township in Jiangsu Province, China. Chemosphere 168, 1658–1668. doi:10.1016/j.Chemosphere.2016.11.088
Jiao, W. T., Chen, W. P., Chang, A. C., and Page, A. L. (2012). Environmental risks of trace elements associated with long-term phosphate fertilizers applications: a review. Environ. Pollut. 168, 44–53. doi:10.1016/j.envpol.2012.03.052
Kabas, S., Faz, A., Acosta, J. A., Zornoza, R., Martínez-Martínez, S., Carmona, D. M., et al. (2012). Effect of marble waste and pig slurry on the growth of native vegetation and heavy metal mobility in a mine tailing pond. J. Geol. Explor 123, 69–76. doi:10.1016/j.gexplo.2012.07.008
Kandalai, S., and Patel, A. (2024). Geomechanical and microstructural behaviour of expansive soil stabilized with red mud and GGBS: an experimental investigation. Arab. J. Sci.Eng. 15. doi:10.1007/s13369-024-09171-7
Kou, Y. Y., Zhao, Q., Yuan, D. H., and Ren, X. H. (2022). Evaluation of GLDA-acid on sludge treatment effect and seed germination analysis. J. Environ. Manage. 320, 115958. doi:10.1016/j.jenvman.2022.115958
Li, X., Yang, H., Zhang, C., Zeng, G. M., Liu, Y. G., Xu, W. H., et al. (2017). Spatial distribution and transport characteristics of heavy metals around an antimony mine area in central China. Chemosphere 170, 17–24. doi:10.1016/j.chemosphere.2016.12.011
Li, Y., Xu, Z., Ren, H., Wang, D., Wang, J., Wu, Z., et al. (2022). Spatial distribution and source apportionment of heavy metals in the Topsoil of Weifang City, East China. Front. Environ. Sci. 10, 893938. doi:10.3389/fenvs.2022.893938
Liu, D. Q., Liu, Z. R., Wang, Y., and Zhou, L. M. (2023). Editorial: understanding heavy metal pollution and control in the environment around metal tailings. Front. Environ. Sci. 11. doi:10.3389/fenvs.2023.1168949
Meng, L. L., Srivastava, A., Kuča, K., and Wu, Q. S. (2022). Earthworm (Pheretima guillelmi)-mycorrhizal fungi (Funneliformis mosseae) association mediates rhizosphere responses in white clover. Appl. Soil Ecol. 172, 104371. doi:10.1016/j.apsoil.2021.104371
Mico, C., Recatala, L., Peris, M., and Sanchez, J. (2006). Assessing heavy metal sources in agricultural soils of an European Mediterranean area by multivariate analysis. Chemosphere 65 (5), 863–872. doi:10.1016/j.chemosphere.2006.03.016
Mohamed, E. S., Jalhoum, M. E. M., Hendawy, E., El-Adly, A. M., Nawar, S., Rebouh, N. Y., et al. (2024). Geospatial evaluation and bio-remediation of heavy metal-contaminated soils in arid zones. Front. Environ. Sci. 12. doi:10.3389/fenvs.2024.1381409
Nambiar, R., Shah, C., Kumar, J., Shrivastav, P. S., and Bhushan, R. (2020). Assessment of contaminants in the northwestern bay of bengal. Environ. Sci. Pollut. Res. 27 (27), 34090–34098. doi:10.1007/s11356-020-09576-5
Pathak, A. K., Kumar, R., Kumar, P., and Yadav, S. (2015). Sources apportionment and spatio-temporal changes in metal pollution in surface and sub-surface soils of a mixed type industrial area in India. J. Geol. Explor 159, 169–177. doi:10.1016/j.gexplo.2015.09.004
Proshad, R., and Idris, A. M. (2023). Evaluation of heavy metals contamination in cereals, vegetables and fruits with probabilistic health hazard in a highly polluted megacity. Environ. Sci. Pollut. Res. 30 (32), 79525–79550. doi:10.1007/s11356-023-27977-0
Ren, C. B., Zhang, Q. Q., Wang, H. W., and Wang, Y. (2021). Characteristics and source apportionment of polycyclic aromatic hydrocarbons of groundwater in Hutuo River alluvial-pluvial fan, China, based on PMF model. Environ. Sci. Pollut. Res. 28 (8), 9647–9656. doi:10.1007/s11356-020-11485-6
Siddiqui, A. U., Jain, M. K., and Masto, R. E. (2020). Pollution evaluation, spatial distribution, and source apportionment of trace metals around coal mines soil: the case study of eastern India. Environ. Sci. Pollut. Res. 27 (10), 10822–10834. doi:10.1007/s11356-019-06915-z
Song, T., Tu, W., Chen, S., Fan, M., Jia, L., Wang, B., et al. (2024). Relationships between high-concentration toxic metals in sediment and evolution of microbial community structure and carbon–nitrogen metabolism functions under long-term stress perspective. Environ. Sci. Pollut. Res. 31, 29763–29776. doi:10.1007/s11356-024-33150-y
Song, X. B., Li, G. X., Fang, X. J., Shao, J. P., He, L. P., and Bi, B. (2015). “Heavy metal contents and accumulation characteristic of dominant plants in tin mining wasteland of Gejiu city, yunnan, China,” in Proc. 5th Int. Conf. Adv. Des. and Manuf. Eng. Editor Z. Jiang (Wollongong, Australia: University of Wollongong), 801–808. doi:10.2991/icadme-15.2015.156
Sowers, T. D., Blackmon, M. D., Wilkin, R. T., Rovero, M., Bone, S. E., Jerden, M. L., et al. (2024). Lead speciation, bioaccessibility, and sources for a contaminated subset of house dust and soils collected from similar United States residences. Environ. Sci. Technol. 58 (21), 9339–9349. doi:10.1021/acs.est.4c01594
Tshisikhawe, C. S., and Ngole-Jeme, V. M. (2024). Temperature induced changes on heavy metal geochemical partitioning and mobility in contaminated and uncontaminated soils. Environ. Pollut. Bioavailab. 36. doi:10.1080/26395940.2024.2317747
Villarruel, C. M., Figueroa, L. A., and Ranville, J. F. (2024). Quantification of bioaccessible and environmentally relevant trace metals in structure ash from a wildland-urban interface fire. Environ. Sci. Technol. 58 (5), 2502–2513. doi:10.1021/acs.est.3c08446
Wang, G., Cao, F., Shan, B., Meng, M., Wang, W., and Sun, R. Y. (2019a). Sources and assessment of mercury and other heavy metal contamination in soils surrounding the wuda underground coal fire area, inner Mongolia, China. Bull. Environ. Contam. Toxicol. 103 (6), 828–833. doi:10.1007/s00128-019-02734-7
Wang, S., Cai, L.-M., Wen, H.-H., Luo, J., Wang, Q.-S., and Liu, X. (2019b). Spatial distribution and source apportionment of heavy metals in soil from a typical county-level city of Guangdong Province, China. Sci. Total Environ. 655, 92–101. doi:10.1016/j.scitotenv.2018.11.244
Wang, Y. Z., Duan, X. J., and Wang, L. (2020). Spatial distribution and source analysis of heavy metals in soils influenced by industrial enterprise distribution: case study in Jiangsu Province. Sci. Total Environ. 710, 134953. doi:10.1016/j.scitotenv.2019.134953
Wang, Z. H., Liu, X. Y., and Qin, H. Y. (2019c). Bioconcentration and translocation of heavy metals in the soil-plants system in Machangqing copper mine, Yunnan Province, China. J. Geol. Explor 200, 159–166. doi:10.1016/j.gexplo.2019.02.005
Xu, X. H., Zhao, Y. C., Zhao, X. Y., Wang, Y. D., and Deng, W. J. (2014). Sources of heavy metal pollution in agricultural soils of a rapidly industrializing area in the Yangtze Delta of China. Ecotoxicol. Environ. Saf. 108, 161–167. doi:10.1016/j.ecoenv.2014.07.001
Yan, G. W., Gao, Y. J., Xue, K., Qi, Y. J., Fan, Y., Tian, X., et al. (2023). Toxicity mechanisms and remediation strategies for chromium exposure in the environment. Front. Environ. Sci. 11. doi:10.3389/fenvs.2023.1131204
Yang, H. Q., Huang, G. Z., Chen, C. W., and Chen, L. C. (2024). Hyperspectral imaging for mineral composition and weathering recognition of rock slope on the reservoir bank. Bull. Eng. Geol. Environ. 83, 193. doi:10.1007/s10064-024-03694-x
Yang, S. Y., He, M. J., Zhi, Y. Y., Chang, S. X., Gu, B. J., Liu, X. M., et al. (2019). An integrated analysis on source-exposure risk of heavy metals in agricultural soils near intense electronic waste recycling activities. Environ. Int. 133, 105239. doi:10.1016/j.envint.2019.105239
Yu, Y. H., Wang, J. L., Cheng, F., and Chen, Y. (2020). Soil moisture by remote sensing retrieval in the tropic of cancer of yunnan province. Pol. J. Environ. Stud. 29 (2), 1981–1993. doi:10.15244/pjoes/110203
Zhang, C., Jing, D. J., Wu, C. Z., Li, S. J., Cheng, N. N., Li, W., et al. (2021). Integrating chemical Mass balance and the community multiscale air quality models for source identification and apportionment of PM 2.5. Process Saf. Environ. Prot. 149, 665–675. doi:10.1016/j.psep.2021.03.033
Zhang, X., Yang, H. H., and Cui, Z. J. (2018). Evaluation and analysis of soil migration and distribution characteristics of heavy metals in iron tailings. J. Clean. Prod. 172, 475–480. doi:10.1016/j.jclepro.2017.09.277
Zhang, Y. Q., Jiang, B., Gao, Z. J., Wang, M., Feng, J. G., Xia, L., et al. (2024). Health risk assessment of soil heavy metals in a typical mining town in north China based on Monte Carlo simulation coupled with Positive matrix factorization model. Environ. Res. 251, 118696. doi:10.1016/j.envres.2024.118696
Zhao, K. L., Fu, W. J., Qiu, Q. Z., Ye, Z. Q., Li, Y. F., Tunney, H., et al. (2019). Spatial patterns of potentially hazardous metals in paddy soils in a typical electrical waste dismantling area and their pollution characteristics. Geoderma 337, 453–462. doi:10.1016/j.geoderma.2018.10.004
Zhao, Y. L., Han, R., Cui, N., and And Guo, L. (2021). The impact of urbanization on ecosystem health in typical karst areas: a case study of liupanshui city, China. Int. J. Env. Res. Pub. He. 18, 93. doi:10.3390/ijerph18010093
Keywords: karst, heavy metals, spatial distribution, source analysis, ecological risk assessment
Citation: Jia L, Liang H, Fan M, Guo S, Yue T, Wang M, Su M, Chen S, Wang Z and Fu K (2024) Spatial distribution and source apportionment of soil heavy metals in the areas affected by non-ferrous metal slag field in southwest China. Front. Environ. Sci. 12:1407319. doi: 10.3389/fenvs.2024.1407319
Received: 26 March 2024; Accepted: 25 June 2024;
Published: 19 July 2024.
Edited by:
Rubén Forján, University of Oviedo, SpainReviewed by:
Chengyu Xie, Xiangtan University, ChinaAleksandra Wdowczyk, Wroclaw University of Environmental and Life Sciences, Poland
Copyright © 2024 Jia, Liang, Fan, Guo, Yue, Wang, Su, Chen, Wang and Fu. This is an open-access article distributed under the terms of the Creative Commons Attribution License (CC BY). The use, distribution or reproduction in other forums is permitted, provided the original author(s) and the copyright owner(s) are credited and that the original publication in this journal is cited, in accordance with accepted academic practice. No use, distribution or reproduction is permitted which does not comply with these terms.
*Correspondence: Shu Chen, Y3JpY2tfY2hlbkBob3RtYWlsLmNvbQ==