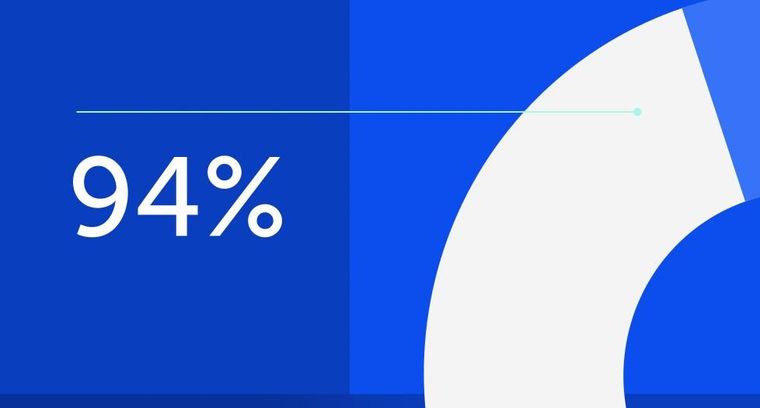
94% of researchers rate our articles as excellent or good
Learn more about the work of our research integrity team to safeguard the quality of each article we publish.
Find out more
ORIGINAL RESEARCH article
Front. Environ. Sci., 05 April 2024
Sec. Social-Ecological Urban Systems
Volume 12 - 2024 | https://doi.org/10.3389/fenvs.2024.1390910
This article is part of the Research TopicDigital Governance, Ecological Resilience and Resident Well-BeingView all 7 articles
In the context of global efforts to alleviate climate change, this study focuses on investigating the significance and impact of low-carbon city pilot policies (LCCPP), a key strategy in the reduction of greenhouse gas emissions. Drawing on data from prefecture-level cities spanning 2004 to 2019, the study explores the potential of LCCPP in enhancing green total factor energy efficiency (GTFEE) employing a multitemporal Difference-in-Differences (DID) model and examines its transmission mechanisms. First, LCCPP is shown to be effective in enhancing GTFEE. Second, heterogeneity testing indicates that the impact of LCCPP on GTFEE is more pronounced in “two control zones”, namely, in developing and mature resource cities. Third, industrial restructuring is identified as the key mechanism through which LCCPP influences GTFEE.
As urbanization and industrialization intensifying, the manufacturing, construction, and electric power/thermal sectors significantly impact China’s energy consumption (Cheng et al., 2020) and are key contributors to its energy consumption. Official data indicate that total energy consumption rose over the 5-year period from 2016 to 2020. The consumption shares of coal stood at 62.2% in 2016 and fell to 56.8% in 2020. Although the proportion of coal has decreased, compared to oil, natural gas, primary power, and other energy sources, coal’s share remains the highest, making the resulting carbon emission issue critically important (Matsumura et al., 2014). In most regions, reduction in carbon emissions entails a decrease in energy consumption (Al-mulali et al., 2012). Furthermore, current energy management is often marked by substantial waste (Fernando and Hor, 2017). According to the National Energy Administration, China’s actual effective energy utilization rates at only 60%–75%, indicating that 25%–40% of energy is wasted. In certain areas, power outages have highlighted issues like overall energy tightening, coal shortages, and challenges in energy utilization. Despite stricter energy efficiency and emission reduction regulations, energy efficiency has not shown significant improvement, a development that is puzzling given that this period marks the zenith of China’s top-down approach to new urbanization. Consequently, enhancing the effective utilization rate of coal and the development of a dual control system for energy consumption are identified as urgent priorities that demand immediate attention.
To address energy waste and carbon emissions, China introduced the concept of peaking carbon emissions and achieving carbon neutrality, known as “dual carbon” goals, at the 75th United Nations General Assembly, mandating that all regions actively develop carbon peaking plans, continuously optimize industrial structures, and set up carbon emission reduction support tools. As a sensitive area of global climate change, extreme weather causes heavy economic losses in China. The “dual carbon” goals delineate pathways to carbon economic development and manifest China’s commitment to addressing global warming problem. In combating global climate change, China has initiated pilot policy for the building of low-carbon cities (LCCPP). The LCCPP aims to foster a low-carbon economy in the city, including low-carbon production and consumption, with the goal of creating a resource-saving, eco-friendly society and a positive, sustainable energy ecosystem. At present, many countries in the international community have adopted eco-city development as a strategic public policy and steer urban growth, amassing considerable successful experiences. For example, eco-towns in the United Kingdom, Portland, the United States, and Singapore boast numerous innovative approaches in physical space planning, ecological technology application, and planning and construction management that serve as valuable references. The reasons for choosing the LCCPP as the research subject include two aspects. First, the LCCPP has become a necessary option in China’s new urbanization process, aligning with the ecological civilization construction mandates to fulfill the carbon peak and neutrality objectives. Second, the LCCPP is characterized by “weak constraints” and “weak incentives”, and necessitating adjustments to align with China’s new economic development and pollution reduction goals.
In light of this, this paper takes the LCCPP, a holistic and comprehensive environmental policy, as an entry point to explore the following questions: Does the LCCPP facilitate enhancement in GTFEE? Is the energy impact of these policies robust within the intricate backdrop of low-carbon initiatives? Furthermore, does the heterogeneity in the construction of “dual control zones” and urban resource categories influence the energy outcomes of the LCCPP? Consequently, this paper focuses on the LCCPP, utilizing panel data from 277 prefecture-level cities spanning 2004 to 2019, and employs a multitemporal DID model to analyze and examine the aforementioned questions.
Climate change is a common challenge facing humankind and requires global cooperation and action. As the world’s largest developing country, China is actively bolstering its national commitments and has set forth carbon peaking and carbon neutrality goals. While carbon peaking and carbon neutrality goals may facilitate a green, low-carbon urban transition (Jefferson, 2018), stark disparities between carbon emission reductions and urban energy efficiency result in diminished energy efficiency and significant energy waste (Babatunde et al., 2021). In 2010, China initiated its first environment pilot policy, exemplified by the LCCPP. Carbon dioxide (CO2) accounts for the highest percentage of greenhouse gases produced by energy consumption (Li et al., 2016). However, the present debate centers on the relationship between low-carbon policies and energy efficiency.
On the one hand, improving energy efficiency reduces carbon emissions (Yao et al., 2015). The most effective long-term strategy for reducing carbon emissions, especially for emerging economies, is to increase the efficiency of energy utilization (Nibedita and Irfan, 2021) or to adjust the economic policy (Abrell et al., 2022; Hancevic and Sandoval, 2022; Song et al., 2023). Because the overall carbon reduction target remains unchanged, it can drive regional energy efficiency policy reform by reallocating resources. While technologies and policies to improve energy efficiency reduce energy consumption, lower effective prices for energy increase the demand for energy services. When an increase in demand for energy services partially or fully offsets a decrease in energy consumption due to energy efficiency improvements or results in an increase in final energy consumption, the energy rebound effect occurs. The rebound effect has a significant influence on carbon reduction (Wang and Zhang, 2020), leading to an increase in carbon emissions (Böhringer and Rivers, 2021; Halvorsen and Larsen, 2021; Berner et al., 2022). But smart city construction offers viable solutions to the growing challenges of energy management (Selvaraj et al., 2023), so the increase in energy efficiency reduces carbon emissions instead (Golinska-Dawson and Sethanan, 2023). Smart cities are a safe and productive regional service that provides their residents with a good standard of living by optimizing resource development (Martins et al., 2021), and has changed the existing way of life (Mohammad et al., 2023).
On the other hand, diverse responses of carbon emissions are inevitable with irregular changes of energy efficiency shocks mainly because economies are in different trajectories in terms of their development path, resource availability, and environmental regulation; however, environmental policies do not solve the problem between energy efficiency and greenhouse gas emissions (Dirckinck-Holmfeld, 2015; Abban et al., 2020; Akram et al., 2020). As an important aspect of energy consumption, electricity consumption increases CO2 emissions in any time cycle (Salahuddin et al., 2018). Moreover, the increase in energy consumption, measured on a per capita basis, has accelerated CO2 emissions (Begum et al., 2015). An unrealistic technological optimism leads to low confidence in the effectiveness of energy and climate policies (Arvesen et al., 2011). Currently, urbanization has been a worldwide trend, accompanying it is, however, population concentration and spatial expansion, which bring a series of problems, such as population expansion, energy shortages, environmental pollution, but the introduction of smart city policies has effectively overcome these problems (Dong et al., 2022), smart city construction has significantly reduced per capita CO2 emissions, with a reduction effect of approximately 18.42 logarithmic percentage points (Guo et al., 2022).
Currently, improving energy efficiency has become the general consensus for achieving green economic growth in China, and it will be a key element in addressing the issue of climate change (Lee et al., 2017; Bayar and Gavriletea, 2019). Many cities worldwide have recognized that efficient energy utilization is essential to solving a range of environmental problems caused by global warming and have begun to develop policies to achieve long-term goals for lower emission levels (Mohareb and Kennedy, 2014). Thus, the question of whether the pilot policy, represented by low-carbon city construction, can improve green-oriented, efficient energy utilization and thus reduce carbon emissions to achieve carbon reduction targets has emerged as an important research topic. A shift in low-carbon lifestyles will occur when consumers’ various consumption decisions, such as consumption of energy, use of renewable energy technologies, and choice of transportation, are influenced by their interactions with their social peers. At this point, a carbon tax, and more broadly carbon pricing, would increase the relative price of goods and services in carbon-intensive production cycles, thereby encouraging a reduction in their consumption and a shift to low-carbon alternatives (Konc et al., 2021). Thus, the restrictive carbon emission control policy is the most active policy measure to reduce air pollution and promote public health (Gehrsitz, 2017). The pilot policy advocated by low carbon has achieved benefits at the output rate of green products because cities have increased their technological investments and combined technological and structural effects (Cheng et al., 2019). Hence, technological innovation plays an important role in improving energy efficiency, reducing energy intensity and carbon emissions (Zhang and Fu, 2022). In addition, spillovers of technological innovations from the home country have a significant positive correlation with the energy efficiency of neighboring countries (Sun et al., 2021; Adekoya et al., 2023), contributing to the construction of low-carbon cities, but it may be affected by differences in city characteristics (Kennedy et al., 2014; Mohareb and Kennedy, 2014). In addition to low-carbon policies, carbon pricing, markets, and taxes can direct spending to low-carbon city construction.
The main research focus of this paper is outlined as follows. Firstly, 277 prefecture-level cities are taken as the research subjects, with panel data spanning from 2004 to 2019 selected to assess the policy’s impact. Secondly, the SBM-DEA method, accounting for undesired outputs, is employed to determine the annual
The primary contributions of this study lie in two main areas. First, while existing literature focuses predominantly on the interplay between carbon emissions and energy efficiency, it often neglects the link between low-carbon policies, including low-carbon city initiatives, and energy efficiency. Utilizing a panel data sample and a multitemporal DID model at the city level in China, this study conducts a systematic empirical analysis of the impact of LCCPP on GTFEE. Second, the current literature does not adequately address the heterogeneity in quantitative relationships, especially concerning the “two control areas” and the variety of urban resource types. This paper empirically delineates the heterogeneity and mechanisms through which LCCPP influences GTFEE.
Climate change significantly impacts human survival and development, presenting a universal challenge to nations worldwide. Proactively addressing climate change is imperative for China’s economic and social progression, offering a significant opportunity to expedite the shift in economic paradigm and structural reformation. Energy demand in China is projected to increase persistently. Effectively mitigating greenhouse gas emissions and aptly responding to climate change, amidst economic development and the enhancement of living standards, emerge as pressing challenges. Numerous provinces and cities in China have launched low-carbon pilot projects, endeavoring to cultivate practices and insights into economic growth, livelihood enhancement, climate action, carbon intensity reduction, and the promotion of green development amidst the country’s swift industrialization and urbanization.
Carbon emissions have far-reaching effects on human survival and development, posing a serious challenge for all nations globally. To mitigate global warming, China has focused on enhancing energy efficiency (Song et al., 2023), optimizing the energy structure (Ding et al., 2019), adjusting the industrial structure (Zheng et al., 2023), and developing forest carbon sinks (Liu et al., 2012). Over the long term, China’s energy consumption is expected to continue its steady grow, making the study of LCCPP, GTFEE, and their interrelated mechanisms crucial.
The DID model has become a predominantly utilized policy evaluation methodology, extensively employed in social science research (Sanders and Barreca, 2022). When interventions are applied to multiple entities within a treatment group at different times, a DID model incorporating multiple time periods is employed (Wang et al., 2018; Callaway and Sant’Anna, 2021). In July 2010, five provinces and eight cities, such as Shaanxi, Yunnan, Tianjin, and Xiamen, were selected for pilot projects. In April 2012, more cities and provinces were included in the second batch of pilot areas. In January 2017, the government expanded the pilot initiative to encompass 45 regions, such as Wuhai, Shenyang, and Dalian. These sequential pilot implementations of low-carbon policies laid the groundwork for developing a DID model with multiple time periods. The construction of the specific model is outlined as follows.
where
As a significant energy consumer, China faces energy and environmental challenges that constrains economic and social development. Currently, two effective strategies are employed to address the energy issue: The first involves intensifying energy consumption through conservation and emission reduction to mitigate environmental pressure, and the second focuses on enhancing GTFEE to reduce the pressure of energy demand. Improving GTFEE is the pivotal in addressing the energy problem (Berner et al., 2022). According to the literature (Cecchini et al., 2018),
This study assumes that the feasible production set
The vector of input indicators is
The constraints are defined as
The calculation involves input variables including labor, capital, and energy, addition to output variables. For labor, the metric employed is the total number of employees across three industries. Capital is measured by the completed investment in fixed assets, which serving as the indicator. Energy consumption is gauged by fuel consumption, selected as the metric. The analysis also encompasses both positive and negative outputs, namely, GDP and CO2 emissions, respectively.
The policy variable
Economic development level (
Number of industrial enterprises (
Industrial restructuring (
Foreign direct investment
Fiscal decentralization (
Local finance general budget expenditure on science and technology (
Intensity of urban environmental regulation (
For smoother data representation, logarithmic transformations were applied to the absolute numerical indicators (
Assessing policy effectiveness lays the foundation for subsequent empirical analyses. At present, the main energy sources for urban industries encompass natural gas, liquefied petroleum gas, and electricity. Among these, the demand for electricity is the primary driver behind the increase in carbon dioxide emissions. The abundance of coal resources, the scarcity of oil, and limited natural gas in China, along with the relatively low cost of coal, have led to a significant dependence on coal for electricity generation Han and Xie (2017). Coal-fired power plants are the largest source of CO2 emissions among all power generation methods, with the power sector accounting for nearly half of China’s total carbon emissions. Thus, the methodology proposed by Ren and Zhang (2020) is utilized to precisely adjust the data for electricity, natural gas, and liquefied petroleum gas in calculating CO2 emissions.
Where CO2 denotes the industrial CO2 emissions;
Given that CO2 emissions primarily result from coal-based energy consumption, the effectiveness of the policy necessitates evaluation. In Model (1), explanatory variables are substituted with CO2 emissions to ascertain their validity, as shown in Table 2. The second column of Table 2 displays the results of the validity test, incorporating control variables. Furthermore, since LCCPP focuses on regulating local CO2 emissions, there is no intersecting policy between the management of industrial wastewater discharge and CO2 emissions, and their emission reduction strategies differ. Therefore, industrial wastewater discharges are unlikely to be affected by LCCPP, indirectly corroborating the policy’s effectiveness through a falsification test (Wang et al., 2018). Additionally, in Model (1), the explanatory variable is substituted with industrial wastewater discharge to challenge the policy’s effectiveness, as detailed in Table 2.
Table 2 shows that the LCCPP curtails CO2 emissions by at least a significant level of 5%; however, it does not affect industrial wastewater emissions. The findings are consistent with the previous settings. Wastewater treatment is achievable only through nutrient removal processes or by harnessing wastewater potential, which includes reducing energy consumption, pharmaceutical usage, or tapping into the energy inherent in wastewater. While savings in energy and pharmaceutical consumption are beneficial for energy conservation and emission reduction, they fall short of achieving low-carbon objectives.
Considering the evolving nature of the LCCPP, which varies over time and across individuals,
The coefficient
The government is intervening in a timely manner in the energy input and low-emission output of enterprises’ production activities, urging enterprises to optimize the allocation of carbon emission reduction resources and to use limited carbon emission resources judiciously to minimize energy waste and CO2 emissions. Carbon market trading rules explicitly state that factors such as economic growth, industrial restructuring, and energy structure optimization are pivotal in addressing greenhouse gas emissions, underscoring the importance of optimizing energy structures and enhancing GTFEE. Nonetheless, bridging low-carbon policies and energy issues necessitates technological solutions. Therefore, investments in new energy technologies and innovation should be consistently augmented during low-carbon city development to prevent energy waste and achieve genuine efficiency in energy use and carbon reduction. Moreover, environmentally sustainable and resource-efficient energy production and consumption practices are upheld, with the clean and efficient transition of coal usage being continuously enhanced to actively respond to the call for low carbon. The active promotion of new energy sources, such as wind and solar power, is contributing to the gradual emergence of the energy utilization benefits of the pilot policy exemplified by low-carbon city development.
Conducting a parallel trend test is a crucial step and a prerequisite for constructing a DID model. Unlike traditional DID, DID with multiple time periods accommodates policy timing inconsistencies, making it suitable for varied policy timings (Callaway and Sant’Anna, 2021). Therefore, just like the traditional DID model, the multitemporal DID regression still requires a parallel trend test to elucidate the dynamic effects of policies. Taking 2004 as the base period, Figure 1 examines the parallel trends and dynamic effects of multitemporal low-carbon policies.
Figure 1 illustrates that the confidence interval of the pilot policy test intersects with the zero axis up until 2009, thereby satisfying the parallel trend test. However, the policy effect exhibited a 1-year lag. From 2011 onwards, the impact of policy implementation began to materialize, with significant fluctuations observed in 2015. This is primarily attributed to cities progressively refining various supporting mechanisms, including circular development technologies and the formulation of policy documents. The development of a comprehensive institutional framework requires time, and enterprises within the pilot areas are becoming increasingly adept at applying low-carbon technologies.
In 2014, the government explicitly mandated a substantial reduction in CO2 emissions per unit of GDP within 2 years, with specific targets set at a minimum of 4% and 3.5% reductions. Concurrently, regions further specified binding targets for the phasing out of coal-fired boilers and restrictive measures for major air pollutant emissions. In pursuit of a successful green and low-carbon transformation, all regions have persistently refined and adjusted their industrial structures, actively addressed the issue of severe overcapacity, and fostered the development of low-carbon projects. Consequently, the impact of the LCCPP experienced a significant leap in 2015.
Figure 2 illustrates that only the pilot areas were included, with individuals in the control group lacking time and policy intervention being excluded from the robustness checks to more accurately assess the energy effect of the LCCPP.
The comparison between Figures 1, 2 shows that the dynamic effect results remained relatively stable. The corresponding t-value and confidence interval underwent minor changes, which did not impact the dynamic effect results. The aforementioned analysis indicates that the LCCPP has a positive impact on GTFEE, with stable results.
According to the existing literature (Shi and Li, 2020), energy policies are categorized into energy restructuring policies, energy conservation policies, and new energy policies. In response to energy restructuring policies, given coal’s role as the primary energy source, its efficiency directly influences the attainment of carbon reduction target on schedule, inevitably impacting the effectiveness in pilot areas. The eight major coal-producing provinces in China include Henan, Anhui, Shandong, Xinjiang Uygur Autonomous Region, Guizhou, Shaanxi, Shanxi, and Inner Mongolia Autonomous Region. The government prioritizes these provinces and autonomous regions for the formulation of low-carbon policies, adjustments to energy policies, and investments in low-carbon technologies. To mitigate potential interference with prior results, samples from these provinces and autonomous regions were excluded from the overall sample to ensure clearer policy effects.
In response to energy saving and new energy policies, government departments performed demonstration pilot projects in locations such as Beijing and Shanghai in 2009. Thirteen prefecture-level cities were excluded from the sample to eliminate the influence and deviation in the estimation results in Table 4.
Table 4 shows that, after excluding energy restructuring, energy conservation, and new energy policies, the LCCPP improved GTFEE to varying degrees, aligning closely with previous findings and confirming the stability of the results. Faced with the CO2 emission reduction targets established by low-carbon policies, original high-energy-consuming industries often transition towards the service sector, high-tech industries, and other sectors characterized by lower energy inputs and carbon emissions to sustain growth and reduce reliance on energy consumption. This shift leads to changes in the industrial structure and economic development patterns, ultimately enhancing energy efficiency.
The combustion of fossil fuels releases particulate matter, sulfur dioxide, and nitrogen oxides as air pollutants, in addition to CO2, which contributes to atmospheric pollution and greenhouse gas emissions. According to the Atmospheric Pollution Prevention and Treatment Law, the sample is divided into “two control areas” and non “two control areas” in Table 5. The former refers to areas prone to or experiencing acid rain, while the latter denotes regions with significant sulfur dioxide pollution. Subsequently, the analysis explores the heterogeneity of the LCCPP among regions with varying characteristics.
Table 5 demonstrates that within the “two control areas”, the LCCPP significantly enhanced GTFEE. The predominant air pollutants in the “two control areas” are sulfur dioxide, and due to the similar nature of sulfur dioxide and CO2, a synergistic reduction in sulfur emissions can be achieved alongside CO2 reduction. The scope of synergy should broaden from solely focusing on atmospheric pollutants and greenhouse gases to encompassing a wider synergy across energy, climate, and environmental sectors, where the interconnection between pollutants, greenhouse gases, and energy is pivotal. Regarding the synergistic approach, efforts should be made to maximize the cost-effectiveness of synergistic aspects, while relatively independent elements can be addressed separately. At the implementation level of the synergistic path, fundamental aspects such as energy conservation, adjustment of energy and industrial structures, energy decarbonization, and the adoption of green and low-carbon consumption patterns are crucial for the synergistic management of greenhouse gases and pollutants. At the local level, the promotion of synergistic control is critically important, taking into account the differentiated policies across the Eastern, Central, and Western regions. Moreover, local pilot demonstrations should align with the objectives of sustainability, low carbon emissions, and waste reduction. In the non “two control areas”, the ecological and environmental impact of sulfur dioxide and CO2 emissions is less severe. These regions lack definitive long-term emission reduction targets, exhibit weaker environmental regulation, and have lower levels of industrial transformation, upgrading, and technological innovation compared to the “two control areas”. In addition, the “two control areas” policy may incentivize polluting industries or enterprises to relocate from cities within the “two control areas” to cities in non “two control areas”, either within or outside the province, thereby diminishing the catalytic impact of the LCCPP.
Energy, being a crucial component of a country’s economic development, is closely linked to the local resource endowment (Shi and Li, 2020). Considering the varying levels of economic and social development, resource-based cities are categorized into growth, maturity, recessionary, and regenerative phases to assess the heterogeneity of the LCCPP across different city types, as illustrated in Table 6.
Table 6 reveals that cities of different types exhibit varied outcomes. In growing resource-based cities, there is substantial potential for regional economic development, and the resource supply system is robust. Additionally, amidst the push for low-carbon constraints and energy-saving development, investment in energy utilization technology is advanced, ensuring a balanced intensity of resource development and utilization. Moreover, the strategic layout of emerging industries in these regions is forward-looking, with a harmonious interplay between resource and urban development, leading to a significant energy effect from the LCCPP. However, in mature resource-based cities, the LCCPP has yielded contrasting result. In cities like Datong, the overall energy efficiency of key energy-consuming industries falls short of that in more advanced enterprises. The adoption of new-generation manufacturing technologies lags, necessitating improvements in industrial production and energy efficiency. The coal-dominated energy consumption structure poses significant short-term challenges to effective improvement, complicating the adjustment of the energy structure (Zhang et al., 2012). Additionally, while energy-saving initiatives like the retrofitting of coal-fired power plants and the reduction and substitution of coal consumption are progressively being implemented, a positive transformation in energy utilization has yet to be realized. The significance of recessionary and regenerative cities did not meet statistical significance. In these cities, resources are nearing depletion, economic growth is lagging, and urban economic revival is contending with significant ecological challenges (Dou et al., 2023). The pressure to enhance ecological quality is immense, yet the drive for low-carbon city development is lacking. Furthermore, these cities already exhibit minimal reliance on resources, necessitating optimization and adjustment of the economic structure.
This study delves into the internal mechanism between LCCPP and GTFEE. The degree of industrial structure adjustment is selected as the mediating variable to examine the mechanism. At present, the evolution of the industrial structure has not been coordinated with the industrialization, and the extensive development mode has not been fundamentally transformed. Within the context of green economic development, there remains a significant gap in the growth of low-carbon emerging industries. To meet CO2 reduction targets, market-based environmental regulation policies are gradually being implemented in pilot cities. Through the coordination of various policies, the LCCPP has facilitated the restructuring of the industrial structure, with a rational industrial setup playing a significant role among the numerous factors of energy utilization. The industrial transfer and cross-regional energy flow have fostered a closer spatial relationship between the two elements. In fact, the pollution and carbon reduction effects of low-carbon policies are achieved through the impact on industrial structure (Eicke and Weko, 2022), leading to a decrease in carbon emissions and an increase in carbon substitution (Zi-ling et al., 2023). In addition, the upgrade of the industrial structure enhances energy efficiency (Muhammad et al., 2022; Nguyen, 2024a), offering valuable policy insights for motivating industrial firms to adopt higher energy efficiency (Nguyen, 2024b). Therefore, industrial restructuring is identified as the mechanism variable.
Beyond Model (1), which assesses the overall effect, this study also explores the impact of the pilot policy on industrial restructuring and integrates both the pilot policy and industrial restructuring into the model. The construction of the specific model is as follows.
where
In Table 7, the regression coefficients
Drawing on data from prefecture-level cities spanning 2004 to 2019, this study investigates the impact of LCCPP on GTFEE using the multitemporal DID model. The findings reveal that the LCCPP effectively enhances GTFEE, a conclusion that holds up under robustness testing. Heterogeneity testing shows that the energy efficiency impact of LCCPP is more significant in the “two control areas”, with such policies in developing and mature resource cities notably boosting GTFEE. Moreover, industrial restructuring emerges as a crucial mechanism that influences the dynamic between the two, aligning with the findings of Du et al. (2022). The insights gained from this research enrich the low-carbon field and extend the realm of energy efficiency studies. Building on these critical findings, the study delineates its policy implications across several dimensions.
First, it is imperative to bolster the pilot initiatives in low-carbon cities and broaden the scope of the pilot program. The results indicated that, compared to non-pilot areas, the policy in pilot areas significantly improves GTFEE. On one hand, optimizing the economic and emission reduction impacts of the pilot low-carbon city policy is essential to simultaneously enhance both economic and ecological quality. Conversely, the creation of a “pilot-diffusion” mechanism to absorb the successes of pilot cities, account for each city’s unique development context, and systematically expand the policy to additional non-pilot cities nationwide is crucial. Additionally, the government should enhance environmental regulation, increase entry barriers for the secondary sector, encourage capital investments in the service sector, and promote the overall optimization and modernization of the industrial structure. This approach aims to nurture a dynamic, varied market environment conducive to low-carbon city development and improved energy efficiency.
Second, it is imperative to customize the LCCPP according to local conditions. The results underscore that the effectiveness of low-carbon city construction policies in reducing energy consumption is contingent upon the unique attributes of each city. Therefore, local governments should conduct thorough assessments of their specific circumstances, leveraging urban resources and geographical strengths to optimize energy usage, develop a low-carbon economy, and achieve a symbiotic relationship between economic growth and environmental sustainability. The government is advised to give precedence to the advancement of low-carbon initiatives in non “two control areas”, economically declining, and regenerative urban areas, with a focus on their level of economic development, industrial makeup, and innovation capacity, while fostering key economic sectors and sustainable industries. Moreover, intensifying informational campaigns on low-carbon initiatives and guiding businesses toward eco-innovations and sustainable practices are critical steps towards enhancing overall energy efficiency.
Third, it is vital to promote the enhancement and evolution of the industrial structure. The results demonstrate that industrial restructuring acts as a crucial factor in LCCPP aimed at improving GTFEE. In adjusting the industrial framework, the government must actively encourage the adoption of energy-efficient and emission-reducing technologies within industries, support the expansion of the modern service sector, guide manufacturing towards service-oriented models, create low-carbon industrial clusters, and develop a range of low-carbon industrial platforms based around high-tech parks. Pilot cities ought to lead the collaboration between industry, academia, and research institutions, utilize various research facilities, encourage the integration of innovative practices into businesses, and motivate firms to enhance their research and application of low-carbon technologies.
The raw data supporting the conclusion of this article will be made available by the authors, without undue reservation.
FC: Writing–original draft, Writing–review and editing. FG: Methodology, Supervision, Writing–review and editing. JT: Data curation, Software, Writing–review and editing. JL: Data curation, Writing–review and editing.
The author(s) declare that financial support was received for the research, authorship, and/or publication of this article. This research was funded by the Social Science Fund of Henan Province (2022CJJ139), the Doctoral Research Start-up Fund Project (13480042) and the Open Fund of Sichuan Province Cyclic Economy Research Center (XHJJ-2302).
The authors declare that the research was conducted in the absence of any commercial or financial relationships that could be construed as a potential conflict of interest.
All claims expressed in this article are solely those of the authors and do not necessarily represent those of their affiliated organizations, or those of the publisher, the editors and the reviewers. Any product that may be evaluated in this article, or claim that may be made by its manufacturer, is not guaranteed or endorsed by the publisher.
Abban, O. J., Wu, J., and Mensah, I. A. (2020). Analysis on the nexus amid CO 2 emissions, energy intensity, economic growth, and foreign direct investment in Belt and Road economies: does the level of income matter? Environ. Sci. Pollut. Res. 27, 11387–11402. doi:10.1007/s11356-020-07685-9
Abrell, J., Kosch, M., and Rausch, S. (2022). How effective is carbon pricing? a machine learning approach to policy evaluation. J. Environ. Econ. Manag. 112, 102589. doi:10.1016/j.jeem.2021.102589
Adekoya, O. B., Oliyide, J. A., Kenku, O. T., and Ajayi, O. F. (2023). China's technological spillover effect on the energy efficiency of the BRI countries. Energy Policy 182, 113740. doi:10.1016/j.enpol.2023.113740
Akram, R., Majeed, M. T., Fareed, Z., Khalid, F., and Ye, C. (2020). Asymmetric effects of energy efficiency and renewable energy on carbon emissions of BRICS economies: evidence from nonlinear panel autoregressive distributed lag model. Environ. Sci. Pollut. Res. 27, 18254–18268. doi:10.1007/s11356-020-08353-8
Al-mulali, U., Sab, C., and Fereidouni, H. G. (2012). Exploring the bi-directional long run relationship between urbanization, energy consumption, and carbon dioxide emission. Energy 46 (1), 156–167. doi:10.1016/j.energy.2012.08.043
Arvesen, A., Bright, R. M., and Hertwich, E. G. (2011). Considering only first-order effects? How simplifications lead to unrealistic technology optimism in climate change mitigation. Energy Policy 39 (11), 7448–7454. doi:10.1016/j.enpol.2011.09.013
Babatunde, K. A., Said, F. F., Nor, N. G. M., Begum, R. A., and Mahmoud, M. A. (2021). Coherent or conflicting? Assessing natural gas subsidy and energy efficiency policy interactions amid CO2 emissions reduction in Malaysia electricity sector. J. Clean. Prod. 279, 123374. doi:10.1016/j.jclepro.2020.123374
Bayar, Y., and Gavriletea, M. D. (2019). Energy efficiency, renewable energy, economic growth: evidence from emerging market economies. Qual. Quantity 53, 2221–2234. doi:10.1007/s11135-019-00867-9
Begum, R. A., Sohag, K., Abdullah, S. M. S., and Jaafar, M. (2015). CO2 emissions, energy consumption, economic and population growth in Malaysia. Renew. Sustain. Energy Rev. 41, 594–601. doi:10.1016/j.rser.2014.07.205
Berner, A., Bruns, S., Moneta, A., and Stern, D. I. (2022). Do energy efficiency improvements reduce energy use? Empirical evidence on the economy-wide rebound effect in Europe and the United States. Energy Econ. 110, 105939. doi:10.1016/j.eneco.2022.105939
Böhringer, C., and Rivers, N. (2021). The energy efficiency rebound effect in general equilibrium. J. Environ. Econ. Manag. 109, 102508. doi:10.1016/j.jeem.2021.102508
Callaway, B., and Sant’Anna, P. H. (2021). Difference-in-differences with multiple time periods. J. Econ. 225 (2), 200–230. doi:10.1016/j.jeconom.2020.12.001
Cecchini, L., Venanzi, S., Pierri, A., and Chiorri, M. (2018). Environmental efficiency analysis and estimation of CO2 abatement costs in dairy cattle farms in Umbria (Italy): a SBM-DEA model with undesirable output. J. Clean. Prod. 197, 895–907. doi:10.1016/j.jclepro.2018.06.165
Cheng, J., Yi, J., Dai, S., and Xiong, Y. (2019). Can low-carbon city construction facilitate green growth? Evidence from China's pilot low-carbon city initiative. J. Clean. Prod. 231, 1158–1170. doi:10.1016/j.jclepro.2019.05.327
Cheng, Y. W., Mu, D., Ren, H. Y., Fan, T. J., and Du, J. B. (2020). Using a temporal input-output approach to analyze the ripple effect of China's energy consumption. Energy 211, 118641. doi:10.1016/j.energy.2020.118641
Ding, N., Pan, J., Liu, J., and Yang, J. (2019). An optimization method for energy structures based on life cycle assessment and its application to the power grid in China. J. Environ. Manag. 238, 18–24. doi:10.1016/j.jenvman.2019.02.072
Dirckinck-Holmfeld, K. (2015). The options of local authorities for addressing climate change and energy efficiency through environmental regulation of companies. J. Clean. Prod. 98, 175–184. doi:10.1016/j.jclepro.2014.12.067
Dong, F., Li, Y., Li, K., Zhu, J., and Zheng, L. (2022). Can smart city construction improve urban ecological total factor energy efficiency in China? Fresh evidence from generalized synthetic control method. Energy 241, 122909. doi:10.1016/j.energy.2021.122909
Dou, S., Zhu, Y., Xu, D., and Amuakwa-Mensah, F. (2023). Ecological challenges in the economic recovery of resource-depleted cities in China. J. Environ. Manag. 333, 117406. doi:10.1016/j.jenvman.2023.117406
Eicke, L., and Weko, S. (2022). Does green growth foster green policies? Value chain upgrading and feedback mechanisms on renewable energy policies. Energy Policy 165, 112948. doi:10.1016/j.enpol.2022.112948
Fernando, Y., and Hor, W. L. (2017). Impacts of energy management practices on energy efficiency and carbon emissions reduction: a survey of malaysian manufacturing firms. Resour. conservation Recycl. 126, 62–73. doi:10.1016/j.resconrec.2017.07.023
Gehrsitz, M. (2017). The effect of low emission zones on air pollution and infant health. J. Environ. Econ. Manag. 83, 121–144. doi:10.1016/j.jeem.2017.02.003
Golinska-Dawson, P., and Sethanan, K. (2023). Sustainable urban freight for energy-efficient smart cities—systematic literature review. Energies 16 (6), 2617. doi:10.3390/en16062617
Guo, Q., Wang, Y., and Dong, X. (2022). Effects of smart city construction on energy saving and CO2 emission reduction: evidence from China. Appl. Energy 313, 118879. doi:10.1016/j.apenergy.2022.118879
Halvorsen, B., and Larsen, B. M. (2021). Identifying drivers for the direct rebound when energy efficiency is unknown. The importance of substitution and scale effects. Energy 222, 119879. doi:10.1016/j.energy.2021.119879
Han, F., and Xie, R. (2017). Has the concentration of producer services reduced carbon emissions? J. Quantitative Tech. Econ. 34 (03), 40–58. doi:10.13653/j.cnki.jqte.2017.03.003
Hancevic, P. I., and Sandoval, H. H. (2022). Low-income energy efficiency programs and energy consumption. J. Environ. Econ. Manag. 113, 102656. doi:10.1016/j.jeem.2022.102656
Jefferson, M. (2018). Renewable and low carbon technologies policy. Energy policy 123, 367–372. doi:10.1016/j.enpol.2018.07.039
Ji, Y., and Li, C. (2015). Innovation driving and industrial upgrading: the spatial econometric analysis based on China’s provincial panel data. Stud. Sci. Sci. 33 (11), 1651–1659. doi:10.16192/j.cnki.1003-2053.2015.11.007
Kennedy, C., Ibrahim, N., and Hoornweg, D. (2014). Low-carbon infrastructure strategies for cities. Nat. Clim. Change 4 (5), 343–346. doi:10.1038/nclimate2160
Konc, T., Savin, I., and van den Bergh, J. C. (2021). The social multiplier of environmental policy: application to carbon taxation. J. Environ. Econ. Manag. 105, 102396. doi:10.1016/j.jeem.2020.102396
Lee, C. T., Hashim, H., Ho, C. S., Van Fan, Y., and Klemeš, J. J. (2017). Sustaining the low-carbon emission development in Asia and beyond: sustainable energy, water, transportation and low-carbon emission technology. J. Clean. Prod. 146, 1–13. doi:10.1016/j.jclepro.2016.11.144
Li, F. (2012). The home country industrial upgrading effect of outward FDI: an empirical study from an interprovincial panel in China. J. Int. Trade 06, 124–134. doi:10.13510/j.cnki.jit.2012.06.010
List, J. A., and Co, C. Y. (2000). The effects of environmental regulations on foreign direct investment. J. Environ. Econ. Manag. 40 (1), 1–20. doi:10.1006/jeem.1999.1095
Liu, S., Zhou, T., Wei, L., and Shu, Y. (2012). The spatial distribution of forest carbon sinks and sources in China. Chin. Sci. Bull. 57, 1699–1707. doi:10.1007/s11434-012-4998-1
Martins, F., Patrão, C., Moura, P., and de Almeida, A. T. (2021). A review of energy modeling tools for energy efficiency in smart cities. Smart Cities 4 (4), 1420–1436. doi:10.3390/smartcities4040075
Matsumura, E. M., Prakash, R., and Vera-Munoz, S. C. (2014). Firm-value effects of carbon emissions and carbon disclosures. Account. Rev. 89 (2), 695–724. doi:10.2308/accr-50629
Mohammad, A., Modabbir, A. I., and Kamal, M. M. (2023). Economic and environmental impact of energy efficient design of smart lighting system. J. Institution Eng. (India) Ser. B, 104, 1–14.
Mohareb, E. A., and Kennedy, C. A. (2014). Scenarios of technology adoption towards low-carbon cities. Energy policy 66, 685–693. doi:10.1016/j.enpol.2013.10.070
Muhammad, S., Pan, Y. C., Agha, M. H., Umar, M., and Chen, S. Y. (2022). Industrial structure, energy intensity and environmental efficiency across developed and developing economies: the intermediary role of primary, secondary and tertiary industry. ENERGY 247, 123576. doi:10.1016/j.energy.2022.123576
Nguyen, J. (2024a). Beyond policy impacts: internal strategic capabilities as determinants of industrial energy efficiency implementation. ENERGY POLICY 184, 113898. doi:10.1016/j.enpol.2023.113898
Nguyen, J. (2024b). Beyond policy impacts: internal strategic capabilities as determinants of industrial energy efficiency implementation. Energy Policy 184, 113898. doi:10.1016/j.enpol.2023.113898
Nibedita, B., and Irfan, M. (2021). The role of energy efficiency and energy diversity in reducing carbon emissions: empirical evidence on the long-run trade-off or synergy in emerging economies. Environ. Sci. Pollut. Res. 28 (40), 56938–56954. doi:10.1007/s11356-021-14642-7
Ren, Y., and Zhang, G. (2020). Can city innovation dispel haze? China Popul. Resour. Environ. 30 (02), 111–120.
Salahuddin, M., Alam, K., Ozturk, I., and Sohag, K. (2018). The effects of electricity consumption, economic growth, financial development and foreign direct investment on CO2 emissions in Kuwait. Renew. Sustain. energy Rev. 81, 2002–2010. doi:10.1016/j.rser.2017.06.009
Sanders, N. J., and Barreca, A. I. (2022). Adaptation to environmental change: agriculture and the unexpected incidence of the acid rain Program. Am. Econ. J. Econ. Policy 14 (1), 373–401. doi:10.1257/pol.20190060
Selvaraj, R., Kuthadi, V. M., and Baskar, S. (2023). Smart building energy management and monitoring system based on artificial intelligence in smart city. Sustain. Energy Technol. Assessments 56, 103090. doi:10.1016/j.seta.2023.103090
Shi, D., and Li, S. (2020). Emissions trading system and energy use efficiency--measurements and empirical evidence for cities at and above the prefecture level. China Ind. Econ. 390 (09), 5–23. doi:10.19581/j.cnki.ciejournal.2020.09.001
Song, M., Pan, H., Vardanyan, M., and Shen, Z. (2023). Evaluating the energy efficiency-enhancing potential of the digital economy: evidence from China. J. Environ. Manag. 344, 118408. doi:10.1016/j.jenvman.2023.118408
Sun, H., Edziah, B. K., Kporsu, A. K., Sarkodie, S. A., and Taghizadeh-Hesary, F. (2021). Energy efficiency: the role of technological innovation and knowledge spillover. Technol. Forecast. Soc. Change 167, 120659. doi:10.1016/j.techfore.2021.120659
Wang, C., Wu, J., and Zhang, B. (2018). Environmental regulation, emissions and productivity: evidence from Chinese COD-emitting manufacturers. J. Environ. Econ. Manag. 92, 54–73. doi:10.1016/j.jeem.2018.08.004
Wang, Q., and Zhang, F. (2020). Does increasing investment in research and development promote economic growth decoupling from carbon emission growth? An empirical analysis of BRICS countries. J. Clean. Prod. 252, 119853. doi:10.1016/j.jclepro.2019.119853
Yao, X., Zhou, H., Zhang, A., and Li, A. (2015). Regional energy efficiency, carbon emission performance and technology gaps in China: a meta-frontier non-radial directional distance function analysis. Energy Policy 84, 142–154. doi:10.1016/j.enpol.2015.05.001
Zhang, R., and Fu, Y. (2022). Technological progress effects on energy efficiency from the perspective of technological innovation and technology introduction: an empirical study of Guangdong, China. Energy Rep. 8, 425–437. doi:10.1016/j.egyr.2021.11.282
Zhang, W., Duan, X., Yu, L., Zhang, W., and Qi, X. (2012). Comparisons and analysis of the car bon emissions fr om ener gy consumption between the resources- based industrial citie and the moder n industrial city. Econ. Geogr. 32 (01), 119–125. doi:10.15957/j.cnki.jjdl.2012.01.004
Zhao, M., Liu, F., Wang, H., and Sun, W. (2020). Foreign direct investment, environmental regulation and urban green total factor productivity of the yellow river basin. Econ. Geogr. 40 (04), 38–47. doi:10.15957/j.cnki.jjdl.2020.04.005
Zheng, Y., Tang, J., and Huang, F. (2023). The impact of industrial structure adjustment on the spatial industrial linkage of carbon emission: from the perspective of climate change mitigation. J. Environ. Manag. 345, 118620. doi:10.1016/j.jenvman.2023.118620
Zhou, C., and Wei, J. (2007). Industrial structure level measurement model and empirical analysis--taking Shanghai, shenzhen and ningbo as examples. Shanghai J. Econ. 6, 15–21. doi:10.19626/j.cnki.cn31-1163/f.2007.06.003
Keywords: low-carbon city pilot policy, green total factor energy efficiency, difference-in-differences, heterogeneity, mechanism
Citation: Cheng F, Guo F, Tang J and Liu J (2024) Can low-carbon city pilot policies improve urban energy efficiency? evidence from a quasi-natural experiment in China. Front. Environ. Sci. 12:1390910. doi: 10.3389/fenvs.2024.1390910
Received: 24 February 2024; Accepted: 22 March 2024;
Published: 05 April 2024.
Edited by:
Yantuan Yu, Guangdong University of Foreign Studies, ChinaReviewed by:
Ming Qi, China University of Petroleum, ChinaCopyright © 2024 Cheng, Guo, Tang and Liu. This is an open-access article distributed under the terms of the Creative Commons Attribution License (CC BY). The use, distribution or reproduction in other forums is permitted, provided the original author(s) and the copyright owner(s) are credited and that the original publication in this journal is cited, in accordance with accepted academic practice. No use, distribution or reproduction is permitted which does not comply with these terms.
*Correspondence: Jiaomei Tang, dGFuZ2ltMTExN0AxNjMuY29t; Junxia Liu, MTgzMjMxNTk1NzFAMTYzLmNvbQ==
Disclaimer: All claims expressed in this article are solely those of the authors and do not necessarily represent those of their affiliated organizations, or those of the publisher, the editors and the reviewers. Any product that may be evaluated in this article or claim that may be made by its manufacturer is not guaranteed or endorsed by the publisher.
Research integrity at Frontiers
Learn more about the work of our research integrity team to safeguard the quality of each article we publish.