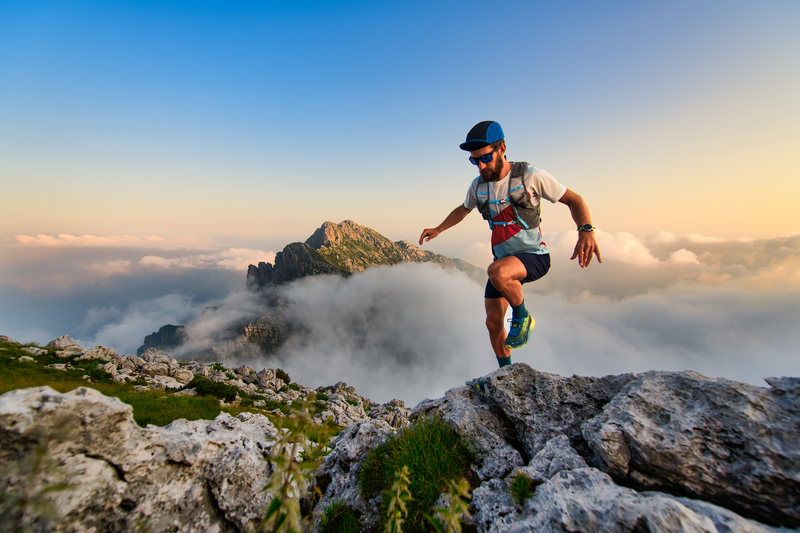
95% of researchers rate our articles as excellent or good
Learn more about the work of our research integrity team to safeguard the quality of each article we publish.
Find out more
ORIGINAL RESEARCH article
Front. Environ. Sci. , 03 January 2025
Sec. Environmental Economics and Management
Volume 12 - 2024 | https://doi.org/10.3389/fenvs.2024.1403246
Introduction: Investigating the relationship between green finance and carbon emission intensity is essential for understanding its role in China’s carbon reduction strategy.
Methods: This study employs the entropy weight method to measure the green finance development index across 30 Chinese provinces.Using double fixed-effects, mediation effect, and Spatial Durbin Models (SDM), the analysis reveals three key findings.
Results: Firstly, green finance directly reduces carbon emission intensity and indirectly does so by fostering technological innovation and optimizing energy structures, with more pronounced effects in western regions, highlighting regional disparities. Secondly, green finance shows a significant spatial spillover effect, reducing carbon emission intensity in neighboring provinces, beyond the impact on economically and geographically similar regions. Lastly, environmental regulation intensity positively influences carbon emission intensity in all models.
Discussion: These findings deepen our understanding of green finance’s role in China’s carbon reduction efforts and provide strong theoretical support for policy formulation, demonstrating its critical role in environmental governance and sustainable development.
Since the Industrial Revolution, the global economy has experienced unprecedented growth, but this has come at the cost of severe environmental problems. Notably, increased carbon emissions have led to global climate change, threatening human health and the sustainability of social development. To mitigate the environmental impact of carbon emissions and achieve green development goals, countries worldwide have set emission reduction and carbon neutrality targets. This requires substantial financial investments to support “green” transformations, such as green technologies, renewable energy, and energy-efficient infrastructure (Liza et al., 2024). According to the International Energy Agency (IEA), approximately USD 53 trillion in energy-related investments is needed by 2035 to meet the 2°C temperature limit stipulated by the Paris Agreement (IEA, 2014). In this context, green finance (GF) has garnered widespread attention as a crucial mechanism for promoting sustainable development.
GF, as defined by the Group of Twenty, an international forum of major economies, refers to “investment and financing activities that generate environmental benefits in support of sustainable development” (G20 GF Study Group, 2016). GF aims to achieve carbon reduction by prioritizing environmentally friendly and sustainable economic activities through various financial instruments and incentives, such as green credit, green bonds, and carbon emission trading schemes. Carbon emission intensity (CEI), defined as carbon emissions per unit of GDP, is considered a core indicator of regional green development and sustainability (Dong et al., 2018). GF facilitates investments in low-carbon projects, promotes innovation and application of low-carbon technologies (Cao et al., 2021), and incentivizes emission reduction behaviors (Qin et al., 2018), thereby reducing CEI.
Despite significant progress in green development, China’s CEI remains significantly higher than the global average and other major economies (IEA, 2021), posing substantial challenges in achieving its “dual carbon” goals. Consequently, the Chinese government places great emphasis on the role of GF, implementing a series of policies to promote carbon reduction. By 2020, China’s green bond issuance exceeded RMB 300 billion, accounting for over 15% of the global green bond market, making it the largest green bond issuer globally (Climate Bonds Initiative, 2020). Exploring the pathways through which GF reduces CEI in China holds significant theoretical and practical implications.
Existing studies indicate that GF can promote carbon reduction primarily through pathways such as fostering clean technology research and application (Chen et al., 2021), facilitating low-carbon energy transitions (Li et al., 2023), and generating spatial spillover effects of policies (Chen & Chen, 2021; Yang et al., 2022). However, the current literature predominantly focuses on the singular impacts of GF on CEI, lacking systematic analysis of its comprehensive impact mechanisms. There is particularly insufficient research and comparative analysis on the spatial spillover effects of GF policies. Additionally, the understanding of how GF influences CEI through technological innovation and energy structure adjustments remains incomplete.
Based on the above, this study proposes the following research questions: Through which pathways does GF impact CEI? How do the spatial spillover effects of GF transmit to other regions, and through which mechanisms? Are there regional differences in the effectiveness of GF, and what are the reasons for these differences?
To address these questions, this study utilizes panel data from 30 Chinese provinces from 2011 to 2020, employing mediation effect models and Spatial Durbin Models (SDM) to explore the pathways through which GF reduces CEI from both horizontal and spatial dimensions. The marginal contributions of this study include: Firstly, a comprehensive analysis of GF’s impact on CEI through heterogeneity analysis and mediation effect models, identifying regional differences and the mediating effects of technological innovation and energy structure. Secondly, the study employs economic geography and adjacency matrices to compare and analyze the results of different matrices and further examines the spillover effects in various regions. This research enriches the existing literature on GF and carbon emissions, providing theoretical and practical guidance for China’s transition to a low-carbon economy, and helping readers better understand the theoretical mechanisms of GF in achieving low-carbon economic transitions.
In examining the impact of GF on carbon emissions, early research primarily focused on exploring the fundamental theoretical relationship between GF and carbon emissions. This included defining GF, its characteristics, functions, and mechanisms of action. It was discovered that GF plays a crucial role in reducing carbon emissions. Cropper and Oates (1992) demonstrated that GF can effectively enhance the level of innovation in pollution-intensive industries. Jin and Mengqi (2011) found that green credit policies have achieved commendable results in energy conservation, emission reduction, and industrial structure optimization. Schmidt (2014) argued that the development of GF encourages the infusion of more “greener” social capital into the financial system, thereby motivating enterprises to innovate technologically and reduce carbon emissions.
As research progressed, scholars began to focus on specific pathways to emission reduction:
First, GF and its products can directly reduce carbon emissions and CEI. Meo and Abd Karim (2022) consider GF to be the best financial strategy for reducing carbon emissions. Wang and Wang (2022) found that green financial instruments are particularly effective in reducing CEI. Ren et al. (2020) confirmed that the development of GF and the use of non-fossil energy can significantly reduce CEI. Zakari (2022) discovered that GF promotes sustainable economic and environmental development, contributing to the achievement of sustainable development goals. Zhang (2023) emphasized the positive impact of establishing carbon emission trading pilots on the sustainable development of low-carbon regions.
Secondly, GF indirectly reduces carbon emissions and CEI by promoting “greenness.” In terms of green technology, Ji et al. (2022) demonstrated that market-based and autonomous regulatory mechanisms promote green technology progress, enhance energy efficiency, and reduce carbon emissions. Liu and Tobias (2023) argue that green financial tools, such as green credit, green bonds, and green investment funds, support green technology innovation in enterprises through financial support and market incentives, thus promoting low-carbon transformation. Chen et al. (2021) and Anquetin et al. (2022) found that carbon emission trading schemes (ETS) provide price signals that incentivize industries to adopt cleaner technologies and more efficient practices, significantly reducing emissions. Regarding energy structure, Yang and Wang (2023) stress the importance of GF in reducing fossil fuel use and promoting renewable energy. Liu et al. (2023) found that green investment promotes the low-carbon development of energy consumption structures in eastern China, supports emerging energy and environmental industries, and fosters sustainable development. Lin et al. (2023) observed that GF lowers CEI by influencing energy structure and energy efficiency. In terms of industrial structure, Gu et al. (2021) found that GF optimizes financial resource allocation, promotes industrial upgrading, and enhances resource and energy efficiency in industrial enterprises.
Thirdly, GF reduces carbon emissions and CEI of other regions through spatial effects. Chen and Chen (2021) confirmed that GF reduces carbon emissions within a province and its surrounding provinces. Guo et al. (2022) observed that GF significantly reduced carbon emissions in the Yangtze River Economic Belt but had no significant spillover effect on neighboring provinces. Liu et al. (2024) found that GF significantly lowers CEI within a province and affects neighboring provinces through spatial spillover effects. Yang et al. (2022) found that carbon emission trading schemes have significant spatial spillover effects, reducing CEI in surrounding areas through policy transmission mechanisms. At the urban level, Wang et al. (2019) found that urban CEI has significant “spatial spillover” effects, highly dependent on regional contexts.
In summary, existing literature indicates that GF has multiple pathways for carbon reduction. Early research primarily established the theoretical foundations of GF, emphasizing its significant role in promoting technological innovation and environmental benefits. Subsequent studies identified specific mechanisms, including direct financial support for green projects, indirect promotion of low-carbon production, and the expansion of GF benefits through spatial spillover effects. However, despite these significant findings, further exploration of the specific mechanisms of GF’s impact remains crucial. Building on this foundation, the present study will deeply analyze the mechanisms through which GF reduces CEI via different pathways and examine the regional differences and spatial spillover effects of GF in China.
GF plays a pivotal role in addressing climate change, primarily through two key mechanisms.
Firstly, GF, through its capital allocation, boosts low-carbon industries and prompts high-carbon enterprises to transform, thus lowering CEI. By offering loans, bonds, and other financial services, it provides a stable funding source for low-carbon industries, reducing their financing costs and supporting their growth and innovation (Gianfrate and Peri, 2019; Fan et al., 2021). Concurrently, it guides high-carbon industries towards clean energy and low-carbon technologies, enhancing their production processes (Liu et al., 2019).
Secondly, GF utilizes market mechanisms like carbon pricing, taxes, and trading to internalize the social cost of emissions, steering businesses and individuals towards energy-saving and emission-reducing practices. It establishes a structured carbon market, setting appropriate price signals for emissions and regulating them through taxes or subsidies (Ren et al., 2020). These mechanisms encourage more efficient, low-carbon choices (Tong et al., 2022), significantly reducing overall energy use and emissions, thereby decreasing CEI. Based on this, we derive the first hypothesis of this paper:
H1. The development of GF has a promotional effect on reducing CEI.
Furthermore, GF effectively promotes technological research and development (R&D) and utilization. On one hand, GF provides funding for the R&D of low-carbon technologies, sharing the costs and risks associated with their development and encouraging more entities to engage in these efforts (Carraro et al., 2012; Taghizadeh-Hesary and Yoshino, 2019). On the other hand, by restricting the financing avenues of high-carbon enterprises, GF compels them to engage in R&D or purchase new technologies to enhance production efficiency and reduce carbon emissions (Yu et al., 2021). As technology spillover and diffusion effects become evident, GF further enhances the production efficiency of the green environmental protection industry, leading to a continuous reduction in CEI.
GF also facilitates the adjustment of energy structures in three ways. First, it supports the R&D of renewable energy technologies, thereby increasing the supply of renewable energy (Xu and Li, 2020). Second, it guides energy consumers, especially high-carbon enterprises, to transition from traditional energy sources to renewable energy sources (Li and Umair, 2023), increasing market demand for renewable energy and further promoting the development of the renewable energy industry. Third, the demonstrative effect of GF enhances consumer awareness and preferences for renewable energy, lowering conversion costs and bolstering the market competitiveness of renewable energy. Optimizing the energy structure can significantly reduce CEI (Lee and Lee, 2022).
H2. GF can effectively reduce CEI through technological innovation and energy structure adjustment, promoting green transformation and sustainable development.
When a specific region successfully adopts GF strategies and significantly reduces CEI, other regions may be inspired by this success and adopt similar strategies and measures. This influence is not limited to the replication of strategies but may also include the diffusion of green technologies, talent exchange, and capital reallocation. For example, Li et al. (2022) found that the diffusion of green technologies significantly promotes technological progress in neighboring regions, reducing their carbon emissions. Additionally, the flow of high-quality talent plays a crucial role in the spatial spillover effects of GF, bringing new technologies, knowledge, management experience, and innovative thinking (Sun et al., 2022). At the same time, the reallocation of capital also facilitates the implementation of green projects in other regions, further reducing CEI (Wang et al., 2019; Guo et al., 2022). Building upon this, we present the third hypothesis:
H3. GF has spatial spillover effects on CEI.
This article constructs a dual fixed effect model to verify the impact of GF on CEI, as shown in Equation 1. The dual fixed effect model accounts for both individual and temporal effects, providing a robust analysis framework (Baltagi and Baltagi, 2008).
where the cgdpit is the CEI for province i in year t, gfdiit is the GF development index for province i in year t, α0 is the constant term, Xcontrol represents a series of control variables, α1 and α2 are the coefficients to be estimated, μi and αt are fix effects, εit is the random error term.
In order to further explore the impact pathways of GF on carbon intensity, an intermediate effect model is constructed on the basis of Hypothesis 2, as shown in Equations 2, 3. The mediation effect model helps to identify indirect effects and clarify the pathways through which GF impacts CEI (Baron & Kenny, 1986).
where Mjit is the mediating variable, j = 1,2, which represents technological innovation and energy structure adjustment. βj0 and ϒj0 are the constant terms, βj1,βj2,ϒj1,ϒj2 are coefficients to be estimated, respectively. Other notations are the same as above.
Based on Hypothesis 3, Spatial econometric models are essential for capturing spatial dependencies and spillover effects, as highlighted by LeSage and Pace (2009). To this end, we have constructed the Spatial Autoregressive Model (SAR), Spatial Error Model (SEM), and Spatial Durbin Model (SDM), as shown in Equations 4–6:
where ρ is the coefficient of the spatial lag term for CEI, wij is the selected weight matrix, wijcgdpit is the spatial lag term for the dependent variable of CEI, wijgfdiit is the spatial lag term for GF, wijXcontrol is the spatial lag term for control variables, α0 is the constant term, α1, α2, θ1, θ2, λ are the coefficients to be estimated. Other notations are the same as above. The appropriate model will be determined through testing.
This paper employs two matrices to validate the presence of spatial spillover effects. The economic geography matrix, denoted as W1, is constructed by multiplying the per capita GDP ratio and geographical distance ratio for each province (Li & Sun, 2023; Sun et al., 2023). W2 is the adjacency matrix, with adjacency equal to 1 and non-adjacency equal to 0, as commonly used in spatial econometric analyses to reflect the immediate spatial relationships between regions (Guo et al., 2022).
This study focuses on the GF Development Index (gfdi), for which there is no universally accepted measure, leading researchers to use proxies like green credit. Drawing from Ren et al. (2020) and Chen and Chen (2021), it considers four main indicators to form the gfdi: Green Credit, Green Securities, Green Insurance, and Green Investment, each further divided into tertiary measures:
Green credit allocation substantially influences investment in renewable energy sectors, facilitating capital infusion into energy conservation and environmental protection domains while concurrently diminishing carbon emissions (He et al., 2019). To quantify its impact, this study employs the ratio of interest expenditure in the six primary high-energy-consuming industries within each province as an inverse indicator.
Green securities serve as a barometer of corporate financing levels within capital markets. This research utilizes the market capitalization ratios of eco-friendly enterprises and the six key energy-intensive industries to assess capital support for green industries and the restraint exerted on high-energy sectors.
Green insurance, predominantly referring to corporate environmental liability insurance, faces a scarcity of comprehensive data since its mandatory implementation in China commenced only in 2013. Consequently, this paper adopts the rural insurance scale ratio and the payout rate as surrogate metrics to gauge the progression of green insurance.
Green investment reflects the financing trends in eco-friendly and energy-efficient sectors. This study uses public expenditure on energy conservation and environmental protection, and the ratio of investment in pollution control, to assess local governments’ financial support for green industry development and pollution efforts. To construct the gfdi, the entropy weight method is used, involving creating a comprehensive indicator framework, data preprocessing to standardize different data dimensions, weighting each indicator, and then aggregating them to form the final evaluation index. The resulting gfdi, with its entropy values and weights, is detailed in Table 1.
Through calculations, the Digital Financial Development Index for 30 provinces in China from 2011 to 2020 has been ascertained.
The dependent variable in this study is lnc, defined as the amount of carbon dioxide produced per unit of GDP. It is calculated as the ratio of carbon dioxide emissions to GDP, i.e., the carbon emissions of each province divided by its GDP. The meaning of this metric is the amount of carbon dioxide emitted for every additional unit of output in that region. A lower CEI indicates stronger carbon reduction effects in that area.
To estimate each province’s carbon emissions, two academic methods exist. One sums fossil fuel combustion emissions, while the other, from the IPCC, converts energy consumption into standard coal units, multiplied by emission coefficients for different energy types. The total emissions are then calculated. This study uses the IPCC method, focusing on the carbon emissions from China’s eight primary energy sources: coal, coke, crude oil, gasoline, kerosene, diesel, fuel oil, and natural gas.The calculation formula is given by Equation 7:
In the formula, e represents the type of primary energy, α represents the consumption of the eth type of primary energy, β represents the conversion coefficient of standard coal for the eth type of energy, and θ represents the carbon emission coefficient for the eth type of energy. The conversion coefficients for standard coal are sourced from the “China Energy Statistical Yearbook 2021,” and the carbon emission coefficients are taken from the “National Greenhouse Gas Emission Inventory Guidelines.” The calculation formula for CEI can thus be derived by Equation 8:
Then, logarithm cgdp to prevent the effects of heteroscedasticity.
The CEI, defined as the carbon emissions to GDP ratio, is shaped by various factors. This research identifies province-level control variables: population density, marketization degree, industrial structure, and environmental regulatory intensity, detailed as follows:
Population Density (lnur): Measured as the logarithm of the ratio of urban population to construction land, in tens of thousands per square kilometre. Higher values indicate increased urbanization, which is often associated with higher production and living activities, potentially raising carbon emissions. However, a denser population also suggests advanced production models and technological aggregation, which can reduce CEI. By logarithmically transforming this variable, we reduce data volatility and enhance the model’s robustness.
Marketization Degree (lnmal): Evaluated using the logarithmically transformed Fan Gang index, where higher values indicate greater marketization. Regions with high marketization tend to have more open and competitive environments, fostering business incentives for energy conservation and emission reduction. This accelerates technological advancement and the adoption of clean energy and low-carbon technologies. The logarithmic transformation helps smooth the data, reducing the impact of outliers.
Industrial Structure (inop): Measured as the ratio of tertiary to secondary industry added value in each province. A higher ratio indicates a shift towards the tertiary industry, which is typically more committed to environmental protection, innovation, and energy efficiency. This transition leads to high-value, low-emission production, thereby reducing regional CEI. Analyzing this ratio helps understand the impact of different industrial structures on carbon emissions.
Environmental Regulation Intensity (egi): Calculated as the ratio of industrial governance investment to secondary industry output, reflecting the stringency of pollution control. More stringent environmental regulations impose stricter constraints on high-energy, high-emission industries, compelling them to reduce energy use and emissions or shift to low-carbon sectors. This regulatory framework also promotes the adoption of low-carbon technology and clean energy, contributing to lower regional CEI. Analyzing this variable helps understand the impact of policy differences on CEI.
This article examines the mediation effect model with two mediators, high-tech innovation and energy consumption structure, to explore how GF aids in reducing carbon emissions and CEI through technological advancement and energy optimization. The measurement methodologies for these variables are as follows:
High-tech Innovation (hite): estimated by comparing the number of high-tech firms in each province to the national total. Higher ratios indicate better high-tech development in the region. Technological advancement can improve energy efficiency, manufacturing processes and equipment, and product added value. Conversely, it can help advance the creation and use of low-carbon and clean energy technologies, take the place of fossil fuels or lessen reliance on them, and significantly lower the intensity of carbon emissions.
Energy Consumption Structure (es): province-specific coal usage to total primary energy consumption. The structure of energy consumption can have a direct impact on greenhouse gas emissions, and locations with greater ratios would have higher CEI.
All the variables mentioned above are presented in Table 2.
This paper selects relevant data from 30 provinces (excluding Xizang Autonomous Region) in mainland China from 2011 to 2020, using statistical yearbooks such as China Energy Statistical Yearbook, China Insurance Yearbook, China Industrial Statistical Yearbook, China Financial Statistical Yearbook, China High tech Industrial Statistical Yearbook, provincial statistical yearbooks, provincial statistical bulletins, wind database, China market database and so on. The data were not processed except for taking the logarithm of some variables.
As shown in Table 3, we adopted a stepwise strategy of adding control variables to analyze the impact of each variable on the regression results. In assessing the impact of gfdi on lnc, Model (1) presents the regression results without control variables, showing a coefficient of −4.429 between gfdi and lnc at the 1% significance level, indicating that GF can significantly reduce CEI. Models (2) to (5) sequentially add control variables such as population density, marketization, industrial structure, and environmental regulation. As these variables are added, the absolute value of the gfdi-lnc coefficient gradually decreases but remains significantly negative at the 1% level. In column (5), the coefficient of gfdi-lnc is −2.584, indicating that for each 1 percentage point increase in gfdi, lnc decreases by 2.584 percentage points. This reflects the positive role of GF in reducing CEI and verifies Hypothesis 1.
In relation to the control variables, their coefficients exhibit significant impacts on CEI, with each being significant at the 1% level. The coefficient of lnur is negative, indicating that urbanization potentially enhances production efficiency, leading to a subsequent reduction in CEI. The lnmal exhibits a negative relationship with CEI, suggesting that as market mechanisms become more prevalent, firms may prioritize operational efficiency and innovation, culminating in the adoption of environmentally sustainable technologies and methodologies. The coefficient associated with inop is also negative, signifying that the ascendance of the tertiary sector is inversely related to CEI, albeit to a lesser extent. The egi in Model (5) is positively correlated with lnc, highlighting the profound influence of regulatory policies. Nonetheless, short-term technological and financial limitations might transiently amplify CEI.
This article employs two ways to assess the robustness of the regression results. One approach entails substituting the dependent variable and replacing the measure of CEI with per capita carbon emissions (rc) for the purpose of conducting a fundamental regression analysis. One alternative approach involves substituting the explanatory variable with its first-order lag term. The test results are presented in Table 4.
From an empirical perspective, the results elucidate that in Model (1) and (2), when the metric of CEI is supplanted by per capita carbon emissions, the association between the GF Development Index and per capita carbon emissions persists as statistically significant, exhibiting a negative correlation at the 1% significance threshold. Excluding the marketization index, which manifested as statistically non-significant, the other control variables maintained analogous significance levels and directionalities as discerned in the preliminary benchmark regression analyses. Transitioning to Model (3) and (4), the deployment of the gfdi as the predominant alternative explanatory variable consistently mirrors the significance levels and directionalities of coefficients observed in the antecedent benchmark regression analyses. Such congruence in findings underscores that the robustness checks corroborate the veracity of the initial benchmark regression outcomes.
In light of the preceding analysis, it is evident that the dispersion of GF development in China is heterogeneous. The eastern region, especially the southeastern coastal zones, exhibits a more advanced level of GF development compared to the central and western regions. Consequently, to elucidate the direct nexus between GF and CEI, it becomes essential to scrutinize the regional disparities in the impact of GF on CEI. This study classifies China’s provinces into three distinct regions: eastern, central, and western, adhering to the regional economic categorization delineated by the China Development and Reform Commission in 2000. Following this classification, a regression analysis is undertaken, culminating in Table 5.
Models (1), (3), and (5) present regression outcomes devoid of additional control variables. Each underscores that the GF development exerts a negative influence on CEI, achieving statistical significance at the 1% level. The absolute magnitude of the coefficient elucidates that the efficacy of GF in attenuating carbon emissions varies across the central, western, and eastern regions.
In the regression configurations delineated in Models (2), (4), and (6), ancillary control variables are incorporated. The results consistently highlight the directional influence of the gfdi across distinct regions, albeit with discernible variations in the magnitude of its impact. Specifically, the regression coefficients for the eastern, central, and western regions are enumerated as −0.814, −2.631, and −5.374, respectively. Such delineations accentuate the preeminent role of gfdi in the western region, followed by the central region, with the eastern region exhibiting the most subdued effect. These regional variances can be attributed to their inherent characteristics. The western region, marked by its heightened CEI and nascent economic and technological evolution, offers a broader scope for carbon emission curtailment via GF endeavors. Additionally, the western region’s abundant natural resources and propitious geographical attributes inherently enhance the potential for sustainable energy ventures, including solar and wind energy. In contrast, the eastern region, characterized by its sophisticated economic and technological milieu, has already embarked on a significant green transition. Thus, the incremental benefits from further emission abatement initiatives might not be as substantial as those in the western region.
Drawing insights from the control variables, both lnur and the degree of lnmal manifest a salient negative correlation with lnc. Remarkably, the influence of lnur is most accentuated in the western region. This can be ascribed to the region’s embryonic urbanization phase and its accelerated urban developmental trajectory. Given the western region’s comparatively diminutive urban populace and sparse urban centers, an augmentation in urban population density signifies profound transformations in economic, energy, and industrial paradigms, as well as societal behaviors. In a condensed timeframe, these metamorphoses are anticipated to culminate in a decrement in CEI. Pertaining to marketization, the lnmal exhibits a gradient, receding from the eastern to the western regions. The eastern region, endowed with a more evolved market infrastructure, galvanizes entities and individuals to champion technological advancements and energy thriftiness, thereby diminishing CEI. Conversely, the incipient phase of the market framework in the western region circumscribes its prospective influence on CEI.
Considering the direct effects, the mediating roles of high-tech level and energy structure adjustment are separately examined to ascertain their presence. The outcomes of this examination are presented in Table 6.
Models (1) and (2) explore the mediating role of technological innovation in the carbon reduction promoted by GF. The results of Model (1) show that the coefficient of gfdi-hite is 0.194, significant at the 1% level. This indicates that for every 1% increase in gfdi, hite increases by 0.194%. GF effectively promotes regional technological advancement by providing specialized funding support to enterprises for investing in green and low-carbon technologies. In Model (2), after adding the mediating variable hite, the coefficient of gfdi-lnc is −2.469, with an absolute value smaller than that of gfdi-lnc without the mediating variable. The coefficient of hite-lnc is −13.56, both significant at the 1% level, indicating that the mediating role of hite is established. Technological innovation can drive the development and application of new technologies, such as new energy technologies, energy-saving technologies, and carbon capture and storage technologies, which can significantly reduce carbon emissions. Additionally, these new technologies also lead to the optimization and upgrading of industrial structures, effectively reducing dependence on high-carbon-emission industries, thereby lowering the carbon emission per unit of economic output.
In Models (3) and (4), the mediating role of energy structure adjustment in GF-promoted carbon reduction is revealed. Model (3) shows that the coefficient of gfdi-es is −0.410, significant at the 1% level, indicating that for every 1% increase in gfdi, es decreases by 0.41%. In Model (4), after adding es, the coefficient of gfdi-lnc is −2.113, with an absolute value smaller than that of gfdi-lnc without the mediating effect. The coefficient of es-lnc is 1.151, both significant at the 1% level, indicating that the mediating effect of es is established. The empirical results show that GF, through its capital allocation function, supports renewable energy projects and low-carbon technologies, reduces the proportion of coal in primary energy, and improves energy efficiency. The optimization of the energy structure significantly reduces CEI.
Therefore, based on the above results, Hypothesis 2 is supported.
Prior to creating a spatial econometric model, it is important to analyse the spatial correlation properties between CEI and the amount of GF development in respect to their geographical and economic distributions. The Table 7 displays the calculated global Moran’s I index.
The global Moran'I index of lnc and gfdi from 2011 to 2020 is positive under the W1 and W2 weight matrices and significant at the 1% level, indicating a spatial correlation. From the perspective of index values, the leading weight matrix has higher index values than the economic geography weight matrix. From the perspective of time trend, the global Moran'I coefficients of lnc and gfdi fluctuate under both weight, showing an upward trend.
Such findings underscore a spatial and economic symbiosis between carbon emissions and the maturation of green financial mechanisms. This interconnectedness is palpable either as a decrement in carbon emissions within a particular province or as an augmentation in the advancement of GF endeavors. Anticipatedly, these transitions bestow beneficial spatial spillover effects upon contiguous provinces. Given the heterogeneity in economic development and geographical adjacency, the spatial ramifications upon neighboring provinces are markedly salient, with an inherent tendency for such impacts to intensify over time.
Before conducting spatial econometric model regression, it is necessary to determine the spatial econometric model used through testing. The model results are shown in the Table 8.
Utilizing the weight matrix W1 for analytical testing, both the Lagrange Multiplier (LM) and the Likelihood Ratio (LR) test outcomes are statistically significant at the 1% confidence level. As posited by Elhorst (2014), the Spatial Durbin Model (SDM) emerges as the appropriate choice for this analysis. The WALD test results, presented subsequently, further reinforce the decision to adopt the SDM model for this investigation. When the weight matrix W2 is employed for testing, the LM’s Spatial lag test achieves statistical significance at the 10% confidence level. Conversely, both the LR and WALD tests manifest significance at the 1% level. Drawing upon the outcomes of the LR and WALD tests, the selection gravitates towards the SDM model for this particular analytical context.
Based on the test results, the fixed-effects Spatial Durbin Model (SDM) was employed to empirically examine the spatial effects of GF development on the reduction of CEI.
As shown in Table 9, when using the economic geography weight matrix W1, the rho value is 0.122, but its statistical significance is insufficient, indicating that there may be positive spatial dependence between spatial units, but its impact on the model is not statistically significant. This may be due to sample size limitations or the effects of other potential unobserved variables. In the estimation of direct effects, both gfdi and various control variables exhibit a negative relationship with lnc, achieving statistical significance at the 1% level, consistent with previous research findings. From a spatial effect perspective, the coefficient of gfdi is −4.825, significant at the 5% level, indicating that the carbon reduction effect of gfdi has a spatial effect. The increase in gfdi not only reduces the lnc in the local region but also decreases the lnc in economically similar and geographically proximate areas. The funds provided by GF and the resulting new technologies produce a diffusion effect in regions with similar economic levels and relative geographical proximity, also promoting the reduction of their CEI. Regarding the control variables, both lnmal and inop display significant positive spatial correlations. This trend may be related to inter-regional industrial migration, especially in the context of accelerated marketization or enhanced support for the tertiary sector, where high CEI enterprises may choose to relocate to areas with similar levels of economic development and geographical proximity.
When using the adjacency weight matrix W2, the rho value is 0.269 and statistically significant at the 1% level. This matrix assigns higher weights to geographically adjacent regions, emphasizing the potential mutual influences and interactions between neighboring areas. In the estimation of direct effects, both gfdi and control variables exhibit negative effects on lnc, statistically significant at the 1% level. From a spatial perspective, the coefficient of gfdi is −2.125, significant at the 1% level, also indicating a spatial effect. Carbon emissions and environmental pollution have significant externalities; emission reduction measures in one region can improve environmental quality in neighboring areas, generating positive environmental benefits over a wider range. Furthermore, the control variable lnur shows a significant positive spatial effect. This can be attributed to the notion that an increase in urban population density in one region might attract more economic activities and population inflow, leading to a short-term rise in CEI in neighboring areas.
Based on the regression results using matrices W1 and W2, Hypothesis 3 of this study is validated, indicating that the GF Development Index exhibits a significant spatial spillover effect on the reduction of CEI.
Upon comparing the regression results under the two matrices, the model associated with matrix W2 exhibits superior goodness-of-fit, and the significance levels of the regression coefficients for various variables are also enhanced. This underscores the notion that in the spatial effect of GF on the reduction of CEI, geographical proximity plays a pivotal role in the mutual influences and interactions among regions. Such a conclusion can be attributed to the fact that geographically adjacent regions tend to share greater similarities and interactions in aspects such as resource endowments, industrial structures, and economic development levels. These shared attributes consequently lead to more pronounced impacts on adjustments in CEI. Moreover, regions in close geographical proximity are more intricately interconnected in terms of transportation networks, energy supply chains, and industrial linkages. These combined factors exert a collective influence on the CEI across regions, resulting in a heightened spatial dependence among neighboring areas. Therefore, by considering the dimension of geographical adjacency, one can more accurately discern the mutual influences and interactions between regions in terms of reducing CEI. Such insights offer robust empirical support for the formulation and enactment of coordinated regional carbon reduction policies.
Given the presence of spatial cross-terms in the Spatial Durbin Model (SDM), the point estimation method it employs is prone to errors and may not directly reflect the extent to which GF impacts CEI. To elucidate this influence with greater precision, this study adopts the methodology proposed by LeSage and Pace (2009). By performing partial differentiation on the variables within the SDM, the spatial effects of GF on CEI are decomposed into direct effects, indirect effects, and total effects.
Table 10 lists the spillover effect results of the two matrices.
Firstly, when using the economic geography weight matrix W1, the spillover effect of gfdi is significant at the 5% level. Compared to W2, the significance level is lower, but the absolute value of the coefficient is larger, indicating that GF exhibits stronger spillover effects in regions with similar economic levels and geographical proximity. Green finance promotes the development and application of low-carbon technologies by providing financial support. These technologies and funds not only have an impact locally but can also diffuse to neighboring regions through inter-firm cooperation and technology transfer, thereby generating spatial spillover effects. In the context of regional economic integration and policy coordination, green finance policies and measures can be mutually referenced and promoted across different regions. The same policies are more likely to yield better results in regions with similar economic levels and geographical proximity. Due to the diversity and complexity of the spillover pathways, the spillover effects become more pronounced, but this also makes predicting and controlling these effects more challenging, thereby reducing the certainty of the spillovers.
Secondly, when using the adjacency matrix W2, the significance of the gfdi spillover effect is higher, being significant at the 1% level, but the spillover effect is relatively weaker. Due to the obvious externalities of carbon emissions and environmental pollution, emission reduction measures in neighboring regions can improve not only the local environmental quality but also reduce the CEI of adjacent regions through these externalities. The certainty of such spillover effects is relatively higher. For example, the environmental renovation projects in the steel industry supported by green finance in Hebei Province have not only improved local air quality but also reduced pollutant emissions, thereby affecting the neighboring Beijing.
To further examine the pathways of spatial spillover effects, a heterogeneity test was conducted following the methodology outlined in Section 5.1.3. The results are presented in Table 11.
When employing matrix W1, the spatial spillover effects of the gfdi were significant only in the Central region, while they were not significant in the Eastern and Western regions. However, upon using matrix W2, the spatial spillover effects of gfdi were notably negative across all three regions, with the absolute values of the coefficients descending in order from the Western to the Central and then to the Eastern region. This aligns with the conclusions derived from non-spatial heterogeneity tests. The Western region exhibits significant potential for environmental improvement, combined with national policy support for the development of this area, promoting the development of GF. This not only significantly reduces carbon emissions within the region but also exerts a more profound spillover effect on adjacent areas.
Through empirical analysis, this study has reached the following conclusions:
First, GF not only directly reduces CEI but also indirectly promotes its reduction by stimulating technological innovation and optimizing the energy structure, thereby validating Hypotheses 1 and Hypotheses 2. This indicates that the role of GF extends beyond providing financial support, as it also drives technological progress and industrial restructuring, achieving broader environmental benefits. This study, focusing on CEI, further validates the findings of Fang et al. (2022), and Ren et al. (2020) on the impact of GF on carbon emissions, providing a more detailed analysis.
Second, the results of heterogeneity analysis and spatial heterogeneity analysis show that GF has a better effect on reducing CEI in areas with high CEI, rich resources, but relatively lagging economies, such as the western regions of China. This finding aligns with the conclusions of Ran & Zhang (2023) and Wang & Ma (2022) obtained through heterogeneity analysis. This study further demonstrates the validity of these conclusions from the perspective of spatial spillover effects. This emphasizes the need for targeted implementation of GF policies in different regions, particularly in economically underdeveloped areas where GF can have greater potential.
Third, GF not only reduces CEI in the implementing regions but also positively impacts neighboring provinces, thereby validating Hypotheses 3. This suggests that GF plays a crucial role in regional cooperation and overall environmental improvement. There are certain disagreements regarding the spatial spillover effects of GF on CEI. This study, from the perspective of CEI, verifies the conclusions of Chen & Chen (2021) and Su et al. (2024), and Further comparisons reveal that the spillover effects are more certain in adjacent regions, while the spillover effects are stronger in regions with similar economic levels and geographical proximity.
Finally, the intensity of environmental regulation has a significant positive impact on CEI in all tests. While Wang & Ma (2022) and Ran & Zhang (2023) validated the effectiveness of environmental regulation intensity in reducing carbon emissions, Pei et al. (2019) and Ngo (2022) found that when the intensity of environmental regulation exceeds a critical value, it can increase the burden on enterprises, leading to higher CEI. This study also validates this conclusion from the perspective of CEI, indicating that reasonable environmental regulation policies are crucial for achieving low-carbon goals.6.2 Suggestions.
Based on the above conclusions, we propose the following recommendations:
First, the establishment of a GF Innovation Center is crucial. This center should facilitate collaboration between the government, financial institutions, enterprises, and research institutions. It can provide services such as green financial product design, market promotion, and risk assessment, helping enterprises better understand and utilize green financial tools.
Second, strengthening policy coordination and resource sharing is essential. This includes promoting regional green finance cooperation to enhance spillover effects and achieve overall environmental improvement. For example, establishing a regional green finance cooperation fund to support inter-provincial green projects and creating a regional green finance information-sharing platform to increase transparency and efficiency in resource allocation. Through the cooperation fund and information-sharing platform, green projects in adjacent provinces can receive more support, and the exchange of experiences and resources can enhance the spillover effects. Governments at all levels within the region should ensure policy consistency and resource sharing, particularly in environmental standards and green project evaluations, by promoting unified environmental assessment standards and simplifying the approval process for inter-provincial projects.
Third, enhancing international and regional green project coordination and cooperation aims to improve the openness of regional financial markets. This includes accelerating the formulation of laws and regulations related to green development and climate change response, and promoting the establishment of institutions, coordination, supervision, and evaluation systems for GF and climate financing.
First, improving tax incentives and low-interest loan policies is critical to continuously encourage the development of GF. The government can implement tax incentive policies, such as reducing or exempting income tax or value-added tax for green enterprises, to encourage investment in low-carbon technologies and renewable energy projects. Additionally, providing low-interest loans or interest subsidy policies can help enterprises reduce financing costs and further promote green investment.
Second, developing differentiated GF policies based on regional characteristics is necessary. To further enhance the effectiveness of GF in the eastern regions and consolidate the positive trends in the western regions, differentiated GF policies must be implemented. In the eastern regions, diverse green financial products such as green bonds, green funds, carbon credits, and carbon trading markets should be encouraged to meet the diverse needs of investors. Simultaneously, environmental rights trading, such as emission rights, water rights, and energy usage rights, should be explored to establish innovative financing models and improve the efficiency of GF in reducing carbon emission intensity. In the western regions, local ecological resources should be leveraged to explore innovative mechanisms that integrate GF with ecological development, utilizing the advantages of GF and the unique industrial characteristics of the regions to activate local financial markets and promote sustainable economic development. GF policies should also avoid the “pollution first, treatment later” model, ensuring that green development and environmental protection progress simultaneously.
Third, balancing the impact of environmental regulation policies on enterprises is vital to avoid excessive regulation that may have negative effects. For instance, regularly evaluating the effectiveness of environmental regulation policies and adjusting their intensity based on the evaluation results is necessary. Different regulatory measures should be applied to enterprises of varying types and sizes to avoid a “one-size-fits-all” approach. Additionally, providing technical support and training can help enterprises enhance their compliance capabilities and technical standards.
This study has drawn several valuable conclusions by analyzing the impact of GF on CEI. However, there are several limitations in both the methodology and conclusions. Firstly, the data is primarily sourced from provincial-level statistics in China, which may not fully account for policy differences and market environments across various countries and regions, thereby limiting the generalizability of the findings. Secondly, while the study employs direct, mediation effect models, and the Spatial Durbin Model (SDM), it fails to fully capture the dynamic relationship between GF and CEI, particularly the long-term effects. Additionally, the interaction between environmental regulatory intensity and GF is not deeply explored, overlooking the potentially complex synergistic or antagonistic relationships. Lastly, due to data availability constraints, certain potential moderating variables were not included in the analysis, potentially affecting the comprehensiveness and accuracy of the results.
Based on the limitations of this study, future research should first incorporate data from more countries and regions to expand the data sources and thus enhance the generalizability of the findings. Secondly, analyses should use methods like GMM or time series to capture the long-term dynamic relationship between GF and CEI. Lastly, attention should be paid to the impact of environmental regulatory intensity on CEI, exploring the synergistic or antagonistic effects of GF under different levels of environmental regulation. Additionally, moderating effects and U-shaped nonlinear relationships are also important areas to explore. Future research should investigate which factors might moderate the impact of GF on CEI and how different policy tools, firm sizes, and industry characteristics influence the effectiveness of GF. By adopting these approaches, we can gain a more comprehensive understanding of the impacts of GF under various policy environments and market conditions, thereby enhancing the validity and reliability of the research.
The raw data supporting the conclusions of this article will be made available by the authors, without undue reservation.
XL: Conceptualization, Data curation, Methodology, Software, Visualization, Writing–original draft, Writing–review and editing, Supervision. JL: Data curation, Formal Analysis, Writing–review and editing. BZ: Resources, Writing–review and editing.
The author(s) declare that no financial support was received for the research, authorship, and/or publication of this article.
Throughout this study, I received invaluable support and assistance for which I am deeply grateful. First and foremost, I extend my heartfelt thanks to my advisor, Professor Chen Yunping. Throughout the research process, Professor Chen’s profound academic expertise and meticulous approach to research provided me with invaluable guidance and support. His academic mentorship, coupled with his personal care and assistance, significantly contributed to my academic progress and growth. I also wish to express my gratitude to my classmates, especially Mei Mengli and Sun Ying. Their partnership in every phase of my research was instrumental. Their suggestions and assistance were crucial to the completion of this study, and our discussions and collaborations were immensely beneficial. Lastly, my deepest appreciation goes to my family, particularly my daughter. Their understanding, support, and encouragement were the strong backbone of my research journey. Their love and encouragement were my endless source of motivation and warmth during busy research times. Thank you once again to everyone who supported and helped me. Without your assistance, my research would not have been as successful. ChatGPT-3.5 was used for language editing and refinement.
Author BZ was employed by Jiangxi Chinese Media Blue Ocean International Investment Co., Ltd.
The remaining authors declare that the research was conducted in the absence of any commercial or financial relationships that could be construed as a potential conflict of interest.
All claims expressed in this article are solely those of the authors and do not necessarily represent those of their affiliated organizations, or those of the publisher, the editors and the reviewers. Any product that may be evaluated in this article, or claim that may be made by its manufacturer, is not guaranteed or endorsed by the publisher.
Anquetin, T., Coqueret, G., Tavin, B., and Welgryn, L. (2022). Scopes of carbon emissions and their impact on green portfolios. Econ. Model. 115, 105951. doi:10.1016/j.econmod.2022.105951
Baltagi, B. H., and Baltagi, B. H. (2008). Econometric analysis of panel data volume 2. Chichester: Wiley, 135–145. doi:10.1007/978-3-030-53953-5
Baron, R. M., and Kenny, D. A. (1986). The moderator–mediator variable distinction in social psychological research: conceptual, strategic, and statistical considerations. J. Pers. Soc. Psychol. 51 (6), 1173–1182. doi:10.1037/0022-3514.51.6.1173
Cao, S., Nie, L., Sun, H., Sun, W., and Taghizadeh-Hesary, F. (2021). Digital finance, green technological innovation and energy-environmental performance: evidence from China's regional economies. J. Clean. Prod. 327, 129458. doi:10.1016/j.jclepro.2021.129458
Carraro, C., Favero, A., and Massetti, E. (2012). Investments and public finance in a green, low carbon, economy. Energy Econ. 34, S15–S28. doi:10.1016/j.eneco.2012.08.036
Chen, X., and Chen, Z. (2021). Can GF development reduce carbon emissions? Empirical evidence from 30 Chinese provinces. Sustainability 13 (21), 12137. doi:10.3390/su132112137
Chen, Z., Su, Y., Wang, X. Y., and Wu, Y. (2021). The price discrimination and environmental effectiveness in carbon emission trading schemes: a theoretical approach. J. Clean. Prod. 283, 125196. doi:10.1016/j.jclepro.2020.125196
Climate Bonds Initiative, China Central Depository Clearing Co. Ltd. (2020). China green bond market report 2020. Available at: https://www.climatebonds.net/resources/reports/china-state-market-2020-report.
Cropper, M. L., and Oates, W. E. (1992). Environmental economics: a survey. J. Econ. Lit. 30 (2), 675–740. doi:10.2307/2727810
Dong, F., Yu, B., Hadachin, T., Dai, Y., Wang, Y., Zhang, S., et al. (2018). Drivers of carbon emission intensity change in China. Resour. Conserv. Recycl. 129, 187–201. doi:10.1016/j.resconrec.2017.10.035
Elhorst, J. P. (2014). Matlab software for spatial panels. Int. Reg. Sci. Rev. 37 (3), 389–405. doi:10.1177/0160017612452429
Fan, H., Peng, Y., Wang, H., and Xu, Z. (2021). Greening through finance? J. Dev. Econ. 152, 102683. doi:10.1016/j.jdeveco.2021.102683
Fang, Z., Yang, C., and Song, X. (2022). How do green finance and energy efficiency mitigate carbon emissions without reducing economic growth in G7 countries? Front. Psychol. 13, 879741. doi:10.3389/fpsyg.2022.879741
G20 GF Study Group (2016). G20 GF synthesis report. Available at: http://www.g20.utoronto.ca/2016/green-finance-synthesis.pdf.
Gianfrate, G., and Peri, M. (2019). The green advantage: exploring the convenience of issuing green bonds. J. Clean. Prod. 219, 127–135. doi:10.1016/j.jclepro.2019.02.022
Gu, B., Chen, F., and Zhang, K. (2021). The policy effect of green finance in promoting industrial transformation and upgrading efficiency in China: analysis from the perspective of government regulation and public environmental demands. Environ. Sci. Pollut. Res. 28 (34), 47474–47491. doi:10.1007/s11356-021-13944-0
Guo, C. Q., Wang, X., Cao, D. D., and Hou, Y. G. (2022). The impact of green finance on carbon emission--analysis based on mediation effect and spatial effect. Front. Environ. Sci. 10, 844988. doi:10.3389/fenvs.2022.844988
He, L., Zhang, L., Zhong, Z., Wang, D., and Wang, F. (2019). Green credit, renewable energy investment and green economy development: empirical analysis based on 150 listed companies of China. J. Clean. Prod. 208, 363–372. doi:10.1016/j.jclepro.2018.10.119
International Energy Agency (2014). WEO-2014 special report: world energy investment outlook. Available at: https://www.iea.org/reports/world-energy-investment-outlook.
International Energy Agency (2021). Global energy review: CO2 emissions in 2021. Available at: https://www.iea.org/reports/global-energy-review-co2-emissions-in-2021-2.
Ji, Y., Xue, J., and Zhong, K. (2022). Does environmental regulation promote industrial green technology progress? Empirical evidence from China with a heterogeneity analysis. Int. J. Environ. Res. Public Health 19 (1), 484. doi:10.3390/ijerph19010484
Jin, D., and Mengqi, N. (2011). The paradox of green credit in China. Energy Procedia 5, 1979–1986. doi:10.1016/j.egypro.2011.03.340
Lee, C. C., and Lee, C. C. (2022). How does GF affect green total factor productivity? Evidence from China. Energy Econ. 107, 105863. doi:10.1016/j.eneco.2022.105863
LeSage, J., and Pace, R. K. (2009). Introduction to spatial econometrics. Chapman and Hall/CRC. doi:10.1201/9781420064254
Li, C., Solangi, Y. A., and Ali, S. (2023). Evaluating the factors of green finance to achieve carbon peak and carbon neutrality targets in China: a delphi and fuzzy AHP approach. Sustainability 15 (3), 2721. doi:10.3390/su15032721
Li, C., and Sun, X. (2023). Spatial effects of green credit on air quality: an empirical test based on the dual carbon perspective. Sustainability 15 (13), 10143. doi:10.3390/su151310143
Li, C., and Umair, M. (2023). Does green finance development goals affects renewable energy in China. Renew. Energy 203, 898–905. doi:10.1016/j.renene.2022.12.066
Li, W., Fan, J., and Zhao, J. (2022). Has green finance facilitated China’s low-carbon economic transition? Environ. Sci. Pollut. Res. 29 (38), 57502–57515. doi:10.1007/s11356-022-19891-8
Lin, Z., Wang, H., Li, W., and Chen, M. (2023). Impact of green finance on carbon emissions based on a two-stage LMDI decomposition method. Sustainability 15 (17), 12808. doi:10.3390/su151712808
Liu, H., Yang, J., Zhao, F., Jiang, L., and Li, N. (2024). Can green finance mitigate China’s carbon emissions and air pollution? An analysis of spatial spillover and mediation pathways. Sustainability 16 (4), 1377. doi:10.3390/su16041377
Liu, L., and Tobias, G. R. (2023). Application of green finance in promoting low-carbon transformation of enterprises. Adv. Sustain 3, 1–6. doi:10.26855/as.2023.06.001
Liu, X., Wang, E., and Cai, D. (2019). Green credit policy, property rights and debt financing: quasi-natural experimental evidence from China. Financ. Res. Lett. 29, 129–135. doi:10.1016/j.frl.2019.03.014
Liu, Z., Lam, J. F., Chen, H., Lin, G., and Chen, H. (2023). Can green investment improve China’s regional energy consumption structure? novel findings and implications from sustainable energy systems perspective. Front. Energy Res. 11, 1273347. doi:10.3389/fenrg.2023.1273347
Liza, F. F., Ahmad, F., Wei, L., Ahmed, K., and Rauf, A. (2024). “Environmental technology development and renewable energy transition role toward carbon-neutrality goals in G20 countries,” in Clean technologies environmental policy, 1–22. doi:10.1007/s10098-024-02804-3
Meo, M. S., and Abd Karim, M. Z. (2022). The role of GF in reducing CO2 emissions: an empirical analysis. Borsa Istanb. Rev. 22 (1), 169–178. doi:10.1016/j.bir.2021.03.002
Ngo, T. Q. (2022). How do environmental regulations affect carbon emission and energy efficiency patterns? A provincial-level analysis of Chinese energy-intensive industries. Environ. Sci. Pollut. Res. 29 (3), 3446–3462. doi:10.1007/s11356-021-15843-w
Pei, Y., Zhu, Y., Liu, S., Wang, X., and Cao, J. (2019). Environmental regulation and carbon emission: the mediation effect of technical efficiency. J. Clean. Prod. 236, 117599. doi:10.1016/j.jclepro.2019.07.074
Qin, J., Zhao, Y., and Xia, L. (2018). Carbon emission reduction with capital constraint under greening financing and cost sharing contract. Int. J. Environ. Res. Public Health. 15 (4), 750. doi:10.3390/ijerph15040750
Ran, C., and Zhang, Y. (2023). The driving force of carbon emissions reduction in China: does green finance work. J. Clean. Prod. 421, 138502. doi:10.1016/j.jclepro.2023.138502
Ren, X., Shao, Q., and Zhong, R. (2020). Nexus between green finance, non-fossil energy use, and carbon intensity: empirical evidence from China based on a vector error correction model. J. Clean. Prod. 277, 122844. doi:10.1016/j.jclepro.2020.122844
Schmidt, T. S. (2014). Low-carbon investment risks and de-risking. Nat. Clim. Change 4 (4), 237–239. doi:10.1038/nclimate2112
Su, X., Qiao, R., and Xu, S. (2024). Impact of green finance on carbon emissions and spatial spillover effects: empirical evidence from China. J. Clean. Prod. 457, 142362. doi:10.1016/j.jclepro.2024.142362
Sun, H., Chen, T., and Wang, C. N. (2023). Spatial impact of digital finance on carbon productivity. Geosci. Front. 101674, 101674. doi:10.1016/j.gsf.2023.101674
Sun, J., Zhai, N., Miao, J., and Sun, H. (2022). Can green finance effectively promote the carbon emission reduction in “local-neighborhood” areas?empirical evidence from China. Agriculture 12 (10), 1550. doi:10.3390/agriculture12101550
Taghizadeh-Hesary, F., and Yoshino, N. (2019). The way to induce private participation in green finance and investment. Financ. Res. Lett. 31, 98–103. doi:10.1016/j.frl.2019.04.016
Tong, J., Yue, T., and Xue, J. (2022). Carbon taxes and a guidance-oriented green finance approach in China: path to carbon peak. J. Clean. Prod. 367, 133050. doi:10.1016/j.jclepro.2022.133050
Wang, J., and Ma, Y. (2022). How does green finance affect CO2 emissions? Heterogeneous and mediation effects analysis. Front. Environ. Sci. 10, 931086. doi:10.3389/fenvs.2022.931086
Wang, R., and Wang, F. (2022). Exploring the role of green finance and energy development towards high-quality economic development: application of spatial Durbin model and intermediary effect model. Int. J. Environ. Res. Public Health. 19 (14), 8875. doi:10.3390/ijerph19148875
Wang, S., Huang, Y., and Zhou, Y. (2019). Spatial spillover effect and driving forces of carbon emission intensity at the city level in China. J.Geogr. Sci. 29, 231–252. doi:10.1007/s11442-019-1594-1
Xu, X., and Li, J. (2020). Asymmetric impacts of the policy and development of green credit on the debt financing cost and maturity of different types of enterprises in China. J. Clean. Prod. 264, 121574. doi:10.1016/j.jclepro.2020.121574
Yang, T., and Wang, R. (2023). Research on the impact of green finance and the digital economy on the energy consumption structure in the context of carbon neutrality. Sustainability 15 (22), 15874. doi:10.3390/su152215874
Yang, Z., Yuan, Y., and Zhang, Q. (2022). Carbon emission trading scheme, carbon emissions reduction and spatial spillover effects: quasi-experimental evidence from China. Front. Environ. Sci. 9, 824298. doi:10.3389/fenvs.2021.824298
Yu, C. H., Wu, X., Zhang, D., Chen, S., and Zhao, J. (2021). Demand for green finance: resolving financing constraints on green innovation in China. Energy Pol. 153, 112255. doi:10.1016/j.enpol.2021.112255
Zakari, A. (2022). The role of green finance in promoting sustainable economic and environmental development. Stud. Appl. Econ. 40 (3). doi:10.25115/eea.v40i3.5398
Keywords: green finance, carbon emission intensity, technological innovation, environmental regulation intensity, spatial spillover effect
Citation: Liu X, Lu J and Zhou B (2025) The impact of green finance on carbon emission intensity in China: mediating and spatial effects. Front. Environ. Sci. 12:1403246. doi: 10.3389/fenvs.2024.1403246
Received: 20 March 2024; Accepted: 01 August 2024;
Published: 03 January 2025.
Edited by:
Hêriş Golpîra, Islamic Azad University, Sanandaj Branch, IranCopyright © 2025 Liu, Lu and Zhou. This is an open-access article distributed under the terms of the Creative Commons Attribution License (CC BY). The use, distribution or reproduction in other forums is permitted, provided the original author(s) and the copyright owner(s) are credited and that the original publication in this journal is cited, in accordance with accepted academic practice. No use, distribution or reproduction is permitted which does not comply with these terms.
*Correspondence: Xiaonan Liu, MTUyMTAwOTUyODNAMTYzLmNvbQ==
Disclaimer: All claims expressed in this article are solely those of the authors and do not necessarily represent those of their affiliated organizations, or those of the publisher, the editors and the reviewers. Any product that may be evaluated in this article or claim that may be made by its manufacturer is not guaranteed or endorsed by the publisher.
Research integrity at Frontiers
Learn more about the work of our research integrity team to safeguard the quality of each article we publish.