- 1State Key Laboratory of Water Environment Simulation, School of Environment, Beijing Normal University, Beijing, China
- 2Advancing Systems Analysis (ASA) Program International Institute for Applied Systems Analysis, Laxenburg, Austria
- 3Changsha Social Laboratory of Artificial Intelligence, School of Science, Hunan University of Technology and Business, Changsha, China
- 4School of Economics, Peking University, Beijing, China
- 5School of Business, Jiangnan University, Wuxi, China
As indispensable contributors to the energy and resource sectors, resource-based cities have played a pivotal role in shaping China’s carbon emission landscape. The carbon emission performance of these cities directly impacts the overall carbon footprint of the country, thus highlighting the significance of enhancing carbon emission efficiency within resource-based urban areas to drive nationwide carbon reduction initiatives effectively. The digital economic development strategy presents a promising avenue for improving carbon emission efficiency in resource-based cities. This study employs the super efficiency epsilon-based measure model to assess the carbon emission performance of 103 resource-based cities over the period from 2011 to 2019. Additionally, it utilizes an intermediate effect model to analyse the impact mechanism of digital economic development on carbon emission performance. The findings reveal a substantial 6.3% enhancement in carbon emission performance in resource-based cities attributed to the implementation of the digital development strategy, primarily driven by technological innovation. Furthermore, the study identifies significant dual-threshold effects within economic growth and environmental regulation, shedding light on the complex interplay between these factors. These insights offer valuable guidance for regional carbon reduction endeavors and serve as a valuable supplement to the understanding of the environmental implications of digital economic development in resource-based cities.
1 Introduction
As the world’s largest energy consumer and carbon emitter, China’s efforts to increase carbon emission performance (CEP) were critical to global and domestic green development. Resource-based cities in China are responsible for supplying more than 90% of the coal and oil and more than 70% of the natural gas and crucial mineral resources required for the country’s economic and social development (NBS, 2021). However, as natural resources are depleted, these cities often encounter issues such as industrial structural imbalances and ecological environmental degradation. By 2060, when China aimed to reach its peak carbon emissions, under a normal scenario, 126 resource-based cities in China are expected to peak their carbon emissions at 7.265 billion tons in 2030, accounting for approximately 60% of the country’s total carbon emissions for that year (Li and Dewan, 2017). Therefore, improving the CEP of resource-based cities in China has become an urgent task.
The rapid expansion of digital economic development has profoundly and extensively impacted China’s production and daily life (Goldfarb and Tucker, 2019; Solomon and van Klyton, 2020; Pan et al., 2022). China’s digital economy reached a staggering scale of 45.5 trillion yuan, representing 39.8% of the country’s gross domestic product (GDP) in 2021 (CAC, 2022). The number of internet users in China has soared to 1.051 billion, resulting in an impressive internet penetration rate of 74.4% (CAC, 2022). Furthermore, digital economic development has played a pivotal role in propelling the digital transformation of the energy industry (Semeraro et al., 2023), corporate governance (Cui et al., 2022), and quality economic development (Ding et al., 2022; He et al., 2023) while also greatly promoting green and sustainable development (Costa et al., 2023).
Previous studies have explored the impact of digital economic development on energy and the environment (Schulte et al., 2016; Haseeb et al., 2019; Ma et al., 2022; Guo et al., 2023; Hong Nham et al., 2023). On the one hand, the influence of digital progress on energy primarily encompasses energy consumption (Salahuddin and Alam, 2016; Lange et al., 2020; Ren et al., 2021; Zheng and Wang, 2021), energy transition (Shahbaz et al., 2022), energy security (Lee C-C. et al., 2022), and energy sustainability (Wang et al., 2022b). On the other hand, the influence of digital economic advancement on emission reduction predominantly encompasses carbon emissions (Paschou et al., 2020; Ahmed and Le, 2021; Xu et al., 2021; Dong et al., 2022; Dwivedi et al., 2022), environmental quality (Xu et al., 2022), and air pollution (Wu L. et al., 2023). While these studies emphasized the ecological and environmental impacts of digital economic development, they did not consider the coupled relationship between economic growth and the environment, with emission performance being the optimal manifestation of this coupling. Additionally, these studies have yet to consider the digital economic development status of resource-based cities and its impact on carbon emissions.
The significance of researching resource-based cities in the context of carbon emission performance (CEP) cannot be overstated. On the one hand, as vital suppliers of energy and resources, these cities directly impact national carbon emission levels (Liao et al., 2022). Enhancing CEP not only contributes to local environmental improvements but also catalyses nationwide carbon reduction efforts. On the other hand, improving CEP in resource-based cities facilitates the efficient utilization of resources and the optimization of energy structures, leading to reduced energy consumption and environmental pollution. This dual benefit aligns with the goals of sustainable economic development and environmental protection (Gu et al., 2022).
Examining the influence of technological innovation on CEP in resource-based cities within the framework of a digital economic development strategy is crucial. First, the evolution of the digital economy disrupts traditional energy consumption patterns, thereby shaping the energy landscape and affecting CEP. Second, the digital economy expands the horizons of CEP research by providing new avenues and methodologies for improvement. Digital technologies enhance energy utilization efficiency and reduce unnecessary energy consumption, thereby positively impacting CEP.
Furthermore, investigating the impact of technological innovation on CEP offers valuable insights for policymakers. By understanding the interplay between digital advancements and CEP, policymakers can formulate scientifically sound energy policies and carbon emission control strategies. Finally, these insights are essential for navigating the transition towards a more sustainable and technologically driven energy future, ensuring the harmonious coexistence of economic growth and environmental preservation.
Therefore, the objective of this study was to improve CEP from the perspective of digital economic development across 103 resource-based cities in China from 2011 to 2019. We employed fixed and mediated effects models to analyse both the direct and indirect impacts of digital economic development on CEP. Furthermore, we conducted robustness tests, including four robustness analysis methods, and analysed threshold effects to uncover potential nonlinear relationships between digital economic development and CEP.
This study contributes to the existing research in the following aspects. 1) Indicator selection: Previous studies have focused predominantly on pollution emission variables, neglecting the coupled relationship between economic growth and environmental pollution (Dong et al., 2022; Wu L. et al., 2023). To address this issue, this study, from a performance perspective, measures the coupling relationship between carbon emissions and economic growth. The super efficiency epsilon-based measure (SE-EBM) model was used to measure CEP. 2) Methodological advancements: Principal component analysis (PCA) is used to construct a comprehensive index of digital economic development, and this study establishes a theoretical framework for understanding the relationship between digital economic development and CEP. This enriches the theoretical and methodological aspects of research on the relationship between digital economic development and environmental issues. 3) Mechanism analysis: Previous studies have seldom considered technological innovation as a mediating variable (He et al., 2021; Khan et al., 2022; Zhang and Liu, 2022) and its impact on the environment. This study underscores the mediating role of technological innovation in the impact of digital economic development on CEP. 4) Heterogeneity: This study constructs a threshold effect model of economic growth and environmental regulation, revealing the influence of digital economic development on economic growth and environmental regulation. This provides insights for further research on the environmental effects of digital economic development.
The logical structure of this study was as follows: Section 2 provides the literature review. Section 3 outlines the methodology employed and describes the data sources utilized. Section 4 presents the results of the empirical analysis, while Section 5 offers conclusions and implications.
2 Literature review
2.1 Carbon emissions in Chinese resource-based cities
As primary suppliers of energy and resources, Chinese resource-based cities directly influence national carbon emission levels. This was crucial for the formulation of carbon reduction policies and the achievement of environmental conservation goals (Li and Dewan, 2017; Wu JX. et al., 2023). Moreover, resource-based cities typically exhibit a significant scale in energy and heavy industries, resulting in potentially elevated carbon emissions, which could significantly impact global climate change and environmental quality (Wan and Liu, 2023). Therefore, conducting research on the CEP of resource-based cities is of great theoretical and practical significance.
The carbon emission situation in Chinese resource-based cities is complex (Pan et al., 2023). On the one hand, most resource-based cities in China have significant carbon emissions that are still in the growth stage and exhibit high spatial correlation and clustering characteristics. Carbon emissions are expected to continue to increase in the near future, but there are differences in the achievement of the 2030 carbon emission peak target under baseline development scenarios across different regions (Mu et al., 2023). On the other hand, due to economic growth and industrialization, certain resource-based cities face high levels of carbon emissions (Qiao et al., 2022). Therefore, exploring the potential pathways through which digital economic development strategies can enhance CEP in Chinese resource-based cities is crucial for the formulation of sustainable and environmentally friendly strategies and measures.
2.2 Impact of digital economic development on carbon emissions
Many studies have examined the positive relationship between digital economic development and carbon emissions reduction (Wang et al., 2019; Wang et al., 2022a; Zhang L. et al., 2022; Wang LL. et al., 2022; Li and Wang, 2022). In contrast, some scholars have argued that digital economic development exacerbates carbon emissions (Ozturk and Ullah, 2022), particularly in China (Zhou et al., 2019; Zhang L. et al., 2022; Wang LL. et al., 2022). Furthermore, an inverted U-shaped correlation between digital economic development and carbon emissions has been reported (Li and Wang, 2022). Despite the divergent findings, certain scholars have posited that digital economic development could promote significant reductions in carbon emissions, mainly through an optimal energy mix that aligns to achieve carbon neutrality (Guo et al., 2022). However, others have noted that digital economic development has substantially increased national carbon emissions (Zhou XY. et al., 2022).
Furthermore, studies have revealed that digital economic development has a pronounced impact on low-carbon sustainable development, particularly in the more economically developed eastern regions (Zhang JN. et al., 2022; Lee and Wang, 2022). Some scholars have highlighted the role of digital finance in advancing environmental sustainability (Ozturk and Ullah, 2022). Finally, some scholars have proposed the use of digital remote sensing technology to monitor the ecological pollution status of the ocean (Chen et al., 2020).
Existing studies have primarily analysed carbon emissions, often neglecting the coupling of environmental pollution and economic growth. Moreover, the literature focusing on resource-based cities seldom considers performance issues. To address this gap, this study took resource-based cities as the research subject, investigated the impact of digital economic development on CEP in these cities, and proposed the following hypotheses:
Hypothesis 1. A digital economic development strategy has a significant positive impact on the CEP of resource-based cities.
2.3 The impact mechanism of digital economic development on carbon emissions
Many studies have shown that digital economic development can exert spillover effects on energy and the environment. Nevertheless, the conclusions were inconsistent (Zhang L. et al., 2022; Shahbaz et al., 2022; Xue et al., 2022). This inconsistency may be due to differences in the data and variables used in different studies. Therefore, indicators of universal significance should be used to reveal the correlation between digital economic development and CEP.
Moreover, existing studies rarely consider the impact of digital economic development on environmental performance, which has resulted in a lack of theoretical support for the relationship between digital economic development and the energy and environmental sectors (Chen et al., 2016; Wurlod and Noailly, 2018; Ajayi and Reiner, 2020; Chakraborty and Mazzanti, 2020; Chen et al., 2020). Therefore, this study explores the impact mechanisms of digital economic development on CEP from a technology innovation perspective. Many scholars have demonstrated that digital economic development is a crucial driver of technological innovation (Apostolov and Coco, 2021; Yang et al., 2022; Radicic and Petkovic, 2023), which has a significant impact on enhancing energy and environmental performance (Ma et al., 2021; Wahab, 2021; Wang and Liu, 2022). Therefore, technological innovation could serve as an intermediary variable in the influence of digital economic development on CEP, which was appropriate. Therefore, we propose the following hypothesis:
Hypothesis 2. Technological innovation has significant mediating effects on the effect of digital economic development on CEP.
Rapid economic development in China has led to significant regional differences in energy consumption, economic growth, and carbon emissions (Zhang W. et al., 2022; Shahbaz et al., 2022). Therefore, improving CEP may have different results due to regional differences. In addition, environmental regulation is a critical factor in achieving carbon neutrality (Ahmed, 2020; Aldieri et al., 2022). An imbalance in environmental regulation could affect CEP. Therefore, regional disparities, economic growth, and environmental regulation heterogeneity may interfere with the impact of digital economic development on CEP, and consequently, these issues require further exploration. Figure 1 depicts the research framework. We also propose the following hypothesis:
Hypothesis 3. The impact of digital economic development on CEP varies across different regions in China.
Hypothesis 4. The correlation between digital economic development and CEP has a threshold effect on economic growth and environmental regulation.
3 Method and data
3.1 Method
3.1.1 Baseline model
In this study, we employed a fixed-effects model to analyse the direct impact of digital economic development on CEP. The rationale behind this choice was that the fixed-effects model could control for time-invariant individual characteristics, thus reducing the influence of confounding variables. We employed digital economic development as the independent variable and CEP as the dependent variable. To reveal improvements in CEP from the perspective of digital economic development, we developed the following model:
where Yit refers to the CEP at city i and time t; DD is the digital economic development;
3.1.2 Panel threshold model
The impact of digital economic development on CEP is influenced by various other factors, resulting in a nonlinear relationship between the two factors. We propose utilizing a threshold model to explore the threshold effect of digital economic development and CEP. As economic growth and environmental regulation vary significantly across different regions (Zhang W. et al., 2022; Shahbaz et al., 2022; Zhou and Li, 2024), they are considered potential threshold variables for revealing the threshold effect of CEP.
By introducing threshold variables, we can better capture the nonlinearity in this relationship and accurately identify the threshold value at which the impact of digital economic development on CEP changes (Zhou and Li, 2022). The use of a threshold effects model was motivated by the desire to reveal potential nonlinear relationships between digital economic development and CEP. Such nonlinear relationships may arise due to critical points or thresholds in economic growth and environmental regulation. Once the threshold variable reaches or surpasses this threshold, its impact on CEP may exhibit nonlinear changes. By employing a threshold effects model, we aim to better understand the complex relationship between digital economic development and CEP and identify potential nonlinear effects, thereby providing policymakers with more precise policy recommendations. We constructed the following model:
where c and
3.1.3 Mediation effect model
Digital economic development has the potential to enhance CEP through increased investment in science and technology innovation. In this study, we designed influence channels specifically targeting scientific input and technological progress. We utilized a mediation effects model to elucidate the pathways through which digital economic development affects CEP. To ensure the accuracy and comprehensiveness of our mechanism analysis model, we employed a professional approach (Lee CC. et al., 2022) as follows:
where M represents the intermediate variable, which includes two types: scientific input (SI) and technological innovation (TI), and the coefficient
3.2 Variables
3.2.1 Dependent variable
The dependent variable in this study was CEP. This variable was a critical index for evaluating the effectiveness of environmental policies. The SE-EBM model has been widely used in the field of environmental ecology because it not only takes into account radial and nonradial distance functions but also allows efficiency values to be greater than 1, making CEP comparable among different cities (Akbari et al., 2020; Jalo et al., 2021; Zhou and Li, 2021). We adopted the SE-EBM model proposed by Tone and Tsutsui (Tone and Tsutsui, 2010), which integrates both radial and nonradial distance functions. The initial model was constructed according to the following equation:
where
The parameter
The model’s input indicators included labor, capital, and energy consumption, while its output indicators included economic development and environmental pollution. The urban working population measured labor, while capital was measured by the sum of current and fixed capital at year-end. Energy consumption was measured by liquefied petroleum gas, natural gas, electricity, and heating. It was converted into standard coal values based on prior research (Ru et al., 2015) (unit: 10,000 tons of standard coal). Economic output was measured by the city’s GDP (unit: 100 million yuan). The environmental outcome was assessed by sulfur dioxide emissions (unit: 10,000 tons) due to the limited availability of carbon emissions and solid waste data at the city level (Bi et al., 2014; Shan et al., 2020; Zhou and Li, 2020; Zhou et al., 2023).
3.2.2 Independent variables
The independent variable in this study was digital economic development, a comprehensive index composed of various elements. According to (Bukht and Heeks, 2017; Li and Wang, 2022; Yi et al., 2022; Zhu and Chen, 2022; Wu L. et al., 2023), digital economic development encompasses five key dimensions: 1) telecom business revenue (measured in ten thousand yuan), 2) computer employment (measured by the number of people employed), 3) internet broadband households (measured in ten thousand households), 4) mobile phone users (measured in 10,000 persons), and 5) the inclusive finance index (measured without units). The PCA method was utilized to simplify and consolidate these dimensions into one index, which is a widely adopted approach for constructing composite indicators (Interlenghi et al., 2017; Pan et al., 2022). Therefore, PCA was adopted to construct a digital economic development index.
3.2.3 Control variables
To mitigate the potential interference of extraneous factors on CEP, we controlled for several relevant variables in this study. Specifically, we included five control variables, namely, 1) population density, which was measured as the ratio of total urban registered population to metropolitan area at year-end (Danish et al., 2020); 2) industrialization, which was measured by the proportion of the GDP of the secondary industry to the total GDP; 3) service industry development, which was calculated as the proportion of tertiary industry added value in GDP; 4) financial development, which was measured as the ratio of urban deposits and loan balance to urban GDP; and 5) energy structure, which was determined using electricity consumption as a share of total energy consumption. We presented the definitions and sources of the variables used in Table 1.
3.3 Data sources
This study utilized panel data encompassing 103 Chinese resource-based cities (see Table A1) from 2011 to 2019. The data sources included the China Urban Statistical Yearbook (CUS, 2021), the National Bureau of Statistics (NBS, 2021), the China Energy Administration (CEA, 2021), and the China Environmental Statistical Yearbook (CES, 2021). The control and dependent variables came from the China Urban Statistical Yearbook and the National Bureau of Statistics. The independent variable was derived from the China Urban Statistical Yearbook, while the environmental pollution variable was sourced from the China Environmental Statistical Yearbook. The results of the statistical analysis of the main variables are summarized in Table 2. The dataset comprised 103 cities spanning 9 years, resulting in a total of 927 observations. To enhance the empirical analysis results, these variables underwent a logarithmic transformation.
4 Results and discussion
4.1 Impact assessment analysis
4.1.1 Direct effect analysis
This study aimed to reveal how to improve CEP from the perspective of digital economic development while controlling for potential issues of serial correlation and heteroscedasticity. We employed a robust standard error estimation equation that was clustered by city and utilized a benchmark regression model.
The results of the direct effect analysis are summarized in Table 3. Columns 1) to 3) present the estimated impact of digital economic development on CEP. The findings indicated that the regression coefficients of LnDD in columns 1) to 3) were all positive. These coefficients were statistically significant at the 1% level and had values of 0.097, 0.068, and 0.063, respectively. This suggested that digital economic development significantly enhanced CEP by 0.063 units. This estimate was consistent with previous studies by (Husaini and Lean, 2022; Shahbaz et al., 2022). The latter analysed the energy transition and found that it increased renewable energy by 0.021 due to digital economic development.
The control variables also produced significant results. The coefficients of the natural logarithm of industrialization (LnSER) and energy structure (LnEL) were positive and statistically significant at the 1% level, suggesting that industrialization and the energy structure promoted CEP. However, no evidence was found to support the role of population density in improving CEP. This finding was inconsistent with the conclusions of several other scholars (Morikawa, 2012). This conclusion was consistent with existing research (Morikawa, 2012). Therefore, Hypothesis 1 was verified.
4.1.2 Mediation effect analysis
Directly analysing the path through which CEP improved was insufficient, and its mediating effect is worth exploring. An intermediary effect model was employed, incorporating scientific input and technological innovation as mediating variables. The study yielded substantial and noteworthy results, which are briefly summarized in Table 4.
Columns 1) and 2) present the estimation results with SI as the mediating variable. In Column 1), the regression coefficient of LnDD was positive, with a value of 0.163, indicating that the digital economy development strategy significantly enhanced SI. Furthermore, in Column 2), the regression coefficients of LnDD and SI were positive, with values of 0.056 and 0.042, respectively, suggesting that SI played a significant mediating role in the impact of the digital economy development strategy on CEP. Additionally, Columns 3) and 4) show the estimation results with TI as the mediating variable. In Column 3), the regression coefficient of LnDD was positive, with a value of 0.347, indicating that the digital economy development strategy significantly enhanced TI. Moreover, in Column 4), both the regression coefficients of LnDD and TI were positive, with values of 0.051 and 0.075, respectively, implying that TI also exerted a significant mediating effect on the impact of the digital economy development strategy on CEP (He et al., 2021; Zhang and Liu, 2022). This conclusion verified Hypothesis 2.
4.1.3 Heterogeneity analysis
The swift expansion of China’s economy has engendered notable regional variances in development, with the eastern region boasting advanced manufacturing and internet industries. In contrast, the central and western areas lag far behind, exacerbating preexisting economic inequality (Herrerias et al., 2016; Guang et al., 2019). To scrutinize these regional heterogeneities, this study partitioned China into eastern, central, and western regions to explore the impact of digital economic development on CEP.
Table 5 indicates that the regression coefficient of LnDD was negative (−0.090) for the samples from the eastern region, suggesting that the digital economy development strategy significantly suppressed CEP in the eastern areas. This could be attributed to the relatively lenient environmental policies and rapid industrial development in the eastern region, which led to persistently high carbon emissions. In contrast, the regression coefficient of LnDD was positive (0.156) for the samples from the central region, indicating that the digital economy development strategy significantly impacted CEP in the central areas. Additionally, the results for the western region showed a positive regression coefficient of 0.082 for LnDD, demonstrating that the digital economy development strategy significantly enhanced CEP in the western region (Guang et al., 2019). These findings suggested that the digital economy development strategy was positively effective in the central and western regions but not in the eastern region, indicating significant heterogeneity among regions. The primary reason for this heterogeneity could be the differences in economic development and the implementation of environmental policies across different regions. These findings lend support to Hypothesis 3.
4.2 Robustness analysis
Robustness analysis contributed to enhancing the effectiveness of hypothesis testing. In quantitative analysis methods, robustness analysis serves as one of the crucial steps. By examining whether there are significant changes in the significance, direction, and magnitude of the coefficients across different model specifications and samples, we can determine the robustness of the impact of the explanatory variables on the dependent variable. Consequently, we conducted three robustness tests: the IV approach, substitution methods, and adjustments to the study period.
4.2.1 IV estimation
Due to the potential correlation between the explanatory variables and the error term in the model, which may lead to inaccurate or misleading estimation results, the use of IV methods could effectively address the issue of endogeneity in regression analysis. An IV was a variable that was highly correlated with the endogenous explanatory variable but uncorrelated with the error term. By employing the IV method, the impact of endogeneity can be eliminated, thus obtaining more accurate estimation results. This study adopted widely used IV methods in energy and environmental economics (Barrera-Santana et al., 2022; Xue et al., 2022). The lagged term CEP was chosen as the first IV (IV1) due to its relation to the independent variable. Additionally, 1984 city telecommunications data were used as IV (IV2) (Nunn and Qian, 2014). This approach considered the impact of telecommunication infrastructure on internet technologies, such as technology level and usage habits. A time-varying variable was employed, and a panel instrument variable was constructed using the interaction term of the number of internet and telephone, which we referred to as “Telephone◊Internet.” Furthermore, we recognized the declining impact of traditional telecommunication tools, such as landline telephones, on economic development with decreasing frequency of use.
The regression coefficients of IV1 and IV2 were positive and significant in both models, indicating the appropriateness of the IV selection, as shown in Table 6. The study addressed the endogeneity problem, and the second-stage regression analysis results showed that the regression coefficient of LnDD was significant at the 1% level. These conclusions were similar to those of the direct effects analysis and greater than those of the baseline regression results, which supported the notion that endogeneity caused a lower impact.
Endogeneity tests showed that digital economy development was endogenous. The LM statistic significantly rejected the original “insufficient identification of IV” hypothesis. Additionally, the Wald F-statistic was greater than that of the Stock-Yogo weak identification test, indicating that the choice of IV was appropriate.
4.2.2 Modifying the method
The substitution method was instrumental in identifying and addressing potential model misspecification issues. When the assumptions of a model do not fully align with the characteristics of actual data, substitution techniques offer a flexible approach to examine whether such mismatches impact the robustness of the results. By comparing model outputs across different substitution methods, we could assess whether the model was overly sensitive to specific methods or function choices, allowing us to adjust the model and enhance its robustness. While the ordinary least squares method is known to have excellent properties when classical assumptions are met, we recognize that the generalized method of moments (GMM) could be valid even when the exact distribution information of random perturbation terms is unavailable (Hashmi and Alam, 2019; Zakari et al., 2022) and when there is heteroskedasticity and autocorrelation of the random perturbation terms that violates classical assumptions. Some scholars have applied GMM to environmental science and economics (Omri and Afi, 2020; Ozturk and Ullah, 2022). Thus, we used this method to conduct a robustness analysis of the impact of digital economic development on CEP.
Table 7 presents the results of the GMM regression technique. Our model tests indicated that all assumptions held, with AR 1) being significant and AR 2) being insignificant, which was consistent with the nature of GMM. Moreover, the Sargan and Hansen tests satisfied the necessary conditions. We found that the regression coefficient of LnDD was 0.059, which is significant at the 1% level. This suggested that digital economic development significantly enhanced CEP, which was consistent with the results obtained from the direct effect analysis. We are confident that these findings ensure the reliability of the conclusions of this study.
4.2.3 Modifying the time
Varying the period of the research sample was conducive to examining whether the model was influenced by specific temporal factors. The model may be affected by various factors, such as policy changes, market fluctuations, or unexpected events during specific time periods, leading to biases in the results. By altering the period of the research sample, we can analyse the extent of these factors’ impact on the model and accordingly adjust the model to enhance its robustness (Vieira et al., 2018). Therefore, we divided the research sample into three time periods (2012–2019, 2013–2018, and 2014–2017) to eliminate the interference of different research cycles on the evaluation results.
Table 8 indicates that in the sample period of 2012–2019, the regression coefficient of LnDD was positively significant, with a value of 0.063, suggesting that the digital economy development strategy significantly promoted CEP. Furthermore, in the sample period of 2013–2018, the regression coefficient of LnDD was also positively significant, with a value of 0.042, implying that the digital economy development strategy significantly improved CEP. Additionally, the results for the sample period of 2014–2017 revealed that the regression coefficient of LnDD was positively significant, with a value of 0.021, indicating that the digital economy development strategy significantly enhanced CEP. These findings suggest that the digital economy development strategy was effective throughout all periods. However, as the research cycle shortens, the impact effect decreases continuously, indicating that the cycle has an influence on the estimation results, and there is significant heterogeneity between different cycles. The most likely reason for this difference was the differences in economic development and market environments across different time periods.
4.3 Threshold effect analysis
This study used a panel threshold model to investigate the nonlinear impact on CEP at various levels of regional economic growth and environmental regulation (Ibrahim and Vo, 2021; Zhou and Li, 2022). Table 9 shows that economic growth and environmental regulation significantly impacted CEP at the single and double thresholds but not at the triple threshold, indicating a double threshold effect.
This study revealed that the impact of digital economic development on CEP varies under different thresholds of economic growth and environmental regulation. Specifically, as the threshold increased, the impact effect gradually changed (Table 10). It was observed that with the increase in the threshold variable, the impact effect of digital economic development also linearly increased, confirming Hypothesis 4. These results are illustrated in Figure 2 and emphasize the importance of considering the role of digital economic development in achieving low-carbon development in resource-based cities.
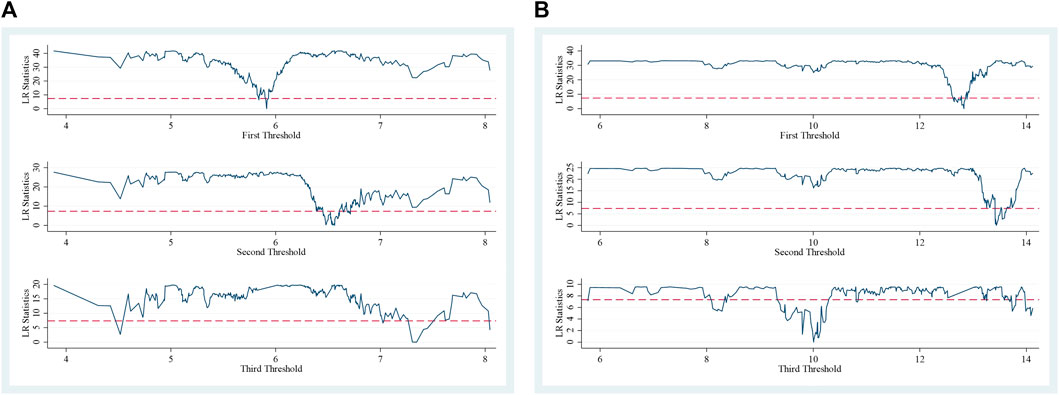
Figure 2. Threshold parameter of threshold variables. Note: a and b refer to the threshold variables economic growth and environmental regulation, respectively; the solid blue line refers to the LR; and the red dotted line indicates the 95% confidence interval.
Therefore, by analysing threshold effects, this study provides valuable insights into the impact of digital economic development on the CEP of resource-based cities.
5 Conclusions and implications
Enhancing the CEP of resource-based cities is a crucial pathway for China to achieve carbon neutrality and foster green development. This study focused on resource-based cities and evaluated the impact of technological innovation on carbon emissions from the perspective of performance under a digital economic development strategy. The study outcomes provide empirical evidence and policy insights for enhancing CEP in resource-based cities, providing a foundation for informed decision-making.
The empirical research revealed that digital economic development improved the CEP of resource-based cities. Specifically, digital economic development significantly enhanced CEP by 6.3%. To enhance the credibility of our conclusions, we conducted four robustness tests, three of which supported our findings. Additionally, we discovered that digital economic development influenced the CEP of resource-based cities by promoting technological innovation. Furthermore, we also observed significant threshold effects of economic development and environmental regulation on this improvement effect.
Based on these insights, some policy recommendations are proposed as follows:
(1) Promotion of Sustainable Digital Economic Development. Resource-based cities should be encouraged to formulate and implement a sustainable digital economic development strategy, emphasizing the application of green, low-carbon, and efficient digital technologies. This includes advancing digital technology innovations that enhance energy efficiency and reduce carbon emissions. Policies supporting the transition of resource-based cities to clean energy should be established, reducing the dependence on high-carbon energy sources. Investments and innovations should be incentivized to promote the adoption of renewable energy and decrease the reliance on traditional energy sources, thereby improving CEP.
(2) Strengthening digital technology training and education. The digital technology skills of urban professionals should be enhanced to ensure their effective adaptation to the development and application of the digital economy. This will contribute to improving the production efficiency and energy utilization efficiency of resource-based cities in the digital economy era. Simultaneously, a comprehensive carbon emission monitoring and data collection system was developed to track and evaluate the actual impact of digital economic development on CEP. This will provide real-time data support for policy formulation and aid in adjusting and optimizing policy measures.
(3) Encouraging Innovation and Research & Development. Fiscal and tax incentives should be provided to encourage enterprises to innovate and conduct research and development in digital technology and clean energy. Collaboration between research institutions and industries should be supported to expedite the dissemination and commercialization of advanced technologies. Additionally, incentive mechanisms should be designed to reward enterprises and cities that achieve significant improvements in CEP through digital economic development. This will help foster a positive competitive environment, encouraging more entities to adopt low-carbon technologies and strategies.
However, there are still some limitations to this study that need to be addressed. For instance, we focused solely on one pollutant when calculating CEP, which may not adequately represent the impact of multiple pollutants. Additionally, our research on the underlying mechanism may also need to consider alternative pathways. Therefore, future research should address these limitations by collecting more indicators, datasets, and channels of mechanism to promote a more comprehensive understanding of the complex interplay between digital economic development and the environment.
Data availability statement
The original contributions presented in the study are included in the article/Supplementary Material, further inquiries can be directed to the corresponding author.
Author contributions
SW: Writing–original draft. AZ: Writing–original draft. YZ: Writing–original draft. GY: Writing–original draft. XP: Writing–original draft, Writing–review and editing.
Funding
The author(s) declare that financial support was received for the research, authorship, and/or publication of this article. This work was supported by the National Natural Science Foundation of China (72304112, 72103022, and 72091511), the Beijing Outstanding Scientist Program (BJJWZYJH01201910027031), the Fundamental Research Funds for the Central Universities (JUSRP123086), the Education Department and Natural Science Foundation of Hunan Province (21B0583, 2022JJ40137, and 2023JJ30196), and a project funded by the China Postdoctoral Science Foundation (2020TQ0048, 2021M700460, and 2022M710421).
Acknowledgments
The authors are grateful to the reviewers for their comments and suggestions, which contributed to the further improvement of this paper.
Conflict of interest
The authors declare that the research was conducted in the absence of any commercial or financial relationships that could be construed as a potential conflict of interest.
Publisher’s note
All claims expressed in this article are solely those of the authors and do not necessarily represent those of their affiliated organizations, or those of the publisher, the editors and the reviewers. Any product that may be evaluated in this article, or claim that may be made by its manufacturer, is not guaranteed or endorsed by the publisher.
References
Ahmed, K. (2020). Environmental policy stringency, related technological change and emissions inventory in 20 OECD countries. J. Environ. Manage. 274, 111209. doi:10.1016/j.jenvman.2020.111209
Ahmed, Z., and Le, H. P. (2021). Linking Information Communication Technology, trade globalization index, and CO(2)emissions: evidence from advanced panel techniques. Environ. Sci. Pollut. R. 28, 8770–8781. doi:10.1007/s11356-020-11205-0
Ajayi, V., and Reiner, D. (2020). European industrial energy intensity: innovation, environmental regulation, and price effects. Energy J. 41, 105–128. doi:10.5547/01956574.41.4.vaja
Akbari, N., Jones, D., and Treloar, R. (2020). A cross-European efficiency assessment of offshore wind farms: a DEA approach. Renew. energ 151, 1186–1195. doi:10.1016/j.renene.2019.11.130
Aldieri, L., Bruno, B., Lorente, D. B., and Paolo Vinci, C. (2022). Environmental innovation, climate change and knowledge diffusion process: how can spillovers play a role in the goal of sustainable economic performance? Resour. Pol. 79, 103021. doi:10.1016/j.resourpol.2022.103021
Andersen, P., and Petersen, N. C. (1993). A procedure for ranking efficient units in data envelopment analysis. Manage Sci. 39, 1261–1264. doi:10.1287/mnsc.39.10.1261
Apostolov, M., and Coco, N. (2021). Digitalization-based innovation - a case study framework. Int. J. Innov. Technol. 18. doi:10.1142/s021987702050025x
Barrera-Santana, J., Marrero, G. A., and Ramos-Real, F. J. (2022). Income, energy and the role of energy efficiency governance. Energy Econ. 108, 105882. doi:10.1016/j.eneco.2022.105882
Bi, G.-B., Song, W., Zhou, P., and Liang, L. (2014). Does environmental regulation affect energy efficiency in China’s thermal power generation? Empirical evidence from a slacks-based DEA model. Energ Policy 66, 537–546. doi:10.1016/j.enpol.2013.10.056
Bukht, R., and Heeks, R. (2017). Defining, conceptualising and measuring the digital economy. SSRN Electron. J. doi:10.2139/ssrn.3431732
CAC (2022). China internet development report 2022, 2022. Beijing: Xinhua News Agency. Available at: http://xinhuanet.com/.
CEA (2021). China energy administration. Available at: http://www.nea.gov.cn/.
CES (2021). Environmental statistical Yearbook, 2021. Beijing: China Statistics Press, National Bureau of Statistics. Available at: http://www.stats.gov.cn/.
Chakraborty, S. K., and Mazzanti, M. (2020). Energy intensity and green energy innovation: checking heterogeneous country effects in the OECD. Struct. Change Econ. Dynam 52, 328–343. doi:10.1016/j.strueco.2019.12.002
Chen, H., Li, W. P., and Xie, X. (2020). Intelligent image monitoring technology of marine environmental pollution information. J. Coast. Res. 112, 1–4. doi:10.2112/jcr-si112-001.1
Chen, Y. C., Han, B. T., and Liu, W. M. (2016). Green technology innovation and energy intensity in China. Nat. Hazards 84, S317–S332. doi:10.1007/s11069-016-2158-7
Costa, F., Frecassetti, S., Rossini, M., and Portioli-Staudacher, A. (2023). Industry 4.0 digital technologies enhancing sustainability: applications and barriers from the agricultural industry in an emerging economy. J. Clean. Prod. 408, 137208. doi:10.1016/j.jclepro.2023.137208
Cui, X., Xu, B., and Razzaq, A. (2022). Can application of artificial intelligence in enterprises promote the corporate governance? Front. Env. Sci-Switz 10. doi:10.3389/fenvs.2022.944467
CUS (2021). China urban statistical Yearbook. Available at: http://www.stats.gov.cn/zs/tjwh/tjkw/tjzl/202302/t20230220_1913734.html.
Danish, , Ulucak, R., and Khan, S. U. D. (2020). Relationship between energy intensity andCO(2)emissions: does economic policy matter? Sustain Dev. 28, 1457–1464. doi:10.1002/sd.2098
Ding, C. H., Liu, C., Zheng, C. Y., and Li, F. (2022). Digital economy, technological innovation and high-quality economic development: based on spatial effect and mediation effect. Sustainability-basel 14, 216. doi:10.3390/su14010216
Dong, F., Hu, M. Y., Gao, Y. J., Liu, Y. J., Zhu, J., and Pan, Y. L. (2022). How does digital economy affect carbon emissions? Evidence from global 60 countries. Sci. Total Environ. 852, 158401. doi:10.1016/j.scitotenv.2022.158401
Dwivedi, Y. K., Hughes, L., Kar, A. K., Baabdullah, A. M., Grover, P., Abbas, R., et al. (2022). Climate change and COP26: are digital technologies and information management part of the problem or the solution? An editorial reflection and call to action. Int. J. Inf. Manage 63, 102456. doi:10.1016/j.ijinfomgt.2021.102456
Goldfarb, A., and Tucker, C. (2019). Digital economics. J. Econ. Lit. 57, 3–43. doi:10.1257/jel.20171452
Gu, Q. F., Wu, Z. Y., and Xie, D. W. (2022). Transformation and development of resource-based cities in China: a review and bibliometric analysis. Front. Env. Sci-Switz 10. doi:10.3389/fenvs.2022.975669
Guang, F. T., He, Y., Le, W., and Sharp, B. (2019). Energy intensity and its differences across China’s regions: combining econometric and decomposition analysis. Energy 180, 989–1000. doi:10.1016/j.energy.2019.05.150
Guo, Q. B., Wang, Y., and Dong, X. B. (2022). Effects of smart city construction on energy saving and CO2 emission reduction: evidence from China. Appl. Energ 313, 118879. doi:10.1016/j.apenergy.2022.118879
Guo, Q. R., Wu, Z., Jahanger, A., Ding, C. C., Guo, B. C., and Awan, A. (2023). The spatial impact of digital economy on energy intensity in China in the context of double carbon to achieve the sustainable development goals. Environ. Sci. Pollut. 30, 35528–35544. doi:10.1007/s11356-022-24814-8
Haseeb, A., Xia, E. J., Saud, S., Ahmad, A., and Khurshid, H. (2019). Does information and communication technologies improve environmental quality in the era of globalization? An empirical analysis. Environ. Sci. Pollut. R. 26, 8594–8608. doi:10.1007/s11356-019-04296-x
Hashmi, R., and Alam, K. (2019). Dynamic relationship among environmental regulation, innovation, CO2 emissions, population, and economic growth in OECD countries: a panel investigation. J. Clean. Prod. 231, 1100–1109. doi:10.1016/j.jclepro.2019.05.325
He, A. P., Xue, Q. H., Zhao, R. J., and Wang, D. P. (2021). Renewable energy technological innovation, market forces, and carbon emission efficiency. Sci. Total Environ. 796, 148908. doi:10.1016/j.scitotenv.2021.148908
He, S., Yang, S., Razzaq, A., Erfanian, S., and Abbas, A. (2023). Mechanism and impact of digital economy on urban economic resilience under the carbon emission scenarios: evidence from China’s urban development. Int. J. Env. Res. Public Health 20, 4454. doi:10.3390/ijerph20054454
Herrerias, M. J., Cuadros, A., and Luo, D. (2016). Foreign versus indigenous innovation and energy intensity: further research across Chinese regions. Appl. Energ 162, 1374–1384. doi:10.1016/j.apenergy.2015.01.042
Hong Nham, N. T., Mai Hoa, T. T., and Ha, L. T. (2023). Influences of digitalization on sustaining marine minerals: a path toward sustainable blue economy. Ocean. Coast. Manage. 239, 106589. doi:10.1016/j.ocecoaman.2023.106589
Husaini, D. H., and Lean, H. H. (2022). Digitalization and energy sustainability in ASEAN. Resour. Conserv. Recy 184, 106377. doi:10.1016/j.resconrec.2022.106377
Ibrahim, M., and Vo, X. V. (2021). Exploring the relationships among innovation, financial sector development and environmental pollution in selected industrialized countries. J. Environ. Manage. 284, 112057. doi:10.1016/j.jenvman.2021.112057
Interlenghi, S. F., de Almeida Bruno, P., Araujo, O. Q. F., and de Medeiros, J. L. (2017). Social and environmental impacts of replacing transesterification agent in soybean biodiesel production: multicriteria and principal component analyses. J. Clean. Prod. 168, 149–162. doi:10.1016/j.jclepro.2017.08.222
Jalo, N., Johansson, I., Kanchiralla, F. M., and Thollander, P. (2021). Do energy efficiency networks help reduce barriers to energy efficiency? -A case study of a regional Swedish policy program for industrial SMEs. Renew. Sust. Energ Rev. 151, 111579. doi:10.1016/j.rser.2021.111579
Khan, M. K., Babar, S. F., Oryani, B., Dagar, V., Rehman, A., Zakari, A., et al. (2022). Role of financial development, environmental-related technologies, research and development, energy intensity, natural resource depletion, and temperature in sustainable environment in Canada. Environ. Sci. Pollut. R. 29, 622–638. doi:10.1007/s11356-021-15421-0
Lange, S., Pohl, J., and Santarius, T. (2020). Digitalization and energy consumption. Does ICT reduce energy demand? Ecol. Econ. 176, 106760. doi:10.1016/j.ecolecon.2020.106760
Lee, C. C., He, Z. W., and Xiao, F. (2022b). How does information and communication technology affect renewable energy technology innovation? International evidence. Renew. energ 200, 546–557. doi:10.1016/j.renene.2022.10.015
Lee, C. C., and Wang, F. H. (2022). How does digital inclusive finance affect carbon intensity? Econ. Anal. Pol. 75, 174–190. doi:10.1016/j.eap.2022.05.010
Lee, C.-C., Yuan, Z., and Wang, Q. (2022a). How does information and communication technology affect energy security? International evidence. Energy Econ. 109, 105969. doi:10.1016/j.eneco.2022.105969
Li, B., and Dewan, H. (2017). Efficiency differences among China’s resource-based cities and their determinants. Resour. Pol. 51, 31–38. doi:10.1016/j.resourpol.2016.11.003
Li, M., Pan, X., and Yuan, S. (2022). Do the national industrial relocation demonstration zones have higher regional energy efficiency? Appl. Energ 306, 117914. doi:10.1016/j.apenergy.2021.117914
Li, Z. G., and Wang, J. (2022). The dynamic impact of digital economy on carbon emission reduction: evidence city-level empirical data in China. J. Clean. Prod. 351, 131570. doi:10.1016/j.jclepro.2022.131570
Liao, Q. H., Li, P., Bin Roosli, R., Liu, S. B., Zhang, X. P., Zhang, C., et al. (2022). Carbon emission characteristics of resource-based cities in China. IJST-T Civ. Eng. 46, 4579–4591. doi:10.1007/s40996-022-00876-z
Ma, Q., Murshed, M., and Khan, Z. (2021). The nexuses between energy investments, technological innovations, emission taxes, and carbon emissions in China. Energ Policy 155, 112345. doi:10.1016/j.enpol.2021.112345
Ma, Q., Tariq, M., Mahmood, H., and Khan, Z. (2022). The nexus between digital economy and carbon dioxide emissions in China: the moderating role of investments in research and development. Technol. Soc. 68, 101910. doi:10.1016/j.techsoc.2022.101910
Morikawa, M. (2012). Population density and efficiency in energy consumption: an empirical analysis of service establishments. Energy Econ. 34, 1617–1622. doi:10.1016/j.eneco.2012.01.004
Mu, J., Wang, J., Liu, B., and Yang, M. (2023). Research progress on the evolution and influencing factors of carbon emission in resource-based cities. J. China Coal Soc. 11, 1–15. doi:10.13225/j.cnki.jccs.2023.0716
NBS (2021). National Bureau of statistics statistical Yearbook 2021. Beijing: National Bureau of Statistics. Available at: https://data.stats.gov.cn/easyquery.htm?cn=E0103.
Nunn, N., and Qian, N. (2014). US food aid and civil conflict. Amer. Econ. Rev. 104, 1630–1666. doi:10.1257/aer.104.6.1630
Omri, A., and Afi, H. (2020). How can entrepreneurship and educational capital lead to environmental sustainability? Struct. Change Econ. Dynam. 54, 1–10. doi:10.1016/j.strueco.2020.03.007
Ozturk, I., and Ullah, S. (2022). Does digital financial inclusion matter for economic growth and environmental sustainability in OBRI economies? An empirical analysis. Resour. Conserv. Recy 185, 106489. doi:10.1016/j.resconrec.2022.106489
Pan, M. J., Zhao, X., Lv, K. J., Rosak-Szyrocka, J., Mentel, G., and Truskolaski, T. (2023). Internet development and carbon emission-reduction in the era of digitalization: where will resource-based cities go? Resour. Pol. 81, 103345. doi:10.1016/j.resourpol.2023.103345
Pan, W., Xie, T., Wang, Z., and Ma, L. (2022). Digital economy: an innovation driver for total factor productivity. J. Bus. Res. 139, 303–311. doi:10.1016/j.jbusres.2021.09.061
Paschou, T., Rapaccini, M., Adrodegari, F., and Saccani, N. (2020). Digital servitization in manufacturing: a systematic literature review and research agenda. Ind. Mark. Manag. 89, 278–292. doi:10.1016/j.indmarman.2020.02.012
Qiao, R. S., Chen, W. K., and Qiao, Y. S. (2022). Sustainable development path of resource-based cities-taking datong as an example. Sustainability-basel 14, 14474. doi:10.3390/su142114474
Qu, C. Y., Shao, J., and Shi, Z. K. (2020). Does financial agglomeration promote the increase of energy efficiency in China? Energ Policy 146, 111810. doi:10.1016/j.enpol.2020.111810
Radicic, D., and Petkovic, S. (2023). Impact of digitalization on technological innovations in small and medium-sized enterprises (SMEs). Technol. Forecast. Soc. Change 191, 122474. doi:10.1016/j.techfore.2023.122474
Ren, S. Y., Hao, Y., Xu, L., Wu, H. T., and Ba, N. (2021). Digitalization and energy: how does internet development affect China’s energy consumption? Energy Econ. 98, 105220. doi:10.1016/j.eneco.2021.105220
Ru, M. Y., Tao, S., Smith, K., Shen, G. F., Shen, H. Z., Huang, Y., et al. (2015). Direct energy consumption associated emissions by rural-to-urban migrants in beijing. Environ. Sci. Technol. 49, 13708–13715. doi:10.1021/acs.est.5b03374
Salahuddin, M., and Alam, K. (2016). Information and Communication Technology, electricity consumption and economic growth in OECD countries: a panel data analysis. Int. J. Elec Power 76, 185–193. doi:10.1016/j.ijepes.2015.11.005
Sang, X., Luo, X., Razzaq, A., Huang, Y., and Erfanian, S. (2023). Can agricultural mechanization services narrow the income gap in rural China? Heliyon 9, e13367. doi:10.1016/j.heliyon.2023.e13367
Schulte, P., Welsch, H., and Rexhäuser, S. (2016). ICT and the demand for energy: evidence from OECD countries. Environ. Resour. Econ. 63, 119–146. doi:10.1007/s10640-014-9844-2
Semeraro, C., Olabi, A. G., Aljaghoub, H., Alami, A. H., Al Radi, M., Dassisti, M., et al. (2023). Digital twin application in energy storage: trends and challenges. J. Energy Storage 58, 106347. doi:10.1016/j.est.2022.106347
Shahbaz, M., Wang, J., Dong, K., and Zhao, J. (2022). The impact of digital economy on energy transition across the globe: the mediating role of government governance. Renew. Sust. Energ Rev. 166, 112620. doi:10.1016/j.rser.2022.112620
Shan, Y. L., Huang, Q., Guan, D. B., and Hubacek, K. (2020). China CO2 emission accounts 2016-2017. Sci. Data 7, 54. doi:10.1038/s41597-020-0393-y
Solomon, E. M., and van Klyton, A. (2020). The impact of digital technology usage on economic growth in Africa. Util. Policy 67, 101104. doi:10.1016/j.jup.2020.101104
Tone, K., and Tsutsui, M. (2010). An epsilon-based measure of efficiency in DEA – a third pole of technical efficiency. Eur. J. Oper. Res. 207, 1554–1563. doi:10.1016/j.ejor.2010.07.014
Vieira, N. D. B., Nogueira, L. A. H., and Haddad, J. (2018). An assessment of CO2 emissions avoided by energy-efficiency programs: a general methodology and a case study in Brazil. Energy 142, 702–715. doi:10.1016/j.energy.2017.10.072
Wahab, S. (2021). Does technological innovation limit trade-adjusted carbon emissions? Environ. Sci. Pollut. R. 28, 38043–38053. doi:10.1007/s11356-021-13345-3
Wan, Q. Y., and Liu, J. (2023). Energy efficiency optimization and carbon emission reduction targets of resource-based cities based on BiLSTM-CNN-GAN model. Front. Ecol. Evol. 11. doi:10.3389/fevo.2023.1248426
Wang, J., Dong, K., Dong, X., and Taghizadeh-Hesary, F. (2022a). Assessing the digital economy and its carbon-mitigation effects: the case of China. Energy Econ. 113, 106198. doi:10.1016/j.eneco.2022.106198
Wang, J., Ma, X., Zhang, J., and Zhao, X. (2022b). Impacts of digital technology on energy sustainability: China case study. Appl. Energ 323, 119329. doi:10.1016/j.apenergy.2022.119329
Wang, L. L., Chen, L. Y., and Li, Y. S. (2022c). Digital economy and urban low-carbon sustainable development: the role of innovation factor mobility in China. Environ. Sci. Pollut. R. 29, 48539–48557. doi:10.1007/s11356-022-19182-2
Wang, Q. Z., and Liu, S. X. (2022). How do FDI and technological innovation affect carbon emission efficiency in China? Energies 15, 9209. doi:10.3390/en15239209
Wang, S. J., Wang, J. Y., Fang, C. L., and Li, S. J. (2019). Estimating the impacts of urban form on CO2 emission efficiency in the Pearl River Delta, China. Cities 85, 117–129. doi:10.1016/j.cities.2018.08.009
Wu, J. X., Nie, X., and Wang, H. (2023a). Curse to blessing: the carbon emissions trading system and resource-based cities’ carbon mitigation. Energ Policy 183, 113796. doi:10.1016/j.enpol.2023.113796
Wu, L., Wan, X. W., Jahanger, A., Li, M. Y., Murshed, M., and Balsalobre-Lorente, D. (2023b). Does the digital economy reduce air pollution in China? A perspective from industrial agglomeration. Energy Rep. 9, 3625–3641. doi:10.1016/j.egyr.2023.02.031
Wurlod, J. D., and Noailly, J. (2018). The impact of green innovation on energy intensity: an empirical analysis for 14 industrial sectors in OECD countries. Energy Econ. 71, 47–61. doi:10.1016/j.eneco.2017.12.012
Xu, L., Fan, M. T., Yang, L. L., and Shao, S. (2021). Heterogeneous green innovations and carbon emission performance: evidence at China’s city level. Energy Econ. 99, 105269. doi:10.1016/j.eneco.2021.105269
Xu, S., Yang, C. Y., Huang, Z. H., and Failler, P. (2022). Interaction between digital economy and environmental pollution: new evidence from a spatial perspective. Int. J. Env. Res. Public Health 19, 5074. doi:10.3390/ijerph19095074
Xue, Y., Tang, C., Wu, H. T., Liu, J. M., and Hao, Y. (2022). The emerging driving force of energy consumption in China: does digital economy development matter? Energ Policy 165, 112997. doi:10.1016/j.enpol.2022.112997
Yang, W., Chen, Q. X., Guo, Q. Q., and Huang, X. T. (2022). Towards sustainable development: how digitalization, technological innovation, and green economic development interact with each other. Int. J. Env. Res. Public Health 19, 12273. doi:10.3390/ijerph191912273
Yi, M., Liu, Y. F., Sheng, M. S., and Wen, L. (2022). Effects of digital economy on carbon emission reduction: new evidence from China. Energ Policy 171, 113271. doi:10.1016/j.enpol.2022.113271
Zakari, A., Khan, I., Tan, D. J., Alvarado, R., and Dagar, V. (2022). Energy efficiency and sustainable development goals (SDGs). Energy 239, 122365. doi:10.1016/j.energy.2021.122365
Zhang, J. N., Lyu, Y. W., Li, Y. T., and Geng, Y. (2022a). Digital economy: an innovation driving factor for low-carbon development. Environ. Impact Assess. Rev. 96, 106821. doi:10.1016/j.eiar.2022.106821
Zhang, L., Mu, R. Y., Zhan, Y. F., Yu, J. H., Liu, L. Y., Yu, Y. S., et al. (2022b). Digital economy, energy efficiency, and carbon emissions: evidence from provincial panel data in China. Sci. Total Environ. 852, 158403. doi:10.1016/j.scitotenv.2022.158403
Zhang, M. L., and Liu, Y. (2022). Influence of digital finance and green technology innovation on China’s carbon emission efficiency: empirical analysis based on spatial metrology. Sci. Total Environ. 838, 156463. doi:10.1016/j.scitotenv.2022.156463
Zhang, W., Liu, X. M., Wang, D., and Zhou, J. P. (2022c). Digital economy and carbon emission performance: evidence at China’s city level. Energ Policy 165, 112927. doi:10.1016/j.enpol.2022.112927
Zheng, J. J., and Wang, X. W. (2021). Can mobile information communication technologies (ICTs) promote the development of renewables? evidence from seven countries. Energ Policy 149, 112041. doi:10.1016/j.enpol.2020.112041
Zhou, A., and Li, J. (2020). Impact of income inequality and environmental regulation on environmental quality: evidence from China. J. Clean. Prod. 274, 123008. doi:10.1016/j.jclepro.2020.123008
Zhou, A., and Li, J. (2021). Investigate the impact of market reforms on the improvement of manufacturing energy efficiency under China’s provincial-level data. Energy 228, 120562. doi:10.1016/j.energy.2021.120562
Zhou, A., and Li, J. (2022). How do trade liberalization and human capital affect renewable energy consumption? Evidence from the panel threshold model. Renew. energ 184, 332–342. doi:10.1016/j.renene.2021.11.096
Zhou, A., and Li, J. (2024). Impact of policy combinations on carbon emission performance: evidence from China. Clean. Technol. Envir. doi:10.1007/s10098-024-02773-7
Zhou, A., Wang, S., and Chen, B. (2023). Impact of new energy demonstration city policy on energy efficiency: evidence from China. J. Clean. Prod. 422, 138560. doi:10.1016/j.jclepro.2023.138560
Zhou, A., Xin, L., and Li, J. (2022a). Assessing the impact of the carbon market on the improvement of China’s energy and carbon emission performance. Energy 258, 124789. doi:10.1016/j.energy.2022.124789
Zhou, X. Y., Zhou, D. Q., Wang, Q. W., and Su, B. (2019). How information and communication technology drives carbon emissions: a sector-level analysis for China. Energy Econ. 81, 380–392. doi:10.1016/j.eneco.2019.04.014
Zhou, X. Y., Zhou, D. Q., Zhao, Z. Y., and Wang, Q. W. (2022b). A framework to analyze carbon impacts of digital economy: the case of China. Sustain Prod. Consump 31, 357–369. doi:10.1016/j.spc.2022.03.002
Zhu, M., Tang, H., Elahi, E., Khalid, Z., Wang, K., and Nisar, N. (2022). Spatial-temporal changes and influencing factors of ecological protection levels in the middle and lower reaches of the yellow river. Sustainability-basel 14, 14888. doi:10.3390/su142214888
Zhu, W. J., and Chen, J. J. (2022). The spatial analysis of digital economy and urban development: a case study in Hangzhou, China. Cities 123, 103563. doi:10.1016/j.cities.2022.103563
Appendix A
Keywords: resource-based cities, digital economic development strategy, super efficiency epsilon-based measure model, carbon emission performance, mediation effect model, carbon neutrality
Citation: Wang S, Zhou A, Zhang Y, Yang G and Peng X (2024) Enhancing carbon emission performance in resource-based cities through technological innovation under China’s digital economic development strategy. Front. Environ. Sci. 12:1397063. doi: 10.3389/fenvs.2024.1397063
Received: 06 March 2024; Accepted: 29 March 2024;
Published: 16 April 2024.
Edited by:
Shaojian Wang, Sun Yat-sen University, ChinaReviewed by:
Amar Razzaq, Huanggang Normal University, ChinaJunze Zhang, Chinese Academy of Sciences (CAS), China
Yafei Li, China Agricultural University, China
Copyright © 2024 Wang, Zhou, Zhang, Yang and Peng. This is an open-access article distributed under the terms of the Creative Commons Attribution License (CC BY). The use, distribution or reproduction in other forums is permitted, provided the original author(s) and the copyright owner(s) are credited and that the original publication in this journal is cited, in accordance with accepted academic practice. No use, distribution or reproduction is permitted which does not comply with these terms.
*Correspondence: Xu Peng, jokexutj@163.com
†These authors have contributedequally to this work