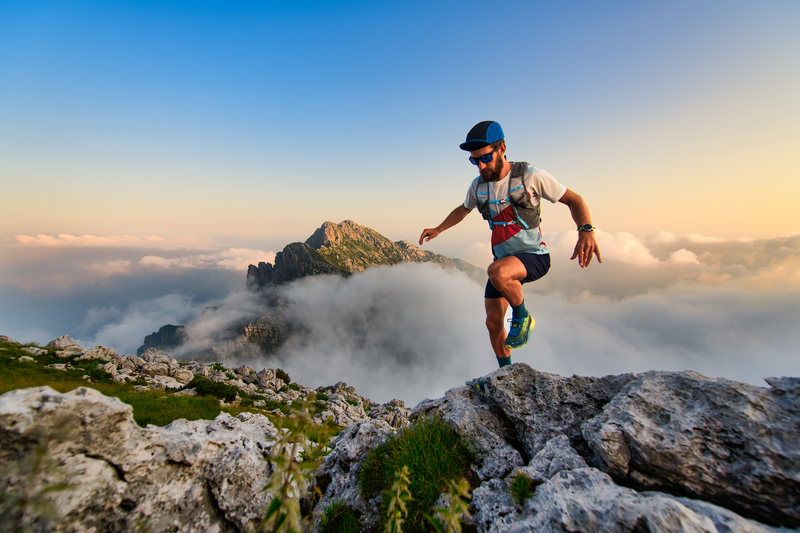
95% of researchers rate our articles as excellent or good
Learn more about the work of our research integrity team to safeguard the quality of each article we publish.
Find out more
ORIGINAL RESEARCH article
Front. Environ. Sci. , 24 July 2024
Sec. Environmental Economics and Management
Volume 12 - 2024 | https://doi.org/10.3389/fenvs.2024.1389876
This article is part of the Research Topic Greenhouse Gas Emission (GHG) Reduction and Economic Structural Transformation View all 18 articles
China has significantly increased its foreign direct investment (FDI). Although these investments help to boost global development, their impacts on the environment are still controversial and deserve careful investigation. This paper uses the super-efficiency SBM model to measure the green total factor productivity (GTFP) of 123 host countries of China’s FDI. On this basis, we adopt the two-step system GMM and dynamic panel threshold effect model to analyze the impact of China’s FDI on the GTFP of host countries. The research results show that: 1) The impact of China’s FDI on the GTFP of host countries is mainly through green technology efficiency. Its impact is inhibitory, followed by a promotional one, with the latter being significantly larger than the former. 2) There is no evidence of the “pollution haven effect” associated with China’s FDI. In fact, for countries with lower environmental regulations, China’s FDI promotes the growth of GTFP more effectively. 3) The innovation level of host countries moderates the relationship between China’s FDI and GTFP growth. For host countries with stronger innovation level, their GTFP growth can be enhanced to a greater extent by China’s FDI.
In the era of globalization, China’s rapidly growing FDI has emerged as a dominant force in international investment. According to the World Investment Report 2023, issued by the United Nations Conference on Trade and Development, China’s outward FDI flow reached US$1.5 trillion, with its year-end stock of US$39.9 trillion in 2022, ranking second and third respectively in the world. China’s FDI spans a wide range of regions and economic environments, exerting far-reaching influence on the world. Meanwhile, increasing attention has been paid to the impact of China’s FDI on global environment in the context of the current challenges posed by climate and environmental issues.
China’s FDI is characterized not only by its rapidly growing scale, but also by the diverse investment fields. As illustrated in Figure 1, a significant portion of China’s FDI has flowed into industries of energy, transport, and mining. The construction and operation of these projects tend to consume large quantities of iron, steel, cement as well as fossil energy, generating substantial carbon dioxide and pollutant emissions (Lu et al., 2010; Danish et al., 2018). All these problems mentioned have raised concerns about carbon emissions and environmental degradation in China’s FDI host countries (Oladipupo and Ajide, 2023). Some critics argue that China’s FDI activities, particularly those directed toward less developed countries, aim to seek “pollution havens,” whereby domestic pollution is transferred to other countries (Mahadevan and Sun, 2020; Gallagher and Qi, 2021). Nevertheless, Chinese authorities have stated that China remains committed to fortifying international collaboration on environmental protection and carbon emission reduction, with the aim of actively promoting the green and sustainable development of FDI host countries. Consequently, it is of paramount importance to study the impact of China’s FDI on the green total factor productivity (GTFP) of host countries and to investigate whether there is a “pollution haven effect” associated with China’s FDI.
GTFP is an enhanced measure based on Total Factor Productivity (TFP), integrating resource consumption and environmental impacts into the overall productivity analysis (Xie et al., 2021). In the context of advancing carbon neutrality goals, GTFP has become a crucial metric for policymakers and scholars to assess the green and sustainable development of economies. Consequently, study on relationship between China’s FDI and the host country’s GTFP can not only provide valuable insights into the environmental impact of China’s outward FDI activities but also plays a vital role in fostering the green and low-carbon development of China’s international economic and trade cooperation.
Recent studies have focused extensively on the impact of FDI on GTFP, yielding various conclusions. Some research has verified that FDI can enhance GTFP in host countries through mechanisms such as technology transfer and spillover effects (Zhou et al., 2019a; Tian, 2022), human capital development (Zhu and Ye, 2018), stimulation of local competition (Zhou et al., 2019b), as well as improvements of regulatory and policy frameworks (Qiu et al., 2021; Luo et al., 2023). Conversely, other researchers have identified potential negative impacts of FDI on green development and GTFP in host countries. Walter (1979) introduced the “pollution haven” hypothesis, suggesting that multinational corporations might transfer pollution-intensive production activities to countries with less stringent environmental regulations through FDI, thereby increasing pollution in host countries (Copeland and Taylor, 2004). Qiu et al. (2021) found evidence of “pollution haven effect” in FDI towards Eastern and Central China, exerting adverse effects on local GTFP. Similarly, Benzaim et al. (2023) observed that U.S. FDI negatively impacted GTFP in OECD countries. Zhao et al. (2022) found, in their study of 284 prefecture-level cities in China, that FDI inflows primarily hindered local GTFP by suppressing green technological advancement, with these adverse effects being more pronounced in resource-dependent and non-coastal cities.
Institutional factors and innovation levels in host countries are recognized as critical moderators in the relationship between FDI and GTFP (Hu et al., 2021; Li et al., 2021; Ma and Cao, 2022; Luo et al., 2023). From the perspective of institution, several key elements contribute to this dynamic. First, the establishment and enforcement of robust legal frameworks can be conducive to a more effective management of FDI, ensuring that investments are directed towards green, sustainable development and mitigating the pollution haven effect (Yu et al., 2021). Second, higher institutional quality provides stable expectations for FDI projects, facilitating the transfer of green technologies to the host country (Xie and Zhang, 2021). Third, companies are compelled by stringent environmental regulations to adopt cleaner technologies, thereby enhancing the positive impact of FDI on the host country’s GTFP (Xiaofei et al., 2021). Regarding the innovation level of host countries, higher innovation capacity enables better absorption of advanced technologies introduced by foreign investors (Desbordes and Franssen, 2019) and facilitates the dissemination and diffusion of these technologies within host countries (Hu et al., 2021). Additionally, FDI enterprises investing in host countries with higher levels of innovation often encounter greater competitive pressures, motivating them to improve their green production efficiency (Xiao et al., 2022).
In recent years, there has been an increasing attention paid to China’s rapidly growing FDI from scholars. Relevant studies on China’s FDI reveal that it is insensitive to the political stability and governance levels of host countries (Chen et al., 2018), but it tends to target these countries with abundant natural resources or advanced technology (Ramasamy et al., 2012; Yao et al., 2017). Research on the outward FDI behavior of listed companies in China demonstrate that China’s FDI exhibits reverse technology spillovers, significantly enhancing domestic GTFP. However, this enhancement is influenced by the heterogeneity of investment sectors, countries, and entities (Zhu and Ye, 2018; Liu and Xin, 2019; Pan et al., 2020; Xu and Zhou, 2023). Based on China’s Belt and Road Initiative, Haiyue and Manzoor (2020) discovered that it helps improve the GTFP of both participating investment enterprises and host countries.
Despite extensive research on the relationship between FDI and GTFP, several critical gaps remain that warrant further exploration. 1) The impact of China’s FDI on the world: as the world’s second-largest in FDI flow and third-largest in FDI stock, China’s FDI has a significant impact globally, especially on the environment. However, existing research primarily examines the reverse effects of China’s FDI on its domestic environment, neglecting the broader environmental impact on host countries and the external environmental impacts of China’s international investment are overlooked against this backdrop. 2) The distinct characteristics of China’s FDI: despite substantial evidence indicating China’s preference for investing in specific regions and sectors, there is a lack of studies on different impacts of China’s FDI across various host countries. This oversight impedes a nuanced understanding of the specific impacts of China’s FDI, including critical investigations into whether China’s investment strategies align with pursuing “pollution havens,” potentially providing vital insights for policy considerations. 3) The dynamics of FDI’s impact on GTFP: most existing research relies on static data to assess FDI’s impact on GTFP, which raises concerns about endogeneity and fails to account for the evolving nature of FDI’s impact on GTFP over time. This static analysis limits the understanding of the interactions between FDI and the environment.
This paper aims to analyze the dynamic impact of China’s FDI on the GTFP of host countries. On this basis, it examines the threshold effects of environmental regulation and innovation levels in the relationship between China’s FDI and the GTFP of host countries. This investigation explores whether China’s FDI exhibits a “pollution haven effect” and seeks to understand the role of host countries’ innovation levels in FDI’s impact on GTFP. Compared to existing literature, the novelty of this study lies in three aspects. 1) Comprehensive data utilization: the study employs extensive datasets from the International Energy Agency, Environmental Performance Index, Penn World Table, World Bank, and the National Bureau of Statistics of China, compiling matched panel data for 123 countries from 2007 to 2019. The extensive sample size and rich dataset ensure high reliability for our research. 2) Refined GTFP calculation: adopting a super-efficiency SBM model that incorporates undesirable outputs, this study calculates GTFP with greater accuracy. This method not only provides a more precise measurement of GTFP but also allows for a detailed decomposition of GTFP changes into technological and scale efficiency components, offering a deeper understanding of the factors driving GTFP changes. 3) Advanced methodologies: employing a two-step system GMM and a dynamic panel threshold effects model, this paper delivers a more detailed analysis of the dynamic impacts of China’s FDI on environment in host countries. It also examines how environmental regulations and innovation levels in host countries modulate the effects of China’s FDI.
The possible contributions of this paper include three main aspects. 1) Research Expansion. This research enriches the discussion on the environmental impacts of China’s FDI and broadens the understanding of its global effects, particularly in relation to the green development of host countries. 2) Research methodology. By adopting innovative methods and models, this study reveals the mechanism and dynamic characteristic of FDI’s impact on the GTFP of host countries, providing new empirical insights. 3) Research objects. Our finding highlights the conditional factors in host countries that moderate the effects of FDI, offering a foundation for policies aimed at promoting green and sustainable development.
FDI plays a crucial role in the economic development of a host country by providing capital, technology, and management expertise. However, the environmental impact of FDI, particularly on GTFP), can vary over time. Initially, host countries need time to assimilate the new technologies and management practices introduced by China’s FDI (Cui and Jiang, 2010). Concurrently, Chinese enterprises must adapt to the regulatory and institutional frameworks of the host countries (Lo et al., 2016). The influx of new investments can also transform the market environment and resource allocation within the host country, forcing local businesses to adjust to changes in the economic landscape and new competitive pressures (Kokko et al., 1996). This adaptation and adjustment process may initially lead to a decline in the host country’s GTFP. Moreover, the Chinese government encourages enterprises to undertake large-scale projects as part of their FDI activities (Yang and Stoltenberg, 2014), often involving significant infrastructure construction such as roads, bridges, and factories (Wang and Gao, 2019). Such construction typically demands large quantities of cement, steel, and fossil fuels, resulting in substantial increases in greenhouse gas emissions and other pollutants (Naboureh et al., 2021).
As China’s FDI progresses beyond the initial adjustment period and construction phases, its positive effects on the GTFP of host countries become apparent in the medium to long term, manifesting in four key aspects. First, China’s FDI facilitates green technology transfer and innovation in host countries through technology transfer and spillover effects (Qin et al., 2022). Supported by preferential policies, Chinese enterprises introduce advanced technologies and expertise via FDI, enabling local enterprises to enhance their production processes and efficiency. Interactions and collaborations with technologically advanced Chinese companies further promote the dissemination and innovation of green production technologies, thereby enhancing the green production efficiency of host countries (Khachoo and Sharma, 2016; Deng et al., 2023). For instance, Chinese renewable energy investments in Pakistan have introduced cutting-edge solar and wind technologies, fostering the local spread and adoption of renewable energy technologies (Doytch and Narayan, 2016). Second, China’s FDI contributes to the advancement and diffusion of local green technologies in the medium to long term through education and training related to green technologies and practices (Sun et al., 2024). Collaborations with local companies, governments, and institutions in technology promotion enhance the progress and adoption of local green technologies (Chou et al., 2014). For example, Chinese investments in Africa’s agriculture sector include sustainable agricultural technologies and water-saving training programs, aiding local farmers in adopting greener production methods. Third, China’s FDI brings demonstrative and competitive effects to host country enterprises. Technologically advanced Chinese companies illustrate the benefits of green technologies and practices through their investments, and their market entry often intensifies competition in host countries, spurring local enterprises to innovate and improve their green production efficiency (Cheung and Ping, 2004). Fourth, high-quality China’s FDI aids in enhancing environmental policies and regulations in host countries. Recent China’s FDI frequently targets sectors such as renewable energy and electric vehicles, which necessitate adherence to high environmental standards. These practices introduce higher environmental benchmarks in host countries, promoting green and sustainable development (Adeel-Farooq et al., 2021). Furthermore, through collaboration with local governments, these investments help improve environmental regulations (Jin et al., 2019). Over the long term, improvements in environmental laws and policies can enhance the GTFP of host countries (Ren et al., 2022; Sun H. et al., 2023).
Derived from the above analysis, our paper proposes the Hypothesis 1a and 1b:
Hypothesis 1a. (H1a):. China’s FDI has a negative impact on the GTFP of host countries in the short term.
Hypothesis 1b. (H1b):. China’s FDI promotes the growth of GTFP in host countries in the medium to long term.
Previous studies have suggested that FDI can create a pollution haven effect in countries with weak environmental regulations, exacerbating local pollution and suppressing GTFP (Marques and Caetano, 2020). However, China’s FDI, influenced by China’s relevant policies and strategic priorities, does not necessarily lead to a pollution haven effect. Even in countries with lower environmental regulations, China’s FDI can effectively promote the growth of the host country’s GTFP. This is due, firstly, to China’s proactive national strategy on climate change, its active participation in green initiatives including the Paris Agreement, and its consistent emphasis on sustainable development in both domestic and foreign investments (Li et al., 2011; Guo et al., 2013). In 2021, China’s Ministry of Commerce and Ministry of Ecology and Environment jointly issued the “Guidelines for Green Development in Foreign Investment and Cooperation,” guiding Chinese enterprises to promote green development in their foreign investment and cooperation activities. Within China’s Belt and Road Initiative (BRI), green, low-carbon, and sustainable development are prioritized (Yin, 2019). In advancing the BRI, China has adopted a series of measures including green infrastructure, green energy, green transportation, and green finance (Coenen et al., 2021; Harlan, 2021), and has initiated the BRI Green Development International Coalition with more than 150 partners from 43 countries, sharing its successful experiences in green and sustainable development with other nations, particularly developing countries, to enhance their GTFP.
Secondly, China holds a leading position in renewable energy technologies such as solar and wind energy and is a major exporter of green technologies (Urban, 2015). China’s FDI often includes the deployment of these green technologies, especially in infrastructure applications, which supports the host countries in obtaining infrastructure that sustains environmental sustainability (Gu and Zhou, 2020; An et al., 2021), such as energy-saving transportation systems, waste management systems, and green urban planning, helping the host countries reduce dependence on fossil fuels and enhance GTFP(Ren et al., 2024). Additionally, China’s FDI typically involves training programs for local workers and managers to ensure that the host countries can effectively utilize and develop green technologies for long-term GTFP growth (Tawiah et al., 2021). The uniqueness of China’s FDI, coupled with its robust policy framework and commitment to sustainable development, ensures that it does not advocate a pollution haven effect. On the contrary, through the construction of green infrastructure and the export of green technologies, China’s FDI can help countries with weak environmental regulations in the long-term development and enhancement of GTFP.
Based on the above analysis, this paper proposes the second hypothesis:
Hypothesis 2. (H2):. China’s FDI does not create a pollution haven effect in countries with weak environmental regulations.
Research and development (R&D) and innovation are pivotal drivers of environmental efficiency in an economy, involving the creation and implementation of new technologies, processes, and practices. The R&D and innovation capabilities of host countries significantly influence the impact of FDI on GTFP. On one hand, the benefits a host country derives from FDI largely depend on its absorptive capacity—the ability to recognize, assimilate, and apply new knowledge and technologies (Sultana and Turkina, 2020). Countries with robust innovation capacities are more adept at integrating and utilizing advanced technologies introduced by FDI, enabling investment projects to quickly adapt and optimize foreign technologies to local conditions, thereby maximizing productivity and minimizing environmental impact. On the other hand, FDI can generate spillover effects in the host country, where advanced technologies and practices diffuse to local businesses through labor mobility, inter-firm cooperation, and supplier relationships (Jordaan, 2017). Host countries with strong innovation capabilities typically possess advanced R&D infrastructure and skilled labor forces, which can more effectively assimilate and leverage the technologies and practices brought by foreign investors. By integrating foreign technologies, these countries can produce synergistic effects and undertake complementary innovations (Fu, 2008). Therefore, host countries with high levels of innovation can amplify the positive spillover effects of FDI, significantly enhancing its impact on GTFP. Based on the above, we propose the following hypothesis:
Hypothesis 3. (H3):. The level of innovation in host countries enhances the positive impact of FDI on GTFP.
To account for the impact of energy consumption and greenhouse gas emissions on the green development of host countries, and to facilitate efficiency analysis, we use the Directional Distance Function based on the Slacks-Based Measure (SBM) model, as proposed by Tone (2001). This method uses a non-radial and non-oriented approach to measure the GTFP of host countries receiving China’s FDI. The formula for calculating GTFP is as follows:
In Eq. 1, each country is posited as a decision-making unit (DMU), with each DMU consisting of
To further investigate the factors influencing changes in GTFP, we introduce the constraint
For the desirable output variable, we use the Gross National Product (GNP) of each country, and for the undesirable output, we use each country’s greenhouse gas emissions. The input variables include the total labor force, capital stock, and total energy supply measured in heat units. Based on Eqs 1–3, and employing the SBM-DEA method, we calculate the GTFP of host countries receiving China’s FDI. The data for the labor force and greenhouse gas emissions are sourced from the World Bank, the energy supply data measured in heat units is obtained from the International Energy Agency, and the capital stock data comes from the Penn World Table (Feenstra et al., 2015).
Previous research indicates that GTFP is influenced by various persistent drivers (Zhou et al., 2019b; Zhang et al., 2021). Additionally, the impact of FDI on host countries exhibits lag effects (Durham, 2004; Mustafa and Santhirasegaram, 2013). Therefore, we incorporate lagged terms of GTFP and China’s FDI into our baseline model. We utilize the following dynamic panel model to examine the influence of China’s FDI on the GTFP of host countries:
In Eq. 4,
To examine the moderating effects of environmental regulations and innovation levels on the impact of China’s FDI on the GTFP of host countries, considering the lagged influence of China’s FDI, we adopt an approach inspired by Hansen (1999). We introduce a modified dynamic panel threshold model, using the host country’s environmental regulations and innovation levels as threshold variables and the lagged terms of China’s FDI as explanatory variables, to construct a dynamic panel threshold regression model:
In Eqs 5, 6,
The values of GTFP, Pure Technical Efficiency (PTE), and Scale Efficiency (SE) for 123 countries are measured using the SBM-DEA method and serve as the dependent variables.
China’s outward FDI includes both stock and flow data. Due to significant reverse investment activities by China, measuring the flow of China’s direct investment in other countries can lead to substantial bias. Therefore, we use the stock of China’s FDI in host countries as the explanatory variable.
Environmental Regulation (ER): Previous studies emphasize that the level of pollutant management can effectively reflect a country’s commitment to environmental protection (Zorpas, 2020; Li and Shi, 2021). Therefore, we use the mean of three scores related to environmental protection and pollutant management from the Environmental Performance Index (EPI) (Wolf et al., 2022) as proxy variables for environmental regulation. These scores are: Controlled Solid Waste (MSW), Wastewater Treatment (WWT), and Ocean Plastic Pollution (OCP). The EPI is published biennially, and missing data are calculated using interpolation methods.
Innovation Level (IL): Following the approach of O’Neale and Hendy (2012) and Mussaiyib and Pradhan (2023), we use the number of patent applications per thousand residents as a proxy variable for measuring a country’s innovation levels. This variable is calculated by dividing the number of patent applications filed by residents of each country by the total population, with patent application data and population data sourced from the World Bank database.
To account for potential economic and social factors that could influence GTFP, this study incorporates the following control variables into the GMM model and panel threshold model:
Technological Progress (lnTP): Technological advancement is widely recognized as a decisive driver of GTFP growth (Wang et al., 2018; Yang X. et al., 2021). Following Yang X. et al. (2021), the total number of resident patent applications is used as a proxy for technological progress, with a logarithmic transformation applied to enhance data stability.
Environmental Regulation (ER): Beyond legislative frameworks, environmental regulation plays a crucial role in promoting economic efficiency, environmental sustainability, encouraging green technological innovation, and ensuring the effective use of resources (Yuan and Xiang, 2018; Zhang and Vigne, 2021). Given its profound impact on GTFP dynamics, ER is included in our analysis both as a key threshold variable and as a control variable. This dual inclusion allows us to dissect the nuanced impacts of environmental policies on GTFP adjustments.
Level of Economic Growth (RGDP): Economic growth is a key factor driving changes in a country’s GTFP (Sun Y. et al., 2023; Wang et al., 2023), represented by the GDP growth rate.
Industrial Structure (IS): The optimization and adjustment of industrial structure can reduce carbon emissions and enhance overall productivity, significantly impacting GTFP (Yang et al., 2022). Following Wang et al. (2021), the percentage of the secondary industry’s output to GDP is used to represent this variable.
Descriptions of each variable are presented in Table 1.
This study constructed a panel dataset comprising data from 123 countries spanning from 2007 to 2019. Data on China’s foreign direct investment (FDI) in other countries was sourced from the annual Statistical Bulletin of China’s Outward Foreign Direct Investment published by the National Bureau of Statistics of China. Environmental regulation scores, derived from three metrics, were obtained from the biennially published Environmental Performance Index. Additional data were sourced from the World Bank database. To mitigate heteroscedasticity and standardize the magnitude of variables, logarithmic transformations were applied to the stock of China’s FDI and the total number of resident patent applications. The GTFP index, technological progress index, and technical efficiency index, as well as the GDP growth rate, labor participation rate, and industrialization rate, were all multiplied by 100 to convert these values into percentages. Table 2 presents the descriptive statistics of each variable.
Using Stata 17 software, a series of tests were conducted on Eq. 4 to select the appropriate econometric model. The estimation results are presented in Table 3. Models I, II, and III represent the estimation results of random effects, fixed effects, and Ordinary Least Squares (OLS), respectively. Model IV represents the estimation results of the two-step system GMM.
In estimating Eq. 4, the inclusion of lagged dependent variables on the right side of the equation introduces endogeneity issues, leading to potential biases in estimations using random effects (RE), fixed effects (FE), and pooled Ordinary Least Squares (OLS). However, the Generalized Method of Moments (GMM) estimation technique mitigates these endogeneity problems through instrumental variables, with the system GMM further addressing issues of weak instruments (Blundell and Bond, 1998). The two-step system GMM requires the absence of second-order autocorrelation in the error terms and the exogeneity of the instruments. Models IV to VI all perform well in this regard; the Arellano-Bond test for autocorrelation does not reject the null hypothesis of no second-order serial correlation, and the Hansen test indicates no overidentification issues. As shown in Model IV of Table 3, the estimates from the two-step system GMM are more significant than those from Models I, II, III. Therefore, we refer to the two-step system GMM estimates in our analysis.
The estimation results reveal that China’s FDI has a significant impact on the host country’s GTFP index, as well as on the Pure Technical Efficiency (PTE) and Scale Efficiency (SE) derived from GTFP changes. Furthermore, the effect varies between the current and lagged periods, with the impact in the lagged period being greater than in the current period, highlighting the lagged nature of the effects of China’s FDI on the GTFP of host countries.
From the coefficients of current-period China’s FDI in the System GMM model, it is observed that a 1% increase in China’s FDI results in a 0.087% decrease in the host country’s current-period GTFP, a 0.044% decrease in Pure Technical Efficiency (PTE), and a 0.009% increase in Scale Efficiency (SE). This suggests that China’s FDI initially suppresses the host country’s GTFP and green technology level but positively impacts scale efficiency. This effect can be attributed to several factors: primarily, during the current period of China’s FDI, the influence is largely due to the construction process of the projects, which might be inefficient and result in significant greenhouse gas emissions, thus reducing the host country’s GTFP and technical efficiency. However, the construction process also contributes to increased production scale and market expansion in the host country, which in turn can enhance scale efficiency. Furthermore, host country enterprises may face challenges in absorbing and integrating new management experiences and technologies. In the initial phase of project introduction, new investments and operational practices might not fully align with the existing environment of the host country. Host country enterprises need time to learn and adapt to the newly introduced managerial expertise and technologies, a process that can temporarily decrease GTFP and technical efficiency.
The coefficients of lagged China’s FDI in the System GMM regression model indicate that a 1% growth in China’s FDI leads to a 0.222% increase in the host country’s GTFP in the following year, a 0.141% increase in PTE, and a 0.034% decrease in Scale Efficiency (SE). This demonstrates that as projects begin operation, China’s FDI effectively promotes growth in the host country’s GTFP and green technology levels, albeit with a slight negative impact on scale efficiency. The reasons for these impacts include the gradual absorption by host country enterprises of the management experience and technology brought by China’s FDI, which significantly enhances their technological levels and operational efficiency. Additionally, Chinese investment introduces more intense market competition and environmental awareness to the host country’s market, which, in the long run, encourages host country enterprises to optimize operations and reduce waste, thereby improving their technological levels and increasing GTFP. On the other hand, as projects are completed and move into the operational phase, the host country faces changes in resource and product demand structures, which might lead to overcapacity in some industries, thus reducing scale efficiency.
Through the analysis using the GMM model, it is found that in the current period of FDI, due to inefficient construction processes and challenges in technology absorption faced by the host country, China’s FDI slightly and temporarily reduces the host country’s GTFP and technical levels. However, over time, as host country enterprises absorb and integrate management experiences and technologies, along with the market competition and environmental awareness brought by Chinese investments, they can effectively promote green technology and enhance GTFP in the host country, far outweighing the initial suppressive effects, although scale efficiency may decline slightly with changes in production and demand structures.
To mitigate potential biases arising from single-variable measurements, we replaced both the independent and dependent variables and re-estimated the models accordingly. Specifically, we replaced the independent variable with the flow of China’s FDI. Since the FDI flow data released by the Chinese National Bureau of Statistics include reverse FDI, which cannot accurately measure the changes in China’s FDI flow to different countries, we processed the FDI stock data through differencing to derive the flow data of China’s FDI for use as the independent variable.
For the dependent variable, we employed Carbon Emission Intensity (CEI), defined as the amount of carbon dioxide emissions per unit of Gross Domestic Product (GDP) for a given country. A reduction in CEI indicates a transition to more efficient production processes, emphasizing the enhancement of green production practices. According to Zhang et al. (2020), CEI is a critical indicator for assessing the level of environmentally sustainable production. Furthermore, empirical evidence provided by Wang and Yan (2022) shows a negative correlation between CEI and GTFP, reinforcing the suitability of CEI as a converse indicator for GTFP. Consequently, CEI (measured in grams per Purchasing Power Parity (PPP) dollar of GDP) is used as an inverse proxy for GTFP. The data on carbon emission intensity are sourced from the World Bank database.
Upon replacing the variables and re-estimating, all models passed the Arellano-Bond test for serial correlation and the Hansen test, and the coefficients of China’s FDI in each model were statistically significant at the 1% level. The empirical results are presented in Table 4.
In Table 4, Models I, II, and III display the estimation results of the system GMM after replacing the independent variable. The coefficients between current-period China’s FDI and both GTFP and PTE are significantly negative, while the coefficients with SE are significantly positive. The coefficients between lagged China’s FDI and both GTFP and PTE are significantly positive, whereas those with SE are significantly negative, indicating that the impact of lagged China’s FDI on GTFP is greater than that of current-period FDI.
Model IV shows the system GMM estimation results after replacing the dependent variable. The coefficient between current-period China’s FDI and the host country’s carbon emission intensity is significantly positive, suggesting that current China’s FDI increases the carbon emissions per unit of GDP in the host country, thereby reducing the host country’s GTFP. However, the coefficient between lagged China’s FDI and the host country’s carbon emission intensity is significantly negative, and its absolute value is greater than that of the coefficient for current-period FDI, indicating that over time, China’s FDI can reduce carbon emissions per unit of GDP in the host country, thereby increasing its GTFP. Overall, the estimation results after replacing both the independent and dependent variables are consistent with the aforementioned test outcomes.
In 2017, the Chinese government implemented a series of policies that significantly tightened restrictions on FDI, resulting in a 36% year-on-year decline in the scale of China’s FDI, marking the first negative growth since China started publishing FDI statistics in 2003. To mitigate the anomalous impact of changes in the Chinese government’s FDI management policies on the estimation results, we excluded the observational data for 2017 and re-estimated using the two-step system GMM. All models passed the Arellano-Bond test for serial correlation and the Hansen test, and the coefficients of China’s FDI in each model were statistically significant at the 1% level, as shown in Table 5.
In Table 5, Models I, II, and III list the two-step system GMM estimation results based on the adjusted sample. The coefficients between current-period China’s FDI and both GTFP and PTE are negative, whereas those with SE are positive. The coefficients between lagged China’s FDI and both GTFP and PTE are positive, whereas those with SE are negative, with all coefficients being statistically significant at the 1% level. The coefficients between lagged FDI and both GTFP and PTE are higher than those for current-period FDI. After adjusting the sample, the estimation results remain robust.
To further ensure the robustness of the model, we removed all control variables and re-estimated using the two-step system GMM. All models passed the Arellano-Bond test for serial correlation and the Hansen test, and the coefficients of China’s FDI in each model were statistically significant at the 1% level. The estimation results are presented in Table 6.
In Table 6, the size and signs of the coefficients between both current and lagged China’s FDI and GTFP and PTE remain consistent with previous research findings. The coefficient between current China’s FDI and SE turned negative; however, its absolute value is only 0.005, indicating a minimal potential reduction effect of current China’s FDI on the host country’s scale efficiency. Nevertheless, the coefficient between lagged China’s FDI and SE remains significantly negative, with an absolute value of 0.05. Overall, in the absence of control variables, the estimation results of the model are broadly consistent with previous results.
Previous analysis based on linear models confirmed that China’s FDI have a significant positive effect on the GTFP of host countries, with this impact exhibiting a lagged nature. Consequently, we use lagged China’s FDI as the explanatory variable and the environmental regulations and innovation levels of host countries as threshold variables to further explore the nonlinear impact of China’s FDI on host countries’ GTFP.
We obtained the asymptotic values of the F-statistics and corresponding p-values through threshold effect bootstrap testing, followed by tests for threshold values. The results of the threshold effect test for environmental regulations in the host country are presented in Table 7 and Figure 2. The three-threshold test yielded an F-statistic of 34.82, which did not pass the significance test, indicating the absence of a triple threshold. The double threshold test’s F-statistic was 39.67, significant at the 5% level, indicating a double threshold effect. The first and second threshold values are 0.5000 and 0.5629, respectively, with confidence intervals as shown in Table 8 and Figure 2.
The threshold effect test results for the innovation level of the host country are shown in Table 7 and Figure 3 The three-threshold test resulted in an F-statistic of 8.31, which did not pass the significance test, indicating the absence of a triple threshold. The double threshold test’s F-statistic was 28.68, significant at the 1% level, revealing a double threshold effect. The first and second threshold values are 20.9542 and 50.9190, respectively, with confidence intervals as detailed in Table 8 and Figure 3.
The test results for threshold effects indicate that the impact of China’s FDI on GTFP in host countries with different levels of environmental regulation and innovation displays nonlinear characteristics, suggesting the feasibility of establishing a threshold panel model.
Based on the estimation of parameters using Eqs 5, 6, the results of the dynamic panel threshold effects model are presented in Table 9.
Model I in Table 9 illustrates the nonlinear characteristics of the impact of China’s FDI on the GTFP of host countries, taking into account environmental regulations (ER). Contrary to many existing studies on environmental regulation, the impact of China’s FDI on the GTFP of host countries decreases as their ER increases. When examining the coefficients of L.lnFDI, we observe that when the ER of the host country is low (ER ≤ 0.052), the growth effect of China’s FDI on GTFP is most pronounced. Specifically, a 1% increase in investment stock results in a 0.621% rise in the host country’s GTFP in the following year. As ER increases to a medium level (0.427 < ER ≤ 0.4337), the coefficient of L.lnFDI decreases to 0.413. At higher levels of ER (ER > 0.4337), the coefficient further declines to 0.249. Despite these variations, the coefficients of L.lnFDI remain significant at the 1% level across all ER levels. These findings indicate that China’s FDI promotes GTFP growth in host countries regardless of their ER levels, challenging the pollution haven hypothesis. Notably, the results reveal that in host countries with lower levels of environmental regulation, the positive effect of China’s FDI on GTFP not only persists but also intensifies. This suggests that China is actively introducing clean and efficient technologies through FDI, thereby enhancing green productivity in these countries. In contrast, countries with higher levels of environmental regulation typically already possess advanced green production capabilities (Yang L. et al., 2021). As a result, the potential for further improvements in green production through China’s FDI diminishes as ER becomes more stringent. Consequently, the growth benefits of China’s FDI on GTFP in these countries gradually weaken.
Model II in Table 9 demonstrates the nonlinear impact characteristics of China’s FDI on the GTFP of host countries under different innovation levels (IL). Specifically, when the IL of the host country is low (IL ≤ 0.053), China’s FDI has a significant positive effect on the host country’s GTFP, with each 1% increase in investment increasing the host country’s GTFP by 0.224%. As the innovation level of the host country increases (0.053<IL ≤ 0.1481), the regression coefficient of FDI on GTFP rises to 0.235%, and when the innovation level increases further (IL > 50.9190), the regression coefficient significantly increases to 0.372% and is significant at the 1% level. This indicates that China’s FDI leads to the transfer and spillover of green technology and management expertise, but the growth effect it brings to the host country’s GTFP is influenced by the host country’s innovation level: the higher the innovation level, the quicker and more efficiently the host country can accept and learn the technology transferred from China, and implement its diffusion domestically (Qin et al., 2022), thereby increasing the growth effect of China’s FDI on the host country’s GTFP.
To ensure the robustness of the threshold effects model, we replaced the threshold variables and re-estimated the model. According to Gaballah and Kanari (2001), the recycling rate (REC) of countries is largely influenced by environmental regulations and sustainable development policies. Thus, we utilized REC as a new threshold variable for measuring environmental regulation, with the recycling rate data sourced from the EPI database. Similarly, Research and Development (R&D) intensity (the percentage of R&D expenditure in GDP) is widely used to measure a country’s innovation level (Bointner, 2014; Khayyat and Lee, 2015). Therefore, we used R&D/GDP as the new threshold variable for measuring innovation level, with the R&D/GDP data obtained from the World Bank database. The threshold effect tests for the new threshold variables showed double threshold effects at a 1% significance level. The estimation results of the dynamic panel threshold effects are displayed in Table 10.
Model I in Table 10 shows the nonlinear impact of China’s FDI on the GTFP of host countries under the new environmental regulation variable. It is observed that when the host country’s environmental regulation level is low (REC≤0.6584), the growth effect of China’s FDI on the host country’s GTFP is highest. As the level of environmental regulation in the host country exceeds the first threshold (0.6584<REC≤0.9471), the growth benefits of China’s FDI weaken. When the host country’s environmental regulation surpasses the second threshold, the impact of China’s FDI on the host country’s GTFP becomes insignificant.
Model II in Table 10 illustrates the nonlinear impact of China’s FDI on the GTFP of host countries when R&D intensity is used as the measure of innovation level. When R&D intensity is below the first threshold (R&D/GDP≤1.1097) and between the first and second thresholds (1.1097 < R&D/GDP≤2.5976), the impact of China’s FDI on the host country’s GTFP is very close, with coefficients of 0.239 and 0.231 respectively. When R&D intensity is above the second threshold (R&D/GDP>2.5967), the impact of China’s FDI on the host country’s GTFP significantly increases to 0.442.
The estimation results after replacing the threshold variables are largely consistent with previous regression results, reflecting the robustness of the threshold effects model. Specifically, first, China’s FDI does not exhibit a ‘pollution haven effect.’ For countries with lower environmental regulation levels, China’s FDI can more effectively enhance their GTFP. However, as the environmental regulation level of the host country increases, the potential for GTFP improvement gradually diminishes, and the promotional effect of China’s FDI on the host country’s GTFP weakens. Second, China’s FDI facilitates the transfer and diffusion of green technologies, and its impact on the host country’s GTFP is influenced by the host country’s innovation level—the stronger the innovation level, the greater the extent to which China’s FDI can promote GTFP growth in the host country.
This study evaluates the GTFP of 123 host countries of China’s FDI from 2007 to 2019 using the super-efficiency SBM model. It then empirically assesses the impact of China’s FDI on the GTFP of these host countries utilizing a two-step system GMM model and a dynamic panel threshold effect model. The findings reveal three key insights:
The findings reveal that China’s FDI significantly enhances the GTFP of host countries, particularly over the medium to long term. Importantly, the data do not support the existence of a “pollution haven effect.” Instead, China’s FDI tends to boost GTFP more effectively in countries with less stringent environmental regulations, underscoring the role of such investments in promoting green growth.
First, China’s FDI significantly enhances the GTFP of host countries. While China’s FDI may initially have a negative impact on GTFP in the short term, it effectively promotes GTFP growth in the medium to long term, primarily through improvements in technological efficiency.
Second, China’s FDI does not induce a “pollution haven effect.” Contrary to findings in other related studies, our results indicate that China’s FDI can more substantially boost GTFP in countries with lower levels of environmental regulation.
Third, the positive impact of China’s FDI on the GTFP of host countries is amplified by higher levels of innovation. As the innovation levels of host countries improve, the beneficial effects of China’s FDI on GTFP increase correspondingly.
Based on the research results, this paper proposes the following policy implications:
First, host countries should strengthen their environmental regulations and innovation systems to maximize the benefits derived from China’s green FDI. This approach not only leverages FDI for environmental gains but also enhances the local economic and technological landscape.
Second, China should continue to integrate sustainability into its FDI strategies, aiming to reduce adverse environmental impacts during project construction and operation. This commitment will help mitigate the initial negative impacts on GTFP while supporting global green development goals.
This study empirically identifies the mechanisms and patterns through which China’s FDI affects the GTFP of host countries and examines the heterogeneity of this impact based on the levels of environmental regulation and innovation in host countries, providing persuasive conclusions. However, there are limitations to this research. Due to the lack of relevant data, the calculation of GTFP only considered undesired environmental outputs from the perspective of greenhouse gas emissions, omitting the impact of other types of pollution on GTFP. Furthermore, the analysis did not disaggregate China’s FDI by industry type. A comparative analysis of different types of FDI on host countries’ GTFP could better elucidate the mechanisms by which FDI influences GTFP. Future studies should consider a broader array of pollution types in the calculation of GTFP and analyze the impact of FDI based on the characteristics of different industries.
The original contributions presented in the study are included in the article/Supplementary material, further inquiries can be directed to the corresponding author.
ZP: Conceptualization, Data curation, Formal Analysis, Investigation, Methodology, Software, Supervision, Validation, Writing–original draft, Writing–review and editing. YH: Project administration, Visualization, Writing–review and editing. RX: Formal Analysis, Funding acquisition, Methodology, Resources, Writing–review and editing.
The author(s) declare that financial support was received for the research, authorship, and/or publication of this article. This study was supported by the Youth Program of the Social Science Department of the Ministry of Education (No. 23YJC630056) and the Tongling University Scientific Research Project (Grant No.2020tlxy19; No.2021tlxy06).
The authors declare that the research was conducted in the absence of any commercial or financial relationships that could be construed as a potential conflict of interest.
All claims expressed in this article are solely those of the authors and do not necessarily represent those of their affiliated organizations, or those of the publisher, the editors and the reviewers. Any product that may be evaluated in this article, or claim that may be made by its manufacturer, is not guaranteed or endorsed by the publisher.
Adeel-Farooq, R. M., Riaz, M. F., and Ali, T. (2021). Improving the environment begins at home: revisiting the links between FDI and environment. Energy 215, 119150. doi:10.1016/j.energy.2020.119150
An, H., Razzaq, A., Nawaz, A., Noman, S. M., and Khan, S. A. R. (2021). Nexus between green logistic operations and triple bottom line: evidence from infrastructure-led Chinese outward foreign direct investment in Belt and Road host countries. Environ. Sci. Pollut. Res. 28 (37), 51022–51045. doi:10.1007/s11356-021-12470-3
Benzaim, S., Ftiti, Z., Khedhaouria, A., and Djermane, R. (2023). US foreign investments: technology transfer, relative backwardness, and the productivity growth of host countries. Q. Rev. Econ. Finance 87, 275–295. doi:10.1016/j.qref.2021.03.012
Blundell, R., and Bond, S. (1998). Initial conditions and moment restrictions in dynamic panel data models. J. Econ. 87 (1), 115–143. doi:10.1016/s0304-4076(98)00009-8
Bointner, R. (2014). Innovation in the energy sector: lessons learnt from R&D expenditures and patents in selected IEA countries. Energy policy 73, 733–747. doi:10.1016/j.enpol.2014.06.001
Chen, W., Dollar, D., and Tang, H. (2018). Why is China investing in Africa? Evidence from the firm level. World Bank Econ. Rev. 32 (3), 610–632.
Cheung, K.-y., and Ping, L. (2004). Spillover effects of FDI on innovation in China: evidence from the provincial data. China Econ. Rev. 15 (1), 25–44. doi:10.1016/s1043-951x(03)00027-0
Chou, T.-L., Chang, J.-Y., and Li, T.-C. (2014). Government support, FDI clustering and semiconductor sustainability in China: case studies of Shanghai, Suzhou and Wuxi in the Yangtze delta. Sustainability 6 (9), 5655–5681. doi:10.3390/su6095655
Coenen, J., Bager, S., Meyfroidt, P., Newig, J., and Challies, E. (2021). Environmental governance of China's belt and road initiative. Environ. Policy Gov. 31 (1), 3–17. doi:10.1002/eet.1901
Copeland, B. R., and Taylor, M. S. (2004). Trade, growth, and the environment. J. Econ. literature 42 (1), 7–71. doi:10.1257/002205104773558047
Cui, L., and Jiang, F. (2010). Behind ownership decision of Chinese outward FDI: resources and institutions. Asia Pac. J. Manag. 27, 751–774. doi:10.1007/s10490-009-9136-5
Danish, , Baloch, M. A., and Suad, S. (2018). Modeling the impact of transport energy consumption on CO 2 emission in Pakistan: evidence from ARDL approach. Environ. Sci. Pollut. Res. 25, 9461–9473. doi:10.1007/s11356-018-1230-0
Deng, X., Li, W., and Ren, X. (2023). More sustainable, more productive: evidence from ESG ratings and total factor productivity among listed Chinese firms. Finance Res. Lett. 51, 103439. doi:10.1016/j.frl.2022.103439
Desbordes, R., and Franssen, L. (2019). Foreign direct investment and productivity: a cross-country, multisector analysis. Asian Dev. Rev. 36 (1), 54–79. doi:10.1162/adev_a_00123
Doytch, N., and Narayan, S. (2016). Does FDI influence renewable energy consumption? An analysis of sectoral FDI impact on renewable and non-renewable industrial energy consumption. Energy Econ. 54, 291–301. doi:10.1016/j.eneco.2015.12.010
Durham, J. B. (2004). Absorptive capacity and the effects of foreign direct investment and equity foreign portfolio investment on economic growth. Eur. Econ. Rev. 48 (2), 285–306. doi:10.1016/s0014-2921(02)00264-7
Feenstra, R. C., Inklaar, R., and Timmer, M. P. (2015). The next generation of the Penn world table. Am. Econ. Rev. 105 (10), 3150–3182. doi:10.1257/aer.20130954
Fu, X. (2008). Foreign direct investment, absorptive capacity and regional innovation capabilities: evidence from China. Oxf. Dev. Stud. 36 (1), 89–110. doi:10.1080/13600810701848193
Gaballah, I., and Kanari, N. (2001). Recycling policy in the European union. JOM 53 (11), 24–27. doi:10.1007/s11837-001-0189-2
Gallagher, K. S., and Qi, Q. (2021). Chinese overseas investment policy: implications for climate change. Glob. Policy 12 (3), 260–272. doi:10.1111/1758-5899.12952
Gu, A., and Zhou, X. (2020). Emission reduction effects of the green energy investment projects of China in belt and road initiative countries. Ecosyst. Health Sustain. 6 (1), 1747947. doi:10.1080/20964129.2020.1747947
Guo, X., Marinova, D., and Hong, J. (2013). China's shifting policies towards sustainability: a low-carbon economy and environmental protection. J. Contemp. China 22 (81), 428–445. doi:10.1080/10670564.2012.748962
Haiyue, L., and Manzoor, A. (2020). The impact of OFDI on the performance of Chinese firms along the Belt and Road. Appl. Econ. 52 (11), 1219–1239. doi:10.1080/00036846.2019.1659501
Hansen, B. E. (1999). Threshold effects in non-dynamic panels: estimation, testing, and inference. J. Econ. 93 (2), 345–368. doi:10.1016/s0304-4076(99)00025-1
Harlan, T. (2021). Green development or greenwashing? A political ecology perspective on China’s green Belt and Road. Eurasian Geogr. Econ. 62 (2), 202–226. doi:10.1080/15387216.2020.1795700
Hu, D., You, K., and Esiyok, B. (2021). Foreign direct investment among developing markets and its technological impact on host: evidence from spatial analysis of Chinese investment in Africa. Technol. Forecast. Soc. Change 166, 120593. doi:10.1016/j.techfore.2021.120593
Jin, J., Du, J., Long, X., and Boamah, K. B. (2019). Positive mechanism of foreign direct investment enterprises on China's environment: analysis of host country regulation and parent company management. J. Clean. Prod. 227, 207–217. doi:10.1016/j.jclepro.2019.04.143
Jordaan, J. A. (2017). Producer firms, technology diffusion and spillovers to local suppliers: examining the effects of Foreign Direct Investment and the technology gap. Environ. Plan. A Econ. Space 49 (12), 2718–2738. doi:10.1177/0308518x17731942
Khachoo, Q., and Sharma, R. (2016). FDI and innovation: an investigation into intra-and inter-industry effects. Glob. Econ. Rev. 45 (4), 311–330. doi:10.1080/1226508x.2016.1218294
Khayyat, N. T., and Lee, J.-D. (2015). A measure of technological capabilities for developing countries. Technol. Forecast. Soc. Change 92, 210–223. doi:10.1016/j.techfore.2014.09.003
Kokko, A., Tansini, R., and Zejan, M. C. (1996). Local technological capability and productivity spillovers from FDI in the Uruguayan manufacturing sector. J. Dev. Stud. 32 (4), 602–611. doi:10.1080/00220389608422430
Li, P., and Shi, L.-L. (2021). Do environmental regulations improve industrial efficiency? Adv. Civ. Eng. 2021, 1–11. doi:10.1155/2021/1979353
Li, Y., Wu, Y., Chen, Y., and Huang, Q. (2021). The influence of foreign direct investment and trade opening on green total factor productivity in the equipment manufacturing industry. Appl. Econ. 53 (57), 6641–6654. doi:10.1080/00036846.2021.1947961
Li, Y., Yang, X., Zhu, X., Mulvihill, P. R., Matthews, H. D., and Sun, X. (2011). Integrating climate change factors into China's development policy: adaptation strategies and mitigation to environmental change. Ecol. Complex. 8 (4), 294–298. doi:10.1016/j.ecocom.2011.08.001
Liu, Z., and Xin, L. (2019). Has China's Belt and Road Initiative promoted its green total factor productivity? evidence from primary provinces along the route. Energy Policy 129, 360–369. doi:10.1016/j.enpol.2019.02.045
Lo, D., Hong, F., and Li, G. (2016). Assessing the role of inward foreign direct investment in Chinese economic development, 1990–2007: towards a synthesis of alternative views. Struct. Change Econ. Dyn. 37, 107–120. doi:10.1016/j.strueco.2016.01.004
Lu, C., Zhang, X., and He, J. (2010). A CGE analysis to study the impacts of energy investment on economic growth and carbon dioxide emission: a case of Shaanxi Province in western China. Energy 35 (11), 4319–4327. doi:10.1016/j.energy.2009.04.007
Luo, Y., Lu, Z., Wu, C., and Mensah, C. N. (2023). Environmental regulation effect on green total factor productivity: mediating role of foreign direct investment quantity and quality. Int. J. Environ. Res. Public Health 20 (4), 3150. doi:10.3390/ijerph20043150
Ma, T., and Cao, X. (2022). FDI, technological progress, and green total factor energy productivity: evidence from 281 prefecture cities in China. Environ. Dev. Sustain. 24 (9), 11058–11088. doi:10.1007/s10668-021-01897-w
Mahadevan, R., and Sun, Y. (2020). Effects of foreign direct investment on carbon emissions: evidence from China and its Belt and Road countries. J. Environ. Manag. 276, 111321. doi:10.1016/j.jenvman.2020.111321
Marques, A. C., and Caetano, R. (2020). The impact of foreign direct investment on emission reduction targets: evidence from high-and middle-income countries. Struct. Change Econ. Dyn. 55, 107–118. doi:10.1016/j.strueco.2020.08.005
Mussaiyib, A. M., and Pradhan, K. C. (2023). Examining the impact of innovations on economic growth in BRICS countries. Glob. Bus. Rev., 09721509231152032. doi:10.1177/09721509231152032
Mustafa, A., and Santhirasegaram, S. (2013). The impact of foreign direct investment on economic growth in Sri Lanka.
Naboureh, A., Bian, J., Lei, G., and Li, A. (2021). A review of land use/land cover change mapping in the China-Central Asia-West Asia economic corridor countries. Big Earth Data 5 (2), 237–257.
Oladipupo, S. A., and Ajide, F. M. (2023). Environmental effect of Chinese FDI in Africa: evidence from pooled mean group. Sustain. Dev. doi:10.1002/sd.2868
O’Neale, D. R., and Hendy, S. C. (2012). Power law distributions of patents as indicators of innovation. PLoS One 7 (12), e49501. doi:10.1371/journal.pone.0049501
Pan, X., Li, M., Wang, M., Chu, J., and Bo, H. (2020). The effects of outward foreign direct investment and reverse technology spillover on China's carbon productivity. Energy Policy 145, 111730. doi:10.1016/j.enpol.2020.111730
Qin, B., Gai, Y., Ge, L., Sun, P., Yu, Y., and Zheng, Y. (2022). FDI, technology spillovers, and green innovation: theoretical analysis and evidence from China. Energies 15 (20), 7497. doi:10.3390/en15207497
Qiu, S., Wang, Z., and Geng, S. (2021). How do environmental regulation and foreign investment behavior affect green productivity growth in the industrial sector? An empirical test based on Chinese provincial panel data. J. Environ. Manag. 287, 112282. doi:10.1016/j.jenvman.2021.112282
Ramasamy, B., Yeung, M., and Laforet, S. (2012). China's outward foreign direct investment: location choice and firm ownership. J. world Bus. 47 (1), 17–25. doi:10.1016/j.jwb.2010.10.016
Ren, X., Xiao, Y., Xiao, S., Jin, Y., and Taghizadeh-Hesary, F. (2024). The effect of climate vulnerability on global carbon emissions: evidence from a spatial convergence perspective. Resour. Policy 90, 104817. doi:10.1016/j.resourpol.2024.104817
Ren, X., Zhang, X., Yan, C., and Gozgor, G. (2022). Climate policy uncertainty and firm-level total factor productivity: evidence from China. Energy Econ. 113, 106209. doi:10.1016/j.eneco.2022.106209
Sultana, N., and Turkina, E. (2020). Foreign direct investment, technological advancement, and absorptive capacity: a network analysis. Int. Bus. Rev. 29 (2), 101668. doi:10.1016/j.ibusrev.2020.101668
Sun, H., Bahizire, G. M., Pea-Assounga, J. B. B., and Chen, T. (2024). Enhancing employee green performance through green training: the mediating influence of organizational green culture and work ethic in the mining sector. J. Clean. Prod. 449, 141105. doi:10.1016/j.jclepro.2024.141105
Sun, H., Chen, T., and Wang, C. N. (2023a). Spatial impact of digital finance on carbon productivity. Geosci. Front. 101674.
Sun, Y., Tang, Y., and Li, G. (2023b). Economic growth targets and green total factor productivity: evidence from China. J. Environ. Plan. Manag. 66 (10), 2090–2106. doi:10.1080/09640568.2022.2061335
Tawiah, V. K., Zakari, A., and Khan, I. (2021). The environmental footprint of China-Africa engagement: an analysis of the effect of China–Africa partnership on carbon emissions. Sci. Total Environ. 756, 143603. doi:10.1016/j.scitotenv.2020.143603
Tian, G. (2022). How does outward foreign direct investment affect green total factor productivity? Evidence from increment and quality improvement. Sustainability 14 (19), 11833. doi:10.3390/su141911833
Tone, K. (2001). A slacks-based measure of efficiency in data envelopment analysis. Eur. J. operational Res. 130 (3), 498–509. doi:10.1016/s0377-2217(99)00407-5
Urban, F. (2015). Environmental innovation for sustainable development: the role of China. Sustain. Dev. 23 (7-8), 203–205. doi:10.1002/sd.1587
Walter, I., and Ugelow, J. L. (1979). Environmental policies in developing countries. Ambio, 102–109.
Wang, B., and Gao, K. (2019). Forty years development of China's outward foreign direct investment: retrospect and the challenges ahead. China and World Econ. 27 (3), 1–24. doi:10.1111/cwe.12278
Wang, H., Cui, H., and Zhao, Q. (2021). Effect of green technology innovation on green total factor productivity in China: evidence from spatial durbin model analysis. J. Clean. Prod. 288, 125624. doi:10.1016/j.jclepro.2020.125624
Wang, L., and Yan, Y. (2022). Environmental regulation intensity, carbon footprint and green total factor productivity of manufacturing industries. Int. J. Environ. Res. Public Health 19 (1), 553. doi:10.3390/ijerph19010553
Wang, X., Li, J., and Wang, N. (2023). Are economic growth pressures inhibiting green total factor productivity growth? Sustainability 15 (6), 5239. doi:10.3390/su15065239
Wang, X., Sun, C., Wang, S., Zhang, Z., and Zou, W. (2018). Going green or going away? A spatial empirical examination of the relationship between environmental regulations, biased technological progress, and green total factor productivity. Int. J. Environ. Res. Public Health 15 (9), 1917. doi:10.3390/ijerph15091917
Wolf, M. J., Emerson, J. W., Esty, D. C., Sherbinin, A. D., and Wendling, Z. A. (2022). 2022 environmental performance index (EPI) results. New Haven, CT: Yale Center for Environmental Law and Policy.
Xiao, S., Wang, S., Zeng, F., and Huang, W.-C. (2022). Spatial differences and influencing factors of industrial green total factor productivity in Chinese industries. Sustainability 14 (15), 9229. doi:10.3390/su14159229
Xiaofei, X., Yanjuan, C., and Yundi, Z. (2021). Impact of environmental regulation and FDI on green total factor productivity: evidence from China. Environ. Eng. Manag. J. (EEMJ) 20 (2), 177–184. doi:10.30638/eemj.2021.018
Xie, F., and Zhang, B. (2021). Impact of China’s outward foreign direct investment on green total factor productivity in “Belt and Road” participating countries: a perspective of institutional distance. Environ. Sci. Pollut. Res. 28, 4704–4715. doi:10.1007/s11356-020-10849-2
Xie, R., Fu, W., Yao, S., and Zhang, Q. (2021). Effects of financial agglomeration on green total factor productivity in Chinese cities: insights from an empirical spatial Durbin model. Energy Econ. 101, 105449. doi:10.1016/j.eneco.2021.105449
Xu, S., and Zhou, Y. (2023). OFDI, industrial structure upgrading and green development—spatial effect based on China’s evidence. Sustainability 15 (3), 2810. doi:10.3390/su15032810
Yang, L., Zhang, J., and Zhang, Y. (2021a). Environmental regulations and corporate green innovation in China: the role of city leaders’ promotion pressure. Int. J. Environ. Res. Public Health 18 (15), 7774. doi:10.3390/ijerph18157774
Yang, X., Jia, Z., and Yang, Z. (2021b). How does technological progress impact transportation green total factor productivity: a spatial econometric perspective. Energy Rep. 7, 3935–3950. doi:10.1016/j.egyr.2021.06.078
Yang, X., and Stoltenberg, C. (2014). A review of institutional influences on the rise of made-in-China multinationals. Int. J. Emerg. Mark. 9 (2), 162–180. doi:10.1108/ijoem-09-2012-0095
Yang, Y., Wei, X., Wei, J., and Gao, X. (2022). Industrial structure upgrading, green total factor productivity and carbon emissions. Sustainability 14 (2), 1009. doi:10.3390/su14021009
Yao, S., Zhang, F., Wang, P., and Luo, D. (2017). Location determinants of China's outward foreign direct investment. China and World Econ. 25 (6), 1–27. doi:10.1111/cwe.12218
Yin, W. (2019). Integrating Sustainable Development Goals into the Belt and Road Initiative: would it be a new model for green and sustainable investment? Sustainability 11 (24), 6991. doi:10.3390/su11246991
Yu, D., Li, X., Yu, J., and Li, H. (2021). The impact of the spatial agglomeration of foreign direct investment on green total factor productivity of Chinese cities. J. Environ. Manag. 290, 112666. doi:10.1016/j.jenvman.2021.112666
Yuan, B., and Xiang, Q. (2018). Environmental regulation, industrial innovation and green development of Chinese manufacturing: based on an extended CDM model. J. Clean. Prod. 176, 895–908. doi:10.1016/j.jclepro.2017.12.034
Zhang, D., and Vigne, S. A. (2021). How does innovation efficiency contribute to green productivity? A financial constraint perspective. J. Clean. Prod. 280, 124000. doi:10.1016/j.jclepro.2020.124000
Zhang, J., Fan, Z., Chen, Y., Gao, J., and Liu, W. (2020). Decomposition and decoupling analysis of carbon dioxide emissions from economic growth in the context of China and the ASEAN countries. Sci. Total Environ. 714, 136649. doi:10.1016/j.scitotenv.2020.136649
Zhang, J., Lu, G., Skitmore, M., and Ballesteros-Pérez, P. (2021). A critical review of the current research mainstreams and the influencing factors of green total factor productivity. Environ. Sci. Pollut. Res. 28 (27), 35392–35405. doi:10.1007/s11356-021-14467-4
Zhao, M., Gao, Y., Liu, Q., and Sun, W. (2022). The impact of foreign direct investment on urban green total factor productivity and the mechanism test. Int. J. Environ. Res. Public Health 19 (19), 12183. doi:10.3390/ijerph191912183
Zhou, Y., Jiang, J., Ye, B., and Hou, B. (2019a). Green spillovers of outward foreign direct investment on home countries: evidence from China's province-level data. J. Clean. Prod. 215, 829–844. doi:10.1016/j.jclepro.2019.01.042
Zhou, Y., Xu, Y., Liu, C., Fang, Z., Fu, X., and He, M. (2019b). The threshold effect of China’s financial development on green total factor productivity. Sustainability 11 (14), 3776. doi:10.3390/su11143776
Zhu, S., and Ye, A. (2018). Does the impact of China’s outward foreign direct investment on reverse green technology process differ across countries? Sustainability 10 (11), 3841. doi:10.3390/su10113841
Keywords: China’s FDI, GTFP, super-efficiency SBM, two-step system GMM, threshold effect
Citation: Pan Z, Hu Y and Xu R (2024) China’s foreign direct investment: driving green growth or seeking pollution havens? Based on the perspective of green total factor productivity. Front. Environ. Sci. 12:1389876. doi: 10.3389/fenvs.2024.1389876
Received: 22 February 2024; Accepted: 03 July 2024;
Published: 24 July 2024.
Edited by:
Zhangqi Zhong, Guangdong University of Foreign Studies, ChinaCopyright © 2024 Pan, Hu and Xu. This is an open-access article distributed under the terms of the Creative Commons Attribution License (CC BY). The use, distribution or reproduction in other forums is permitted, provided the original author(s) and the copyright owner(s) are credited and that the original publication in this journal is cited, in accordance with accepted academic practice. No use, distribution or reproduction is permitted which does not comply with these terms.
*Correspondence: Rongrong Xu, eHVyb25ncm9uZ0B0bHUuZWR1LmNu
Disclaimer: All claims expressed in this article are solely those of the authors and do not necessarily represent those of their affiliated organizations, or those of the publisher, the editors and the reviewers. Any product that may be evaluated in this article or claim that may be made by its manufacturer is not guaranteed or endorsed by the publisher.
Research integrity at Frontiers
Learn more about the work of our research integrity team to safeguard the quality of each article we publish.