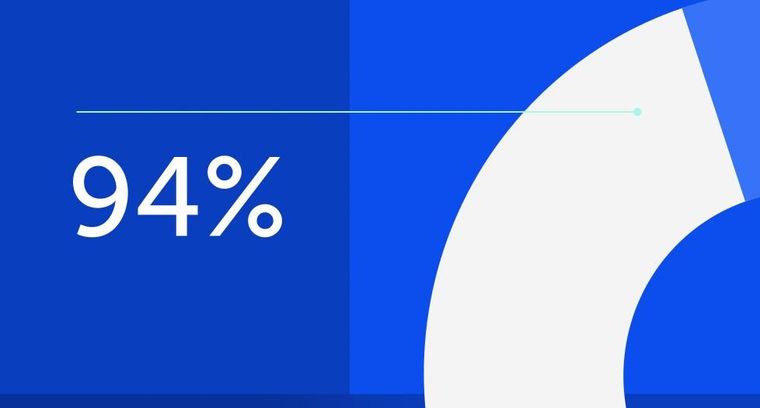
94% of researchers rate our articles as excellent or good
Learn more about the work of our research integrity team to safeguard the quality of each article we publish.
Find out more
ORIGINAL RESEARCH article
Front. Environ. Sci., 06 June 2024
Sec. Environmental Economics and Management
Volume 12 - 2024 | https://doi.org/10.3389/fenvs.2024.1399056
China’s green financial pilot policies (GFPPs) are an important start to build a green financial system in China. It is also an important part of achieving the “dual-carbon” goal. This paper calculates China’s total factor energy efficiency (TFEE), analyzes the impact of GFPPs on TFEE, and explores the conduction mechanism. The results show that the relationship between GFPPs and TFEE in the pilot areas is statistically significant. The GFPP mainly improves the TFEE through the green innovation effect and the optimization of the energy structure. The GFPP has a significant effect on TFEE enhancement in eastern coastal cities, non-old traditional industrial cities, and non-resource-based cities with high initial energy. China should continue to deepen the pilot work of green finance, formulate differentiated green finance policies, and ensure green technology innovation. This study provides empirical evidence for further optimization of green finance and energy efficiency.
In order to cope with global climate change and promote green development, countries around the world have vigorously developed the green economy and promoted the coordinated and sustainable development of the economy, energy, and ecology (Baloch et al., 2021). The use of fossil energy will add a large amount of carbon dioxide (CO2) (Jaffe and Stavins, 1994) and may produce polluting flue gas, threatening the global ecology. In 2020, fossil energy accounted for 83.1% of global energy consumption, while fossil energy accounted for 92% of China’s energy consumption1. To reduce carbon emissions, the Chinese government has announced that it will strive to peak carbon emissions by 2030, achieve carbon neutrality by 2060, and improve the “dual-control” system of energy consumption and total energy consumption. Improving energy efficiency is a crucial part of achieving this goal. Improved energy efficiency not only stimulates economic growth but also helps control carbon emissions and relieve energy shortage and environmental pollution (Yang et al., 2016; Zhang J. et al., 2021; Razzaq et al., 2021; Moslehpour et al., 2023). By comparing the energy supply per unit of gross domestic product (GDP), the world average is 0.171, and the average level of Organization for Economic Co-operation and Development (OECD) countries is 0.102, while China’s is as high as 0.2382. Therefore, China’s energy efficiency urgently needs to be improved.
In order to improve energy efficiency, a major global issue has focused on finance. Finance should give full play to its role in the economy, guide the flow of funds, achieve optimal allocation of resources, and, thus, improve environmental protection (Liu et al., 2020). Green finance can reduce funding support for performing fossil-fuel activities, improve energy efficiency, promote renewable energy development (Khan et al., 2022; Rasoulinezhad and Taghizadeh-Hesary, 2022), and help the flow of funds to environmentally friendly industries (Taghizadeh-Hesary and Yoshino, 2019). In the long run, green finance effectively promotes the sustainable economic development of the economy and realizes the double dividend of the environmental economy (Afzal et al., 2022; Wang and Wang, 2022). China proposed a strategy to build the green finance system in September 2015 and selected pilot areas to establish a green finance reform and innovation pilot zones in June 2017. Since then, China’s green finance policies have officially developed. Although the implementation of China’s green financial reform policy is short, green finance, as a powerful tool to achieve the dual-carbon target, has received widespread attention in China (Li et al., 2021; Lee and Lee, 2022). However, there is still an imperfect supervision and legal environment at present (Volz, 2018), the bond market participation lacks diversity, and the development of green finance in China is not mature. Therefore, whether the policy of China’s green finance reform and innovation pilot zone can reap the dual dividends of the environment and economy and achieve the expected goals of the policy still needs in-depth research. This article takes China’s green finance reform and innovation pilot zone as a quasi-natural experiment and examines the effect and path of the total factor energy efficiency (TFEE) in order to provide reliable experience in the improvement of China’s green financial system and energy efficiency.
The marginal contributions of this paper are as follows: first, this study focuses on China’s green financial pilot policies (GFPPs) and tests the impact of GFPPs on urban-level TFEE, which has not been previously addressed by scholars, and fills the research gaps. Second, in terms of methods, this article uses urban night-light data to decompose the total energy consumption of the provincial-level energy to the prefecture-level cities, realizing the calculation of TFEE at the prefecture-level city level. Third, compared with previous studies on environmental regulation pathways that have focused on innovation and industrial structure upgrading (Zhang et al., 2022), this paper not only verifies the innovation effect but also explores the optimization effect of the energy structure, providing a new perspective for improving energy efficiency. By analyzing the impact of China’s GFPP on TFEE, this article opens the “black box” between the two, providing a new perspective for improving energy efficiency.
The remaining of this article is structured as follows: Section 2 provides a review on green finance and TFEE; Section 3 shows the policy background of GFPPs and theoretical analysis; Section 4 details the data and models; Section 5 displays the regression results and analysis; and Section 6 presents the conclusion and policy recommendations.
Through green credit, green insurance, and green funds, green finance provides financial support for environmental projects and industries to achieve their sustainable development goals (Dörry and Schulz, 2018). Scholars have analyzed the role of green finance in sustainable development from the macro-level. Existing research focuses on multiple countries, confirming that green finance drives the flow of funds to low-carbon projects and innovative technologies, assists financial decision-makers in assessing the magnitude of environmental risks, assists in the transition to a low-carbon economy, and promotes sustainable economic development (Wang and Wang, 2022; Umar and Safi, 2023). The effect of green finance on enterprises and financial institutions from the micro-perspective is discussed. Green finance encourages financial institutions to allocate financial resources and guide capital flow to the cleaning industry (Liu et al., 2019; Qin et al., 2023), reduce the financing cost of enterprises to carry out low-carbon green reform, and promote its transformation to the clean-up industry (Shi et al., 2022).
Green finance in China has received more and more attention. Taking the Chinese green financial policy as a quasi-natural experiment, scholars have investigated its impact on corporate financing costs (Chen et al., 2021; Shi et al., 2022), green technology innovation (Irfan et al., 2022), and green sustainable development (Zhang S. et al., 2021). In addition, scholars use the entropy value method and principal component analysis method to conduct quantitative analysis of Chinese green finance to assess its impact on total factor productivity and financing efficiency (Li et al., 2021; Jin et al., 2021). The research methods are very diverse.
Energy efficiency research focuses on the measurement and influencing factor analysis. Scholars mostly use two types of measurements. One is the single-factor energy efficiency represented by energy consumption per unit of GDP (Jiang et al., 2020). However, a single efficiency factor cannot reflect the economic development level of Chinese cities under the dual constraints of energy conservation and emission reduction goals (Boyd and Pang, 2000). The second type is the total factor energy efficiency, which takes into account the interaction of various factors and can comprehensively reflect the comprehensive energy-saving and emission reduction capabilities of cities. It is a powerful indicator for measuring the progress of carbon neutrality work (Hong et al., 2022). TFEE mainly uses non-parametric methods represented by data envelopment analysis (DEA). Scholars have used the DEA model to measure the energy efficiency of enterprises at the national, regional, or micro-level (Dan, 2007; Zhu et al., 2018; Bashir et al., 2020; Hong et al., 2022). Factors such as foreign trade (Hering and Poncet, 2014), government macro-control (Wu et al., 2020), internet development (Wu et al., 2021), and human capital (Wang, 2023) have significant impacts on energy efficiency.
With the increasing popularity of the concept of green finance, more and more scholars have begun to delve into the close connection between green finance and energy efficiency. Green finance provides financial support for energy efficiency projects, effectively promoting the improvement of energy utilization efficiency and the innovative development of green technologies (Khan et al., 2022; Moslehpour et al., 2023). For example, financial instruments like green credit and green bonds provide funding for energy efficiency and renewable energy projects (Zhang D. et al., 2021). Furthermore, studies have also explored the impact of green finance policies on energy efficiency. These studies evaluate the effects and mechanisms of policies in promoting energy efficiency improvement by analyzing the green finance policy practices in different countries and regions (Mielnik and Goldemberg, 2002; Liu et al., 2022; Wang et al., 2022). The findings indicate that implementing green finance policies can direct funds toward the energy efficiency sector, encourage technological innovation and industrial upgrading, and ultimately lead to improvements in energy efficiency (Levine, 2005; Lee et al., 2023). Due to the high risk of investment in new energy, the promotion of green finance may lead to a reduction in investment efficiency (He et al., 2019). Therefore, there is uncertainty in the current study about the effect of green finance on energy efficiency.
Table 1 provides a literature review showcasing the relationship between green finance and energy efficiency. Overall, there is no conclusion on whether green finance can effectively promote energy efficiency (Liu et al., 2020). More importantly, there are few studies that focus on the China GFPP to explore the effectiveness of energy efficiency. Green finance has an impact on energy efficiency by generating innovative effects, and other conduction mechanisms between green finance and energy efficiency still need to be explored.
China introduced the “Overall Plan for Ecological Civilization System Reform” in September 2015, prioritizing the establishment of a green financial system as a strategic goal. This plan highlighted the significance of green finance within the national supply-side reform, defining it as economic activities that support environmental improvements, address climate change, and enhance energy resource efficiency. To bolster knowledge and practices in green finance, China selected specific regions in Zhejiang, Jiangxi, Guangdong, Guizhou, and Xinjiang in June 2017 as focused green finance reform and innovation pilot zones. In November 2019, China unveiled the “Overall Plan for the Construction of the Green Financial Reform and Innovation Pilot Zone in Lanzhou New Area, Gansu Province,” designating Lanzhou New Area in Gansu Province as the latest site for green financial reform and innovation experiments.
The Chinese government has implemented various incentive policies to promote green finance and environmental conservation. Financial and tax policies have been strengthened to support green development by providing tax incentives and subsidies for green finance projects. Financial institutions are encouraged to introduce innovative green financial products like green credit, bonds, and funds (Feng and Shen, 2022). Mechanisms supporting carbon emission reduction have been established to fund green finance projects cost-effectively. The government increases support for green initiatives by creating green development funds, attracting private capital, and investing in green ventures. Market mechanisms such as green guarantee funds and insurance are being set up to reduce risks and enhance the creditworthiness of green projects, aiding in their financial sustainability. These policies have driven innovation in China’s green financial products and services, playing a vital role in environmental preservation and sustainable growth (Liu et al., 2019; Lee and Lee, 2022).
Green finance has the characteristics of financial resource allocation function and environmental regulation (Khan et al., 2022). The implementation of green finance optimizes resource allocation and promotes green technology innovation (Irfan et al., 2022). According to the “Porter hypothesis,” environmental regulations can produce an innovative compensation effect. In the context of green financial policy, financial institutions inevitably restrict funds for enterprises and projects that do not meet policy requirements and cause environmental violations. Under the environmental constraints of energy saving and emission reduction, the green financial policy causes high energy and high-polluting enterprises to generate costs and survival pressure, forcing enterprises to carry out technological innovation. Green finance policies can facilitate the flow of funds to green industries and projects, thereby easing the financial constraints of enterprises that meet policy criteria and encouraging them to engage in green innovation (Umar and Safi, 2023). With cost promotion and funding support, companies will choose to improve technology innovation to reduce pollutant emission concentrations (Volz, 2018; Irfan et al., 2022). Under cost pressures and with financial support, companies have taken the initiative to strengthen their green technology innovation efforts. These innovations include improvements in existing production processes and technologies, as well as the innovative development of environmentally friendly technologies to improve energy efficiency (Van den Bergh, 2013; Zhao et al., 2021). Therefore, we propose hypothesis 1.
H1. GFPP enables enterprises to generate a green innovation effect under environmental constraints, cost promotion, and financial support, promoting the improvement of TFEE from the perspective of technological change.
Fossil energy is the main source of carbon emissions. Improving the energy structure and reducing the proportion of fossil energy are important ways to achieve the “dual-carbon” goal (Sun and Chen, 2022). The green financial policy has the threshold for green financing, increasing the financing costs of high-pollution enterprises. In order to meet the financing standards, in addition to selecting technological innovation, enterprises will turn their attention to energy, electricity, and other energy sources such as low-emission pollution, and reduce the proportion of fossil energy. The implementation of the green financial policy can drive the development of the low-carbon energy-saving industry (Barua and Aziz, 2022). High-emission and high-pollution industries are restricted, expanding the market demand for new energy continuously. In addition, green insurance provides risk protection for the optimization of corporate energy structure, promotes new energy companies to expand reproduction, and increases the proportion of clean energy in the market (Lee et al., 2023).
As the main source of energy supply in China, coal has greatly limited the improvement of China’s energy efficiency (Han et al., 2007). The consumption of a large amount of coal leads to increased pollutant emissions, which is not conducive to the improvement of energy efficiency (Liu et al., 2020). Reducing the proportion of fossil fuel usage and promoting the development and utilization of new energy can not only reduce environmental pollution but also help improve energy efficiency. Therefore, China’s GFPP can promote the optimization of the energy structure and improve energy efficiency. Therefore, hypothesis 2 is proposed.
H2. GFPP increases the threshold for industrial financing and reduces the development risks of the new energy industry, resulting in changes in the energy structure of enterprises and promoting the improvement of TFEE.
The logical analysis of the mechanism verification is shown in Figure 1.
China conducted two batches of green finance pilots in 2017 and 2019, which can be regarded as quasi-natural experiments. In this study, the pilot cities approved by the country are used as the processing group, and the remaining cities are used as the control group to evaluate the impact of GFPPs on TFEE.
The difference-in-differences (DID) method can solve endogenous problems caused by leakage variables, which are widely used in policy assessment (Beck et al., 2010). The model 1 is constructed as follows:
i indicates the city. t represents the year.
This article selects the slacks-based measurement (SBM) model to calculate the TFEE of 280 prefecture-level cities from 2010 to 2020. This model assumes n decision-making units (DMUs). Input variable, desirable output, and undesirable output vectors are
ρ represents efficiency. λ indicates the weight vector.
Due to the lack of energy consumption data, this article uses night-light data for the simulation measurement of variables to obtain prefecture-level city energy consumption data. This method is widely used in economics research (Hong et al., 2022). The brighter the night light, the more economic activities at night, which means that the economy is relatively developed and with more energy consumption (Zhang et al., 2023). This method adopts a linear model without interception items to decompose the provincial energy consumption data according to the night-light data to various cities.
The policy pilot dummy variable Post×Treat is the core explanatory variable. When urban i starts with green finance pilots in the t year, Post×Treat in the t year and later years is all 1; otherwise, it is 0. This article intends to use all the 11 pilot cities as the experimental group. Due to the significant data missing in Changji and Hami in Xinjiang Province, this article eventually takes nine cities in Quzhou, Huzhou, Guangzhou, Guiyang, Anshun, Nanchang, Jiujiang, Karamay, and Lanzhou as the experimental group, and the remaining 271 cities as the control group.
In order to control the possible impact of other factors and alleviate endogenous problems, this article avoids the input and output indicators when selecting the control variables as much as possible. The control variables and the reasons for their selection are as follows: the level of economic development—a higher level of local economic development is conducive to improving energy efficiency; the level of opening to the outside world—in order to avoid the pressure of environmental regulations in their own countries, foreign industries usually move their heavily polluting industries to other countries and regions with weak environmental regulations. This can have a significant impact on the economy, environment, and energy efficiency of the relocating country (Feng and Liang, 2022); the degree of government regulation—over-regulation can be detrimental to market development, lead to irrational resource allocation, and make energy less efficient to use; the level of human capital—energy efficiency is influenced by the quality of human capital, which determines the level of innovation in firms; and the level of digitalization—digital technology has important applications in generating, transmitting, and storing energy. In addition, the control variables include the level of industrialization, the level of consumption power, and the level of scientific and technological inputs. Control variable symbols and definitions are shown in Table 3.
This article selects prefecture-level cities from 2010 to 2020 as the research sample. Cities with less data lack are supplemented by the interpolation method, and cities with more missing data are deleted. Considering the model optimization, this article eliminates four municipalities, namely, Beijing, Tianjin, Shanghai, and Chongqing. This article deletes cities that have been revoked or newly built during the period of 2010–2020. In terms of foreign data, this article uses the average exchange rate of the US dollar exchange for Chinese yuan. All indicators involved in price factors have been reduced to unchanged prices based on 2010. The original data are obtained from the China Statistical Yearbook, Chinese City Statistical Yearbook, and Chinese Energy Statistical Yearbook. Table 4 shows descriptive statistics of the main variables.
The benchmark regression results are shown in Table 5. Column (1) shows the results of controlling the urban individual-fixed effect and time-fixed effect. The policy interaction coefficient is significantly positive at a significance level of 5%. Column (2) shows the results of adding the control variables. The policy interaction coefficient is significantly positive at the 1% significance level, showing that GFPPs can effectively improve the TFEE in pilot areas.
The DID model requires the processing group and the control group to meet the parallel trend assumption, that is, the TFEE change trends of the two are consistent before the implementation of GFPPs. This article adopts the tailing treatment method, sums the samples of the first seven phases of the policy implementation, and sets the seventh phase of the policy implementation as the base year. The parallel trend test of TFEE in the processing group and the control group is conducted, and the results are shown in Figure 1. Before the GFPP execution year, the coefficient of TFEE is around 0 and does not reject 0 in the 95% confident interval, which is in line with the parallel trend assumption.
The results from the dynamic perspective are shown in Figure 2 and Table 6. Before the implementation of GFPPs, the coefficient of the interaction item is not significant, indicating that the TFEE of the pilot cities did not change significantly before the policy implementation. In the year of policy implementation, the TFEE in pilot areas improved significantly. Every year after policy implementing, the interaction coefficients are significantly positive at a significance level of 5%, indicating that the GFPP has a positive impact on the TFEE in the pilot cities.
In order to verify the robustness of the results, this paper uses the super-SBM method to calculate TFEEnew and unit GDP energy consumption (PE) (ton standard coal/10,000 yuan) to replace the TFEE for regression analysis. In column (1) of Table 7, the interaction coefficients are still positive at a significance level of 5%. In column (2) of Table 7, the interaction coefficients are significantly negative at 5%, indicating that GFPPs can reduce PE and improve the TFEE. The results after replacing the energy efficiency indicators are consistent with those of the benchmark regression. This also verifies the robustness of our conclusions.
During the samples of empirical tests, China also introduced a series of related policies to improve the environment. In order to accurately and effectively identify whether the improvement in energy efficiency is caused by the GFPP, this paper controls the influences of other policies on the results. Since the time of the pollution emission transaction pilot policy and carbon emission transaction pilot policy is relatively close, Column (3) of Table 7 shows the regression results of excluding the pilot cities in the model. The interaction coefficient is still significant at a significance level of 5%. Column (4) of Table 7 excludes the impact of environmental information-disclosure policies in the model, and the results are still significant. Therefore, the conclusion that GFPPs can promote the improvement in the TFEE in pilot cities is stable.
In order to solve the error caused by the endogenous problem in the regression, this article uses the propensity score matching (PSM) method to match the sample of the experimental group with the control group sample. This paper uses the nuclear matching method and selects the government regulation level, human capital level, economic development level, industrialization level, level of opening to the outside world, and scientific input level as the matching partition variables. The results are shown in Figure 3. After matching, all collaborative variables are closer to 0 than before processing, and the deviation is significantly reduced. As shown in Figure 4, the scores of the matching experimental group and the control group tend to be closer, indicating that there is no significant difference in the observations after matching, and the matching results are valid. The results after matching are shown in column (5) of Table 7. The coefficient of the interaction item is still significant at a significance level of 1%, and the results show that GFPPs can significantly improve the TFEE of pilot cities. This conclusion is the same as the benchmark regression results and passed the robust test.
In order to test whether the changes in the trend of the processing group and the control group after the intervention of the policy are affected by other policies or random factors, this paper conducts the placebo test by the fictional treatment group. This article conducts placebo test 500 more times than in the original sample. Nine prefecture-level cities are randomly selected as the processing group for executive policy, and the remaining cities are used as the control group to re-analyze the regression analysis. The interaction coefficient and corresponding p statistics are obtained. Figure 5 shows the nuclear density distribution of the interaction coefficient. The interaction coefficient mostly falls to the left of the reference regression coefficient. The average value of the interaction items coefficient is 0.003, which is less than the benchmark regression result value of 1.038. The policy effect of the green finance pilot shows obvious location orientation and has the most significant effect on the energy efficiency improvement of the green financial pilot cities.
This article studies the heterogeneous impact of GFPPs on the TFEE in pilot cities in different regions based on the geographical location of the eastern, central, and west3 and coastal and inland areas4. The results are shown in Table 8. The GFPP plays a significant role in promoting the improvement of the TFEE in the eastern cities and coastal pilot cities and has no significant effect on TFEE improvement in pilot cities in the central cities and western regions. The effect of the TFEE of the inland pilot cities is not as high as that in the coastal pilot cities. The possible reason is that the economically developed cities are mainly concentrated in the eastern coastal areas, with strong financial strength. Improving the level of innovation and improving the energy structure can enhance the TFEE. The eastern region is rich in resources and has relatively complete institutional support, which will not have a great impact on energy efficiency.
In order to verify the heterogeneity in geographical locations caused by economic development, this article divides sample cities into economically developed cities and economically underdeveloped cities based on the average value of the per capita GDP in 2010, and the results are shown in columns (1) and (2) of Table 9. The significances of coefficients of economically developed cities are significantly higher than those of economically underdeveloped cities, indicating that the GFPP has a greater impact on the TFEE in economically developed cities. The reason may be that economically developed regions have stronger funds and innovative strength, can more effectively promote the development of the local green financial market, and significantly improve the TFEE (Wang and Wang, 2022).
The level of initial energy efficiency is different, which may lead to the difference in environmental regulation costs, so the degree of policy influence will show differences. In this article, the sample cities are divided into high-initial efficiency cities and lower-initial efficiency cities based on the average value of the TFEE in 2010. The heterogeneity test is performed, and the results are shown in columns (3) and (4) of Table 9. High-initial efficiency cities are affected by GFPPs at a significance level of 1%, and cities with lower initial energy efficiency have lower significance. This may be that cities with high initial energy efficiency are mainly concentrated in developed cities in the eastern region, with a high level of economic and financial development, and can more effectively develop green financial policies and promote the improvement of energy efficiency in pilot areas.
In this article, 280 sample cities are divided into 74 old industrial base cities and 206 non-old industrial base cities. The regression results are shown in columns (1) and (2) of Table 10. The coefficients of non-old industrial base cities are significantly positive at a significance level of 1%, and the coefficient of the old industrial base cities is not significant. The GFPP has a more significant impact on the TFEE in pilot cities in non-old industrial base cities.
In this article, 280 sample cities are divided into 113 resource-based cities and 167 non-resource-based cities to examine different resource endowment cities influenced by GFPPs. The results are shown in columns (3) and (4) of Table 10. The interaction coefficient of non-resource-based cities is significantly positive at a significant level of 1%, and the interaction coefficient of resource-based cities is not significant, indicating that the GFPP has a significant impact on the TFEE in pilot cities of non-resource-based cities. Old industrial bases and resource-based cities with high energy-consuming and high-emission industries are usually invested in energy to exchange for outputs. Economic growth also brings a lot of energy waste. In the face of the impact of policies, it is very difficult to transform green finance and industrial greening in these cities in the short term, and the effect of improving energy efficiency in the short term is not as good as other cities.
In order to explore the inherent impact mechanism of the urban TFEE in GFPPs, this article selects urban green innovation and energy structure variables to test the mechanism by observing the impact of core independent variables on intermediary variables. The conventional “three-step” test procedure may cause endogenous problems in the mediating variable, so this paper adopts the approach proposed by Jiang (2022). The results of the first-step analysis are shown in Table 10. The implementation of the GFPP significantly increases green innovation and improves the energy structure. The influence of mediating variables on the TFEE in the second step is described in the theoretical analysis section of this paper. The models are as follows in Eqs 4, 5:
This article uses green patent applications (GINNO) as an intermediary variable and uses the intermediary model to verify the mechanism of the green innovation effect. The results are shown in column (1) of Table 11. The GFPP is significantly positive at the 10% significance level. The GFPP guides financial resources to the green environmental protection industry, which effectively increases its investment in Green Technology and Innovation and improves the level of technological innovation. The GFPP brings great external pressure to high-emission, high-pollution enterprises, prompting them to achieve the upgrading and technological innovation. Technological progress can promote the improvement of the marginal productivity of the production factor, reduce energy consumption loss, reduce pollution emissions, and improve energy efficiency (Zhao et al., 2021). Therefore, the GFPP can improve energy efficiency by promoting green technology innovation. This is consistent with the conclusion obtained by Zhang et al. (2022).
This article uses the urban energy structure (EC) as the intermediary variable to verify the energy structure optimization-effect mechanism. The results are shown in column (2) of Table 11. The GFPP is significantly negative at the 10% level. Green finance provides funding for energy structure optimization for pilot areas, reduces the entry threshold of green low-carbon enterprises, and guides industries to reduce the proportion of high-emission energy. This can promote the development of more new energy industries and form a cleaner, low-carbon, and efficient energy industry system to improve the energy efficiency (Liu et al., 2020).
This article takes GFPPs as a quasi-natural experiment and tests the impact of GFPPs on the TFEE in pilot cities. The results show that the GFPP has a significant effect on the improvement of the TFEE in pilot cities. These results are in line with the findings obtained by Zhang (2024). Green financial policies can not only promote the improvement of energy efficiency but also affect the energy efficiency of pilot cities by supporting green innovation and improving the energy structure. The impact of GFPPs on the TFEE of pilot cities has urban heterogeneity. Green finance is more conducive to cities with more developed coastal economies in the eastern coast. The initial energy efficiency of these cities is relatively high, and the policy effects are more significant. The GFPP is more significant for non-resource-based cities and non-old industrial base cities.
The central government should deepen the pilot work of green finance and improve energy efficiency. The pilot areas should focus more on the areas that are conducive to carbon reduction, better promote the transformation of low carbon in the economy and society, and form a good trend of green finance to promote the low-carbon transformation. Governments can focus their policies on the use of clean energy, make funds flow to renewable and low-carbon energy sources, and guide companies to reduce their use of oil and coal. Additionally, local governments should take full account of economic and resource characteristics. For economically developed eastern coastal cities, it is necessary to make full use of the mature financial market system and focus on the optimization of the energy structure to improve energy efficiency. For the inland and western regions with underdeveloped economies, it is beneficial to combine with their own economic and industrial positioning, optimize the construction of environmentally friendly industries, and establish a green financial system with regional characteristics. Green technology should be inspired in terms of financing costs and performance evaluation. It is necessary to pay attention to the leading position of scientific and technological innovation inputs in the improvement of energy efficiency. The government should innovate service methods and enrich green financial products. It is needed to optimize the transparency of the special subsidy mechanism and the reward and punishment mechanism to help leading enterprises innovate.
This study has several limitations. First, due to the unobservability and inaccessibility of the data, the energy estimates in this paper cannot be updated to the latest year, which may have some implications for policy research. Future research can update the data, expand the sample time window, and make policy research more reasonable and effective. Second, this paper studies the impact of GFPPs on the urban TFEE from the prefecture-level city level. Future research can refine the research level and consider the impact of policies from the perspective of enterprises.
The original contributions presented in the study are included in the article/Supplementary Material; further inquiries can be directed to the corresponding author.
SY: conceptualization, data curation, methodology, supervision, and writing–review and editing. SW: formal analysis, investigation, software, validation, and writing–original draft. XC: funding acquisition, project administration, resources, visualization, and writing–review and editing.
The author(s) declare that financial support was received for the research, authorship, and/or publication of this article. The National Natural Science Foundation of China General Program: Research on the Legitimacy Strategies of Platform Enterprises of Business Incubation (Number: 72074058). The National Social Science Fund of China: Research on the Mechanism and Path of the Impact of Digital Trade on China's Industrial Transformation and Upgrading (Number: 21BTJ060).
The authors declare that the research was conducted in the absence of any commercial or financial relationships that could be construed as a potential conflict of interest.
All claims expressed in this article are solely those of the authors and do not necessarily represent those of their affiliated organizations, or those of the publisher, the editors, and the reviewers. Any product that may be evaluated in this article, or claim that may be made by its manufacturer, is not guaranteed or endorsed by the publisher.
The Supplementary Material for this article can be found online at: https://www.frontiersin.org/articles/10.3389/fenvs.2024.1399056/full#supplementary-material
1These figure results are obtained from EI’s 2021 “Statistical Review of World Energy.”
2These figure results are obtained from the 2022 “China Energy Statistical Yearbook.”
3The eastern region includes 11 provinces, namely, Beijing, Tianjin, Hebei, Liaoning, Shanghai, Jiangsu, Zhejiang, Fujian, Shandong, Guangdong, and Hainan. The central region includes 10 provinces, namely, Shanxi, Inner Mongolia, Jilin, Heilongjiang, Anhui, Jiangxi, Henan, Hubei, Hunan, and Guangxi. The western region includes nine provinces, namely, Sichuan, Guizhou, Yunnan, Tibet, Shaanxi, Gansu, Qinghai, Ningxia, and Xinjiang.
4The coastal area includes Shandong, Guangdong, Guangxi, Jiangsu, Hebei, Zhejiang, Hainan, Fujian, and Liaoning. The inland area includes Yunnan, Inner Mongolia, Jilin, Sichuan, Ningxia, Anhui, Shanxi, Xinjiang, Jiangxi, Henan, Hubei, Hunan, Gansu, Guizhou, Shaanxi, Qinghai, and Heilongjiang.
Afzal, A., Rasoulinezhad, E., and Malik, Z. (2022). Green finance and sustainable development in Europe[J]. Econ. research-Ekonomska istraživanja, 35(1): 5150–5163. doi:10.1080/1331677x.2021.2024081
Baloch, M. A., Ozturk, I., Bekun, F. V., and Khan, D. (2021). Modeling the dynamic linkage between financial development, energy innovation, and environmental quality: does globalization matter? Bus. Strategy Environ. 30 (1), 176–184. doi:10.1002/bse.2615
Barua, S., and Aziz, S. (2022) Making green finance work for the sustainable energy transition in emerging economies[M]//Energy-growth nexus in an era of globalization. Elsevier, 353–382.
Bashir, M. A., Sheng, B., Doğan, B., Sarwar, S., and Shahzad, U. (2020). Export product diversification and energy efficiency: empirical evidence from OECD countries. Struct. Change Econ. Dyn. 55, 232–243. doi:10.1016/j.strueco.2020.09.002
Beck, T., Levine, R., and Levkov, A. (2010). Big bad banks? The winners and losers from bank deregulation in the United States. J. finance 65 (5), 1637–1667. doi:10.1111/j.1540-6261.2010.01589.x
Boyd, G. A., and Pang, J. X. (2000). Estimating the linkage between energy efficiency and productivity. Energy policy 28 (5), 289–296. doi:10.1016/s0301-4215(00)00016-1
Chen, Q., Ning, B., Pan, Y., and Xiao, J. (2021). Green finance and outward foreign direct investment: evidence from a quasi-natural experiment of green insurance in China. Asia Pac. J. Manag. 39, 899–924. doi:10.1007/s10490-020-09750-w
Dan, S. (2007). Regional differences in China's energy efficiency and conservation potentials. China and World Econ. 15 (1), 96–115. doi:10.1111/j.1749-124x.2007.00052.x
Dörry, S., and Schulz, C. (2018). Green financing, interrupted. Potential directions for sustainable finance in Luxembourg. Local Environ. 23 (7), 717–733. doi:10.1080/13549839.2018.1428792
Feng, Y., and Liang, Z. (2022). How does green credit policy affect total factor productivity of the manufacturing firms in China? The mediating role of debt financing and the moderating role of environmental regulation. Environ. Sci. Pollut. Res. 29 (21), 31235–31251. doi:10.1007/s11356-021-17984-4
Feng, Y., and Shen, Q. (2022). How does green credit policy affect total factor productivity at the corporate level in China: the mediating role of debt financing and the moderating role of financial mismatch. Environ. Sci. Pollut. Res. 29, 23237–23248. doi:10.1007/s11356-021-17521-3
Han, Z.-Y., Fan, -Y., Jiao, J.-L., Yan, J.-S., and Wei, Y.-M. (2007). Energy structure, marginal efficiency and substitution rate: an empirical study of China. Energy 32 (6), 935–942. doi:10.1016/j.energy.2006.10.008
He, L., Liu, R., Zhong, Z., Wang, D., and Xia, Y. (2019). Can green financial development promote renewable energy investment efficiency? A consideration of bank credit. Renew. Energy 143, 974–984. doi:10.1016/j.renene.2019.05.059
Hering, L., and Poncet, S. (2014). Environmental policy and exports: evidence from Chinese cities. J. Environ. Econ. Manag. 68 (2), 296–318. doi:10.1016/j.jeem.2014.06.005
Hong, Q., Cui, L., and Hong, P. (2022). The impact of carbon emissions trading on energy efficiency: evidence from quasi-experiment in China's carbon emissions trading pilot. Energy Econ. 110, 106025. doi:10.1016/j.eneco.2022.106025
Irfan, M., Razzaq, A., Sharif, A., and Yang, X. (2022). Influence mechanism between green finance and green innovation: exploring regional policy intervention effects in China. Technol. Forecast. Soc. Change 182, 121882. doi:10.1016/j.techfore.2022.121882
Jaffe, A. B., and Stavins, R. N. (1994). Energy-efficiency investments and public policy. Energy J. 15 (2), 43–65. doi:10.5547/issn0195-6574-ej-vol15-no2-3
Jiang, Q., Yang, S., Tang, P., and Bao, L. (2020). Promoting the polluters? The competing objectives of energy efficiency, pollutant emissions, and economic performance in Chinese municipalities. Energy Res. Soc. Sci. 61, 101365. doi:10.1016/j.erss.2019.101365
Jiang, T., and Luo, Z. B. (2022). LOC102724163 promotes breast cancer cell proliferation and invasion by stimulating MUC19 expression. China Ind. Econ. 23 (5), 100–120. doi:10.3892/ol.2022.13220
Jin, Y., Gao, X., and Wang, M. (2021). The financing efficiency of listed energy conservation and environmental protection firms: evidence and implications for green finance in China. Energy Policy 153, 112254. doi:10.1016/j.enpol.2021.112254
Khan, M. A., Riaz, H., Ahmed, M., and Saeed, A. (2022). Does green finance really deliver what is expected? An empirical perspective[J]. Borsa Istanb. Rev., 22(3): 586–593. doi:10.1016/j.bir.2021.07.006
Lee, C.-C., and Lee, C.-C. (2022). How does green finance affect green total factor productivity? Evidence from China. Energy Econ. 107, 105863. doi:10.1016/j.eneco.2022.105863
Lee, C.-C., Wang, F., and Chang, Y. F. (2023). Does green finance promote renewable energy? Evidence from China. Resour. Policy 82, 103439. doi:10.1016/j.resourpol.2023.103439
Levine, R. (2005). Chapter 12 finance and growth: theory and evidence. Handb. Econ. growth 1 (Part A), 865–934. doi:10.1016/s1574-0684(05)01012-9
Li, M., Hamawandy, N. M., Wahid, F., Rjoub, H., and Bao, Z. (2021). Renewable energy resources investment and green finance: evidence from China. Resour. Policy 74, 102402. doi:10.1016/j.resourpol.2021.102402
Liu, H., Yao, P., Latif, S., Aslam, S., and Iqbal, N. (2022). Impact of Green financing, FinTech, and financial inclusion on energy efficiency. Environ. Sci. Pollut. Res. 29, 18955–18966. doi:10.1007/s11356-021-16949-x
Liu, H., Zhang, Z., Zhang, T., and Wang, L. (2020). Revisiting China’s provincial energy efficiency and its influencing factors. Energy 208, 118361. doi:10.1016/j.energy.2020.118361
Liu, R., Wang, D., Zhang, L., and Zhang, L. (2019). Can green financial development promote regional ecological efficiency? A case study of China. Nat. Hazards 95, 325–341. doi:10.1007/s11069-018-3502-x
Mielnik, O., and Goldemberg, J. (2002). Foreign direct investment and decoupling between energy and gross domestic product in developing countries. Energy policy 30 (2), 87–89. doi:10.1016/s0301-4215(01)00080-5
Moslehpour, M., Aldeehani, T. M., Sibghatullah, A., Tai, T. D., Phan, T. T. H., and Ngo, T. Q. (2023). Dynamic association between technological advancement, green finance, energy efficiency and sustainable development: evidence from Vietnam. Econ. Research-Ekonomska Istraživanja 36 (3), 2190796. doi:10.1080/1331677x.2023.2190796
Qin, M., Zhang, X., Li, Y., and Badarcea, R. M. (2023). Blockchain market and green finance: the enablers of carbon neutrality in China. Energy Econ. 118, 106501. doi:10.1016/j.eneco.2022.106501
Rasoulinezhad, E., and Taghizadeh-Hesary, F. (2022). Role of green finance in improving energy efficiency and renewable energy development. Energy Effic. 15, 14–12. doi:10.1007/s12053-022-10021-4
Razzaq, A., Sharif, A., Najmi, A., Tseng, M. L., and Lim, M. K. (2021). Dynamic and causality interrelationships from municipal solid waste recycling to economic growth, carbon emissions and energy efficiency using a novel bootstrapping autoregressive distributed lag. Resour. Conservation Recycl. 166, 105372. doi:10.1016/j.resconrec.2020.105372
Shi, J., Yu, C., Li, Y., and Wang, T. (2022). Does green financial policy affect debt-financing cost of heavy-polluting enterprises? An empirical evidence based on Chinese pilot zones for green finance reform and innovations. Technol. Forecast. Soc. Change 179, 121678. doi:10.1016/j.techfore.2022.121678
Sun, H., and Chen, F. (2022). The impact of green finance on China's regional energy consumption structure based on system GMM. Resour. Policy 76, 102588. doi:10.1016/j.resourpol.2022.102588
Taghizadeh-Hesary, F., and Yoshino, N. (2019). The way to induce private participation in green finance and investment[J]. Finance Res. Lett., 31: 98–103. doi:10.1016/j.frl.2019.04.016
Umar, M., and Safi, A. (2023). Do green finance and innovation matter for environmental protection? A case of OECD economies. Energy Econ. 119, 106560. doi:10.1016/j.eneco.2023.106560
Van den Bergh, J. C. (2013). Environmental and climate innovation: limitations, policies and prices. Technol. Forecast. Soc. Change 80 (1), 11–23. doi:10.1016/j.techfore.2012.08.004
Wang, K. H., Zhao, Y. X., Jiang, C. F., and Li, Z. Z. (2022). Does green finance inspire sustainable development? Evidence from a global perspective. Econ. Analysis Policy 75, 412–426. doi:10.1016/j.eap.2022.06.002
Wang, Y. (2023). Exploring resource endowment and human capital impact on regional energy efficiency in China in the context of COP26. Resour. Policy 81, 103422. doi:10.1016/j.resourpol.2023.103422
Wang, Z., and Wang, X. (2022). Research on the impact of green finance on energy efficiency in different regions of China based on the DEA-Tobit model. Resour. Policy 77, 102695. doi:10.1016/j.resourpol.2022.102695
Wu, H., Hao, Y., and Ren, S. (2020). How do environmental regulation and environmental decentralization affect green total factor energy efficiency: evidence from China. Energy Econ. 91, 104880. doi:10.1016/j.eneco.2020.104880
Wu, H., Hao, Y., Ren, S., Yang, X., and Xie, G. (2021). Does internet development improve green total factor energy efficiency? Evidence from China. Energy Policy 153, 112247. doi:10.1016/j.enpol.2021.112247
Yang, M., Hu, Z., and Yuan, J. (2016). The recent history and successes of China's energy efficiency policy. Wiley Interdiscip. Rev. Energy Environ. 5 (6), 715–730. doi:10.1002/wene.213
Zhang, D., Awawdeh, A. E., Hussain, M. S., Ngo, Q. T., and Hieu, V. M. (2021a). Assessing the nexus mechanism between energy efficiency and green finance. Energy Effic. 14, 85–18. doi:10.1007/s12053-021-09987-4
Zhang, J., Patwary, A. K., Sun, H., Raza, M., Taghizadeh-Hesary, F., and Iram, R. (2021b). Measuring energy and environmental efficiency interactions towards CO2 emissions reduction without slowing economic growth in central and western Europe. J. Environ. Manag. 279, 111704. doi:10.1016/j.jenvman.2020.111704
Zhang, M., Xie, W., and Gao, W. (2022). Have environmental regulations promoted green technological innovation in cities? Evidence from China’s green patents. Plos one 17 (12), e0278902. doi:10.1371/journal.pone.0278902
Zhang, S., Dong, R., Jiang, J., Yang, S., Cifuentes-Faura, J., Peng, S., et al. (2024). Whether the green credit policy effectively promote green transition of enterprises in China? Empirical analysis and mechanism verification. Environ. Res. 244, 117910. doi:10.1016/j.envres.2023.117910
Zhang, S., Wu, Z., Wang, Y., and Hao, Y. (2021c). Fostering green development with green finance: an empirical study on the environmental effect of green credit policy in China. J. Environ. Manag. 296, 113159. doi:10.1016/j.jenvman.2021.113159
Zhang, X., Cai, Z., Song, W., and Yang, D. (2023). Mapping the spatial-temporal changes in energy consumption-related carbon emissions in the Beijing-Tianjin-Hebei region via nighttime light data. Sustain. Cities Soc. 94, 104476. doi:10.1016/j.scs.2023.104476
Zhao, N., Liu, X., Pan, C., and Wang, C. (2021). The performance of green innovation: from an efficiency perspective. Socio-Economic Plan. Sci. 78, 101062. doi:10.1016/j.seps.2021.101062
Keywords: green finance, total factor energy efficiency, green technology innovation, energy structure, China
Citation: Yu S, Wang S, Cheng X and Li L (2024) Green finance and total factor energy efficiency: theoretical mechanisms and empirical tests. Front. Environ. Sci. 12:1399056. doi: 10.3389/fenvs.2024.1399056
Received: 11 March 2024; Accepted: 10 May 2024;
Published: 06 June 2024.
Edited by:
Dingde Xu, Sichuan Agricultural University, ChinaCopyright © 2024 Yu, Wang, Cheng and Li. This is an open-access article distributed under the terms of the Creative Commons Attribution License (CC BY). The use, distribution or reproduction in other forums is permitted, provided the original author(s) and the copyright owner(s) are credited and that the original publication in this journal is cited, in accordance with accepted academic practice. No use, distribution or reproduction is permitted which does not comply with these terms.
*Correspondence: Xiaolan Cheng, ODc1MDc4NzYyQHFxLmNvbQ==
Disclaimer: All claims expressed in this article are solely those of the authors and do not necessarily represent those of their affiliated organizations, or those of the publisher, the editors and the reviewers. Any product that may be evaluated in this article or claim that may be made by its manufacturer is not guaranteed or endorsed by the publisher.
Research integrity at Frontiers
Learn more about the work of our research integrity team to safeguard the quality of each article we publish.