- School of Natural Resources, Faculty of Geographical Science, Beijing Normal University, Beijing, China
Haze is a pollution phenomenon that has become increasingly frequent in recent years, primarily composed of SO2, NOx, and particulate matter. Since the “PM2.5 Crisis” in 2013, the Beijing-Tianjin-Hebei region has been plagued by haze. After a series of high-intensity management activities, the air quality in Beijing has continued to improve. To investigate the effectiveness of the haze control measures in Beijing, a dynamic management model was constructed using the system dynamics approach and implemented using Stella software. The model is simulated and evaluated the contribution to different governance strategies (increasing investment in science and technology innovation, reducing motor vehicle ownership, reducing coal consumption, and a combination policy) to reduce haze in Beijing from 2010 to 2025. The validity and robustness of the model were verified through model testing and validation. The simulation results showed that: (1) the combination policy has the most significant effect on reducing energy consumption and pollution emissions, which would reduce energy consumption by 38.93 million tons and pollution emissions by 128.33 thousand tons in 2025, (2) among the single treatment measures, reducing industrial coal consumption is the most effective measure to reducing haze, (3) it is necessary to strengthen the joint prevention and control of the Beijing-Tianjin-Hebei region in the future to promote the sustainable development of the regional environment.
1 Introduction
Haze is an increasingly frequent pollution phenomenon in recent years, primarily caused by human activities and consists of SO2, NOx and particulate matter (Chen et al., 2022). Compared with fog, haze not only affects visibility but also poses significant health risks. China, as the world’s largest developing country, the rapid industrialization and urbanization have led to severe haze pollution, especially in Beijing-Tianjin-Hebei region (Zhang et al., 2018; Li et al., 2023), which severely hampers social and economic development and residents’ health (Hu et al., 2015; Han et al., 2016; Liao and Wang, 2019; Zhao and Wang, 2024). In 2011, Beijing ranked among the lowest in the world in terms of air quality (WHO, 2011). As a result, it is crucial to implement effective strategies and measures with the actual situation to alleviate the haze pollution crisis and promote sustainable development in the region.
Simultaneously, public demand to address haze weather has grown significantly, which has greatly boosted the government’s determination to tackle the haze (Gao et al., 2017). The Beijing Municipal Government has formulated and promulgated a series of local laws and regulations, such as The Beijing Municipal Air Pollution Prevention and Control Regulations, The Beijing Clean Air Action Plan 2016, and The Beijing Three - Year Action Plan to Win the Blue-Sky Defense War. These efforts have resulted in continuous improvements in the air quality of Beijing, with the average concentration of PM2.5 decreasing to 38 micrograms per cubic meter in 2020. However, the current level is still far from the World Health Organization’s global standard of less than 10 micrograms per cubic meter per year for safety and health, indicating that there is still a long way to go in the management of haze.
The formation of haze has been extensively studied, with researchers focusing on various factors such as economic development, energy consumption structure, and technological advancements in order to understand and mitigate haze pollution (Liu, Y. et al., 2023; Wang, X. et al., 2023b). Wang et al. (2015) identified vehicle emissions as a significant contributor to haze in Beijing, emphasizing the potential of new energy vehicles in reducing air pollutant emissions. Building upon this, Zhang et al. (2020) investigated the impact of Beijing’s new energy vehicle subsidy policy in reducing atmospheric pollutants, determining the optimal proportion of new energy vehicles for promotion. Examining the spatial effects of economic development and energy consumption structure on haze pollution, Li and Mao (2020) employed spatial autoregressive and spatial error models. Their research revealed a strong correlation between haze pollution and changes in energy consumption structure and economic development, with a relationship resembling an inverted U-shaped curve. Technological developments play an essential role in haze control. Yi et al. (2020) constructed a theoretical framework to examine the relationship between technological progress heterogeneity and haze pollution. Using the Generalized Method of Moments (GMM), they empirically investigated the diverse functions that different types of technological progress serve in reducing haze.
The above studies offer valuable insights into controlling haze pollution. However, most of these studies are limited to qualitative analyses and lack dynamic quantitative analysis. Based on this, this study introduces the system dynamics (SD) approach, which is based on feedback control theory and emphasizes system behavior, structure, and causal feedback relationships, and is suitable for dealing with long-term and cyclical problems (Anderies, 2015; Wang, X. et al., 2023a). Simulation experiments based on system dynamics can play the role of a system laboratory based on a human-machine combination to obtain rich and deep information and provide a strong basis for decision-making. Such as urban household waste management policies (Xiao et al., 2020), air pollution charging (APCF) policy (Jia et al., 2019), lead pollution under different policies (Joshi et al., 2021), and impacts of various decisions and measures on agricultural greenhouse gas emissions (Dace et al., 2015), etc. These studies provide a theoretical basis for subsequent policy formulation.
This study attempts to propose a dynamical model of Beijing haze pollution system by treating the changes in science and technology innovation input, motor vehicle ownership, and coal consumption as dynamical system behaviors. It deeply investigates the dynamic changes and feedback mechanism between influencing factors and haze pollution. Nitrogen oxides (NOx), sulfur dioxide (SO2), and total suspended particulates (TSP) are selected as the indicators of air pollution level (Chen et al., 2022). Relevant historical data from 2010 to 2020 are used for simulation. The purpose of this study is to provide feasible and effective policy recommendations for the next stage of haze control and to promote the sustainable economic development environment in the Beijing-Tianjin-Hebei region.
2 Material and methods
2.1 Causal loop diagram
A causal loop diagram is a technique that illustrates the feedback effects of information within a system. Causality refers to the relationship between a cause and result, while the loop represents the closed chain formed by these two elements. “+” denotes causation in the same direction, while “-” indicates opposite directions for change (Tang, 2019).
Previous studies have illustrated that economic development (Wu et al., 2020; Dong et al., 2021), pollutant emissions (Zhang et al., 2020) and energy consumption (Li and Mao, 2020) are the critical factors influencing the formation of haze. Thus, in this study, the haze control system is divided into three modules: economic, environmental and energy, which interact and constrain each other through their respective input and output variables. A causal feedback loop can be established based on the coupling relationships among these three systems as well as their respective internal environments (Figure 1).
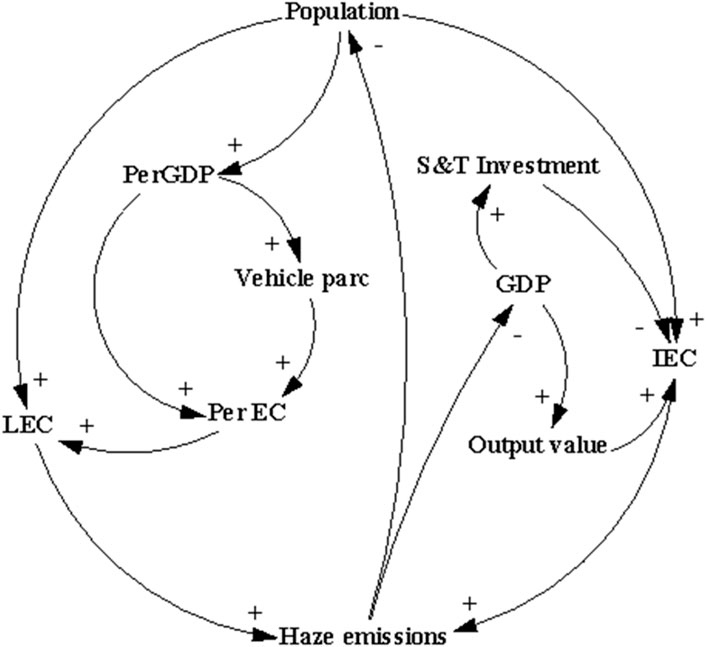
Figure 1. Causal loop diagrammed of smog control in Beijing. Notes: (Per EC: Per capita energy consumption; LEC: Life energy consumption; IEC: Industrial energy consumption; S&T Investment: Investment in scientific and technological innovation).
There exists a highly interdependent relationship among the economy, environment and energy systems. As the primary agents of economic activities and energy consumption, human beings intricately linked to the entire system, which is also susceptible to damage from atmospheric pollution. In the living energy consumption-haze emission loop, the total amount of living energy consumption is controlled by influencing the per capita energy consumption through per capita GDP and motor vehicle ownership. Additionally, in the industrial energy consumption-haze emission loop, the total GDP, the industrial structure and the input of science and technology control the total industrial energy consumption.
2.2 SD model construction
Stock and flow are two crucial variables in system dynamics modeling that simulate the process of system feedback loops over time (Harandi et al., 2024). A stock is a cumulative amount that characterizes the state of the system and provides the basis for decision-making and action. The flow changes the stock and is the rate variable that determines the rate of stock change. When the flow is positive, the stock increases, and when the flow is negative, the stock decreases. Based on the causal loop diagram, the Beijing haze control system is quantified by constructing a stock flow diagram.
Firstly, according to the feedback relationship between different variables and the operability principle of the system dynamics model, we further refined the above three modules and constructed a three-level indicators evaluation system (Table 1).
2.2.1 Socio-economic module
The socio-economic module mainly considers the population, industrial structure, output level, motor vehicles, and science and technology. We directly use the population size as data for the population indicator (Wang et al., 2019) and incorporate the feedback loop of population dynamics through birth and death rates. Industrial emissions of pollutants are a crucial contributor to the occurrence of haze, thus rendering industrial structure a significant variable (Shi et al., 2020). According to the meaning of industrial structure, i.e., the proportion of each sector in the overall social production, this study further selects the share of primary, secondary and tertiary sectors in GDP to describe the industrial structure (Hao et al., 2016; Gan et al., 2021; Zhou et al., 2021). Moreover, GDP and GDP per capita are the most intuitive indicators to reflect the level of economic development of a country or region (Zhao et al., 2018; Zhou et al., 2021). Additionally, previous research has shown that motor vehicle ownership (Zhou et al., 2019; Li and Mao, 2020) and R&D funding (Wu et al., 2020; Gan et al., 2021) are also major variables in the analysis of factors affecting haze. Among them, the population and GDP are horizontal variables, while per capita GDP serves as an auxiliary variable connected with other modules.
2.2.2 Environmental module
The environmental module consists mainly of gaseous pollutant emissions (SO2 and NOx emissions) (Yang et al., 2020; Li et al., 2021) and total suspended particulates (TSP emissions) (Shang et al., 2020), which are known to be direct contributors to haze.
2.2.3 Energy module
Energy consumption is a critical factor in the formation of haze (Tang et al., 2018). It can be broadly categorized into two types: industrial energy consumption and living energy consumption (Zhou et al., 2019). The industrial energy consumption was further analyzed based on the enterprise composition of primary, secondary, and tertiary industries, as well as their degree of impact on environmental quality (Li, Z. et al., 2019). Additionally, the energy consumption per unit of output value is also an important factor to consider (Liu and Xia, 2018; Dong et al., 2019). The living energy consumption is represented by the per capita energy consumption (Hao et al., 2016) and the living coal consumption (Liu et al., 2020). China’s urban air pollution is mainly caused by its coal-based energy structure, so the coal consumption ratio (Dong et al., 2019; Chen et al., 2022) is used as an auxiliary variable in the energy and environmental module. Generally, coal consumption is mainly utilized for metallurgy, power generation, building, and chemicals, as well as residential consumption (Liu et al., 2017). The latter is referred to as living coal consumption, while the first four categories are classified as industrial coal consumption.
Based on the coupling mechanism of the socio-economic, environment and energy, this paper constructs a stock/flow map of the Beijing haze control system, as shown in Figure 2.
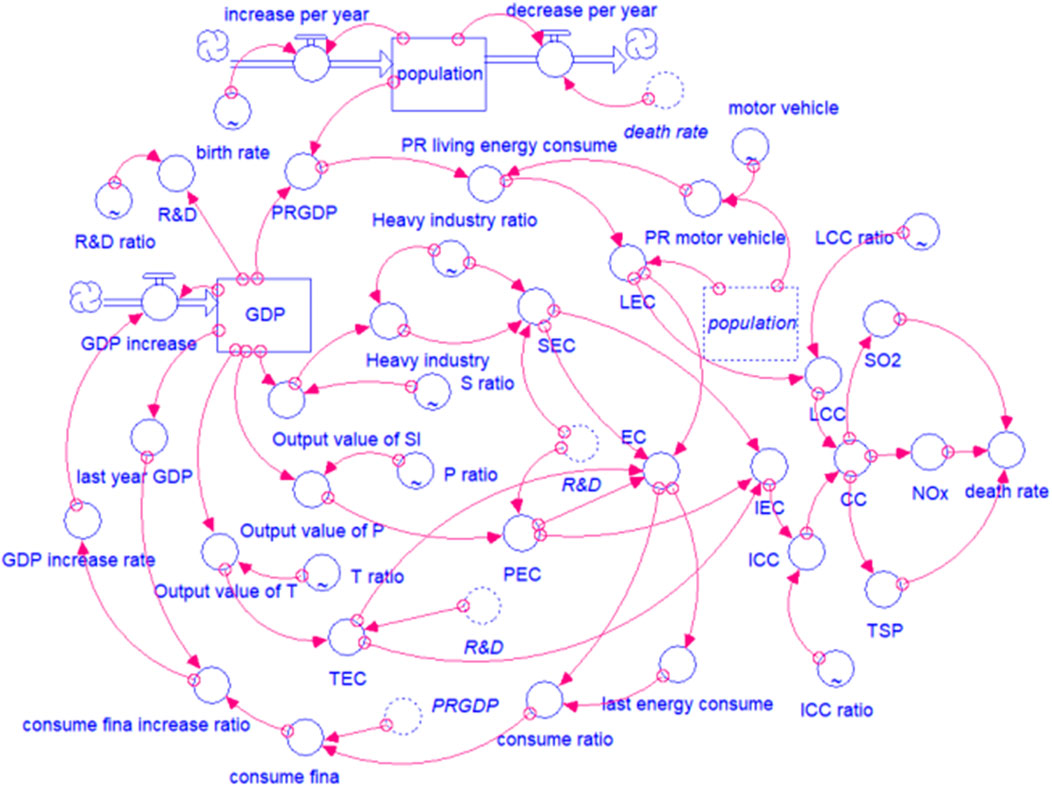
Figure 2. Stocks and flows of Beijing haze control system. Notes: Please refer to Tabel 1 for the full names of the indicators.
2.3 Model assumption
From the perspective of haze mitigation, we made several assumptions as follows. Firstly, from the viewpoint of sensitivity and robustness analysis, we focused on the simulation time step and set 1 year, 0.5 year and 0.25 year to test the robustness of the model. Secondly, based on the perspective of emission reduction effect, our study focused on quantifying the degree of air pollution with SO2, NOx and TSP, where NOx refers to NO and NO2. Thirdly, we mainly considered industrial and living energy consumption in terms of energy consumption. Finally, we included scientific and technological investment and motor vehicle ownership at the socio-economic level to reveal the impact of haze control.
Settings: Initial time = 2010, final time = 2025, time step = 1, unit of time: Year.
2.4 Quantized model
The Beijing haze control system is a complex system that has a high integration of the socio-economic, energy and environment, taking into account the stability of the indicators and the correlation between variables. Based on the structural characteristics of the haze control system, the model parameters are set by methods such as arithmetic averaging (birth rate, etc.), trend methods (motor vehicle ownership, etc.), table functions (industry output value, etc.), regression methods (per capita GDP and per capita energy consumption relationship, etc.), and adjusting the parameters according to the actual situation in Beijing to achieve model optimization.
2.5 Model verification
Model testing was conducted to assess the realism of model’s structural behavior. This involved several tests, such as the boundary appropriateness test, extreme conditions test, reality test, dimensional consistency testing, sensitivity and robustness test, etc. In this paper, we will focus on the reality test and robustness test to evaluate the model’s performance.
2.5.1 Model reliability test
This study conducted model testing using historical data from 2010 to 2020 for model testing, and the reliability was evaluated using the mean absolute percentage error (MAPE) based on the error between the actual data and the simulated data, as follows:
where n is the number of real and simulated values, and in this study, n is 10, x and y represent the actual and simulated values, respectively. A MAPE of less than 10% indicates a highly accurate prediction, while a MAPE between 10% and 20% indicates a good prediction. A MAPE between 20% and 50% indicates a feasible prediction, while a MAPE greater than 50% indicates an incorrect prediction.
2.5.2 Model robustness test
Behavioral sensitivity is a critical concern for dynamic simulation models, as it refers to the extent of change in model behavior when varying parameters or altering mathematical descriptors. When the model behavior is insensitive to parameter changes, it is considered to have good robustness. This study focused on testing the model’s robustness by varying the simulation time step.
3 Simulation and result analysis
3.1 Model testing and validation
3.1.1 Model reliability test
In this paper, statistical data from 2010 to 2020 were utilized to verify the accuracy of the Beijing haze prevention and control model. To ensure the credibility of the model’s subsequent simulation results, six indicators were selected for accuracy testing: GDP, total energy consumption (EC), total suspended particulates (TSP), nitrogen oxide emissions (NOx), sulfur dioxide emissions (SO2), and R&D.
The results show that real and simulated values of each test indicator exhibit a consistent trend, and the differences are small (Figure 3). Among them, the MAPE value of TSP is 37.63%, indicating a predictable behavior, while the other variables range between 0% and 20%, indicating a good fit, strong adaptability, and replication of the model. Therefore, the model can effectively reveal the complex relationships among the socio-economic, energy and environment of Beijing (Table 2). Moreover, it can provide the basis for subsequent simulation and prediction.
3.1.2 Model robustness test
To test the robustness of the haze control system model, we shortened the simulation steps to 0.5 year and 0.25-year. As shown in Figure 4, the deviation of key variables remained small after changing the simulation time steps. To further quantify this change, we calculated the average rate of change for the 0.5 and 0.25-year time steps. The average rate of change for key variables was very small (less than 6%), particularly for NOx and population. Changing the time step had little effect on the model’s robustness (Table 3). These results demonstrate that the model is robust.
3.2 Maintaining the status quo
This study formulated five measures based on the internal links of the environment, energy, and socio-economic modules, using the development of Beijing, motor vehicle ownership, R&D funds, living coal consumption, and industrial consumption as regulatory variables to simulate the quantitative impact of haze control strategies on Beijing’s haze development. These measures include increasing scientific and technological input, reducing the number of motor vehicles, reducing living and industrial coal consumption, and a combination policy. To compare the effects of different regulatory variables on governance, the parameters were first kept unchanged, and simulations and predictions were made based on the current development trend of Beijing.
The emissions of major pollutants that cause haze weather have continued to decline, with a relatively large reduction from 2010 to 2016 (a total reduction of 18.4 ten thousand tons), which is closely related to the implementation of various emission reduction laws and regulations in Beijing’s 12th Five-Year Plan. However, from 2016 to 2025, the emissions of SO2, NOx, and TSP continued to decline at lower rates due to policy emission reduction thresholds or a failure to implement policies, resulting in a slowdown in the decline of pollutant emissions. Overall, NOx emissions remain the highest, followed by SO2 and TSP (Figure 5A). In 2017, Beijing’s energy consumption reached its highest level (79.23 million tons) and has since rapidly declined to only 14.2 million tons in 2025 (Figure 6).
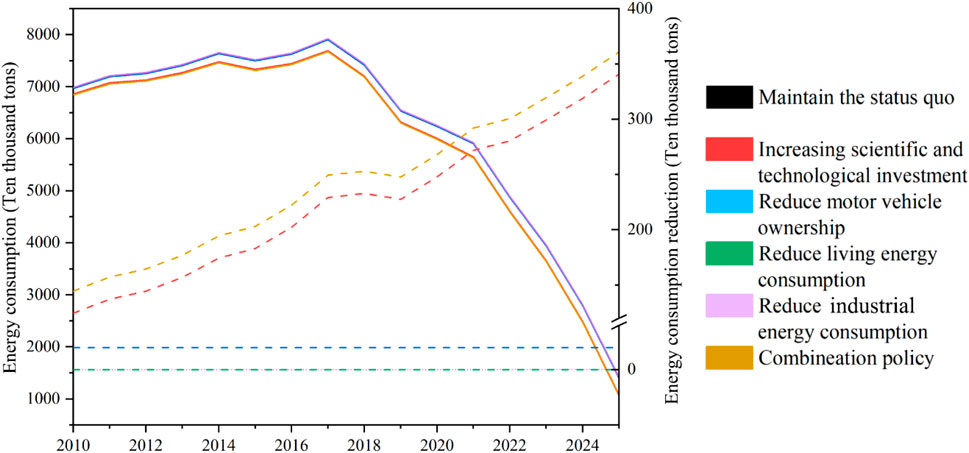
Figure 6. Comparison of energy consumption. Notes: The solid line indicates the energy consumption, and the dashed line indicates the reduction in energy consumption.
3.3 Increasing scientific and technological investment
Increasing the investment in science and technology can effectively reduce the energy consumption per unit of industry output value, thereby reducing the industrial energy consumption and controlling pollutant emissions. This paper characterizes science and technology investment using R&D expenditure as an indicator, and uses an adjustment ratio of 0.002 based on the proportion of R&D to GDP from 2010 to 2020 to test the quantitative effect of increasing science and technology investment on haze control, taking into account the internal relationships and feasibility of the system.
The growth of scientific and technological input leads to a reduction in industrial energy consumption and total energy consumption, resulting in a decrease of 35.72 million tons compared to the status quo in 2025 (Figure 6). In addition, with the increase of scientific and technological input, the trend of pollutant emissions has not changed, but the emissions have decreased. Specifically, the emissions of NOx, SO2 and TSP have decreased by 13.62 thousand tons, 14.67 thousand tons and 9.85 thousand tons, respectively (Figure 5B).
3.4 Reducing motor vehicle ownership
With the rapid development of the urbanization level and social economy, the ownership of private cars continues to increase, and coupled with traffic congestion in Beijing, automobile exhaust emissions have become one of the main sources of haze. However, completely limiting or reducing the growth of motor vehicles would impede meeting the growing demand of people for a better quality of life. Therefore, this study reduces motor vehicle ownership by an average of 15 ten thousand vehicles per year, equivalent to the average growth rate of motor vehicle ownership in Beijing from 2010 to 2020.
By controlling the ownership of motor vehicles, the rate of increase in energy consumption has been directly reduced. By 2025, Beijing’s total energy consumption is expected to decrease by 1.41% compared to maintaining the status quo, resulting in a total reduction of 3.21 million tons of energy consumption during the study period (Figure 6). However, the policy of reducing motor vehicle ownership has not led to a significant decrease in haze pollutant emissions, resulting in a total reduction of only 3,726 tons of the three pollutants from 2010 to 2025 (Figure 5C).
3.5 Decreasing coal consumption
Coal, as the most reliable and secure energy source in China, holds a dominant position in the energy consumption structure. However, it is a highly polluting and emission-intensive energy source. The combustion of coal, particularly in scattered and inefficient processes, coupled with inadequate implementation of environmental protection measures such as dust removal and desulfurization, has resulted in severe air pollution, especially in urban areas. In this study, the proportion of both industrial and residential coal consumption in Beijing was reduced by an equivalent magnitude ratio of 0.01, based on the average decreasing proportion observed from 2010 to 2020.
Reducing coal consumption has a small impact on energy consumption (Figure 6), but it can effectively reduce air pollutant emissions. Adjusting industrial coal consumption by 2025 is projected to result in a decrease of 26.11 thousand tons of SO2, 24.24 thousand tons of NOx, and 17.53 thousand tons of TSP, totaling 67.88 thousand tons (Figure 5D). Compared to industrial coal, adjusting living coal consumption has a relatively smaller effect on reducing haze pollutants, with a total reduction of 27.1 thousand tons during the study period. This includes 10.42 thousand tons of SO2, 9.68 thousand tons of NOx, and seven thousand tons of TSP (Figure 5E).
3.6 Simulation results of combining policies
By increasing R&D investment, reducing motor vehicle ownership, and decreasing coal consumption, effective control of energy consumption can be achieved. As a result, by 2025, Beijing’s energy consumption is projected to decrease to 10.59 million tons of standard coal, representing a 25.78% reduction compared to the level under the status quo. The peak of energy consumption still occurred in 2017 but has since declined by 2.49 million tons of energy consumption (Figure 6).
Furthermore, the combined policy approach has shown significant effects in reducing haze emissions. Compared to maintaining the status quo, the combination policy has resulted in reductions of 45.8 thousand tons of NOx, 49.4 thousand tons of SO2, and 33.1 thousand tons of TSP (Figure 5F).
4 Discussion
4.1 Factors contributing to haze
Although the influence of industrial structure on Beijing’s haze weather is no longer dominant (Tian et al., 2014), the rapid urbanization and industrialization experienced in the past decades have led to substantial coal consumption and severe air pollution (Man et al., 2018; Zhang, L. et al., 2021). According to the Beijing Statistical Yearbook, the proportion of coal consumption has declined from 29.59% in 2010 to 1.5% in 2020. As a result, Beijing’s haze pollution has gradually decreased. Combined with the simulation results of our study, reducing industrial coal consumption was the most effective measure to reduce air pollutant emissions, and living coal consumption should not be neglected (Figure 5). However, as coal consumption has become a lower proportion of total energy consumption, it may no longer be the main factor affecting Beijing’s haze in the future.
Increased science and technology investment is highly efficient in reducing energy consumption (Figure 6) and pollution emissions (Figure 5B). This efficiency is mainly attributed to technological innovation in governance technology, production technology and structural optimization (Yuan and Xie, 2015), involving the development of clean energy (Luo et al., 2014), emission reduction and other areas (Wang and Luo, 2020). These factors represent the primary avenues for mitigating haze pollution in the future.
Furthermore, the contribution of controlling motor vehicle ownership to reducing energy consumption and pollution emissions is relatively limited, partly due to the impact of travel restriction policies on emission reduction (Wagner et al., 2016). On the other hand, On the other hand, the primary factor behind this contribution is the development of new energy vehicles, which has been recognized as one of the most effective approaches to address energy and environmental challenges (Shi et al., 2016; Liu et al., 2019).
This study has certain limitations. First, it mainly discusses the impact of governance measures on haze. It is known that meteorological conditions and atmospheric oxidation are also important factors affecting the formation of haze (Zhang et al., 2023). For instance, the East Asian winter monsoon significantly affects the formation of winter haze in China (Zhao et al., 2021). Additionally, when the atmospheric oxidation capacity is high, oxidants react chemically with pollutants to generate secondary aerosols, which will also increase the concentration of particulate matter (Liu, J. et al., 2023; Wang, Y. et al., 2023). In addition, while the study selected SO2, NOx and TSP as indicators to characterize haze pollution, it is important to recognize the complex and nonlinear transformation of these primary pollutants into secondary pollutants. Therefore, when reducing emissions of a single type of primary pollutant, the improvement of air quality as a comprehensive concept encompassing various levels of primary and secondary pollutants may not be as effective as anticipated.
4.2 Policy effect
When we validated the simulation data using historical data from 2010 to 2020, as shown in Table 2, we found that the simulation accuracy of pollutant emissions as well as energy consumption decreased relative to GDP and R&D, especially TSP emissions. This is due to Beijing’s special political and economic status. Under the support of the central budget, its air pollution control is a political task. The pollutant emission is regulated by policy factors and shows nonlinear relationship. As early as 1998, under the direct direction of the central leadership, Beijing’s air pollution control became a top priority for China’s environmental management (Zhang et al., 2016). For instance, in 2017, Beijing Municipal Environmental Protection Bureau issued the Air Pollution Control Plan of Beijing during the 13th Five-Year Plan Period (2016–2020), due to the delay of policy effects, some causality in the system is disproportionate, resulting in a decrease in the simulation accuracy. By combining Figure 3, we discovered that the simulated values of NOx, SO2 and TSP in 2020 are all more than actual values, especially the simulated value of TSP has a change rate of more than 200% from the actual value, which directly caused its MAPE to exceed 30%, and this situation might attribute to the fact that the model does not consider the influence of government policies. Limited to the characteristics of the system dynamics model itself, the system is impossible to react immediately to the nonlinear policy effects on the emissions reduction. So even if we set the emission coefficient of the pollutants to constant, this simplification will not negatively affect the simulation results of the SD model because the model is more structure-oriented than parameter-oriented (Shen et al., 2009).
4.3 Energy rebound effect
The total energy consumption has a direct impact on the occurrence of haze weather in Beijing. While the application of emissions reduction technology can effectively reduce the energy consumption per unit output and control the haze, there is a risk of an energy rebound effect associated with technological progress in energy consumption reduction. In energy economics, the rebound effect is often defined as an increase in energy consumption due to technological advances (Wagner et al., 2016; Yang and Li, 2017). Technological advances reduce the cost of energy services, leading to increased use of fossil fuels to replace labor and capital in production, partially or completely offsetting the anticipated energy-saving effects. Therefore, it is crucial to consider the energy rebound effect when formulating energy conservation and emission reduction policies (Lin and Zhao, 2016).
Although energy efficiency improvements may contribute to the rebound effect, they remain essential in reducing energy consumption. Thus, regulatory measures should be strengthened to mitigate the potential rebound effect that could undermine the benefits of energy consumption reduction. Previous studies have suggested that increasing carbon taxes (Minsch, 2012), driving energy markets reform, adjusting energy prices (Wang et al., 2016), improving the structure of energy consumption, and reducing coal consumption (Liao and Wang, 2019) can effectively suppress the rebound effect.
4.4 Regional governance
Haze pollution exhibits strong spatial spillover and spatial correlation, rendering unilateral and localized haze control methods ineffective in addressing regional haze pollution comprehensively (Cheng et al., 2017; Li, L. et al., 2019; Wang et al., 2017). Recent studies have suggested frequent trans-regional transport of pollutants between different regions such as the North China Plain (NCP) and Yangtze River Delta (YRD) (Zhang et al., 2023; Zhang, J. et al., 2021). Therefore, trans-regional pollution control is more sustainable to further reduce pollutant concentrations (Yuan and Han, 2019). The air quality management program implemented for the Beijing Olympic Games, which was the first regional mechanism-based air quality management initiative in China, achieved remarkable results (Feng et al., 2024). This management approach encompassed policies such as factory closures, vehicle use restrictions, stringent control of construction site dust, and the prohibition of straw burning. Furthermore, the effectiveness of region-based governance policies was demonstrated during the APEC meeting in Beijing in 2014 and the G20 summit in Hangzhou in 2016. However, regional governance poses challenges due to significant disparities in the level of regional economic development and industrial structure within the Beijing-Tianjin-Hebei region (Ma et al., 2022), necessitating national-level macro-policy control to ensure coordination of benefits and cost-sharing for haze control (Cui et al., 2019).
5 Conclusion and implications
5.1 Main conclusions
Firstly, the haze pollutant emission model developed in this study provides improved simulation capabilities for the Beijing haze pollution system, and the obtained simulation results demonstrate a certain level of reliability.
Secondly, through dynamic simulation analysis of the key variables of the model, it is demonstrated that an appropriate combination of management strategies, such as reducing motor vehicle ownership, increasing science and technology investment and reducing coal emissions, can effectively reduce pollutant emissions while also controlling energy consumption. Specifically, compared to maintaining the status quo, the implementation of these strategies results in significant reductions in NOx, SO2 and TSP emissions by 45.8 thousand tons, 49.4 thousand tons, and 33.1 thousand tons, respectively. Additionally, energy consumption is projected to decrease by 38.93 million tons.
Thirdly, among the single treatment measures, reducing industrial coal consumption is identified as the most effective measure to reduce haze. It is followed by increasing investment in science and technology, reducing living coal consumption and controlling motor vehicle ownership, in that order.
5.2 Policy implications
An effective approach to reducing pollutant emissions is the implementation of a well-balanced combination of policies. To mitigate the energy rebound effect, control haze emissions, improve the atmospheric environment, and ensure sustainable economic development, it is crucial to implement energy price constraints, appropriately adjust carbon taxes, and enact other relevant policies. In the context of preventing and controlling haze pollution in Beijing, strengthening regional collaborative efforts is essential. Firstly, raising public awareness of regional ecological issues is paramount, with the government taking the lead in fostering collaborative governance and gradually establishing a framework involving government, enterprises, and the public. Additionally, considering the significant spatial disparities in economic development and industrial structure among cities in the region, it is imperative to address cost-sharing issues in a scientifically sound manner. Consequently, the government should establish a fair compensation mechanism for Hebei and Tianjin, preventing the breakdown of collaborative governance and promoting the sustainable development of the regional economic environment in alignment with the cost of governance and the economic development of each city.
Data availability statement
The original contributions presented in the study are included in the article/Supplementary Material, further inquiries can be directed to the corresponding authors.
Author contributions
FL: Software, Visualization, Writing–original draft. YL: Conceptualization, Methodology, Writing–original draft. HT: Supervision, Validation, Project administration, Writing–review and editing.
Funding
The author(s) declare that financial support was received for the research, authorship, and/or publication of this article. This research was supported by the Second Tibetan Plateau Scientific Expedition and Research Program (STEP) (2019QZKK0606) and the System Analysis and Decision-making course construction project.
Conflict of interest
The authors declare that the research was conducted in the absence of any commercial or financial relationships that could be construed as a potential conflict of interest.
Publisher’s note
All claims expressed in this article are solely those of the authors and do not necessarily represent those of their affiliated organizations, or those of the publisher, the editors and the reviewers. Any product that may be evaluated in this article, or claim that may be made by its manufacturer, is not guaranteed or endorsed by the publisher.
Supplementary material
The Supplementary Material for this article can be found online at: https://www.frontiersin.org/articles/10.3389/fenvs.2024.1400717/full#supplementary-material
References
Anderies, J. M. (2015). Understanding the dynamics of sustainable social-ecological systems: human behavior, institutions, and regulatory feedback networks. Bull. Math. Biol. 77 (2), 259–280. doi:10.1007/s11538-014-0030-z
Chen, Y., Zhu, Z., and Cheng, S. (2022). Industrial agglomeration and haze pollution: evidence from China. Sci. Total Environ. 845, 157392. doi:10.1016/j.scitotenv.2022.157392
Cheng, Z., Li, L., and Liu, J. (2017). Identifying the spatial effects and driving factors of urban PM2.5 pollution in China. Ecol. Indic. 82, 61–75. doi:10.1016/j.ecolind.2017.06.043
Cui, D., Hao, W. U., and Dianting, W. U. (2019). A review and prospect of regional governance in the Beijing-Tianjin-Hebei region. Prog. Geogr. 38 (01), 1–14. doi:10.18306/dlkxjz.2019.01.001
Dace, E., Muizniece, I., Blumberga, A., and Kaczala, F. (2015). Searching for solutions to mitigate greenhouse gas emissions by agricultural policy decisions--Application of system dynamics modeling for the case of Latvia. Sci. Total Environ. 527-528, 80–90. doi:10.1016/j.scitotenv.2015.04.088
Dong, F., Li, J., Zhang, X., and Zhu, J. (2021). Decoupling relationship between haze pollution and economic growth: a new decoupling index. Ecol. Indic. 129, 107859. doi:10.1016/j.ecolind.2021.107859
Dong, F., Zhang, S., Long, R., Zhang, X., and Sun, Z. (2019). Determinants of haze pollution: an analysis from the perspective of spatiotemporal heterogeneity. J. Clean. Prod. 222, 768–783. doi:10.1016/j.jclepro.2019.03.105
Feng, T., Sun, Y., Shi, Y., Ma, J., Feng, C., and Chen, Z. (2024). Air pollution control policies and impacts: a review. Renew. Sustain. Energy Rev. 191, 114071. doi:10.1016/j.rser.2023.114071
Gan, T., Yang, H., Liang, W., and Liao, X. (2021). Do economic development and population agglomeration inevitably aggravate haze pollution in China? New evidence from spatial econometric analysis. Environ. Sci. Pollut. Res. Int. 28 (5), 5063–5079. doi:10.1007/s11356-020-10847-4
Gao, J., Woodward, A., Vardoulakis, S., Kovats, S., Wilkinson, P., Li, L., et al. (2017). Haze, public health and mitigation measures in China: a review of the current evidence for further policy response. Sci. Total Environ. 578, 148–157. doi:10.1016/j.scitotenv.2016.10.231
Han, F., Xu, J., He, Y., Dang, H., Yang, X., and Meng, F. (2016). Vertical structure of foggy haze over the beijing–tianjin–hebei area in january 2013. Atmos. Environ. 139 (aug), 192–204. doi:10.1016/j.atmosenv.2016.05.030
Hao, Y., Liu, Y., Weng, J.-H., and Gao, Y. (2016). Does the Environmental Kuznets Curve for coal consumption in China exist? New evidence from spatial econometric analysis. Energy 114, 1214–1223. doi:10.1016/j.energy.2016.08.075
Harandi, A. A. H., Valmohammadi, C., and Hosseini, S. H. (2024). Enhancing the intellectual capital of research and development centres using business intelligence: a system dynamics approach. Syst. Res. Behav. Sci. doi:10.1002/sres.3003
Hu, Y., Lin, J., Zhang, S., Kong, L., Fu, H., and Chen, J. (2015). Identification of the typical metal particles among haze, fog, and clear episodes in the Beijing atmosphere. Sci. Total Environ. 511, 369–380. doi:10.1016/j.scitotenv.2014.12.071
Jia, S., Liu, X., and Yan, G. (2019). Effect of APCF policy on the haze pollution in China: a system dynamics approach. Energy Policy 125, 33–44. doi:10.1016/j.enpol.2018.10.012
Joshi, B. V., Vipin, B., Ramkumar, J., and Amit, R. K. (2021). Impact of policy instruments on lead-acid battery recycling: a system dynamics approach. Resour. Conservation Recycl. 169, 105528. doi:10.1016/j.resconrec.2021.105528
Li, C., Ma, X., Fu, T., and Guan, S. (2021). Does public concern over haze pollution matter? Evidence from Beijing-Tianjin-Hebei region, China. Sci. Total Environ. 755 (Pt 1), 142397. doi:10.1016/j.scitotenv.2020.142397
Li, L., Xuemin, L., Jiaoju, G., Xianghua, C., and Jun, W. (2019). Regional differences in spatial spillover and hysteresis effects: a theoretical and empirical study of environmental regulations on haze pollution in China. J. Clean. Prod. 230, 1096–1110. doi:10.1016/j.jclepro.2019.04.248
Li, M., and Mao, C. (2020). Spatial effect of industrial energy consumption structure and transportation on haze pollution in beijing-tianjin-hebei region. Int. J. Environ. Res. Public Health 17 (15), 5610. doi:10.3390/ijerph17155610
Li, Y., Han, Z., Song, Y., Li, J., Sun, Y., and Wang, T. (2023). Impacts of the COVID-19 lockdown on atmospheric oxidizing capacity and secondary aerosol formation over the Beijing-Tianjin-Hebei region in Winter-Spring 2020. Atmos. Environ. 295, 119540. doi:10.1016/j.atmosenv.2022.119540
Li, Z., Shao, S., Shi, X., Sun, Y., and Zhang, X. (2019). Structural transformation of manufacturing, natural resource dependence, and carbon emissions reduction: evidence of a threshold effect from China. J. Clean. Prod. 206, 920–927. doi:10.1016/j.jclepro.2018.09.241
Liao, M., and Wang, Y. (2019). China's energy consumption rebound effect analysis based on the perspective of technological progress. Sustainability 11 (5), 1461. doi:10.3390/su11051461
Lin, B., and Zhao, H. (2016). Technological progress and energy rebound effect in China׳s textile industry: evidence and policy implications. Renew. Sustain. Energy Rev. 60, 173–181. doi:10.1016/j.rser.2016.01.069
Liu, J., Xue, L., Huang, X., Wang, Z., Lou, S., and Ding, A. (2023). Intensified haze formation and meteorological feedback by complex terrain in the North China Plain region. Atmos. Ocean. Sci. Lett. 16 (2), 100273. doi:10.1016/j.aosl.2022.100273
Liu, R., Li, Q., and Zhao, Y. (2017). Analysis of existing problems and improvement schemes for substituting electricity for scattered coal in China. Sustainability 9 (5), 744. doi:10.3390/su9050744
Liu, X., Wang, Z., Sun, X., Zhang, L., and Zhang, M. (2020). Clarifying the relationship among clean energy consumption, haze pollution and economic growth–based on the empirical analysis of China's Yangtze River Delta Region. Ecol. Complex. 44, 100871. doi:10.1016/j.ecocom.2020.100871
Liu, X., and Xia, H. (2018). Empirical analysis of the influential factors of haze pollution in China—based on spatial econometric model. Energy and Environ. 30 (5), 854–866. doi:10.1177/0958305x18813648
Liu, Y., Ren, T., Liu, L., Ni, J., and Yin, Y. (2023). Heterogeneous industrial agglomeration, technological innovation and haze pollution. China Econ. Rev. 77, 101880. doi:10.1016/j.chieco.2022.101880
Liu, Y., Wang, Y., Li, D., and Yu, Q. (2019). Life cycle assessment for carbon dioxide emissions from freeway construction in mountainous area: primary source, cut-off determination of system boundary. Resour. Conservation Recycl. 140, 36–44. doi:10.1016/j.resconrec.2018.09.009
Luo, L., Li, S., and Economics, S. O. (2014). Technological progress,industrial structure and China's industrial carbon emissions. Sci. Res. Manag. 35 (06), 8–13. doi:10.19571/j.cnki.1000-2995.2014.06.002
Ma, S., Li, L., Ke, H., and Zheng, Y. (2022). Environmental protection, industrial structure and urbanization: spatiotemporal evidence from beijing–tianjin–hebei, China. Sustainability 14 (2), 795. doi:10.3390/su14020795
Man, Y., Han, Y., Hu, Y., Yang, S., and Yang, S. (2018). Synthetic natural gas as an alternative to coal for power generation in China: life cycle analysis of haze pollution, greenhouse gas emission, and resource consumption. J. Clean. Prod. 172, 2503–2512. doi:10.1016/j.jclepro.2017.11.160
Minsch, F., Goldblatt Spreng, D., and Goldblatt, D. L. (2012) Lessons for problem-solving energy research in the social sciences, 52. Netherlands: Springer, 273–319. doi:10.1007/978-94-007-2333-7_14
Shang, D., Peng, J., Guo, S., Wu, Z., and Hu, M. (2020). Secondary aerosol formation in winter haze over the Beijing-Tianjin-Hebei Region, China. Front. Environ. Sci. Eng. 15 (2), 34. doi:10.1007/s11783-020-1326-x
Shen, Q., Chen, Q., Tang, B. S., Yeung, S., Hu, Y., and Cheung, G. (2009). A system dynamics model for the sustainable land use planning and development. Habitat Int. 33 (1), 15–25. doi:10.1016/j.habitatint.2008.02.004
Shi, T., Zhang, W., Zhou, Q., and Wang, K. (2020). Industrial structure, urban governance and haze pollution: spatiotemporal evidence from China. Sci. Total Environ. 742, 139228. doi:10.1016/j.scitotenv.2020.139228
Shi, X., Wang, X., Yang, J., and Sun, Z. (2016). Electric vehicle transformation in Beijing and the comparative eco-environmental impacts: a case study of electric and gasoline powered taxis. J. Clean. Prod. 137, 449–460. doi:10.1016/j.jclepro.2016.07.096
Tang, D., Xu, H., and Yang, Y. (2018). Mutual influence of energy consumption and foreign direct investment on haze pollution in China: a spatial econometric approach. Pol. J. Environ. Stud. 27 (4), 1743–1752. doi:10.15244/pjoes/78046
Tang, H. (2019) Systems engineering methods and applications - systems analysis and decision. Beijing: Higher Education Press.
Tian, Y., Govindan, K., and Zhu, Q. (2014). A system dynamics model based on evolutionary game theory for green supply chain management diffusion among Chinese manufacturers. J. Clean. Prod. 80, 96–105. doi:10.1016/j.jclepro.2014.05.076
Wagner, G., Gillingham, K., and Rapson, D. (2016). The rebound effect and energy efficiency policy. Rev. Environ. Econ. policy 10 (1), 68–88. doi:10.1093/reep/rev017
Wang, C., Du, X., and Liu, Y. (2017). Measuring spatial spillover effects of industrial emissions: a method and case study in Anhui province, China. J. Clean. Prod. 141, 1240–1248. doi:10.1016/j.jclepro.2016.09.182
Wang, Q., Zhuang, G., Huang, K., Liu, T., Deng, C., Xu, J., et al. (2015). Probing the severe haze pollution in three typical regions of China: characteristics, sources and regional impacts. Atmos. Environ. 120, 76–88. doi:10.1016/j.atmosenv.2015.08.076
Wang, X., Dong, Z., and Susnik, J. (2023a). System dynamics modelling to simulate regional water-energy-food nexus combined with the society-economy-environment system in Hunan Province, China. Sci. Total Environ. 863, 160993. doi:10.1016/j.scitotenv.2022.160993
Wang, X., and Luo, Y. (2020). Has technological innovation capability addressed environmental pollution from the dual perspective of FDI quantity and quality? Evidence from China. J. Clean. Prod. 258, 120941. doi:10.1016/j.jclepro.2020.120941
Wang, X., Su, Z., and Mao, J. (2023b). How does haze pollution affect green technology innovation? A tale of the government economic and environmental target constraints. J. Environ. Manage 334, 117473. doi:10.1016/j.jenvman.2023.117473
Wang, Y., Chen, S., and Yao, J. (2019). Impacts of deregulation reform on PM2.5 concentrations: a case study of business registration reform in China. J. Clean. Prod. 235, 1138–1152. doi:10.1016/j.jclepro.2019.06.312
Wang, Y., Ma, Y., Yan, C., Yao, L., Cai, R., Li, S., et al. (2023). Sulfur dioxide transported from the residual layer drives atmospheric nucleation during haze periods in beijing. Geophys. Res. Lett. 50 (6). doi:10.1029/2022gl100514
Wang, Y., Tao, S., Gao, W., Ji, D., Wang, L., Li, Y., et al. (2016). The challenge and opportunity on preventing and controlling air pollution of beijing. Bull. Chin. Acad. Sci. doi:10.1093/reep/rev017
WHO (2011) Outdoor air pollution in the world cities. Geneva, Switzerland: World Health Organization.
Wu, W., Zhang, M., and Ding, Y. (2020). Exploring the effect of economic and environment factors on PM2.5 concentration: a case study of the Beijing-Tianjin-Hebei region. J. Environ. Manage 268, 110703. doi:10.1016/j.jenvman.2020.110703
Xiao, S., Dong, H., Geng, Y., Tian, X., Liu, C., and Li, H. (2020). Policy impacts on Municipal Solid Waste management in Shanghai: A system dynamics model analysis. J. Clean. Prod. 262, 121366. doi:10.1016/j.jclepro.2020.121366
Yang, L., and Li, J. (2017). Rebound effect in China: evidence from the power generation sector. Renew. Sustain. Energy Rev. 71, 53–62. doi:10.1016/j.rser.2016.12.111
Yang, S., Duan, F., Ma, Y., Li, H., Ma, T., Zhu, L., et al. (2020). Mixed and intensive haze pollution during the transition period between autumn and winter in Beijing, China. Sci. Total Environ. 711, 134745. doi:10.1016/j.scitotenv.2019.134745
Yi, M., Wang, Y., Sheng, M., Sharp, B., and Zhang, Y. (2020). Effects of heterogeneous technological progress on haze pollution: evidence from China. Ecol. Econ. 169, 106533. doi:10.1016/j.ecolecon.2019.106533
Yuan, G., and Han, J. (2019). Is China's air pollution control policy effective? Evidence from Yangtze River Delta cities. J. Clean. Prod. 220, 110–133. doi:10.1016/j.jclepro.2019.01.287
Yuan, Y. J., and Xie, R. H. (2015). Empirical research on the relationship of industrial agglomeration,technological innovation and environmental pollution. Stud. Sci. Sci. 33 (09), 1340–1347. doi:10.16192/j.cnki.1003-2053.2015.09.007
Zhang, H., Wang, S., Hao, J., Wang, X., Wang, S., Chai, F., et al. (2016). Air pollution and control action in Beijing. J. Clean. Prod. 112, 1519–1527. doi:10.1016/j.jclepro.2015.04.092
Zhang, J., Li, W., Wang, Y., Teng, X., Zhang, Y., Xu, L., et al. (2023). Structural collapse and coating composition changes of soot particles during long-range transport. J. Geophys. Res. Atmos. 128 (19). doi:10.1029/2023jd038871
Zhang, J., Yuan, Q., Liu, L., Wang, Y., Zhang, Y., Xu, L., et al. (2021). Trans-regional transport of haze particles from the North China plain to Yangtze River Delta during winter. J. Geophys. Res. Atmos. 126 (8). doi:10.1029/2020jd033778
Zhang, L., Wang, L., and Chai, J. (2020). Influence of new energy vehicle subsidy policy on emission reduction of atmospheric pollutants: a case study of Beijing, China. J. Clean. Prod. 275, 124069. doi:10.1016/j.jclepro.2020.124069
Zhang, L., Yang, M., Zhang, P., Hao, Y., Lu, Z., and Shi, Z. (2021). De-coal process in urban China: what can we learn from Beijing's experience? Energy 230, 120850. doi:10.1016/j.energy.2021.120850
Zhang, X., Shi, M., Li, Y., Pang, R., and Xiang, N. (2018). Correlating PM2.5 concentrations with air pollutant emissions: a longitudinal study of the Beijing-Tianjin-Hebei region. J. Clean. Prod. 179, 103–113. doi:10.1016/j.jclepro.2018.01.072
Zhao, D., Chen, H., Li, X., and Ma, X. (2018). Air pollution and its influential factors in China's hot spots. J. Clean. Prod. 185, 619–627. doi:10.1016/j.jclepro.2018.02.181
Zhao, Q., and Wang, Y. (2024). The effect of haze pollution on rural-to-urban migrants' long-term residence intentions. Environ. Sci. Pollut. Res. Int. 31 (4), 5896–5911. doi:10.1007/s11356-023-31557-7
Zhao, S., Feng, T., Tie, X., Li, G., and Cao, J. (2021). Air pollution zone migrates south driven by East Asian winter monsoon and climate change. Geophys. Res. Lett. 48 (10). doi:10.1029/2021gl092672
Zhou, H., Jiang, M., Huang, Y., and Wang, Q. (2021). Directional spatial spillover effects and driving factors of haze pollution in North China Plain. Resour. Conservation Recycl. 169, 105475. doi:10.1016/j.resconrec.2021.105475
Keywords: system dynamics model, combination policy, haze management, governance effect, sustainable development
Citation: Lv F, Lu Y and Tang H (2024) Simulating the effect of haze management using system dynamics: a case study of Beijing. Front. Environ. Sci. 12:1400717. doi: 10.3389/fenvs.2024.1400717
Received: 15 March 2024; Accepted: 24 May 2024;
Published: 11 June 2024.
Edited by:
Abdelazim Negm, Zagazig University, EgyptCopyright © 2024 Lv, Lu and Tang. This is an open-access article distributed under the terms of the Creative Commons Attribution License (CC BY). The use, distribution or reproduction in other forums is permitted, provided the original author(s) and the copyright owner(s) are credited and that the original publication in this journal is cited, in accordance with accepted academic practice. No use, distribution or reproduction is permitted which does not comply with these terms.
*Correspondence: Haiping Tang, tanghp@bnu.edu.cn