- 1Water Conservancy and Civil Engineering College, Inner Mongolia Agricultural University, Hohhot, China
- 2Inner Mongolia Key Laboratory of Protection and Utilization of Water Resources, Hohhot, China
- 3Collaborative lnnovation Center for lntegrated Management of Water Resources and Water Environment in the Inner Mongolia Reaches of the Yellow River, Hohhot, China
The Inner Mongolia Reach of the Yellow River Basin is characterized by a relative scarcity of meteorological stations. While satellite precipitation products can complement observations from meteorological stations, their limited spatial resolution restricts their efficacy in regional studies. This study utilizes the GPM IMERG precipitation dataset, considering various factors that influence the spatial distribution of precipitation, such as the Normalized Difference Vegetation Index (NDVI), elevation, slope, aspect, and topographical relief, to construct a multiscale geographically weighted regression (MGWR) model. A spatial downscaling method for the GPM IMERG precipitation dataset is proposed, and its reliability is validated through an accuracy assessment. Moreover, the scale differences in the impact of different factors on the spatial pattern of precipitation in the Inner Mongolia Reach of the Yellow River Basin are scrutinized. The results indicate that: 1) The downscaled GPM IMERG precipitation data (1 km × 1 km) exhibit enhanced accuracy compared to the pre-downscaled data (approximately 11 km × 11 km). The correlation coefficient, Bias, and RMSE of the annual precipitation data after downscaling of GPM IMERG are 0.865, 6.05%, and 68.50 mm/year, respectively. For the monthly downscaled precipitation data, the correlation coefficient, Bias, and RMSE are 0.895, 6.09%, and 16.25 mm/month, respectively. The downscaled GPM IMERG precipitation dataset exhibit high accuracy on both annual and monthly temporal scales. 2) Different factors demonstrate localized effects on precipitation in both dry and wet years. Elevation is the dominant factor influencing the spatial heterogeneity of annual precipitation. The findings from this study can provide technical support for hydrological modeling, drought monitoring, and water resource management in data-scarce areas of the Inner Mongolia Reach of the Yellow River Basin.
1 Introduction
Precipitation is a crucial component of the global water cycle and a key driver of surface hydrological processes (Zhang et al., 2014). Obtaining high temporal and spatial resolution raster precipitation data is of great importance in fields such as ecology, hydrology, and meteorology (Ma et al., 2021; Xue et al., 2022; Li et al., 2023). Conventional approaches to precipitation data collection rely on spatial interpolation of data from rain gauge measurements. However, the accuracy of interpolation is limited by the coverage and representativeness of the rain gauge stations, making it difficult to obtain precise regional precipitation spatial distribution information, especially in arid and semi-arid areas with complex terrain and sparsely distributed stations (Fang et al., 2013; Wang et al., 2022).
Satellite remote sensing-based precipitation estimation offers comprehensive coverage, continuous time series, and convenient data acquisition, making it an effective approach for regional or global-scale precipitation measurements (Kidd and Levizzani, 2011; Tang et al., 2020). Using satellite remote sensing technology, applications like the Tropical Rainfall Measuring Mission Multi-satellite Precipitation Analysis (TMPA) (Huffman et al., 2007), Integrated Multi-satellite Retrievals for Global Precipitation Measurement (GPM IMERG) (Nan et al., 2021), Global Satellite Mapping of Precipitation (GSMaP) (Kubota et al., 2007), Multi-Source Weighted-Ensemble Precipitation (MSWEP) (Beck et al., 2017), Climate Prediction Center Morphing technique (CMORPH) (Joyce et al., 2004), and Precipitation Estimation from Remotely Sensed Information using Artificial Neural Networks-Climate Data Record (PERSIANN-CDR) (Ashouri et al., 2015) have been developed. However, the spatial resolution of the precipitation dataset is coarse (approximately 11km–28 km) (Joyce et al., 2004; Huffman et al., 2007; Kubota et al., 2007; Ashouri et al., 2015; Beck et al., 2017; Nan et al., 2021), limiting its ability to accurately depict precipitation distribution patterns at the scale of small watersheds (Yu et al., 2020). Spatial downscaling methods can be used to effectively overcome this limitation (Kofidou et al., 2023).
Downscaling methods include dynamic and statistical downscaling. Dynamic downscaling involves scale reduction by simulating atmospheric physical processes using models (Sylla et al., 2009; Hu et al., 2018). Although this method possesses a clear physical basis, its applicability is limited due to high computational costs and extensive data requirements. Statistical downscaling relies on empirical statistical relationships between the target variable and explanatory variables to achieve downscaling (Kofidou et al., 2023). It is characterized by relatively lower computational demands, flexibility in application, and ease of operation. It is, therefore, widely used in downscaling studies of satellite remote sensing precipitation products. Immerzeel et al. through the analysis of the correlation between TRMM precipitation and annual scale NDVI, established a regional precipitation downscaling model based on an exponential regression (ER) model, obtaining TRMM precipitation dataset for the Iberian Peninsula in Spain with a spatial resolution of 1 km (Immerzeel et al., 2009). Building upon the research by Immerzeel et al., Jia et al. considered that the spatial distribution of precipitation is influenced by more than a single variable. They included topographic factors within the scope of their variables and established a Multiple Linear Regression (MLR) model between TRMM, NDVI, and topographic factors, enhancing the TRMM precipitation dataset for the Qaidam Basin in China from a 0.25° resolution to 1 km (Jia et al., 2011). Jing et al. demonstrated that using the random forest (RF) model for precipitation downscaling achieves higher simulation accuracy than both the exponential regression and the linear regression models (Jing et al., 2016). However, these models assume that the relationships between precipitation and environmental variables are homogeneous in space and do not account for the spatial non-stationarity between precipitation and variable factors, because their relationship should vary with changes in spatial location (Brunsdon et al., 1998). The Geographically Weighted Regression (GWR) model can account for the spatial non-stationarity between precipitation and explanatory variables in downscaling studies of precipitation (Xu et al., 2015). However, the GWR model assumes that all variable factors have the same optimal bandwidth, neglecting scale differences in the effects of different influencing factors on precipitation (Arshad et al., 2021). Thus, Fotheringham et al. proposed the Multi-Scale Geographically Weighted Regression (MGWR) model, based on the GWR model, which considers different spatial bandwidths for various influencing factors (Fotheringham et al., 2017). This model better reveals scale differences in the mechanisms of various factors affecting precipitation. Noor et al. applied the MGWR model and the RF model to downscale the TRMM precipitation dataset (Noor et al., 2023), while Arshad et al. used the MGWR model and the GWR model for downscaling the TRMM precipitation dataset over the Indus River Basin (Abdollahipour et al., 2021). The results showed that the simulation accuracy of the MGWR model was superior to the other two models. Therefore, the MGWR model can be widely used in precipitation downscaling studies.
Currently, downscaling studies based on the MGWR model are relatively scarce and primarily focused on the TRMM precipitation dataset. The TRMM mission ceased operation on 8 April 2015, and its successor, the Global Precipitation Measurement (GPM) mission, has ushered in a new era of satellite precipitation measurement (Tang et al., 2016). The GPM Core Observatory (GPMCO) is equipped with a dual-frequency radar (Ku and Ka bands), capable of detecting lower minimum echo intensities and employing a high-sensitivity mode for staggered sampling (Hou et al., 2014). Additionally, the microwave radiometer at the GPMCO has four high-frequency channels, providing more accurate data for light precipitation intensity and solid precipitation (Hou et al., 2014).
Currently, there are relatively many studies on downscaling of GPM IMERG precipitation datasets in humid areas (Ma et al., 2018; Min et al., 2020; Yan et al., 2021a), but relatively few in arid and semi-arid areas. The Inner Mongolia Reach of the Yellow River Basin is located in an arid and semi-arid area, where the terrain is diverse and complex, and precipitation exhibits distinct regional and seasonal variations (Wang et al., 2023). In this study, we focused on the Inner Mongolia Reach of the Yellow River Basin. We selected the GPM IMERG precipitation dataset and used NDVI, elevation, slope, aspect, and topographical relief as explanatory variables to construct a Multi-Scale Geographically Weighted Regression (MGWR) model. This model was used to generate precipitation data at a resolution of 1 km at both monthly and annual scales for the years 2001–2019. The resulting dataset offers essential support for meteorological and hydrological research within the basin.
2 Materials and methods
2.1 Study area
The Inner Mongolia Reach of the Yellow River Basin is situated in the upper reaches of the Yellow River (37°37′–41°50′N, 106°28′–112°50′E), covering a total area of approximately 149,029 km2. This accounts for about 18.63% of the total area of the Yellow River Basin, with elevations ranging from 843 to 2,315 m (Figure 1). The study area is located in an arid and semi-arid region, characterized by drought and scarce rainfall, strong evaporation, large diurnal temperature variations, and is typical of a mid-temperate continental climate (Wang et al., 2023). It has an annual average precipitation of 305 mm and an average annual temperature of 6.5°C (Zhang et al., 2023). Annual average Precipitation gradually decreases from east to west (Table 1). In the basin, grasslands account for 74.20%, cultivated land for 18.95%, and forests for 6.85% (Zhang et al., 2023).
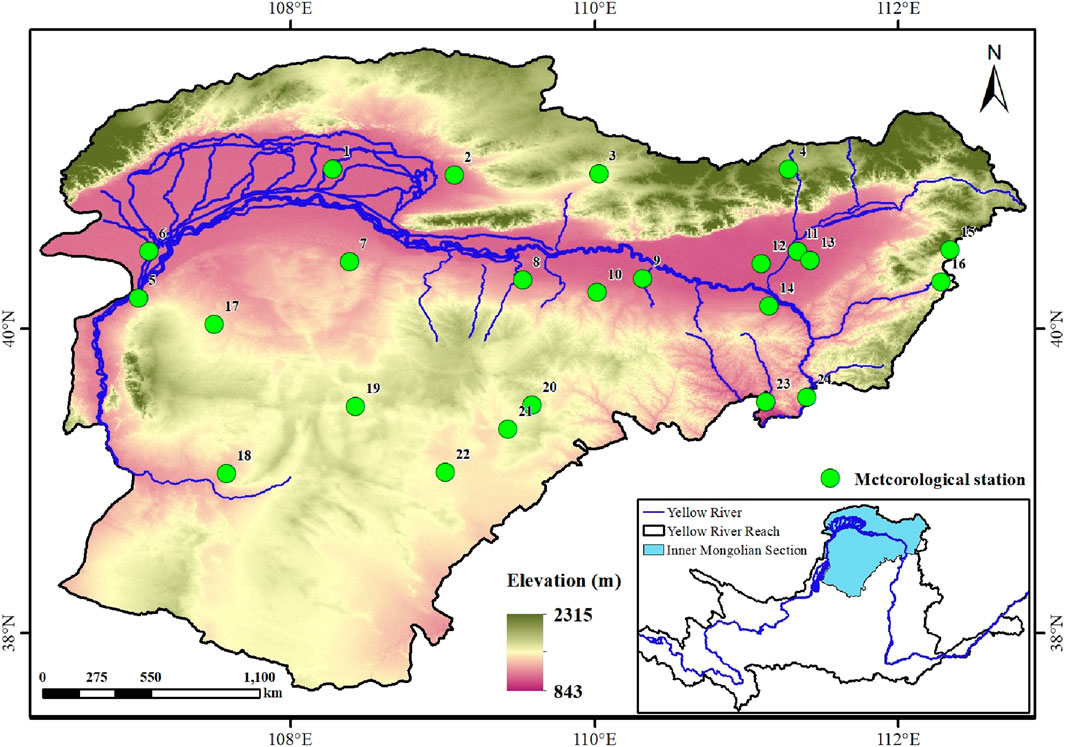
Figure 1. Location of the Inner Mongolia Reach of the Yellow River Basin and the meteorological stations in the study area.
2.2 Data preparation
2.2.1 GPM IMERG
IMERG is a new generation of multi-satellite combined precipitation data introduced through the GPM program. It offer three types of products (Early, Late, and Final) based on different data inversion algorithms. The IMERG Final product is considered more accurate owing to its incorporation of rain gauge data from the Global Precipitation Climatology Centre (GPCC) (Wang et al., 2017). The IMERG V06 integrates information from available GPM and TMPA sensors. This integration involves mutual calibration, merging, interpolation, and fusion to generate consistent precipitation estimates from June 2000 to the present (Yu et al., 2022). For this study, we selected IMERG V06 (IMERG_Final) daily data for the period 2001–2019, which we obtained from the official NASA website (https://www.nasa.gov/). This dataset has a spatial resolution of 0.1 ° × 0.1 ° (approximately 11 km × 11 km), and annual and monthly precipitation data were derived from the daily dataset.
2.2.2 Environment variables
Shuttle Radar Topography Mission (SRTM) data, accessible at http://www.gscloud.cn, were utilized to derive a Digital Elevation Model (DEM) with a spatial resolution of 90 m × 90 m. Within ArcGIS 10.7, topographic factors such as elevation, slope, aspect, and topographical relief were extracted from the DEM data. NDVI data, sourced from the Moderate Resolution Imaging Spectroradiometer (MODIS) on the Terra satellite, were obtained from NASA at https://ladsweb.modaps.eosdis.nasa.gov/. MOD13A3 monthly composite NDVI data, with a spatial resolution of 1 km × 1 km, were used. The MOD13A3 data underwent preprocessing using the MODIS Reprojection Tool (MRT) software, and annual NDVI data were derived was generated using a maximum value composite method. To maintain consistency with the GPM IMERG precipitation dataset and MGWR downscaling, NDVI, elevation, slope, aspect, and topographical relief data were resampled to two spatial scales, 0.1° and 1 km, in ArcGIS 10.7. This resampling was performed using the cubic convolution method because it offers good smoothing performance, detail preservation, and edge sharpening. Cubic convolution yields more satisfactory results compared to the Nearest Neighbor and Bilinear Interpolation methods (Molinaro et al., 2005).
2.2.3 Rain gauge data
Meteorological station precipitation data from 2001 to 2019 were acquired from the China Meteorological Data Network (http://data.cma.cn) to validate the accuracy of both the original GPM IMERG precipitation data and the downscaled precipitation data. A total of 24 meteorological stations were chosen, with observed precipitation data having an accuracy of 0.1 mm. Daily data from these stations were aggregated into monthly and annual precipitation values. Basic information about these stations is available in Table 1.
2.3 Methods
2.3.1 Multiscale geographically weighted regression (MGWR)
The Multiscale Geographically Weighted Regression (MGWR) model is a regional regression model, that is widely used to study dynamic relationships between target and explanatory variables (Fotheringham et al., 2017). It allows each explanatory variable to vary at different spatial scales, facilitating the capture of spatial non-stationarity relationships among them. The MGWR model is expressed as follows:
where Yi represents the target variable;
where
Based on previous studies, the adaptive bi-square was chosen to solve the weight matrix, with the AICc (corrected Akaike information criterion) as the bandwidth selection criterion, and the golden section search method was used to determine the bandwidth (Chao et al., 2018; Arshad et al., 2021). All processes were conducted using MGWR 2.2 software. The formula for the adaptive bi-square is as follows:
where
2.3.2 Precipitation downscaling process based on MGWR
Previous research indicates that NDVI, elevation, slope, aspect, and topographical relief are important factors influencing precipitation (Wang et al., 2022; Bai et al., 2023). Considering the spatial non-stationarity between precipitation and factors such as vegetation and topography, and the considerable scale differences in the spatial impact of NDVI, elevation, slope, aspect, and ruggedness on precipitation, this study used the MGWR model to downscale the GPM IMERG precipitation dataset at both monthly and annual scales. The specific steps were as follows (Figure 2):
(1) Data preparation: Environmental variables with spatial resolutions of 0.1° and 1 km, as well as the original GPM IMERG precipitation data at a resolution of 0.1°, were prepared. The GPM IMERG data spanned the period from January 2001 to December 2019, and was summarized at both monthly and annual scales. Environmental variables included NDVI, elevation, slope, aspect, and topographical relief, with NDVI aligned with the temporal scale of the GPM IMERG precipitation data.
(2) MGWR model establishment: Duan et al. have found that the lag time of vegetation response to precipitation in the study area is approximately 10 days (Duan et al., 2019). Therefore, at a monthly scale, the current month’s NDVI data was selected as the explanatory variable for the monthly precipitation scale model. At monthly and annual time scales, the GPM IMERG data with a resolution of 0.1° were used as the target variable, and NDVI, elevation, slope, aspect, and topographical relief of the same resolution and time scale were used as explanatory variables. The MGWR model was constructed at monthly and annual scales to obtain the regression coefficients
(3) Parameter interpolation: Using the Kriging method, intercepts, slopes, and regression residuals from step (2) were interpolated. This yielded high-resolution (1 km) raster data of regression coefficients, intercept terms, and residuals at monthly and annual scales.
(4) Downscaling completion: Based on Eq. 1, monthly and annual precipitation values at a 1 km resolution were obtained after downscaling using the MGWR model.
2.3.3 Simulation accuracy assessment
Using the MGWR downscaling approach outlined in Section 2.3.2, downscaled GPM IMERG data for the years 2001–2019 were generated. The accuracy of the downscaled results (1 km) and the original GPM IMERG precipitation data (0.1°) at annual and monthly scales was validated using observed data from 24 meteorological stations in the study area. Three indicators—correlation coefficient (R), Bias, and root mean square error (RMSE)—were employed for the validation (Wang et al., 2022), with the following formulas:
Where Mi (mm) and
3 Results
3.1 Accuracy of downscaled GPM IMERG precipitation
Observed precipitation from 24 meteorological stations in the study area for the period 2001–2019 were used to validate the GPM IMERG precipitation data that were downscaled using the MGWR method. Figures 3, 4 represent the validation results of annual and monthly downscaled and original precipitation, respectively.
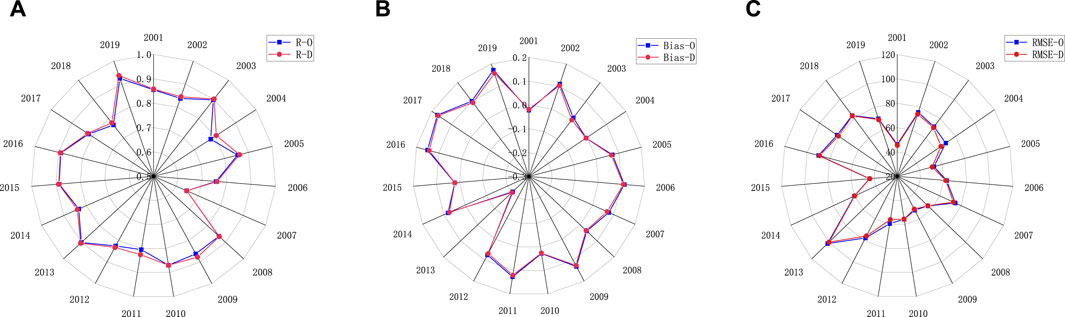
Figure 3. Correlation coefficient (R) (A), Bias (B), and root mean squared error (RMSE) (C) of the observed and downscaled annual precipitation from 2001 to 2019. D represents the downscaled precipitation and O denotes the original GPM IMERG precipitation, similarly hereinafter.
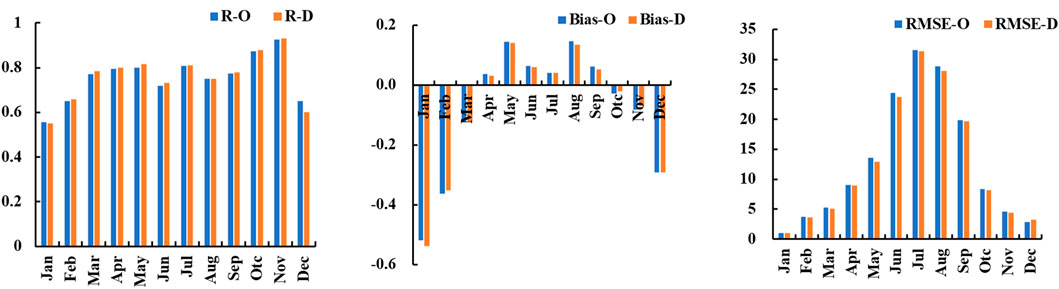
Figure 4. Correlation coefficient (R), Bias, and root mean squared error (RMSE) values between measured precipitation and monthly precipitation from 2001 to 2019 before and after Downscaling.
The accuracy of annual precipitation from 2001 to 2019 is shown in Figure 3 The accuracies of the downscaled precipitation are better than that of the original precipitation. The annual trends of three accuracy indicators for the original GPM IMERG and the downscaled precipitation data were consistent (Figure 3). Overall, on an annual scale, the downscaled data maintained a certain level of accuracy while providing an improved reflection of the distribution of precipitation in the study area. The correlation coefficient of the downscaled annual precipitation varied from 0.648 to 0.937, with an average of 0.843, indicating good correlation between the annual downscaled precipitation and the measured data from the meteorological stations. The Bias varied from −0.219 to 0.177, with an average of 0.059. Except for the year 2013, the Bias was less than 0.2, and Bias values were mostly positive, suggesting that the simulated annual precipitation was generally overestimated compared to the measured data from the meteorological stations. The RMSE ranged from 45.53 mm to 99.88 mm, with an average of 66.33 mm.
As shown in Figure 4, at a monthly scale, the trends of the three accuracy indicators for both the original GPM IMERG and the downscaled precipitation remained consistent. Overall, the accuracy of the downscaled precipitation was greater than that of the original precipitation from February to November. However, from December to the following January, the accuracy of the downscaled precipitation was lower than that of the original GPM IMERG. The correlation coefficient for the downscaled precipitation ranged from 0.552 to 0.932, with an average of 0.758. Precipitation estimation accuracy in spring and autumn was higher than that in summer and winter. This was attributed to higher amount of precipitation in summer and the predominance of snowfall in winter. The Bias was positive from April to October, peaking in August (0.134), and negative from November to March, reaching its lowest value in January (−0.538). This suggested that increased vegetation growth and precipitation contribute to an overestimation of monthly downscaled precipitation results. The RMSE exhibited a unimodal variation pattern correlated with the amount of monthly precipitation, ranging from 1.075 mm to 31.333 mm, with an average of 12.528 mm.
3.2 Downscaling results of GPM IMERG precipitation datasets
Figures 5, 6 present a comparison of the spatial distribution of annual and monthly average precipitation in the study area from 2001 to 2019, before and after downscaling. Downscaling using the MGWR model considerably improved the spatial resolution of the annual and monthly average GPM IMERG images compared to the original GPM IMERG images. While the spatial distribution remained consistent before and after downscaling, the representation of precipitation distribution became more refined post-downscaling. The multi-year average precipitation demonstrated a decreasing trend from the southeast to the northwest of the study area. Multi-year average precipitation ranged from 145.4 to 475.4 mm before downscaling and from 138.8 to 481.3 mm after downscaling. Compared to the original GPM IMERG data, the range of the downscaling simulation results increased. While enhancing the spatial resolution, the precipitation information became more comprehensive. The maximum monthly average precipitation occurred in July, and the minimum in January. The spatial distribution trends of monthly average precipitation and annual average precipitation are consistent.
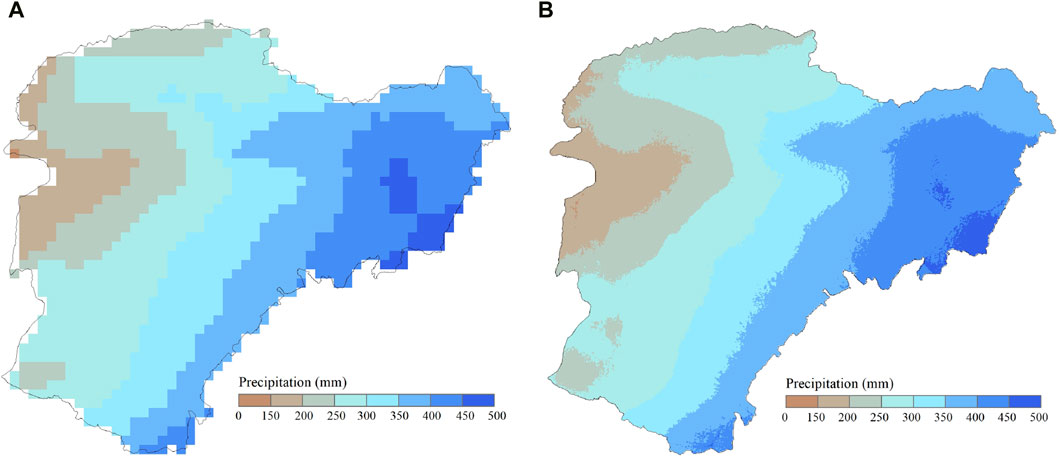
Figure 5. Spatial distributions of the (A) original and (B) downscaled mean annual precipitation from 2001 to 2019.
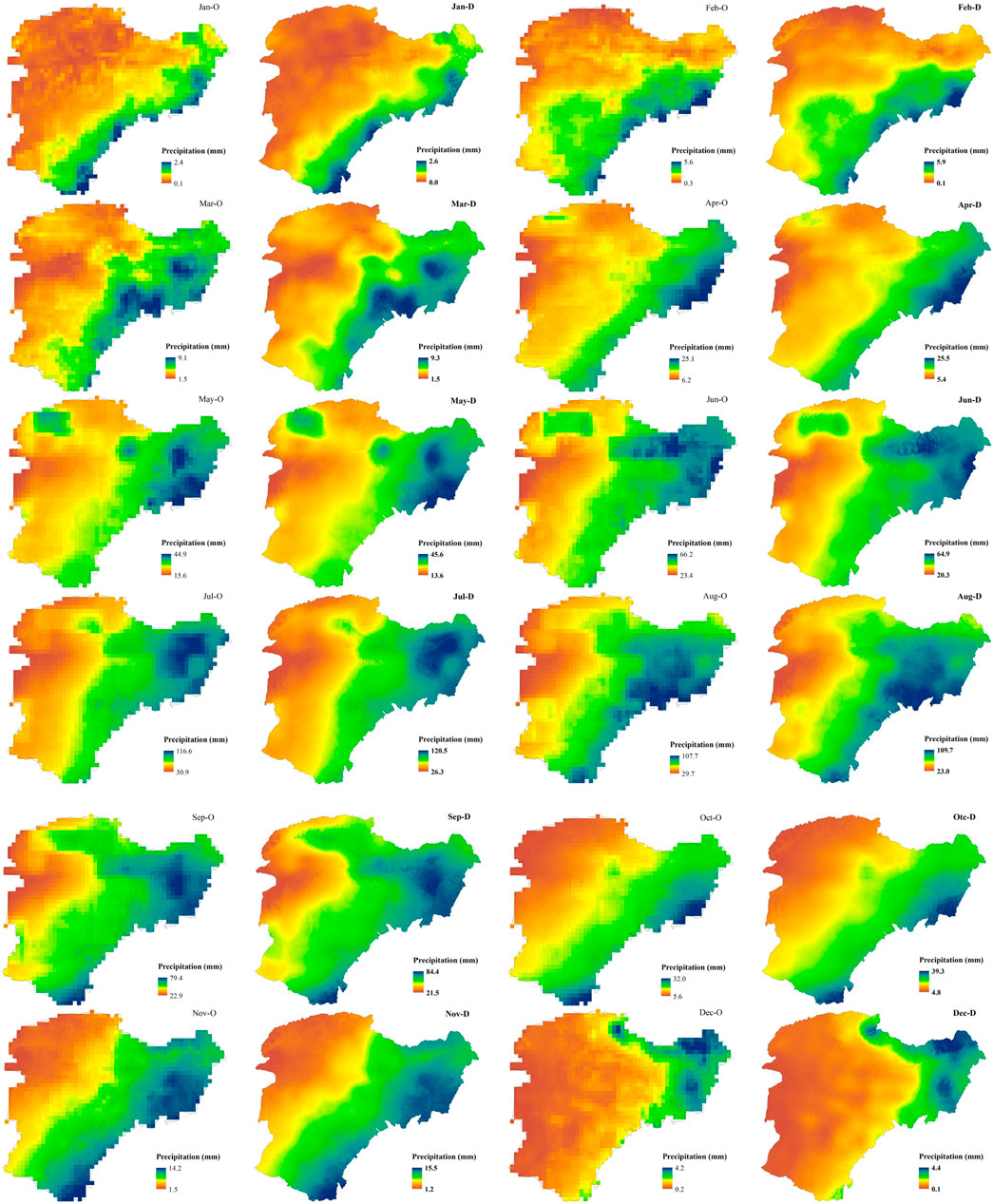
Figure 6. Spatial distributions of the (O) original and (D) downscaled mean monthly precipitation from 2001 to 2019.
3.3 Analysis of variable effect scale based on the MGWR model
To investigate scale differences in the impact of terrain and vegetation factors on the spatial distribution of precipitation at an annual scale, this study selected the years 2005 and 2016 as typical dry and wet years, respectively. The bandwidths in the MGWR model were used to understand the range of influence of terrain and vegetation factors in each typical year. Smaller bandwidths indicated that the variable had a more localized influence on precipitation, designating it as a local influencing factor, whereas larger bandwidths suggested that the variable had a regional influence, designating it as a regional influencing factor (Fotheringham et al., 2017). Table 2 presents the bandwidth sizes of each variable obtained from the MGWR model, revealing relatively small differences in the scale of impact of variables in the dry year and comparatively larger differences in the wet year. However, variables in each typical year demonstrated localized impacts. Overall, precipitation in the Inner Mongolia Reach of the Yellow River Basin exhibited considerable spatial variation across different terrain and vegetation cover intervals.
Regression coefficients indicate the extent of the impact of vegetation and terrain factors on the spatial distribution of precipitation. The trend of the regression coefficients (RC) of variables in each typical year was generally consistent (Figure 7). In dry and wet years, The areas in which NDVI had a positive effect on the spatial variation in annual precipitation accounted for 59.73% and 61.88% of the total area in the dry and wet years, respectively. The positive effect of NDVI on annual precipitation was greater in wet years than in dry years. This was because the presence of ample soil moisture in wet years allowed plants to absorb more water from the soil and release it into the atmosphere through their leaves, increasing the atmospheric moisture content and promoting precipitation (Vicente-Serrano et al., 2013). In dry years, areas where topographical relief, aspect, and slope had a positive effect on the spatial variation in annual precipitation accounted for 68.05%, 50.40%, and 53.96% of the total area, respectively. In wet years, these areas where topographical relief, aspect, and slope positively influenced the spatial variation in annual precipitation accounted for 68.93%, 55.77%, and 69.53% of the total area, respectively. Elevation primarily exerted a negative effect on the spatial variation of annual precipitation, affecting 86.38% and 84.43% of the total area in dry and wet years, respectively. As elevation, topographical relief, and slope increased, their impact on annual precipitation gradually diminished due to the weakening distribution of spatial precipitation influenced by terrain on the transport and vertical movement of atmospheric moisture (Sokol and Bliznák, 2009).
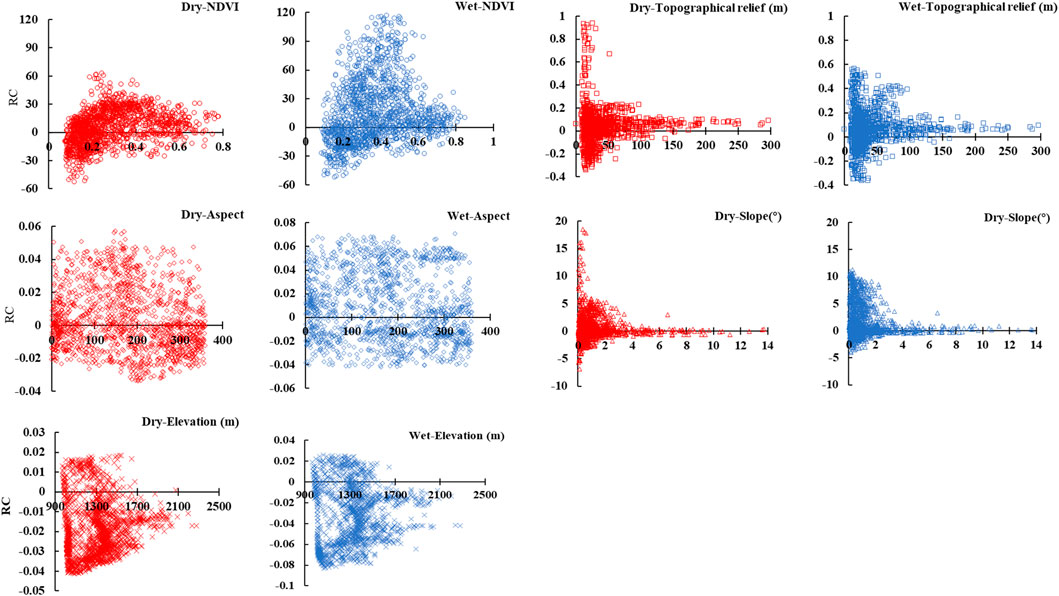
Figure 7. Variation patterns of regression coefficients of each variable with respect to the variables in typical years.
Utilizing the absolute values of standardized regression coefficients to identify the primary factors influencing spatial precipitation differences, Figure 8 illustrates that, irrespective of dry or wet years, elevation emerges as the predominant factor in shaping precipitation variations in the Inner Mongolia Reach of the Yellow River Basin, encompassing approximately 50% of the basin area.
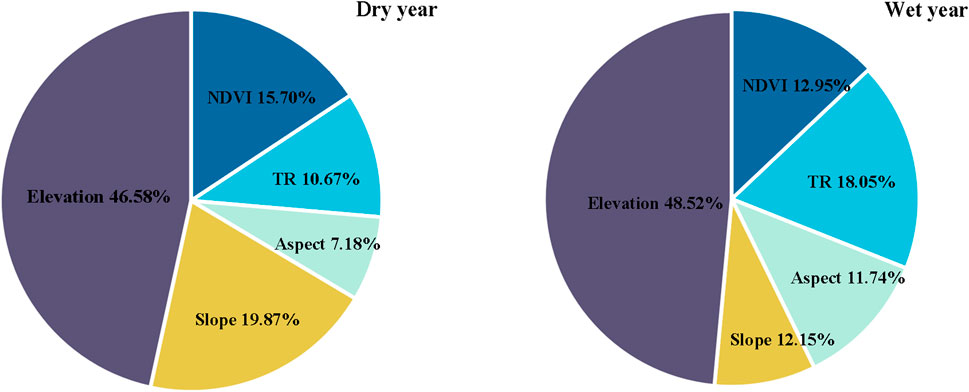
Figure 8. Proportion of Variable Impacts on Precipitation in the MGWR Model. TR represents topographical relief.
4 Discussion
The downscaled simulation data, generated through the MGWR model, were consistent with the GPM IMERG data in terms of spatial distribution of precipitation and exhibited improved spatial resolution and more detailed precipitation information. This is in agreement with the findings of Arshad et al., who employed the MGWR model for downscaling TRMM data in the Indus Basin (Arshad et al., 2021). However, at the monthly scale, the accuracy of some of the downscaled precipitation data was lower than that of the GPM IMERG data. Arshad et al. used the Geographically Weighted Regression Disaggregation Approach (GDA) to implement corrections based on meteorological station data for downscaled data with lower accuracy than the original data, and the accuracy of the resulting downscaled data was superior to that of the original data (Arshad et al., 2021). In the Inner Mongolia Reach of the Yellow River Basin, the scarcity of meteorological station data and difficulty in obtaining this data have precluded the possibility of interpolation corrections based on meteorological station data for downscaled simulation data. This highlights the fact that the accuracy of downscaled data obtained only through linear downscaling may not consistently be superior to that of the original data. Therefore, the development of new downscaling algorithms is imperative to obtain more accurate and reliable precipitation datasets at high spatial resolution.
The selection of appropriate explanatory variables plays a crucial role in the precipitation downscaling process and the performance of the MGWR model. In this study, five explanatory variables were chosen for precipitation downscaling: NDVI, elevation, slope, aspect, and topographical relief. These variables were selected based on their regional importance and overall influence on the spatial variation in precipitation (Lu et al., 2020). The five chosen explanatory variables are commonly employed in precipitation downscaling studies across various global basins (Chen et al., 2014; Zhang et al., 2017; Zhang et al., 2018). It is noteworthy that additional environmental variables, such as surface characteristics (soil moisture and evapotranspiration) (Chen et al., 2019; Yan et al., 2021b) and meteorological factors (temperature, humidity, radiation, atmospheric circulation, and cloud cover) (Arshad et al., 2021) can impact the spatial distribution of precipitation. Future studies should consider incorporating these environmental variables to further assess the downscaling performance of precipitation.
5 Conclusion
Analysis of the spatial distribution of data before and after downscaling indicated that the detailed features were better represented post-downscaling. Following downscaling, the GPM IMERG precipitation dataset exhibited a relative increase in correlation coefficient, Bias, and RMSE when compared to the values calculated from the measured precipitation data. Overall, the accuracy of the data after downscaling was somewhat enhanced and the data reflected the actual precipitation information and distribution patterns across various time scales in the study area with greater accuracy.
The MGWR model adopted different bandwidths for different variables, thereby demonstrating the varying scale of influence of different factors. The findings of this study indicate that the patterns of spatial variation in both dry and wet years in the Inner Mongolia Reach of the Yellow River Basin are determined by multiple spatial scale processes of several variable factors. The impact of NDVI, elevation, aspect, slope, and topographical relief displayed a localized effect on precipitation in both wet and dry years. The MGWR regression results highlighted elevation as the primary factor influencing the spatial differentiation of precipitation in both wet and dry years.
In summary, for the GPM IMERG precipitation dataset, the application of the MGWR model enhances the spatial resolution of precipitation data, revealing more detailed features. It also ensures the consistency of data accuracy and spatial distribution. This can provide a relatively reliable high-resolution precipitation dataset for drought monitoring, hydrological modeling, and water resource management in the Inner Mongolia Reach of the Yellow River Basin.
Data availability statement
The data presented in this study are available on request from the corresponding author.
Author contributions
LT: Conceptualization, Data curation, Formal Analysis, Investigation, Methodology, Software, Visualization, Writing–original draft. LD: Conceptualization, Data curation, Funding acquisition, Project administration, Resources, Supervision, Writing–review and editing.
Funding
The author(s) declare that financial support was received for the research, authorship, and/or publication of this article. This work was supported by the National Natural Science Foundation of China (U23A2001 and 51939006), the National Natural Science Foundation of China (the Yellow River Water Science Research Joint Fund U2243234), the National Key Research and Development Plan Project of China (2022YFC3204401 and 2021YFC3201200), the Major Science and Technology Projects of Inner Mongolia Autonomous Region (2020ZD0009 and 2022EEDSKJXM005), the Inner Mongolia Autonomous Region Science and Technology Leading Talent Team (2022LJRC0007), the Natural Science Foundation of Inner Mongolia Autonomous Region of China (2020JQ06) and the Inner Mongolia Agricultural University Basic Research Business Expenses Project (BR221012 and BR221204).
Conflict of interest
The authors declare that the research was conducted in the absence of any commercial or financial relationships that could be construed as a potential conflict of interest.
Publisher’s note
All claims expressed in this article are solely those of the authors and do not necessarily represent those of their affiliated organizations, or those of the publisher, the editors and the reviewers. Any product that may be evaluated in this article, or claim that may be made by its manufacturer, is not guaranteed or endorsed by the publisher.
References
Abdollahipour, A., Ahmadi, H., and Aminnejad, B. (2021). Evaluating the reconstruction method of satellite-based monthly precipitation over Golestan province, Northern Iran. Acta geophys. 69 (6), 2305–2323. doi:10.1007/s11600-021-00623-4
Arshad, A., Zhang, W. C., Zhang, Z. J., Wang, S. H., Zhang, B., Cheema, M. J. M., et al. (2021). Reconstructing high-resolution gridded precipitation data using an improved downscaling approach over the high altitude mountain regions of Upper Indus Basin (UIB). Sci. Total Environ. 784, 147140. doi:10.1016/j.scitotenv.2021.147140
Ashouri, H., Hsu, K. L., Sorooshian, S., Braithwaite, D. K., Knapp, K. R., Cecil, L. D., et al. (2015). PERSIANN-CDR daily precipitation climate data record from multisatellite observations for hydrological and climate studies. Bull. Am. Meteorol. Soc. 96 (1), 69–83. doi:10.1175/bams-d-13-00068.1
Bai, X. Y., Fan, Z. M., and Yue, T. X. (2023). Dynamic pattern-effect relationships between precipitation and vegetation in the semi-arid and semi-humid area of China. CATENA 232, 107425. doi:10.1016/j.catena.2023.107425
Beck, H. E., van Dijk, A. I. J. M., Levizzani, V., Schellekens, J., Miralles, D. G., Martens, B., et al. (2017). MSWEP: 3-hourly 0.25° global gridded precipitation (1979–2015) by merging gauge, satellite, and reanalysis data. Hydrol. Earth Syst. Sci. 21, 589–615. doi:10.5194/hess-21-589-2017
Brunsdon, C., Fotheringham, S., and Charlton, M. (1998). Geographically weighted regression. J. R. Stat. Soc. Ser. D. Stat. 47, 431–443. doi:10.1111/1467-9884.00145
Chao, L. J., Zhang, K., Li, Z. J., Zhu, Y. L., Wang, J. F., and Yu, Z. B. (2018). Geographically weighted regression based methods for merging satellite and gauge precipitation. J. Hydrol. 558, 275–289. doi:10.1016/j.jhydrol.2018.01.042
Chen, F. R., Liu, Y., Liu, Q., and Li, X. (2014). Spatial downscaling of TRMM 3B43 precipitation considering spatial heterogeneity. Int. J. Remote Sens. 35, 3074–3093. doi:10.1080/01431161.2014.902550
Chen, S. D., Zhang, L. P., She, D. X., and Chen, J. (2019). Spatial downscaling of tropical rainfall measuring mission (TRMM) annual and monthly precipitation data over the middle and lower reaches of the yangtze River Basin, China. China. Water 11, 568. doi:10.3390/w11030568
Duan, L. M., Fan, K. K., Li, W., and Liu, T. X. (2019). Spatial downscaling algorithm of TRMM precipitation based on multiple high-resolution satellite data for Inner Mongolia, China. Theor. Appl. Climatol. 135, 45–59. doi:10.1007/s00704-017-2347-7
Fang, J., Du, J., Xu, W., Shi, P. J., Li, M., and Ming, X. D. (2013). Spatial downscaling of TRMM precipitation data based on the orographical effect and meteorological conditions in a mountainous area. Adv. Water Resour. 61, 42–50. doi:10.1016/j.advwatres.2013.08.011
Fotheringham, A. S., Yang, W. B., and Kang, W. (2017). Multiscale geographically weighted regression (MGWR). Ann. Am. Assoc. Geogr. 107, 1247–1265. doi:10.1080/24694452.2017.1352480
Hou, A. Y., Kakar, R. K., Neeck, S., Azarbarzin, A. A., Kummerow, C. D., Kojima, M., et al. (2014). The global precipitation measurement mission. Bull. Am. Meteorol. Soc. 95, 701–722. doi:10.1175/bams-d-13-00164.1
Hu, X. M., Xue, M., McPherson, R. A., Martin, E., Rosendahl, D. H., and Qiao, L. (2018). Precipitation dynamical downscaling over the great plains. J. Adv. Model. Earth Syst. 10, 421–447. doi:10.1002/2017ms001154
Huffman, G. J., Bolvin, D. T., Nelkin, E. J., Wolff, D. B., Adler, R. F., Gu, G. J., et al. (2007). The TRMM multisatellite precipitation analysis (TMPA): quasi-global, multiyear, combined-sensor precipitation estimates at fine scales. J. Hydrometeorol. 8, 38–55. doi:10.1175/jhm560.1
Immerzeel, W. W., Rutten, M. M., and Droogers, P. (2009). Spatial downscaling of TRMM precipitation using vegetative response on the Iberian Peninsula. Remote Sens. Environ. 113 (2), 362–370. doi:10.1016/j.rse.2008.10.004
Jia, S. F., Zhu, W. B., Lu, A. F., and Yan, T. T. (2011). A statistical spatial downscaling algorithm of TRMM precipitation based on NDVI and DEM in the Qaidam Basin of China. Remote Sens. Environ. 115 (12), 3069–3079. doi:10.1016/j.rse.2011.06.009
Jing, W. L., Yang, Y. P., Yue, X. F., and Zhao, X. D. (2016). A comparison of different regression algorithms for downscaling monthly satellite-based precipitation over North China. Remote Sens. 8 (10), 835. doi:10.3390/rs8100835
Joyce, R. J., Janowiak, J. E., Arkin, P. A., and Xie, P. P. (2004). CMORPH: a method that produces global precipitation estimates from passive microwave and infrared data at high spatial and temporal resolution. J. Hydrometeorol. 5, 487–503. doi:10.1175/1525-7541(2004)005<0487:camtpg>2.0.co;2
Kidd, C., and Levizzani, V. (2011). Status of satellite precipitation retrievals. Hydrol. Earth Syst. Sci. 15, 1109–1116. doi:10.5194/hess-15-1109-2011
Kofidou, M., Stathopoulos, S., and Gemitzi, A. (2023). Review on spatial downscaling of satellite derived precipitation estimates. Environ. Earth Sci. 82, 424. doi:10.1007/s12665-023-11115-7
Kubota, T., Shige, S., Hashizurne, H., Aonashi, K., Takahashi, N., Seto, S., et al. (2007). Global precipitation map using satellite-borne microwave radiometers by the GSMaP project: production and validation. IEEE Trans. Geosci. Remote Sens. 45, 2259–2275. doi:10.1109/tgrs.2007.895337
Li, X. Y., Wang, Y. T., Xue, B. L., A, Y. L., Zhang, X. J., and Wang, G. Q. (2023). Attribution of runoff and hydrological drought changes in an ecologically vulnerable basin in semi-arid regions of China. Hydrol. Process. 37 (10), e15003. doi:10.1002/hyp.15003
Lu, X. Y., Tang, G. Q., Wang, X. Q., Liu, Y., Wei, M., and Zhang, Y. X. (2020). The development of a two-step merging and downscaling method for satellite precipitation products. Remote Sens. 12 (3), 398. doi:10.3390/rs12030398
Ma, N., Szilagyi, J., and Zhang, Y. Q. (2021). Calibration-free complementary relationship estimates terrestrial evapotranspiration globally. Water Resour. Res. 57 (9), e2021WR029691. doi:10.1029/2021wr029691
Ma, Z. Q., Tan, X., Yang, Y., Chen, X., Kan, G. Y., Ji, X., et al. (2018). The first comparisons of IMERG and the downscaled results based on IMERG in hydrological utility over the ganjiang River Basin. Water 10 (10), 1392. doi:10.3390/w10101392
Min, X. X., Ma, Z. Q., Xu, J. T., He, K., Wang, Z. G., Huang, Q. L., et al. (2020). Spatially downscaling IMERG at daily scale using machine learning approaches over zhejiang, southeastern China. Front. Earth Sci. 8, 146. doi:10.3389/feart.2020.00146
Molinaro, A. M., Simon, R., and Pfeiffer, R. M. (2005). Prediction error estimation: a comparison of resampling methods. Bioinformatics 21, 3301–3307. doi:10.1093/bioinformatics/bti499
Nan, L. J., Yang, M. X., Wang, H., Xiang, Z. L., and Hao, S. K. (2021). Comprehensive evaluation of global precipitation measurement mission (GPM) IMERG precipitation products over mainland China. Water 13, 3381. doi:10.3390/w13233381
Noor, R., Arshad, A., Shafeeque, M., Liu, J. P., Baig, A., Ali, S., et al. (2023). Combining APHRODITE rain gauges-based precipitation with downscaled-TRMM data to translate high-resolution precipitation estimates in the indus basin. Remote Sens. 15 (2), 318. doi:10.3390/rs15020318
Sokol, Z., and Bliznák, V. (2009). Areal distribution and precipitation-altitude relationship of heavy short-term precipitation in the Czech Republic in the warm part of the year. Atmos. Res. 94 (4), 652–662. doi:10.1016/j.atmosres.2009.03.001
Sylla, M. B., Gaye, A. T., Pal, J. S., Jenkins, G. S., and Bi, X. Q. (2009). High-resolution simulations of West African climate using regional climate model (RegCM3) with different lateral boundary conditions. Theor. Appl. Climatol. 98 (3-4), 293–314. doi:10.1007/s00704-009-0110-4
Tang, G. Q., Ma, Y. Z., Long, D., Zhong, L. Z., and Hong, Y. (2016). Evaluation of GPM Day-1 IMERG and TMPA Version-7 legacy products over Mainland China at multiple spatiotemporal scales. J. Hydrol. 533, 152–167. doi:10.1016/j.jhydrol.2015.12.008
Tang, Y. F., Wu, J., Bai, L., and Wang, B. (2020). Reliability of gridded precipitation products in the Yellow River Basin, China. Remote Sens. 12, 374. doi:10.3390/rs12030374
Vicente-Serrano, S. M., Gouveia, C., Camarero, J. J., Begueria, S., Trigo, R., Lopez-Moreno, J. I., et al. (2013). Response of vegetation to drought time-scales across global land biomes. Proc. Natl. Acad. Sci. U. S. A. 110 (1), 52–57. doi:10.1073/pnas.1207068110
Wang, H., Zang, F., Zhao, C. Y., and Liu, C. L. (2022). A GWR downscaling method to reconstruct high-resolution precipitation dataset based on GSMaP-Gauge data: a case study in the Qilian Mountains, Northwest China. Sci. Total Environ. 810, 152066. doi:10.1016/j.scitotenv.2021.152066
Wang, Y. X., Duan, L. M., Tong, X., Liu, T. X., Li, D. F., and Li, W. (2023). Non-stationary modeling of wet-season precipitation over the Inner Mongolia section of the Yellow River basin. Theor. Appl. Climatol. 151, 389–405. doi:10.1007/s00704-022-04279-y
Wang, Z. L., Zhong, R. D., Lai, C. G., and Chen, J. C. (2017). Evaluation of the GPM IMERG satellite-based precipitation products and the hydrological utility. Atmos. Res. 196, 151–163. doi:10.1016/j.atmosres.2017.06.020
Xu, S. G., Wu, C. Y., Wang, L., Gonsamo, A., Shen, Y., and Niu, Z. (2015). A new satellite-based monthly precipitation downscaling algorithm with non-stationary relationship between precipitation and land surface characteristics. Remote Sens. Environ. 162, 119–140. doi:10.1016/j.rse.2015.02.024
Xue, B. L., A, Y. L., Wang, G. Q., Helman, D., Sun, G., Tao, S. L., et al. (2022). Divergent hydrological responses to forest expansion in dry and wet basins of China: implications for future afforestation planning. Water Resour. Res. 58 (5), e2021WR031856. doi:10.1029/2021wr031856
Yan, X., Chen, H., Tian, B. R., Sheng, S., Wang, J. X., and Kim, J. S. (2021a). A downscaling-merging scheme for improving daily spatial precipitation estimates based on random forest and cokriging. Remote Sens. 13 (11), 2040. doi:10.3390/rs13112040
Yan, X., Chen, H., Tian, B. R., Sheng, S., Wang, J. X., and Kim, J. S. (2021b). A downscaling-merging scheme for improving daily spatial precipitation estimates based on random forest and cokriging. Remote Sens. 13 (11), 2040. doi:10.3390/rs13112040
Yu, C., Hu, D. Y., Liu, M. Q., Wang, S. S., and Di, Y. F. (2020). Spatio-temporal accuracy evaluation of three high-resolution satellite precipitation products in China area. Atmos. Res. 241, 104952. doi:10.1016/j.atmosres.2020.104952
Yu, L. F., Leng, G. Y., and Python, A. (2022). A comprehensive validation for GPM IMERG precipitation products to detect extremes and drought over mainland China. Weather Clim. Extrem. 36, 100458. doi:10.1016/j.wace.2022.100458
Zhang, H. R., Zhang, J. N., Lv, Z. Z., Yao, L. J., Zhang, N., and Zhang, Q. (2023). Spatio-temporal assessment of landscape ecological risk and associated drivers: a case study of the Yellow River Basin in Inner Mongolia. Land 12, 1114. doi:10.3390/land12061114
Zhang, Q., Peng, J. T., Singh, V. P., Li, J. F., and Chen, Y. Q. D. (2014). Spatio-temporal variations of precipitation in arid and semiarid regions of China: the Yellow River basin as a case study. Glob. Planet. Change. 114, 38–49. doi:10.1016/j.gloplacha.2014.01.005
Zhang, Q., Shi, P. J., Singh, V. P., Fan, K. K., and Huang, J. J. (2017). Spatial downscaling of TRMM-based precipitation data using vegetative response in Xinjiang, China. Int. J. Climatol. 37, 3895–3909. doi:10.1002/joc.4964
Zhang, T., Li, B. L., Yuan, Y. C., Gao, X. Z., Sun, Q. L., Xu, L. L., et al. (2018). Spatial downscaling of TRMM precipitation data considering the impacts of macro-geographical factors and local elevation in the Three-River Headwaters Region. Remote Sens. Environ. 215, 109–127. doi:10.1016/j.rse.2018.06.004
Keywords: precipitation, GPM IMERG, MGWR, downscaling, scale difference
Citation: Tu L and Duan L (2024) Spatial downscaling analysis of GPM IMERG precipitation dataset based on multiscale geographically weighted regression model: a case study of the Inner Mongolia Reach of the Yellow River basin. Front. Environ. Sci. 12:1389587. doi: 10.3389/fenvs.2024.1389587
Received: 21 February 2024; Accepted: 20 March 2024;
Published: 09 April 2024.
Edited by:
Zhiming Han, Northwest A&F University, ChinaReviewed by:
Peng Sun, Anhui Normal University, ChinaNing Ma, Chinese Academy of Sciences (CAS), China
Zhaoqiang Zhou, Northeast Agricultural University, China
Copyright © 2024 Tu and Duan. This is an open-access article distributed under the terms of the Creative Commons Attribution License (CC BY). The use, distribution or reproduction in other forums is permitted, provided the original author(s) and the copyright owner(s) are credited and that the original publication in this journal is cited, in accordance with accepted academic practice. No use, distribution or reproduction is permitted which does not comply with these terms.
*Correspondence: Limin Duan, ZHVhbmxpbWluODIwMTE2QDE2My5jb20=